⚠️ 以下所有内容总结都来自于 大语言模型的能力,如有错误,仅供参考,谨慎使用
🔴 请注意:千万不要用于严肃的学术场景,只能用于论文阅读前的初筛!
💗 如果您觉得我们的项目对您有帮助 ChatPaperFree ,还请您给我们一些鼓励!⭐️ HuggingFace免费体验
2025-01-21 更新
DiffStereo: High-Frequency Aware Diffusion Model for Stereo Image Restoration
Authors:Huiyun Cao, Yuan Shi, Bin Xia, Xiaoyu Jin, Wenming Yang
Diffusion models (DMs) have achieved promising performance in image restoration but haven’t been explored for stereo images. The application of DM in stereo image restoration is confronted with a series of challenges. The need to reconstruct two images exacerbates DM’s computational cost. Additionally, existing latent DMs usually focus on semantic information and remove high-frequency details as redundancy during latent compression, which is precisely what matters for image restoration. To address the above problems, we propose a high-frequency aware diffusion model, DiffStereo for stereo image restoration as the first attempt at DM in this domain. Specifically, DiffStereo first learns latent high-frequency representations (LHFR) of HQ images. DM is then trained in the learned space to estimate LHFR for stereo images, which are fused into a transformer-based stereo image restoration network providing beneficial high-frequency information of corresponding HQ images. The resolution of LHFR is kept the same as input images, which preserves the inherent texture from distortion. And the compression in channels alleviates the computational burden of DM. Furthermore, we devise a position encoding scheme when integrating the LHFR into the restoration network, enabling distinctive guidance in different depths of the restoration network. Comprehensive experiments verify that by combining generative DM and transformer, DiffStereo achieves both higher reconstruction accuracy and better perceptual quality on stereo super-resolution, deblurring, and low-light enhancement compared with state-of-the-art methods.
