⚠️ 以下所有内容总结都来自于 大语言模型的能力,如有错误,仅供参考,谨慎使用
🔴 请注意:千万不要用于严肃的学术场景,只能用于论文阅读前的初筛!
💗 如果您觉得我们的项目对您有帮助 ChatPaperFree ,还请您给我们一些鼓励!⭐️ HuggingFace免费体验
2025-01-21 更新
Leveraging Confident Image Regions for Source-Free Domain-Adaptive Object Detection
Authors:Mohamed Lamine Mekhalfi, Davide Boscaini, Fabio Poiesi
Source-free domain-adaptive object detection is an interesting but scarcely addressed topic. It aims at adapting a source-pretrained detector to a distinct target domain without resorting to source data during adaptation. So far, there is no data augmentation scheme tailored to source-free domain-adaptive object detection. To this end, this paper presents a novel data augmentation approach that cuts out target image regions where the detector is confident, augments them along with their respective pseudo-labels, and joins them into a challenging target image to adapt the detector. As the source data is out of reach during adaptation, we implement our approach within a teacher-student learning paradigm to ensure that the model does not collapse during the adaptation procedure. We evaluated our approach on three adaptation benchmarks of traffic scenes, scoring new state-of-the-art on two of them.
无源域自适应目标检测是一个有趣但鲜有研究的话题。它的目标是将源预训练检测器适应到不同的目标域,而适应过程中不使用源数据。迄今为止,还没有针对无源域自适应目标检测定制的数据增强方案。为此,本文提出了一种新的数据增强方法,该方法会裁剪出目标图像区域中检测器确信的部分,连同各自的伪标签一起进行增强,并合并到一个具有挑战性的目标图像中,以适应检测器。由于适应过程中无法访问源数据,我们在教师-学生学习范式内实施我们的方法,以确保模型在适应过程中不会崩溃。我们在三个交通场景适配基准测试集上评估了我们的方法,并在其中两个上取得了最新状态。
论文及项目相关链接
Summary
源自由域自适应目标检测是一个备受关注但鲜有研究的话题。该研究旨在将一个源预训练的目标检测器适应到一个特定的目标域,且在不使用源数据的情况下进行适应。迄今为止,还没有针对源自由域自适应目标检测的数据增强方案。为此,本文提出了一种新型数据增强方法,该方法能够裁剪出目标图像中检测器信心十足的区域,按照它们的伪标签进行增强,并将它们融合成具有挑战性的目标图像以适应检测器。由于适应过程中无法获取源数据,我们在教师学生学习范式中实施此方法,以确保模型在适应过程中不会崩溃。我们在三个交通场景自适应基准测试上评估了我们的方法,并在其中两个上取得了最新状态。
Key Takeaways
- 源自由域自适应目标检测旨在将源预训练的目标检测器适应到特定的目标域,而无需使用源数据进行适应。
- 当前缺乏针对源自由域自适应目标检测的数据增强方法。
- 本文提出了一种新型数据增强方法,通过裁剪并增强目标图像中的关键区域来提高检测器的适应性。
- 该方法利用伪标签来标识目标图像中的关键区域。
- 在教师学生学习范式中实施此方法,以确保模型在适应过程中的稳定性。
- 在三个交通场景自适应基准测试中评估了该方法,表现出优异的性能。
点此查看论文截图
