⚠️ 以下所有内容总结都来自于 大语言模型的能力,如有错误,仅供参考,谨慎使用
🔴 请注意:千万不要用于严肃的学术场景,只能用于论文阅读前的初筛!
💗 如果您觉得我们的项目对您有帮助 ChatPaperFree ,还请您给我们一些鼓励!⭐️ HuggingFace免费体验
2025-01-18 更新
Efficient Few-Shot Medical Image Analysis via Hierarchical Contrastive Vision-Language Learning
Authors:Harrison Fuller, Fernando Gabriela Garcia, Victor Flores
Few-shot learning in medical image classification presents a significant challenge due to the limited availability of annotated data and the complex nature of medical imagery. In this work, we propose Adaptive Vision-Language Fine-tuning with Hierarchical Contrastive Alignment (HiCA), a novel framework that leverages the capabilities of Large Vision-Language Models (LVLMs) for medical image analysis. HiCA introduces a two-stage fine-tuning strategy, combining domain-specific pretraining and hierarchical contrastive learning to align visual and textual representations at multiple levels. We evaluate our approach on two benchmark datasets, Chest X-ray and Breast Ultrasound, achieving state-of-the-art performance in both few-shot and zero-shot settings. Further analyses demonstrate the robustness, generalizability, and interpretability of our method, with substantial improvements in performance compared to existing baselines. Our work highlights the potential of hierarchical contrastive strategies in adapting LVLMs to the unique challenges of medical imaging tasks.
医疗图像分类中的小样本学习由于标注数据的有限性和医疗图像本身的复杂性,面临巨大挑战。在这项工作中,我们提出了自适应视觉语言微调与分层对比对齐(HiCA)的新框架,该框架利用大型视觉语言模型(LVLMs)进行医学图像分析。HiCA引入了两阶段微调策略,结合领域特定预训练和分层对比学习,在多个层次上对齐视觉和文本表示。