扩散模型(DM)在图像修复方面表现优异,但在立体图像修复领域尚未得到广泛应用。扩散模型应用于立体图像修复面临一系列挑战。重建两个图像的需求加剧了扩散模型的计算成本。此外,现有的潜在扩散模型通常关注语义信息,并在潜在压缩过程中去除高频细节,而这正是图像修复所重视的。为了解决上述问题,我们首次尝试在立体图像修复领域引入扩散模型,提出一种高频感知扩散模型——DiffStereo。具体来说,DiffStereo首先学习高质量图像(HQ)的潜在高频表示(LHFR)。然后,在所学空间内训练扩散模型以估计立体图像的LHFR,并将其融合到基于变压器的立体图像修复网络中,为对应的高质量图像提供有益的高频信息。LHFR的分辨率保持与输入图像相同,保留了原始纹理无失真。同时通道压缩减轻了扩散模型的计算负担。此外,当将LHFR集成到修复网络时,我们设计了一种位置编码方案,在修复网络的不同深度提供独特的指导。综合实验证明,通过结合生成性扩散模型和变压器,DiffStereo在立体超分辨率、去模糊和低光增强方面实现了更高的重建精度和更好的感知质量,相较于其他先进方法具有优势。
论文及项目相关链接
PDF 9 pages, 6 figures
Summary
本文提出了一个名为DiffStereo的高频感知扩散模型,用于立体图像恢复。该模型首次尝试将扩散模型应用于该领域,解决了立体图像恢复中面临的挑战。通过学习高质量图像的高频表示,DiffStereo训练扩散模型估计立体图像的高频表示,然后将其融合到基于变换的图像恢复网络中,从而提供高质量图像的有益高频信息。该模型通过保持高频表示的分辨率与输入图像相同,保留了固有的纹理,并通过减少通道压缩减轻了扩散模型的计算负担。实验证明,结合生成扩散模型和变换器,DiffStereo在立体超分辨率、去模糊和低光增强方面实现了较高的重建精度和更好的感知质量。
Key Takeaways
- 扩散模型在图像恢复领域取得了显著的进展,但尚未在立体图像恢复中得到应用。
- 首次提出名为DiffStereo的高频感知扩散模型,应用于立体图像恢复。
- DiffStereo学习高质量图像的高频表示并将其应用于估计立体图像的高频信息。
- 通过将高频表示融合到基于变换的图像恢复网络中,DiffStereo提高了图像的感知质量。
- DiffStereo通过保持高频表示的分辨率与输入图像相同,保留了纹理细节,同时降低了计算成本。
- 结合生成扩散模型和变换器技术,DiffStereo在立体图像恢复的多个任务上取得了显著的成果。
点此查看论文截图
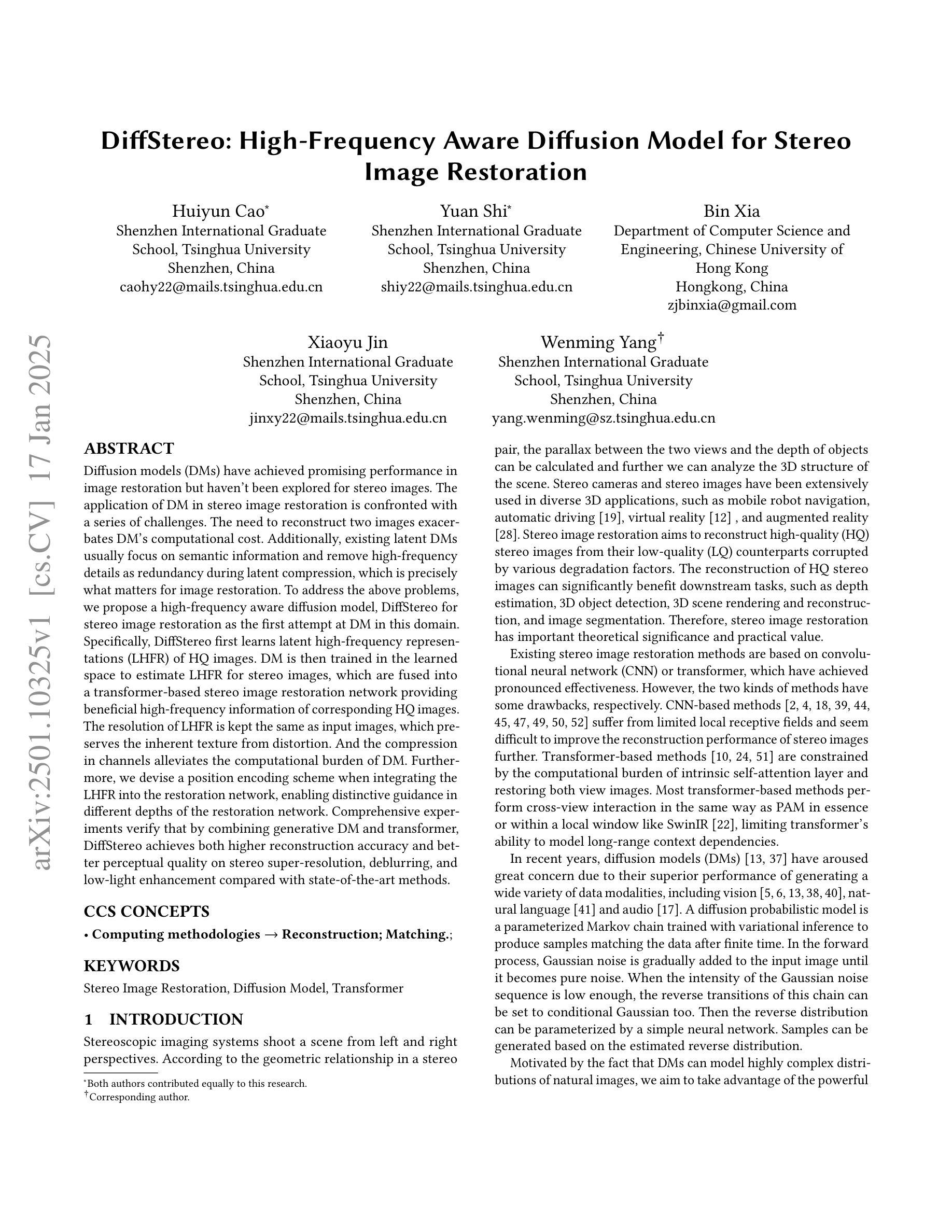
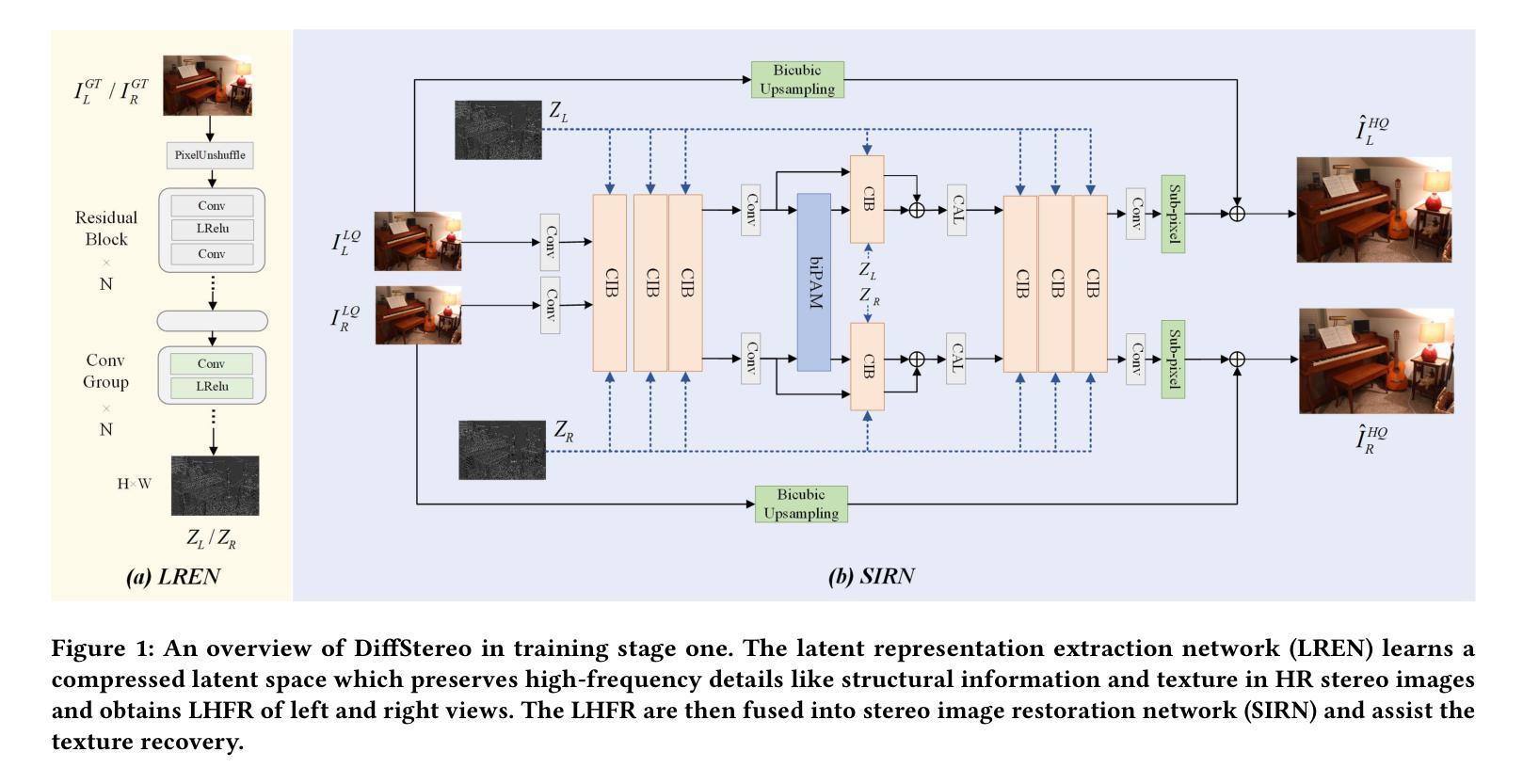
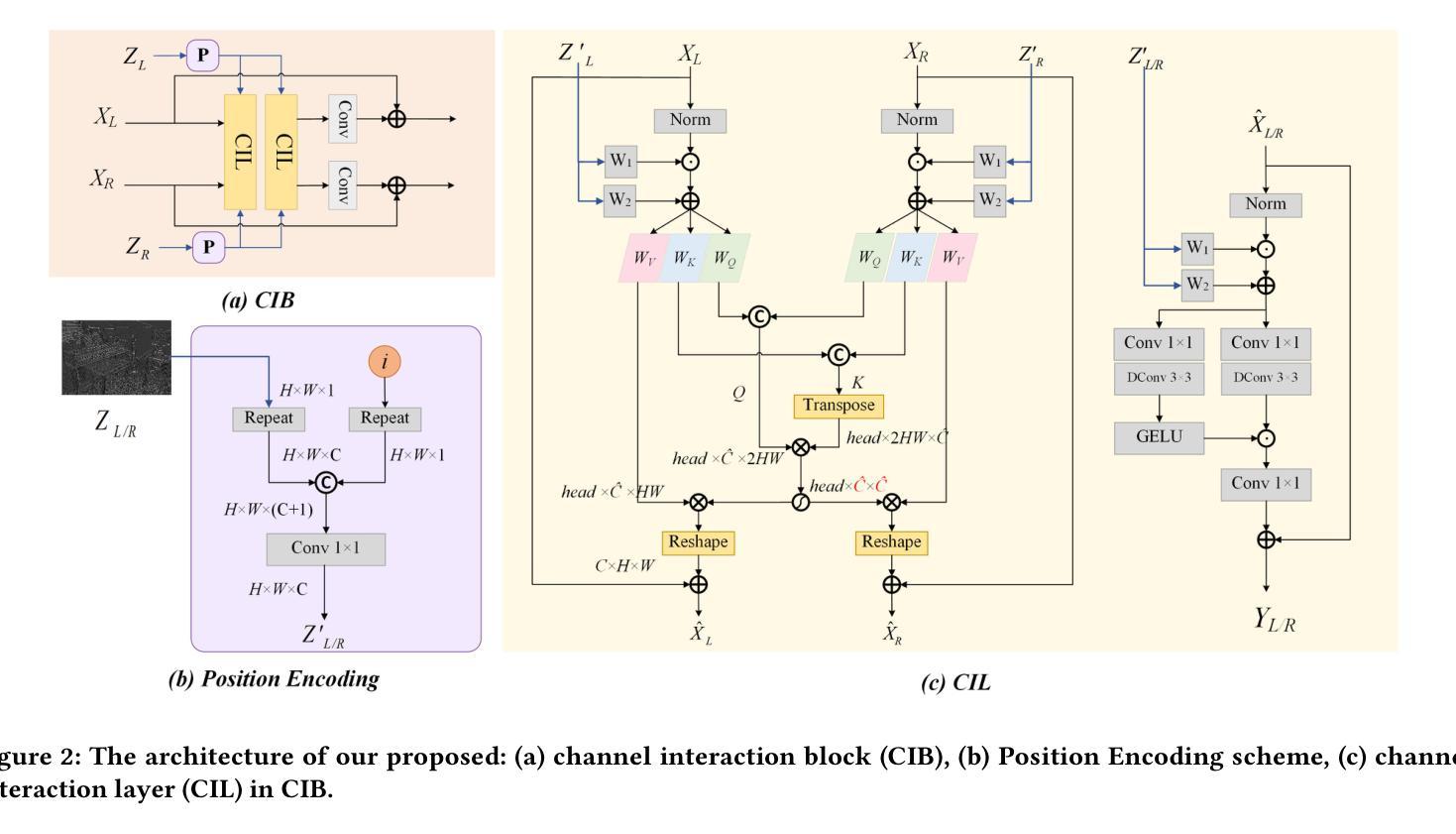
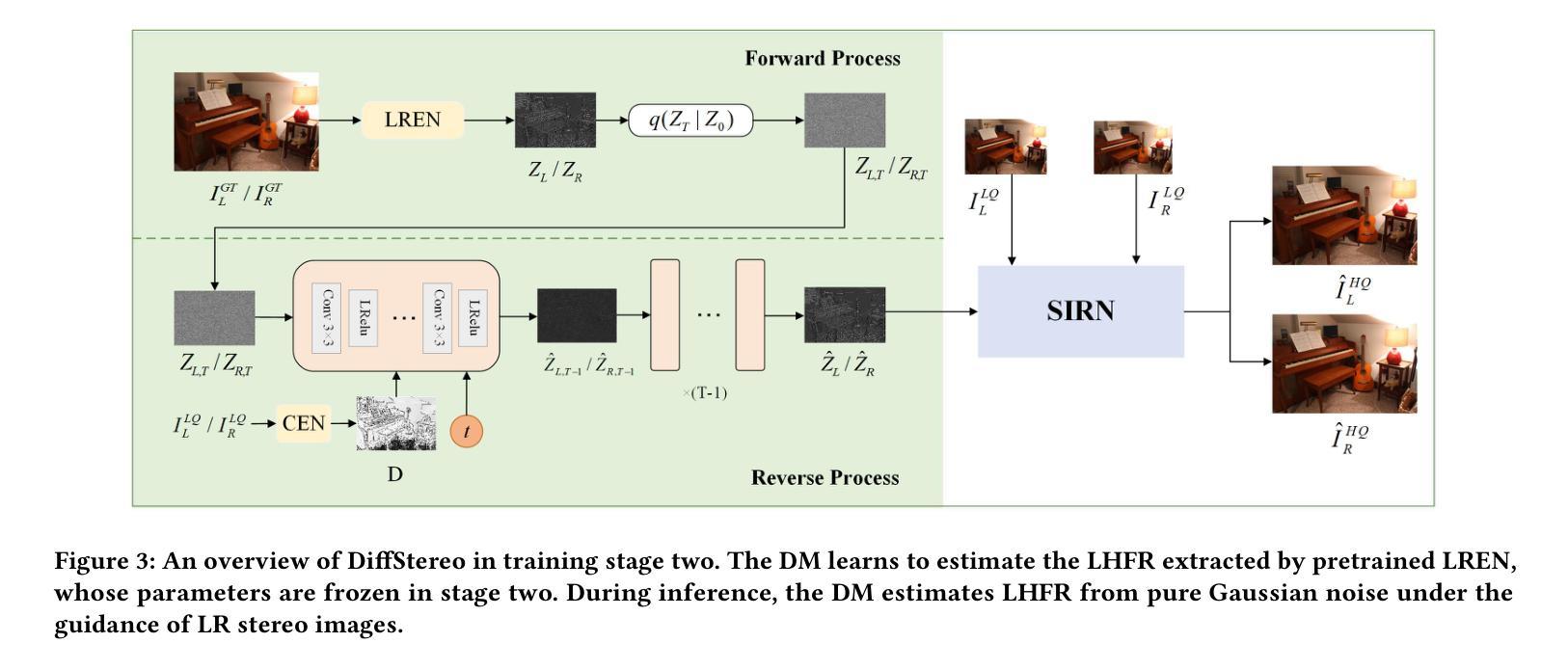
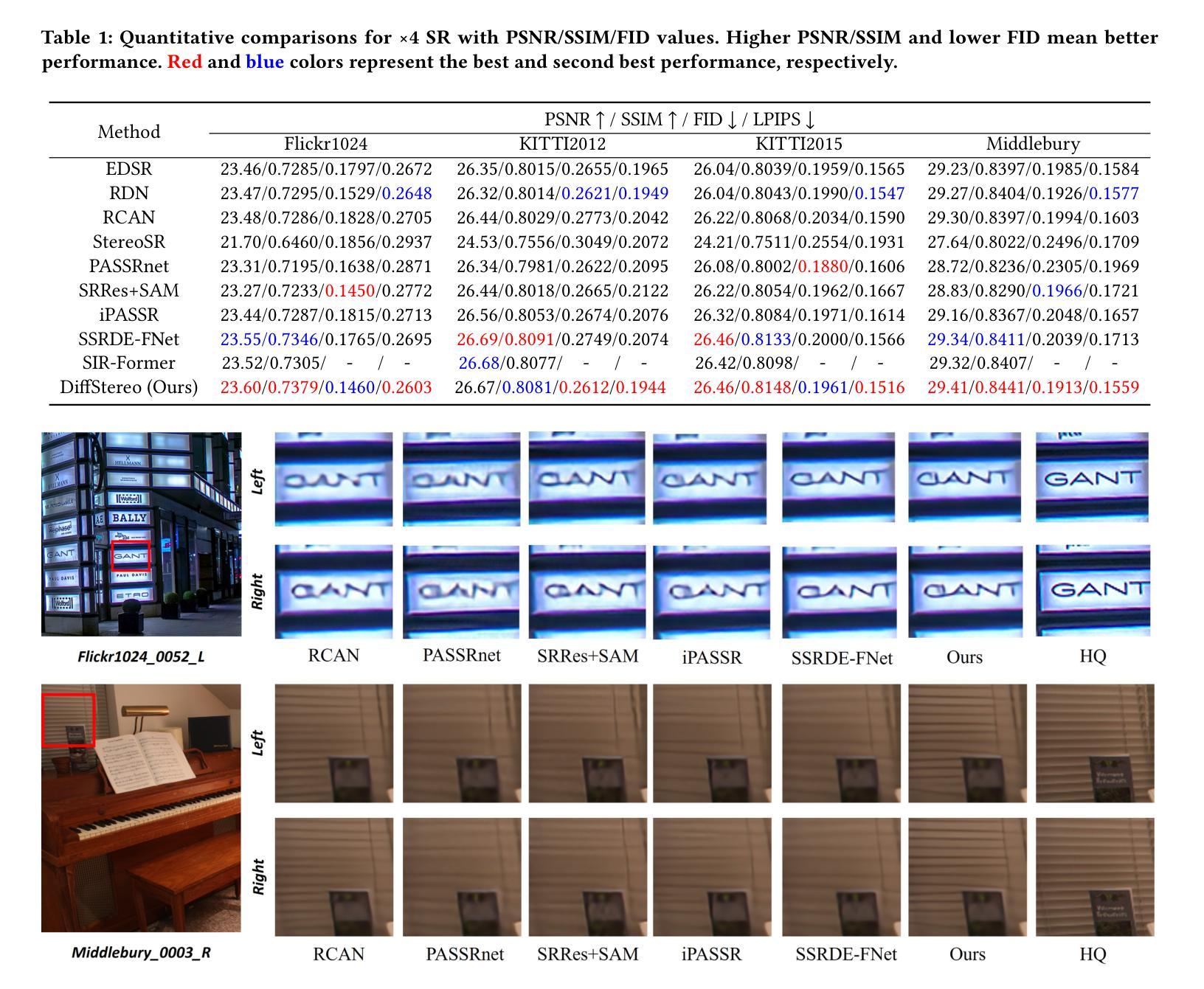
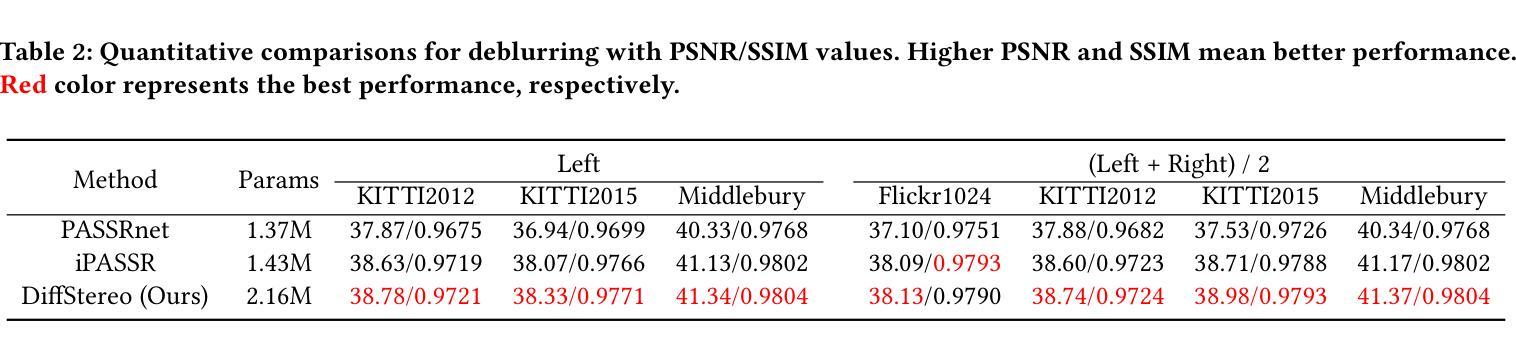
DiffuEraser: A Diffusion Model for Video Inpainting
Authors:Xiaowen Li, Haolan Xue, Peiran Ren, Liefeng Bo