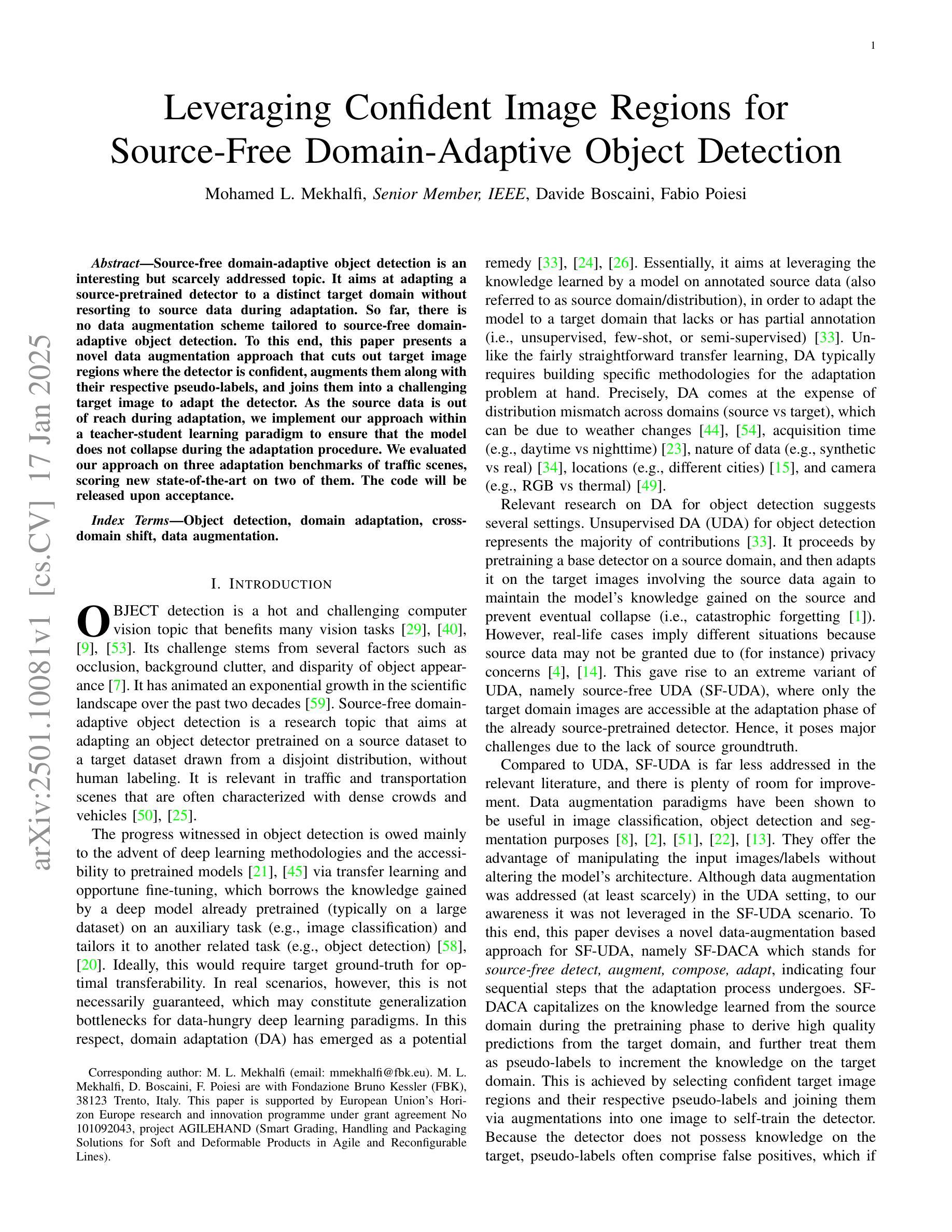
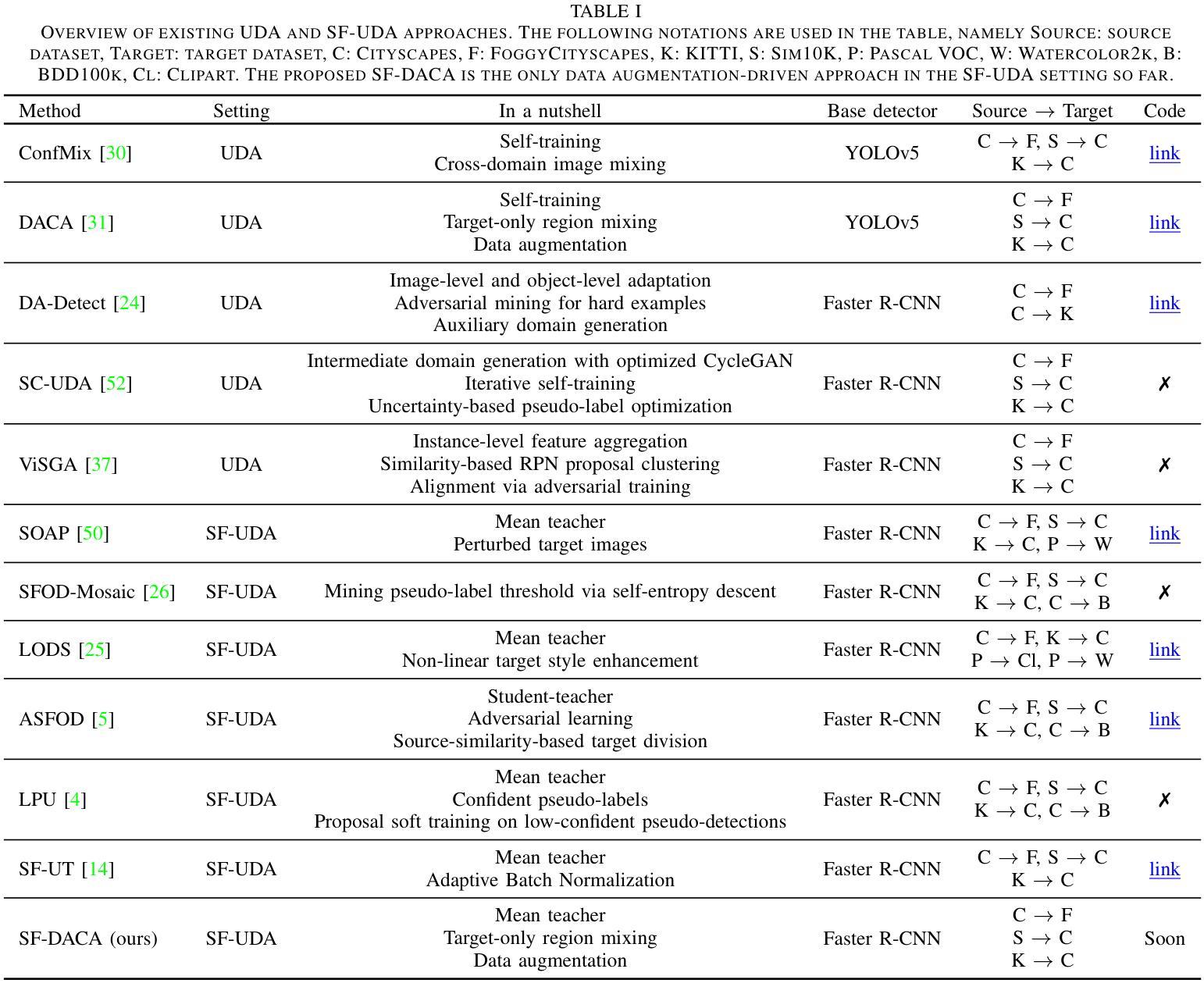
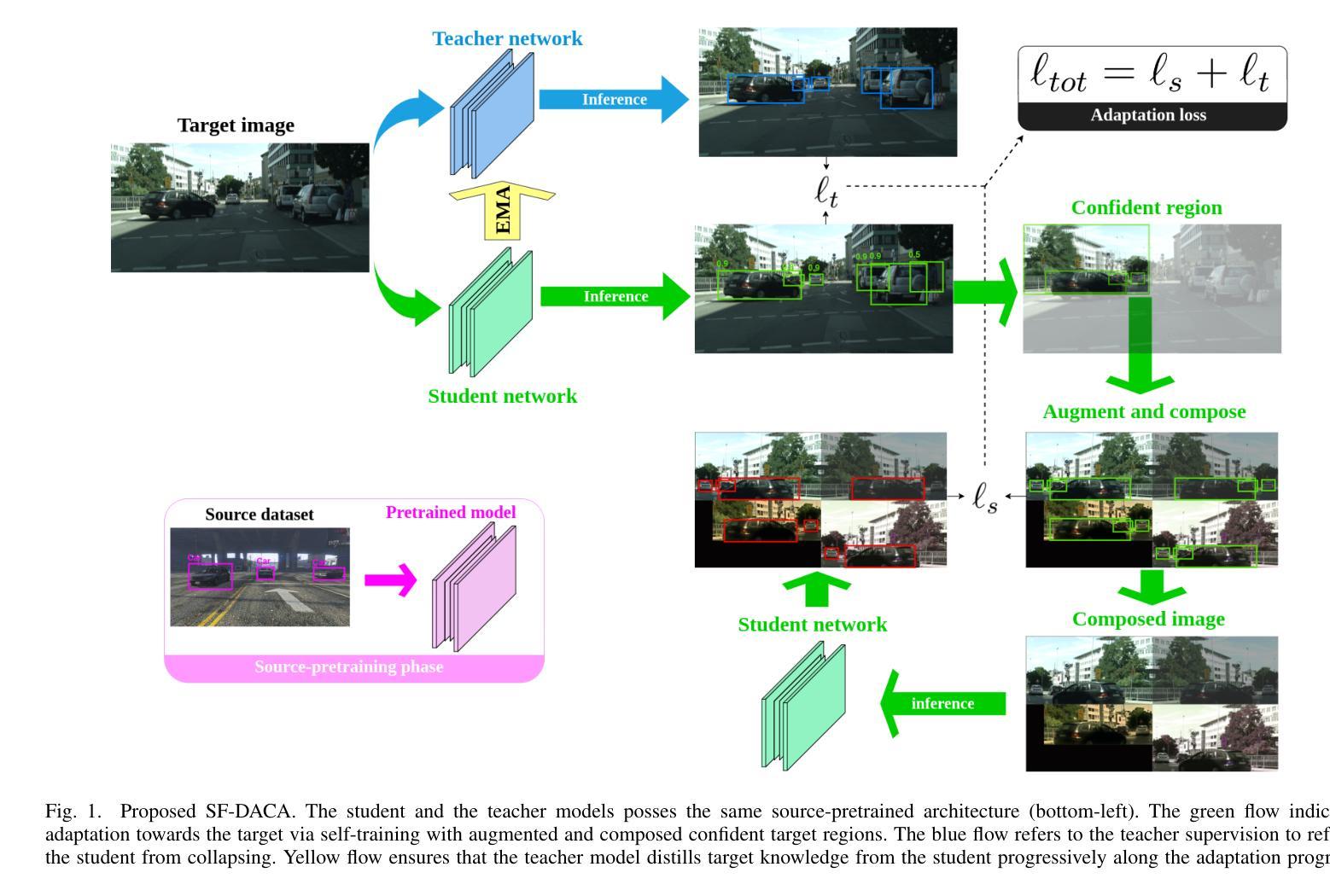
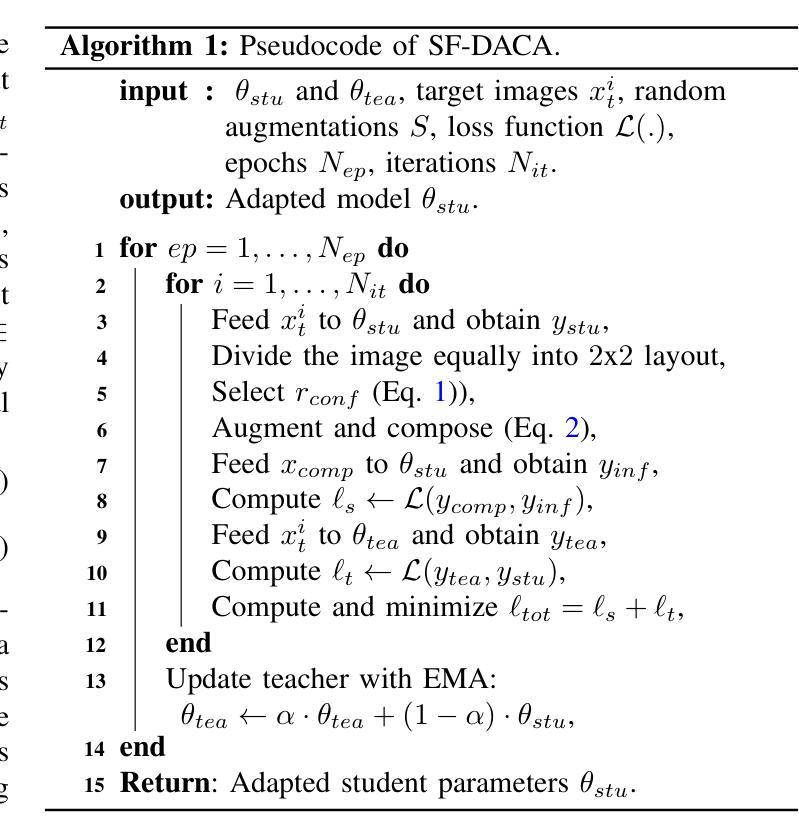
MoRe: Class Patch Attention Needs Regularization for Weakly Supervised Semantic Segmentation
Authors:Zhiwei Yang, Yucong Meng, Kexue Fu, Shuo Wang, Zhijian Song
Weakly Supervised Semantic Segmentation (WSSS) with image-level labels typically uses Class Activation Maps (CAM) to achieve dense predictions. Recently, Vision Transformer (ViT) has provided an alternative to generate localization maps from class-patch attention. However, due to insufficient constraints on modeling such attention, we observe that the Localization Attention Maps (LAM) often struggle with the artifact issue, i.e., patch regions with minimal semantic relevance are falsely activated by class tokens. In this work, we propose MoRe to address this issue and further explore the potential of LAM. Our findings suggest that imposing additional regularization on class-patch attention is necessary. To this end, we first view the attention as a novel directed graph and propose the Graph Category Representation module to implicitly regularize the interaction among class-patch entities. It ensures that class tokens dynamically condense the related patch information and suppress unrelated artifacts at a graph level. Second, motivated by the observation that CAM from classification weights maintains smooth localization of objects, we devise the Localization-informed Regularization module to explicitly regularize the class-patch attention. It directly mines the token relations from CAM and further supervises the consistency between class and patch tokens in a learnable manner. Extensive experiments are conducted on PASCAL VOC and MS COCO, validating that MoRe effectively addresses the artifact issue and achieves state-of-the-art performance, surpassing recent single-stage and even multi-stage methods. Code is available at https://github.com/zwyang6/MoRe.