我们在Chest X光片和乳腺超声两个基准数据集上评估了我们的方法,在少量样本和零样本设置下均达到了最先进的性能。进一步的分析表明了我们方法的稳健性、通用性和可解释性,与现有基线相比,性能有了显著的提升。我们的工作突出了分层对比策略在适应医疗成像任务的独特挑战时大型视觉语言模型的潜力。
论文及项目相关链接
Summary
适应于医学图像分类中的小样学习挑战的新方法——基于层次对比对齐的适应视觉语言微调(HiCA)。该方法利用大型视觉语言模型(LVLMs)进行医学图像分析,引入两阶段微调策略,结合领域特定预训练和层次对比学习,在多个层次上对齐视觉和文本表示。在胸部X光片和乳腺超声两个基准数据集上的评估结果表明,该方法在小样学习和零样本学习环境下均达到领先水平。进一步分析显示,该方法具有稳健性、通用性和可解释性,与现有基线相比性能显著提高。本研究突显了层次对比策略在适应医学成像任务独特挑战中的潜力。
Key Takeaways
- 医学图像分类中的小样学习面临挑战,因为标注数据有限且医学图像复杂。
- 提出了一种新的方法——HiCA,结合大型视觉语言模型(LVLMs)进行医学图像分析。
- HiCA采用两阶段微调策略,包括领域特定预训练和层次对比学习。
- 在Chest X-ray和Breast Ultrasound两个基准数据集上取得了领先水平。
- HiCA方法具有稳健性、通用性和可解释性。
- 与现有方法相比,HiCA在性能上有显著提高。
点此查看论文截图
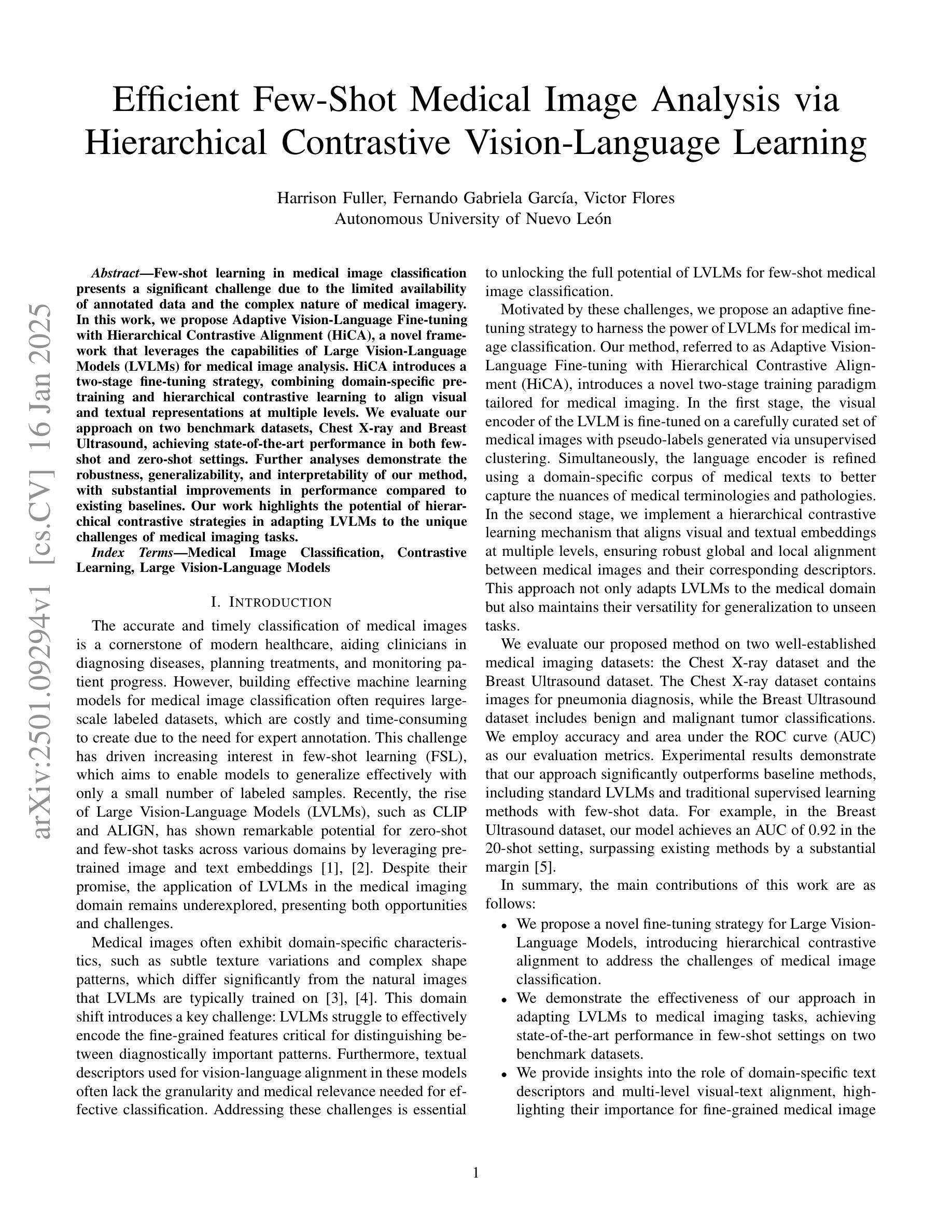
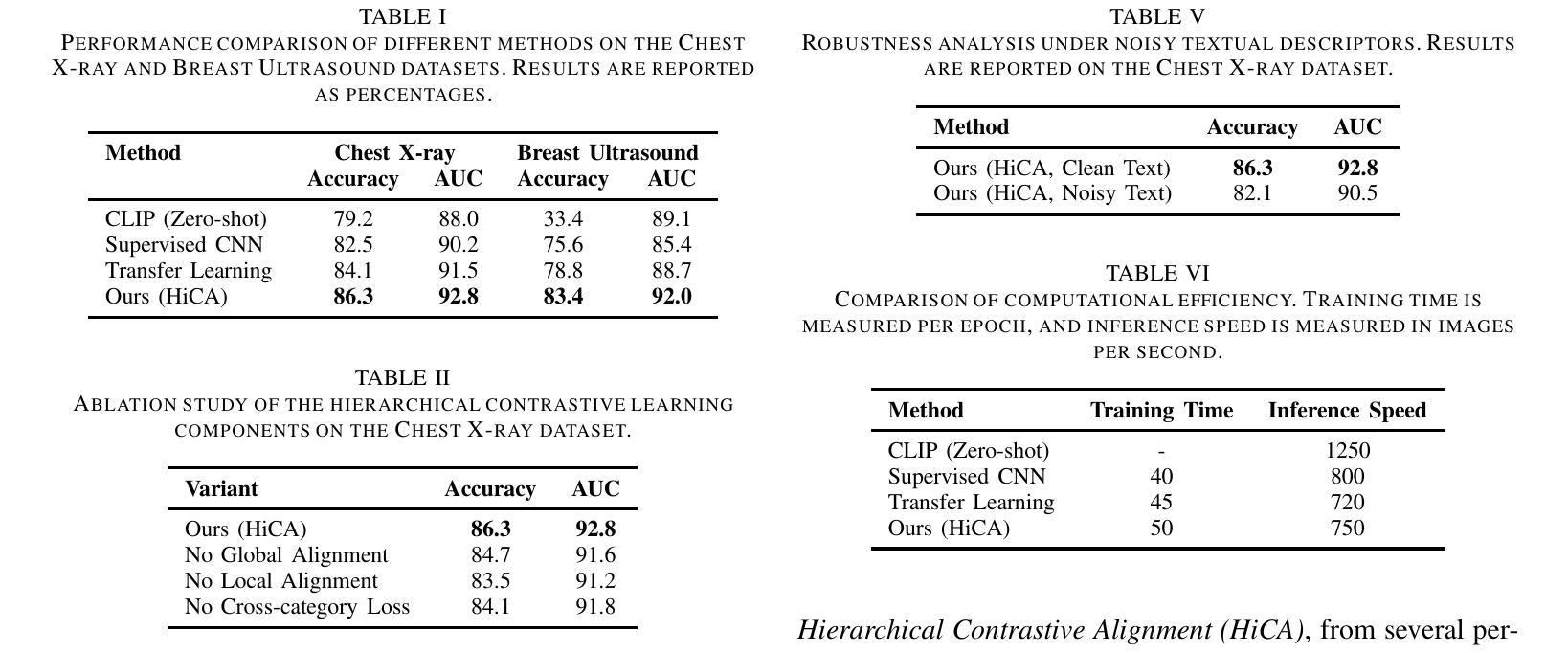
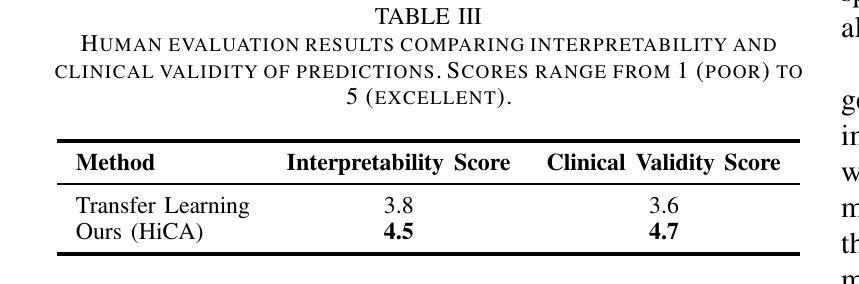
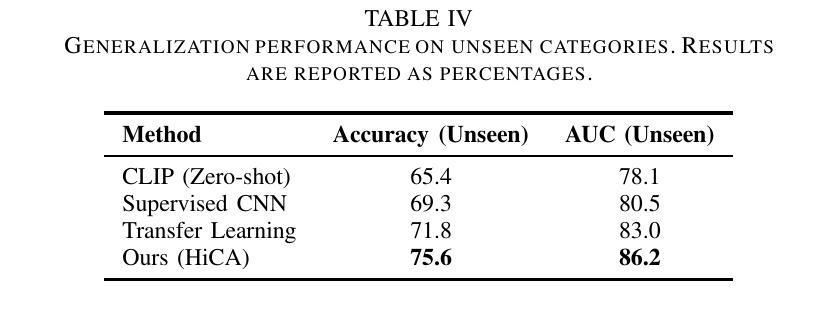