Recent video inpainting algorithms integrate flow-based pixel propagation with transformer-based generation to leverage optical flow for restoring textures and objects using information from neighboring frames, while completing masked regions through visual Transformers. However, these approaches often encounter blurring and temporal inconsistencies when dealing with large masks, highlighting the need for models with enhanced generative capabilities. Recently, diffusion models have emerged as a prominent technique in image and video generation due to their impressive performance. In this paper, we introduce DiffuEraser, a video inpainting model based on stable diffusion, designed to fill masked regions with greater details and more coherent structures. We incorporate prior information to provide initialization and weak conditioning,which helps mitigate noisy artifacts and suppress hallucinations. Additionally, to improve temporal consistency during long-sequence inference, we expand the temporal receptive fields of both the prior model and DiffuEraser, and further enhance consistency by leveraging the temporal smoothing property of Video Diffusion Models. Experimental results demonstrate that our proposed method outperforms state-of-the-art techniques in both content completeness and temporal consistency while maintaining acceptable efficiency.
最近的视频补全算法结合了基于流的像素传播和基于转换器的生成,利用光学流恢复纹理和对象,同时使用来自相邻帧的信息,同时通过视觉转换器完成遮罩区域。然而,这些方法在处理大遮罩时经常遇到模糊和时间不一致的问题,这突显了需要具有增强生成能力的模型。最近,扩散模型因其令人印象深刻的性能而成为图像和视频生成中的一项重要技术。在本文中,我们介绍了基于稳定扩散的DiffuEraser视频补全模型,旨在以更高的细节和更连贯的结构填充遮罩区域。我们结合先验信息提供初始化和弱条件设置,这有助于减少噪声伪影并抑制幻觉。此外,为了提高长序列推理期间的时序一致性,我们扩大了先验模型和DiffuEraser的时间感受野,并借助视频扩散模型的时序平滑属性进一步提高了一致性。实验结果表明,我们提出的方法在内容完整性和时间一致性方面优于现有技术,同时保持可接受的效率。
论文及项目相关链接
PDF 11pages, 13figures
Summary