弱监督语义分割(WSSS)通常使用图像级别的标签和类别激活图(CAM)来实现密集预测。最近,视觉转换器(ViT)提供了一种从类别补丁注意力生成定位图的替代方案。然而,由于对这类注意力的建模约束不足,我们观察到定位注意力图(LAM)经常面临伪影问题,即语义相关性极小的补丁区域会被类别标记错误激活。在这项工作中,我们提出MoRe来解决这个问题,并进一步研究LAM的潜力。我们的研究结果表明,对类别补丁注意力施加额外的正则化是必要的。为此,我们首先将注意力视为一种新型的有向图,并提出图类别表示模块,以隐含地正则化类别补丁实体之间的交互。它确保类别标记动态地凝聚相关补丁信息,并在图级别上抑制不相关的伪影。其次,受分类权重CAM能够保持对象定位平滑的启发,我们设计了定位感知正则化模块,以显式地正则化类别补丁注意力。它直接从CAM挖掘标记关系,并以可学习的方式监督类别和补丁标记之间的一致性。在PASCAL VOC和MS COCO上进行了大量实验,验证了MoRe有效地解决了伪影问题,并实现了最先进的性能,超越了最近的单阶段甚至多阶段方法。代码可在https://github.com/zwyang6/MoRe找到。
论文及项目相关链接
PDF AAAI 2025
Summary
在图像级别的标签下,基于弱监督语义分割(WSSS)通常使用类激活图(CAM)来实现密集预测。然而,由于缺乏对类注意力建模的足够约束,定位注意力图(LAM)常常存在伪像问题。