基于稳定扩散的DiffuEraser视频修复模型能够有效填补遮挡区域,细节更丰富且结构更连贯。该模型利用先验信息初始化并提供弱条件,减少噪声伪影并抑制幻觉。同时,通过扩大时间接收域并利用视频扩散模型的时序平滑特性,提高了长序列推理的时空一致性。实验结果证明,该方法在内容完整性和时序一致性方面优于现有技术,同时保持了可接受的效率。
Key Takeaways
- DiffuEraser结合流传播的像素和基于转换器的生成方法恢复纹理和对象。
- 当前方法在遇到大遮罩时可能出现模糊和时序不一致的问题。
- 扩散模型在图像和视频生成方面的突出表现使其成为理想选择。
- DiffuEraser利用先验信息进行初始化和弱条件化,减少噪声伪影和抑制幻觉。
- 通过扩大时间接收域提高时序一致性。
- 利用视频扩散模型的时序平滑特性进一步增强一致性。
点此查看论文截图
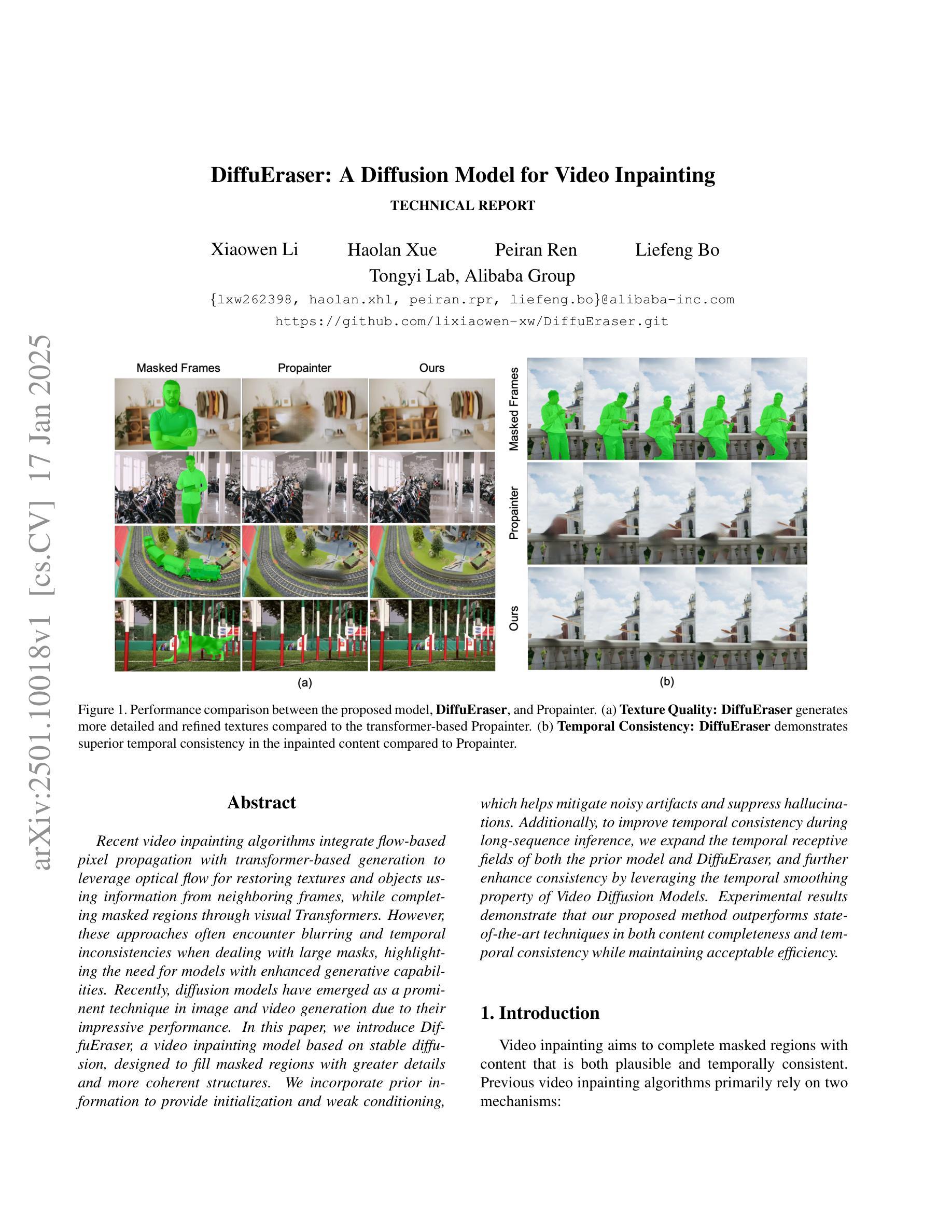
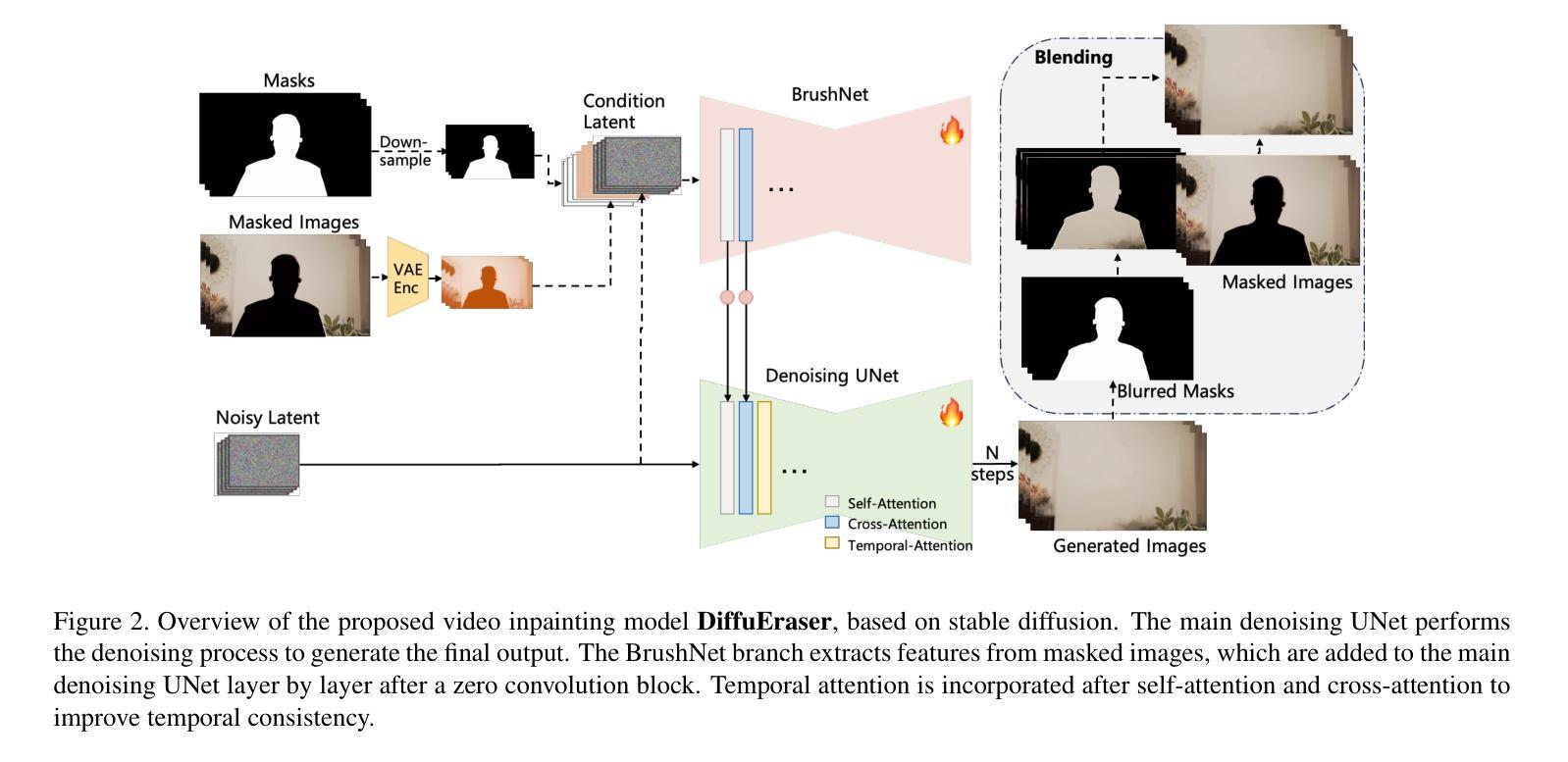
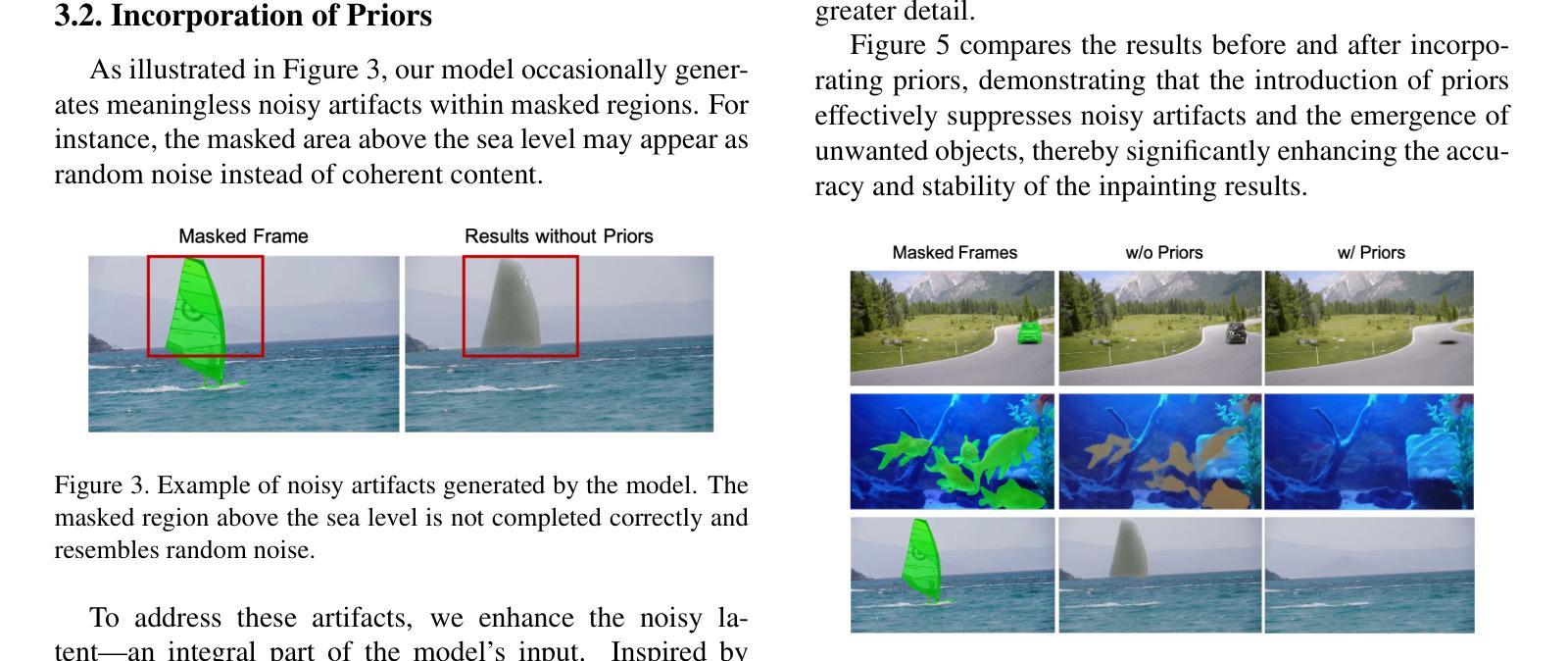
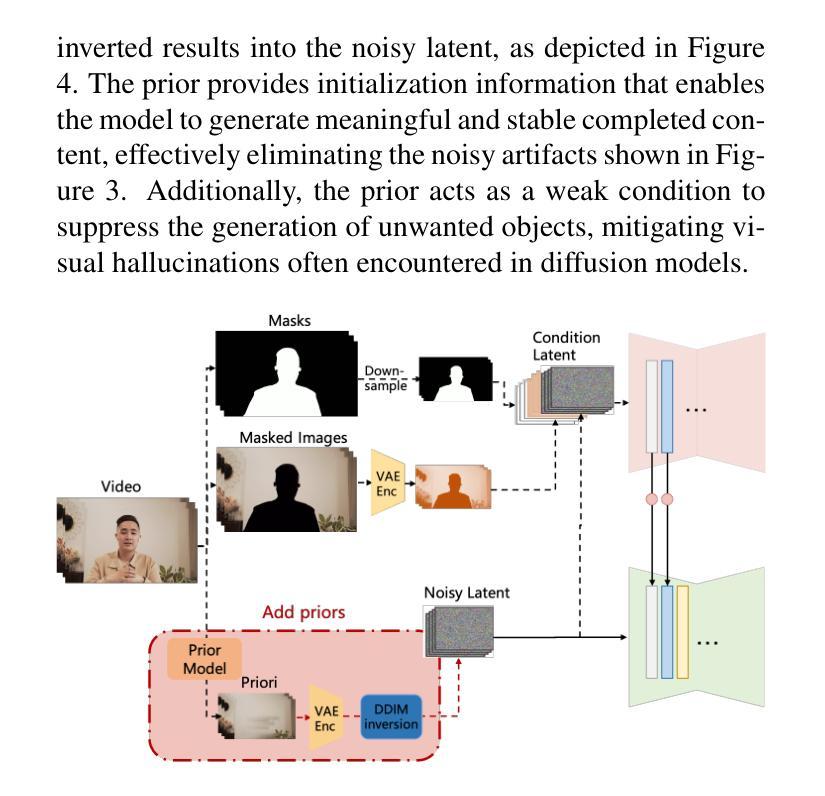
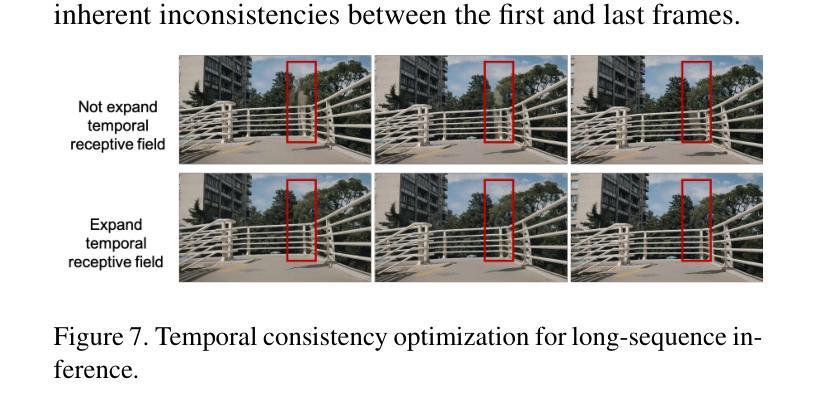
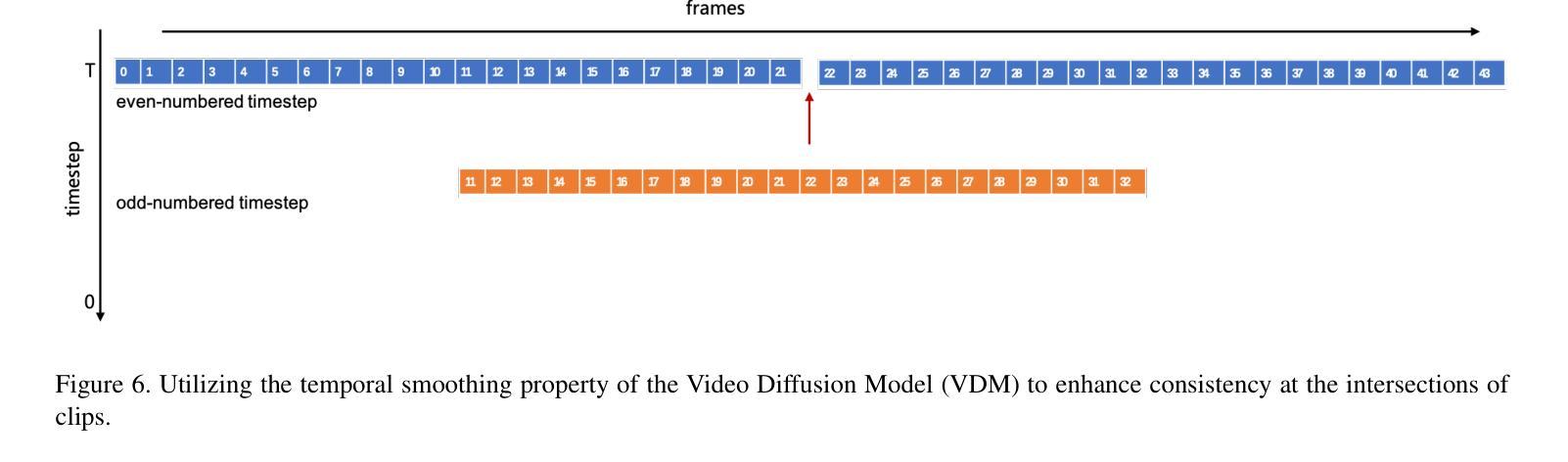
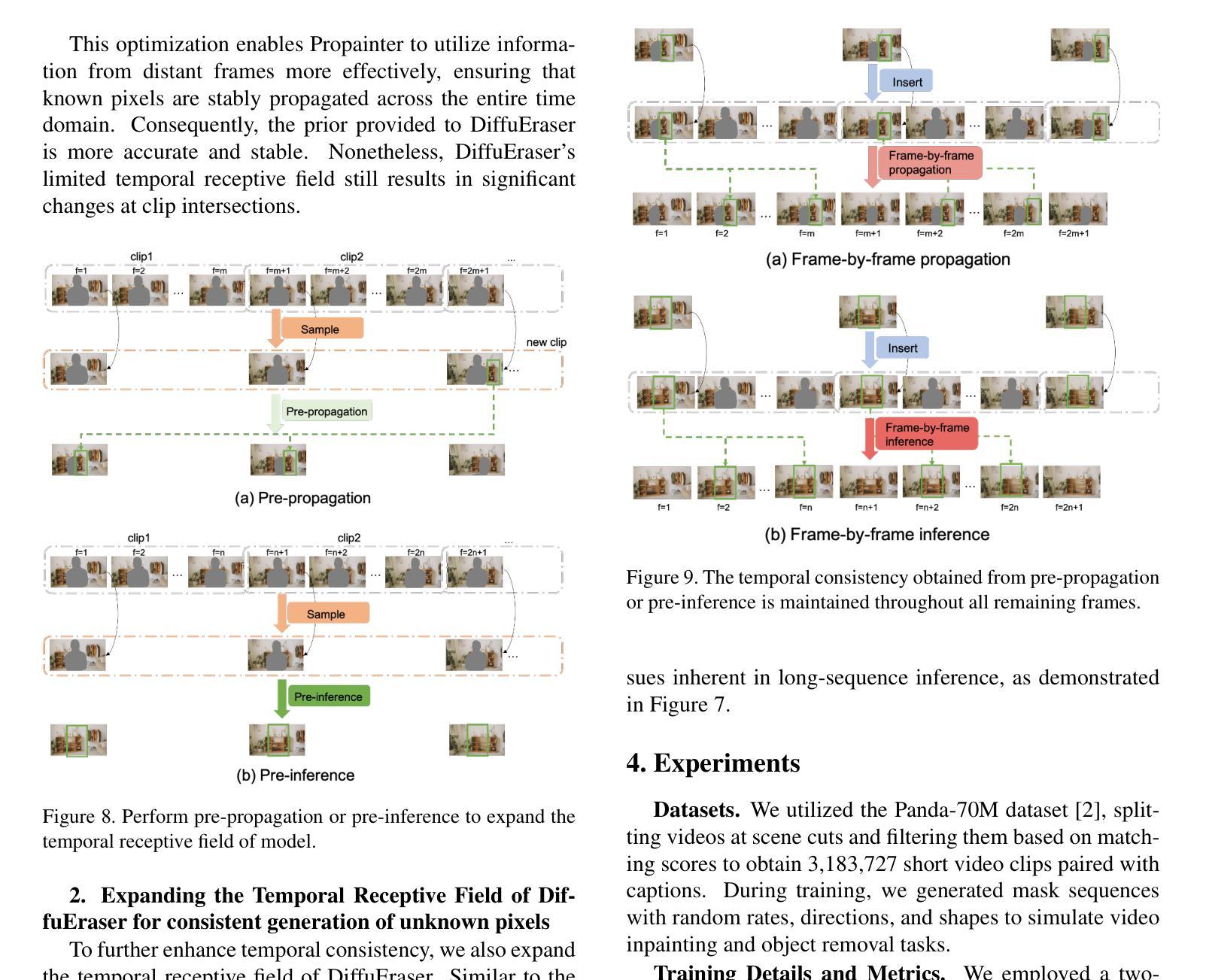
Physics-informed DeepCT: Sinogram Wavelet Decomposition Meets Masked Diffusion
Authors:Zekun Zhou, Tan Liu, Bing Yu, Yanru Gong, Liu Shi, Qiegen Liu
Diffusion model shows remarkable potential on sparse-view computed tomography (SVCT) reconstruction. However, when a network is trained on a limited sample space, its generalization capability may be constrained, which degrades performance on unfamiliar data. For image generation tasks, this can lead to issues such as blurry details and inconsistencies between regions. To alleviate this problem, we propose a Sinogram-based Wavelet random decomposition And Random mask diffusion Model (SWARM) for SVCT reconstruction. Specifically, introducing a random mask strategy in the sinogram effectively expands the limited training sample space. This enables the model to learn a broader range of data distributions, enhancing its understanding and generalization of data uncertainty. In addition, applying a random training strategy to the high-frequency components of the sinogram wavelet enhances feature representation and improves the ability to capture details in different frequency bands, thereby improving performance and robustness. Two-stage iterative reconstruction method is adopted to ensure the global consistency of the reconstructed image while refining its details. Experimental results demonstrate that SWARM outperforms competing approaches in both quantitative and qualitative performance across various datasets.
扩散模型在稀疏视图计算机断层扫描(SVCT)重建中显示出显著潜力。然而,当网络在有限的样本空间上进行训练时,其泛化能力可能会受到限制,导致在陌生数据上的性能下降。对于图像生成任务,这可能会导致细节模糊以及区域间的不一致性等问题。为了缓解这一问题,我们提出了基于辛氏图的小波随机分解和随机掩膜扩散模型(SWARM),用于SVCT重建。具体而言,在辛氏图中引入随机掩膜策略,有效地扩大了有限的训练样本空间。这使模型能够学习更广泛的数据分布,增强了对数据不确定性的理解和泛化。此外,对辛氏图小波的高频成分应用随机训练策略,增强了特征表示,提高了捕获不同频段细节的能力,从而提高了性能和稳健性。采用两阶段迭代重建方法,确保重建图像的整体一致性,同时细化其细节。实验结果表明,SWARM在不同数据集上的定量和定性性能均优于其他方法。
论文及项目相关链接
Summary:扩散模型在稀疏视角计算机断层扫描(SVCT)重建中显示出显著潜力,但在有限样本空间训练的网络在陌生数据上的表现可能会受到限制。为解决图像生成任务中细节模糊和区域间不一致等问题,我们提出了基于辛氏图小波随机分解和随机掩膜扩散模型(SWARM)的SVCT重建方法。通过辛氏图中引入随机掩膜策略有效扩展了有限的训练样本空间,提高了模型对数据不确定性的理解和泛化能力。此外,对辛氏图小波高频成分采用随机训练策略,增强了特征表示和捕捉不同频段细节的能力,提高了性能和鲁棒性。采用两阶段迭代重建方法,确保重建图像的整体一致性并优化细节。实验结果证明SWARM在多个数据集上定量和定性性能均优于其他方法。
Key Takeaways:
- 扩散模型在SVCT重建中具有显著潜力。
- 在有限样本空间训练的网络可能限制了其在陌生数据上的表现。
- SWARM模型通过引入随机掩膜策略扩展了训练样本空间。
- 随机训练策略提高了模型对数据不确定性的理解和泛化能力。
- 对辛氏图小波高频成分采用随机训练策略,增强了特征表示和捕捉细节的能力。
- 采用两阶段迭代重建方法确保图像全局一致性并优化细节。
点此查看论文截图
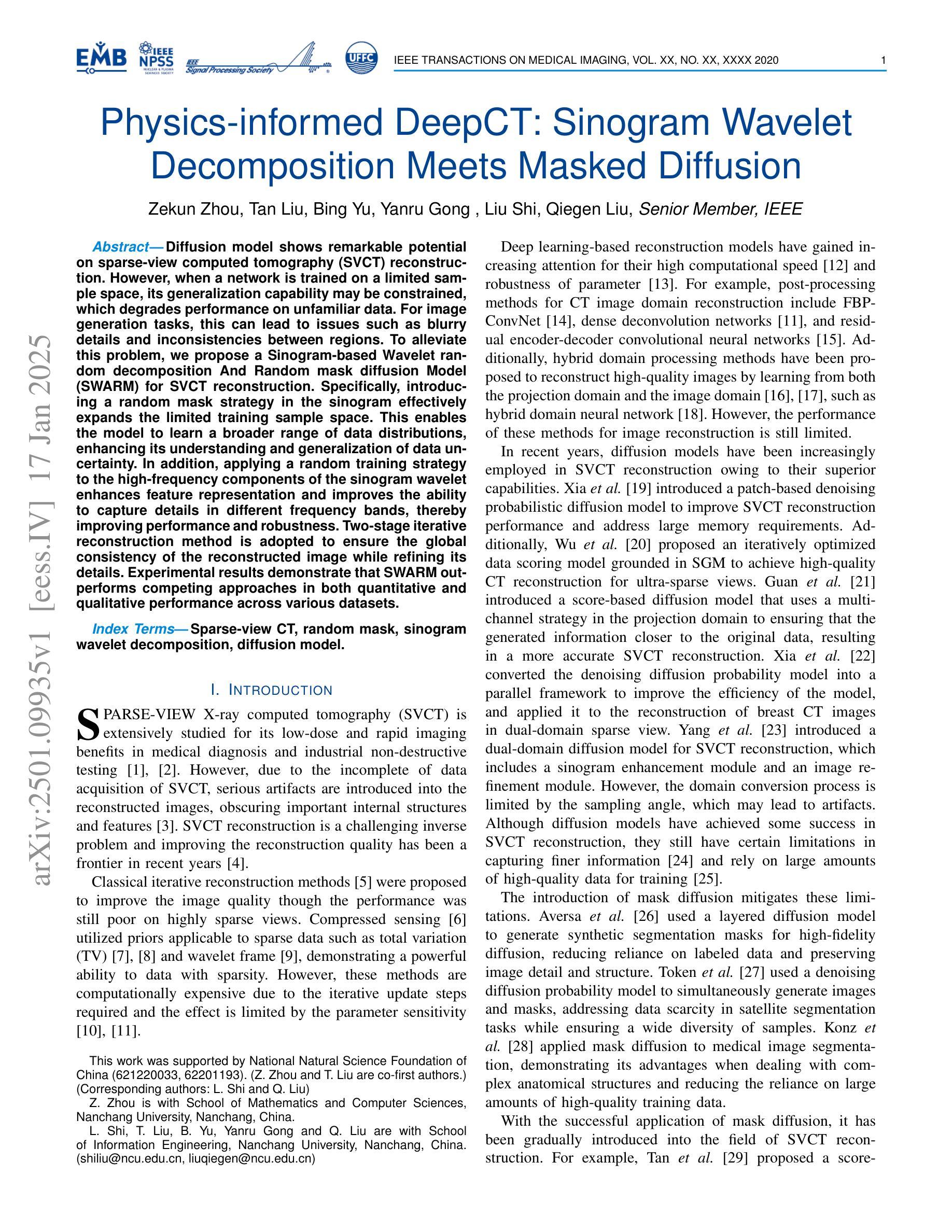
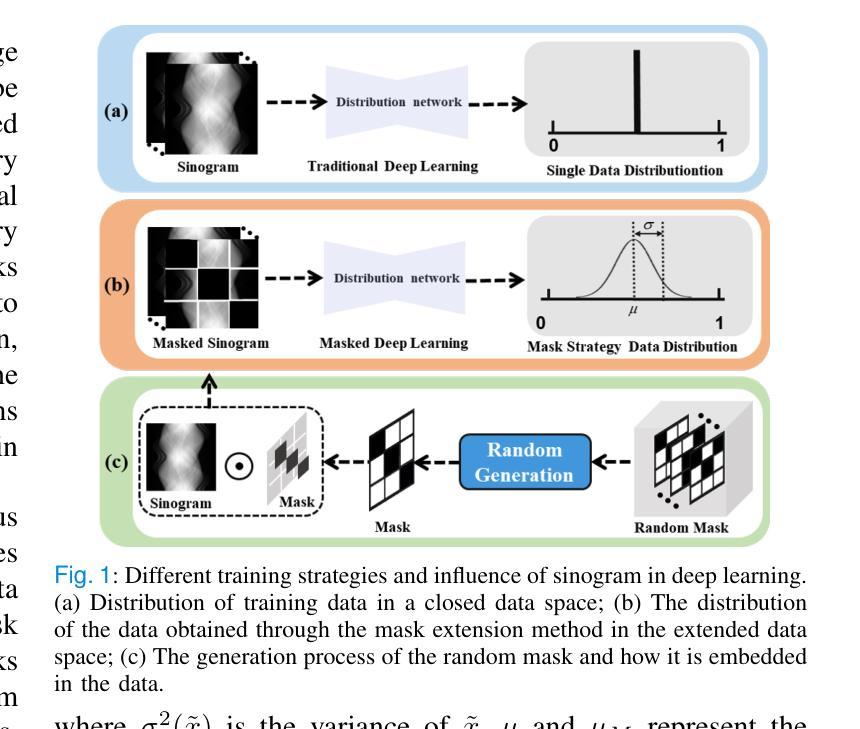
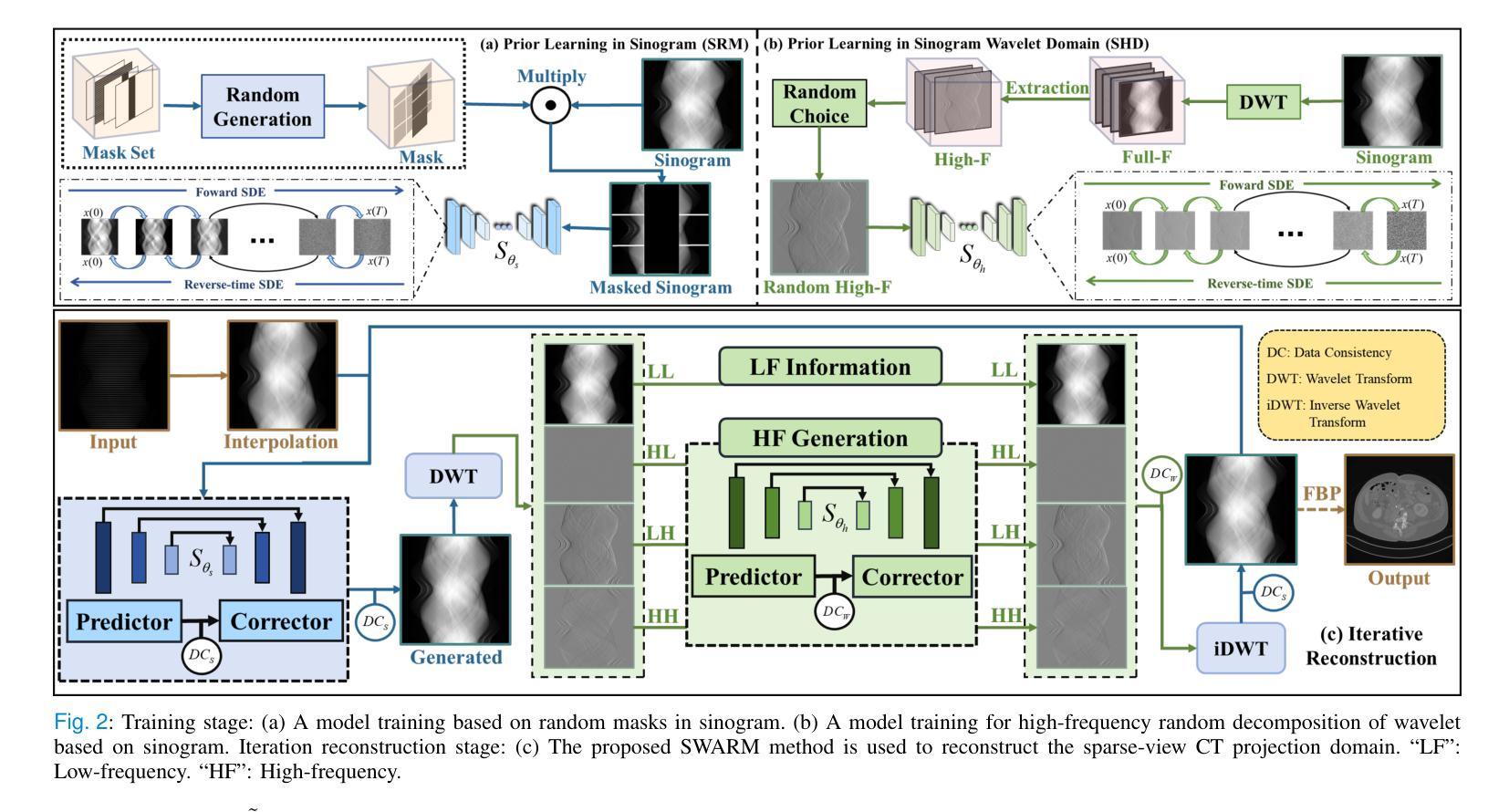
CrossModalityDiffusion: Multi-Modal Novel View Synthesis with Unified Intermediate Representation
Authors:Alex Berian, Daniel Brignac, JhihYang Wu, Natnael Daba, Abhijit Mahalanobis