为解决这一问题并进一步探索LAM的潜力,本文提出了MoRe方法。该方法首先对类-补丁注意力施加额外的正则化约束。将注意力视为新型的有向图,并提出图类别表示模块,确保类标记动态凝聚相关补丁信息并抑制无关伪像。此外,受分类权重中的CAM保持对象定位平滑的启发,开发了定位感知正则化模块来明确正则化类-补丁注意力。该方法直接从CAM挖掘标记关系并学习监督类标记与补丁标记的一致性。在PASCAL VOC和MS COCO上的大量实验验证,MoRe有效解决了伪像问题并取得了最新的最佳性能。
Key Takeaways
- WSSS通常使用CAM实现密集预测,但存在伪像问题。
- MoRe方法通过额外的正则化约束解决LAM的伪像问题。
- 提出图类别表示模块,将注意力视为有向图来处理类-补丁关系。
- 利用定位感知正则化模块来明确正则化类-补丁注意力,从CAM中学习监督类与补丁标记的一致性。
- MoRe在PASCAL VOC和MS COCO上取得了最新的最佳性能。
点此查看论文截图
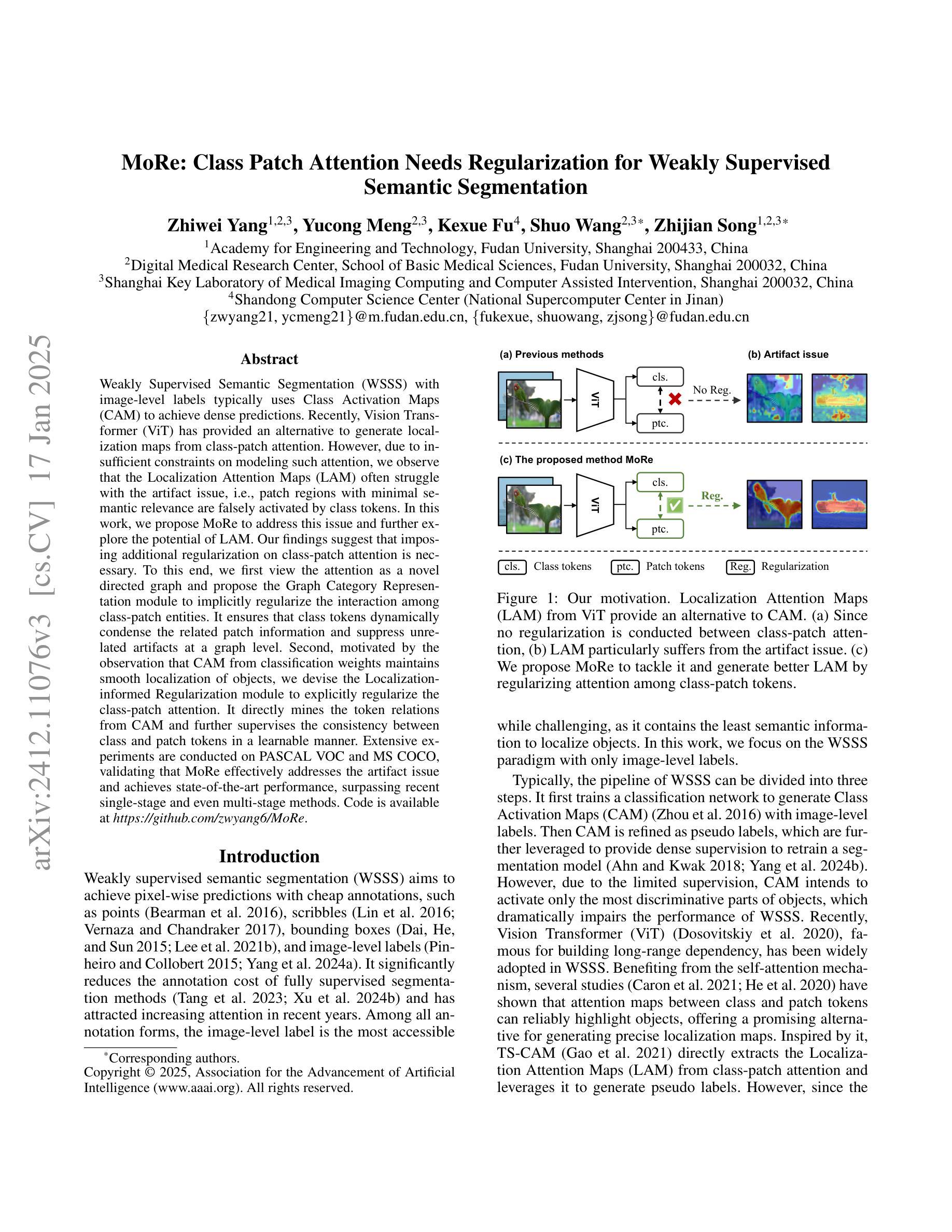
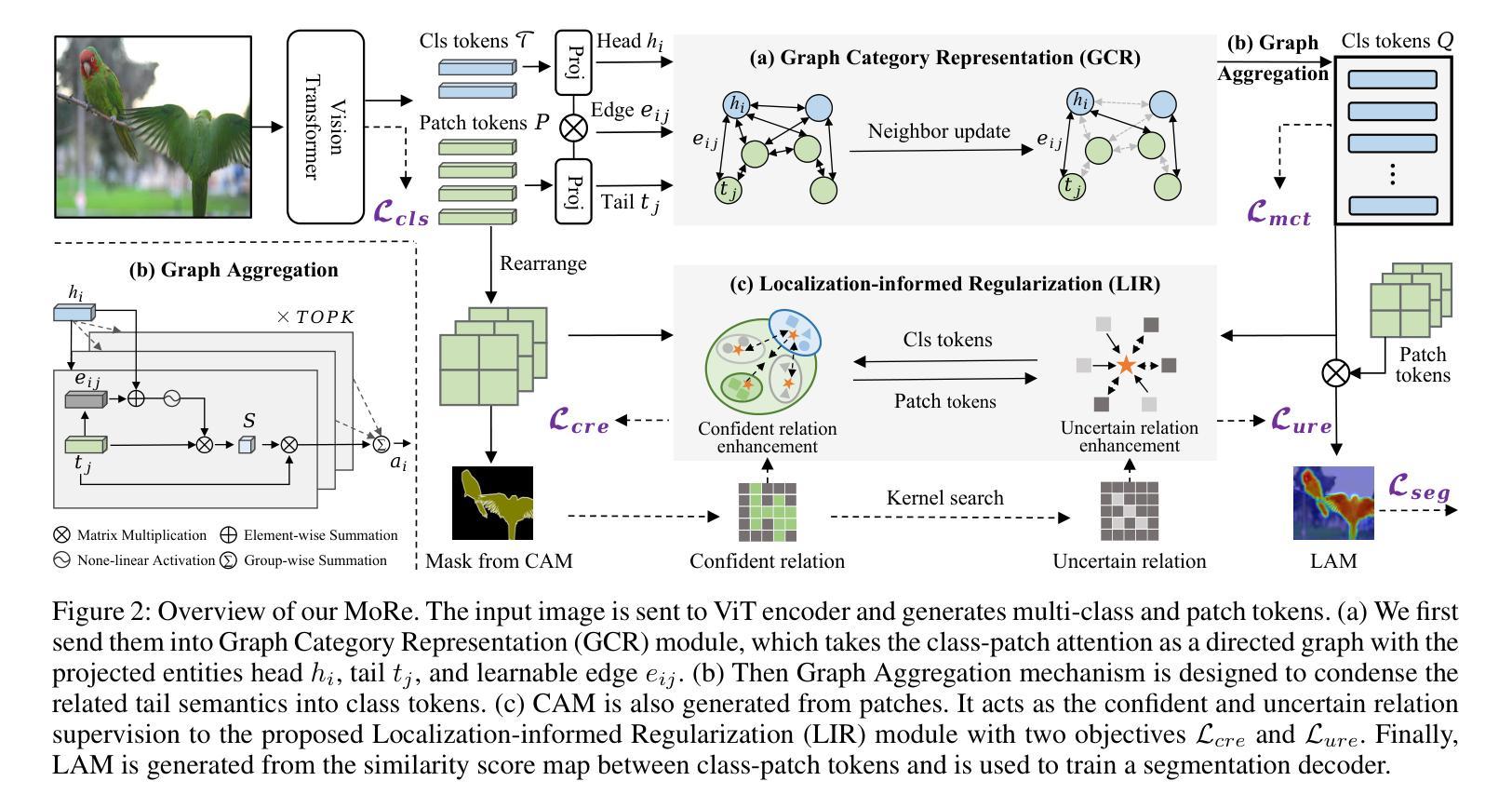
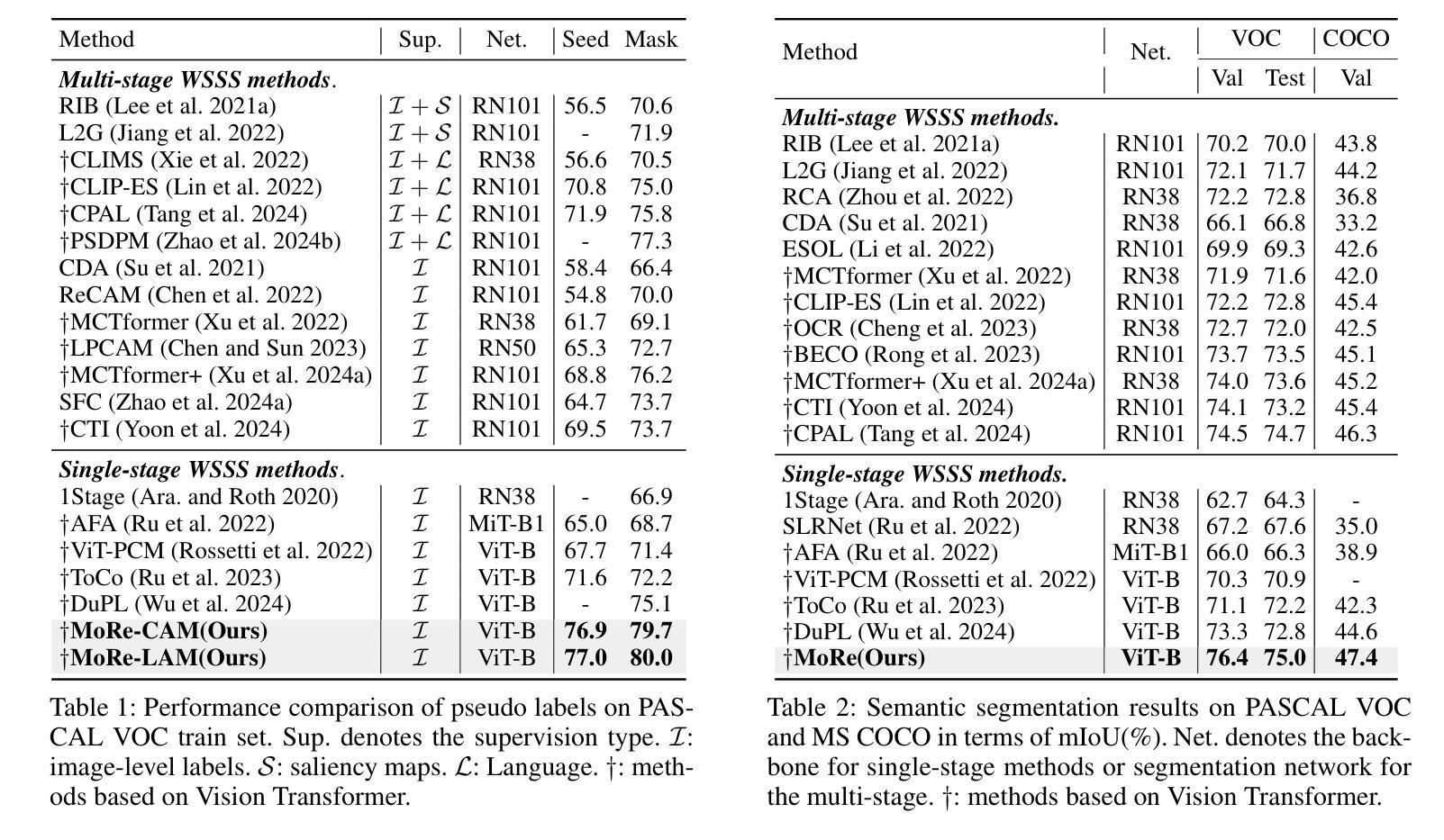
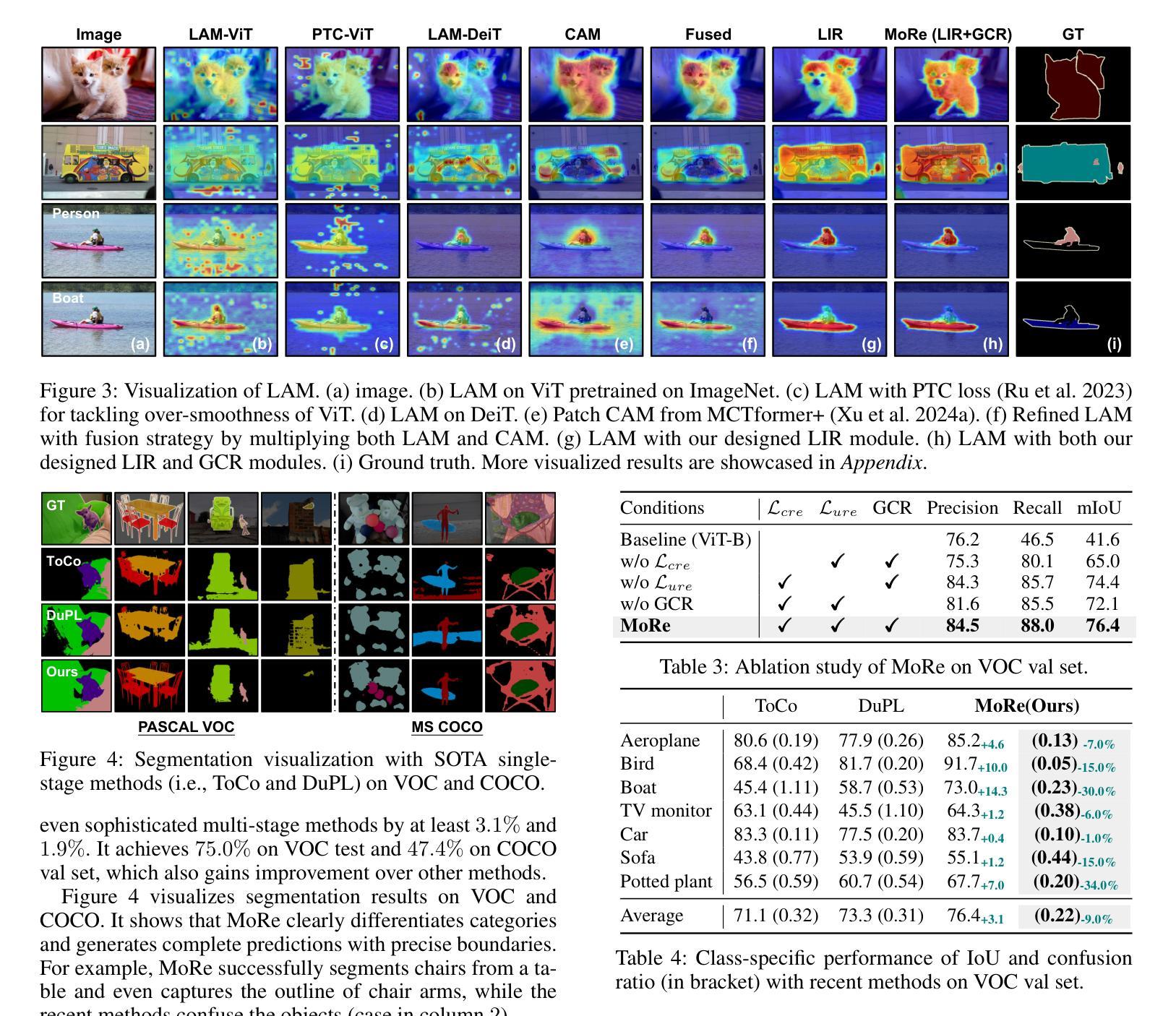