Geospatial imaging leverages data from diverse sensing modalities-such as EO, SAR, and LiDAR, ranging from ground-level drones to satellite views. These heterogeneous inputs offer significant opportunities for scene understanding but present challenges in interpreting geometry accurately, particularly in the absence of precise ground truth data. To address this, we propose CrossModalityDiffusion, a modular framework designed to generate images across different modalities and viewpoints without prior knowledge of scene geometry. CrossModalityDiffusion employs modality-specific encoders that take multiple input images and produce geometry-aware feature volumes that encode scene structure relative to their input camera positions. The space where the feature volumes are placed acts as a common ground for unifying input modalities. These feature volumes are overlapped and rendered into feature images from novel perspectives using volumetric rendering techniques. The rendered feature images are used as conditioning inputs for a modality-specific diffusion model, enabling the synthesis of novel images for the desired output modality. In this paper, we show that jointly training different modules ensures consistent geometric understanding across all modalities within the framework. We validate CrossModalityDiffusion’s capabilities on the synthetic ShapeNet cars dataset, demonstrating its effectiveness in generating accurate and consistent novel views across multiple imaging modalities and perspectives.
地理空间成像利用来自不同感知模式的数据,如EO、SAR和LiDAR,从地面无人机到卫星视图的各种数据。这些异构输入为场景理解提供了重大机会,但在没有精确地面真实数据的情况下,对几何的准确解释却带来了挑战。为了解决这一问题,我们提出了CrossModalityDiffusion,这是一个模块化框架,旨在在不同模态和视角生成图像,而无需事先了解场景几何。CrossModalityDiffusion采用特定模态的编码器,这些编码器接受多个输入图像并产生感知几何特征的体积,这些体积编码了相对于其输入相机位置的场景结构。特征体积所处的空间成为统一输入模态的共同基础。这些特征体积被重叠并使用体积渲染技术从新的视角渲染成特征图像。渲染的特征图像用作特定模态扩散模型的调节输入,从而合成所需输出模态的新图像。在本文中,我们展示了联合训练不同的模块可以确保框架内所有模态的几何理解的一致性。我们在合成的ShapeNet汽车数据集上验证了CrossModalityDiffusion的能力,证明了它在生成多个成像模态和视角的新颖且准确的视图方面的有效性。
论文及项目相关链接
PDF Accepted in the 2025 WACV workshop GeoCV
Summary
本文提出了一个跨模态扩散模型框架(CrossModalityDiffusion),该框架可在不同模态和视角生成图像,无需对场景几何的先验知识。该框架采用模态特定编码器处理多种输入图像,生成包含场景结构的特征体积,并通过体积渲染技术从新颖视角呈现特征图像。这些特征图像为特定模态的扩散模型提供条件输入,从而合成目标模态的新颖图像。本文验证了在不同模块联合训练下,该框架在多模态和透视场景中的几何一致性理解能力。在ShapeNet汽车数据集上的实验表明,该框架能生成准确且一致的新视角跨模态图像。
Key Takeaways
- Geospatial成像利用多种传感器模态的数据,如EO、SAR和LiDAR,从地面无人机到卫星视图不等。
- 跨模态扩散模型框架(CrossModalityDiffusion)设计用于不同模态和视角生成图像,无需对场景几何先验知识。
- CrossModalityDiffusion采用模态特定编码器生成包含场景结构的特征体积,并将它们放置在统一的空间中以融合不同模态。
- 通过体积渲染技术从新颖视角呈现特征图像。
- 特征图像为特定模态的扩散模型提供条件输入,合成目标模态的新颖图像。
- 联合训练不同模块确保了跨所有模态的几何一致性理解。
点此查看论文截图
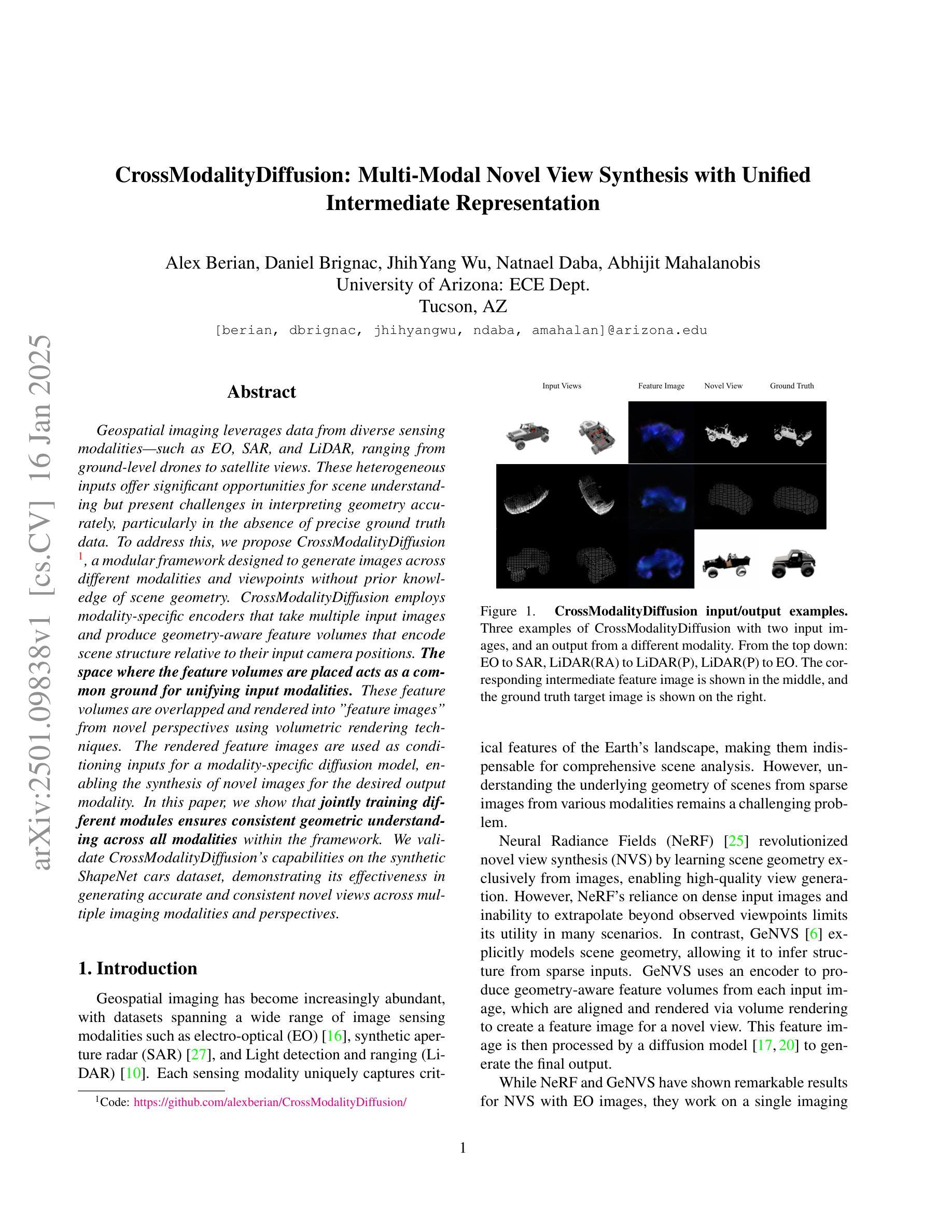
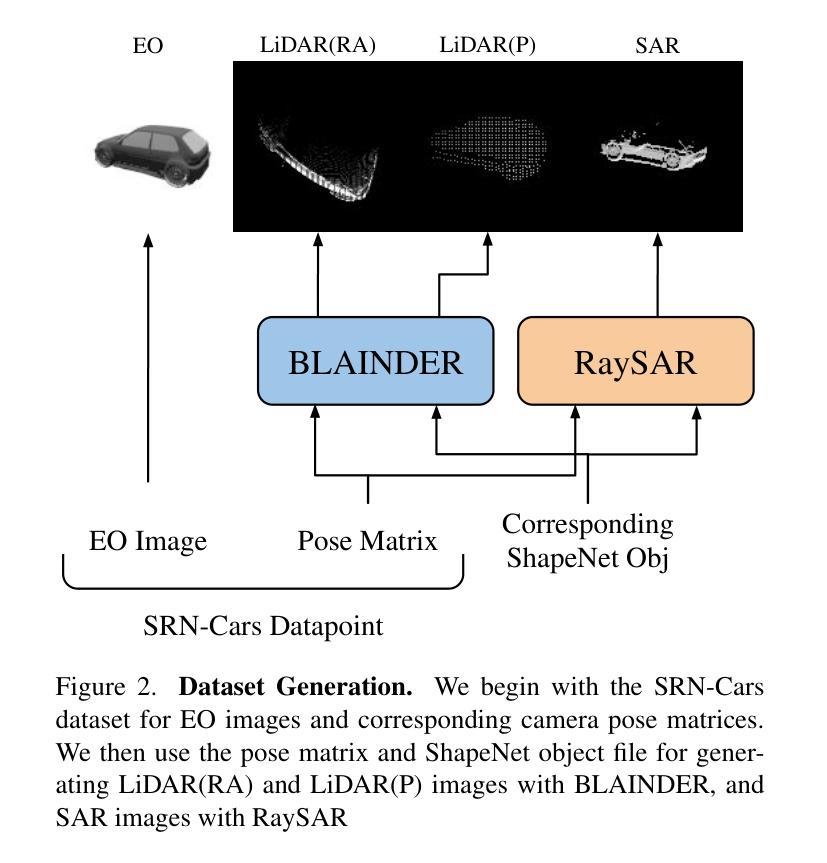
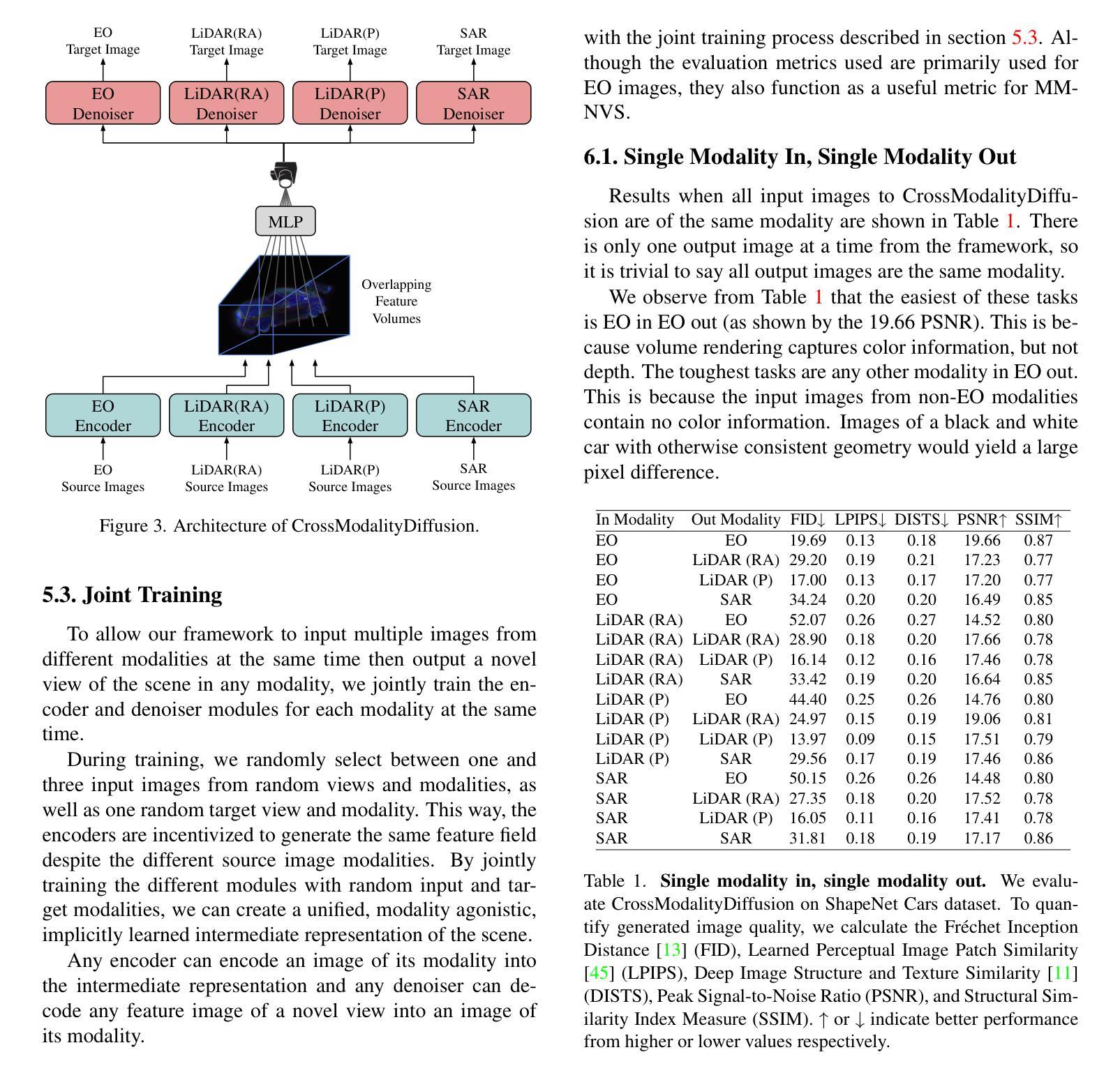
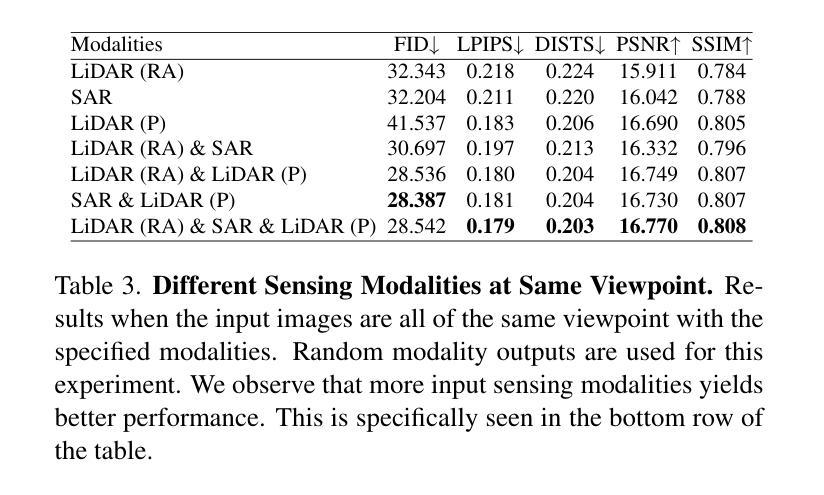
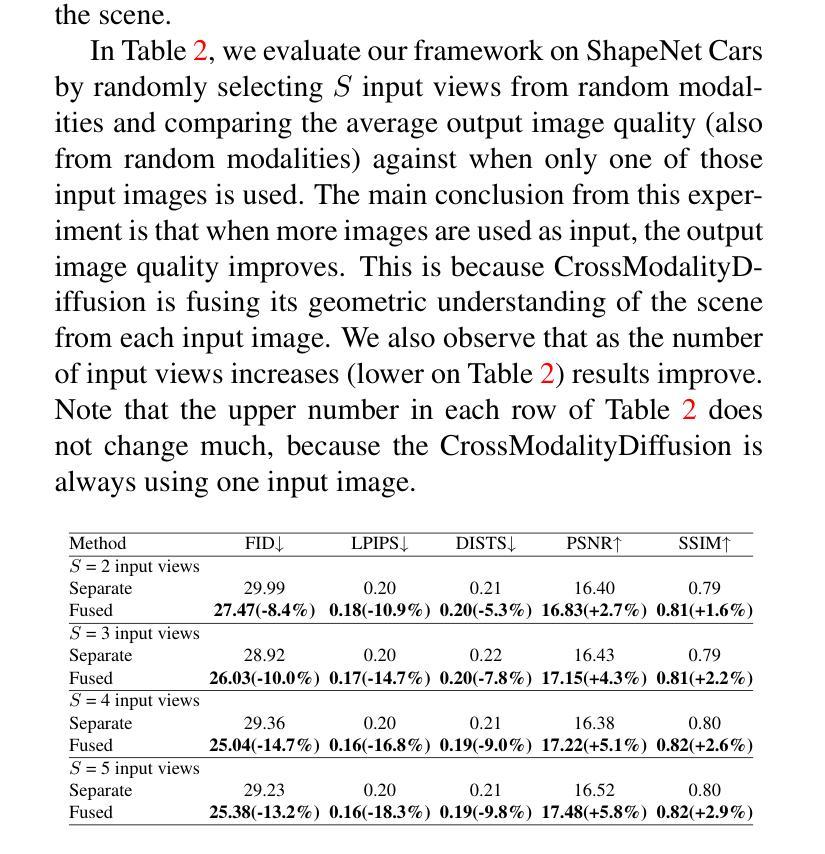
Lossy Compression with Pretrained Diffusion Models
Authors:Jeremy Vonderfecht, Feng Liu
We apply the DiffC algorithm (Theis et al. 2022) to Stable Diffusion 1.5, 2.1, XL, and Flux-dev, and demonstrate that these pretrained models are remarkably capable lossy image compressors. A principled algorithm for lossy compression using pretrained diffusion models has been understood since at least Ho et al. 2020, but challenges in reverse-channel coding have prevented such algorithms from ever being fully implemented. We introduce simple workarounds that lead to the first complete implementation of DiffC, which is capable of compressing and decompressing images using Stable Diffusion in under 10 seconds. Despite requiring no additional training, our method is competitive with other state-of-the-art generative compression methods at low ultra-low bitrates.
我们将DiffC算法(Theis等人,2022年)应用于Stable Diffusion 1.5、2.1、XL和Flux-dev,并证明这些预训练模型是出色的有损图像压缩器。至少自Ho等人(2020年)以来,已经了解使用预训练扩散模型进行有损压缩的原理算法,但反向信道编码的挑战使得此类算法从未得到全面实施。我们介绍了一些简单的解决方案,首次实现了完整的DiffC实现,能够在不到10秒内使用Stable Diffusion对图像进行压缩和解压缩。我们的方法无需额外的训练,在低超低比特率下与其他最先进的生成压缩方法具有竞争力。
论文及项目相关链接
Summary
将DiffC算法应用于Stable Diffusion 1.5、2.1、XL和Flux-dev等预训练模型,证明这些模型具有出色的有损图像压缩能力。虽然早在Ho等人在2020年就已经了解了利用预训练扩散模型进行有损压缩的原理,但由于反向信道编码的挑战,相关算法一直没有完全实现。研究团队提出了简单对策,首次完成了DiffC的完整实现,能在不到10秒内使用Stable Diffusion对图像进行压缩和解压缩。该方法无需额外训练,在低超低比特率下与其他先进的生成压缩方法相比具有竞争力。
Key Takeaways
- DiffC算法成功应用于多种预训练扩散模型,包括Stable Diffusion 1.5、2.1、XL和Flux-dev,展示出色的有损图像压缩能力。
- 尽管自Ho等人在2020年提出利用预训练扩散模型进行有损压缩的原理以来,相关算法一直面临反向信道编码的挑战,但研究团队成功解决了这一问题。
- 研究团队首次实现了DiffC的完整版本,能够在短时间内(不到10秒)完成图像的压缩和解压缩过程。
- 该方法无需对模型进行额外训练,具有实际应用价值。
- 与其他先进的生成压缩方法相比,该方法在超低比特率下表现出竞争力。
- 这一进展为利用预训练扩散模型进行图像压缩提供了新的可能性和实际应用场景。
点此查看论文截图
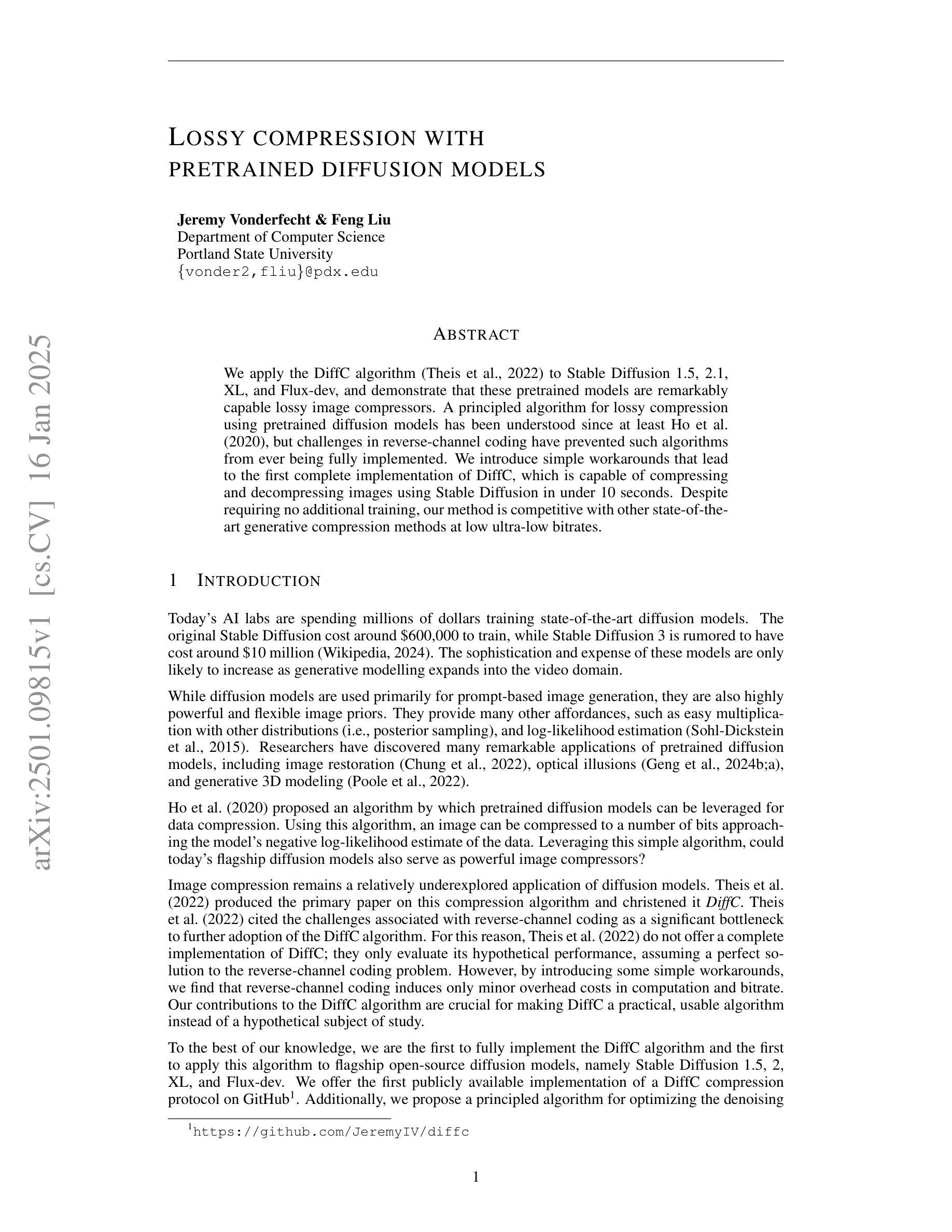
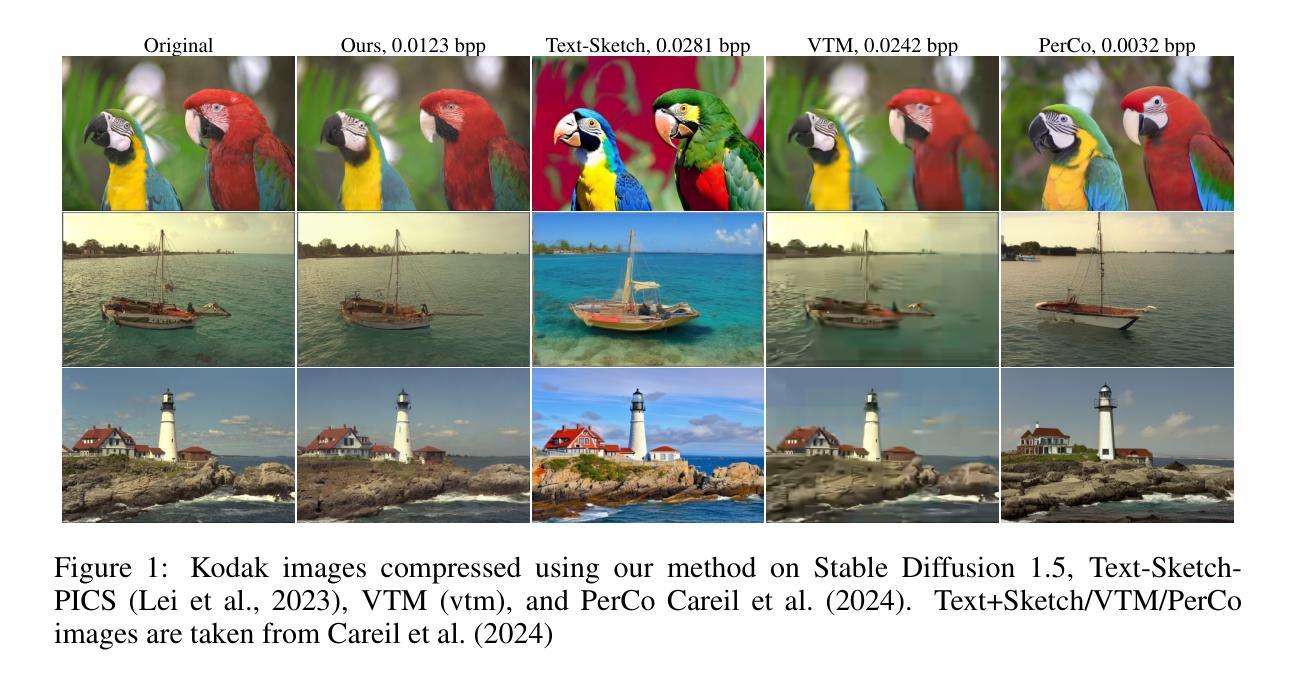
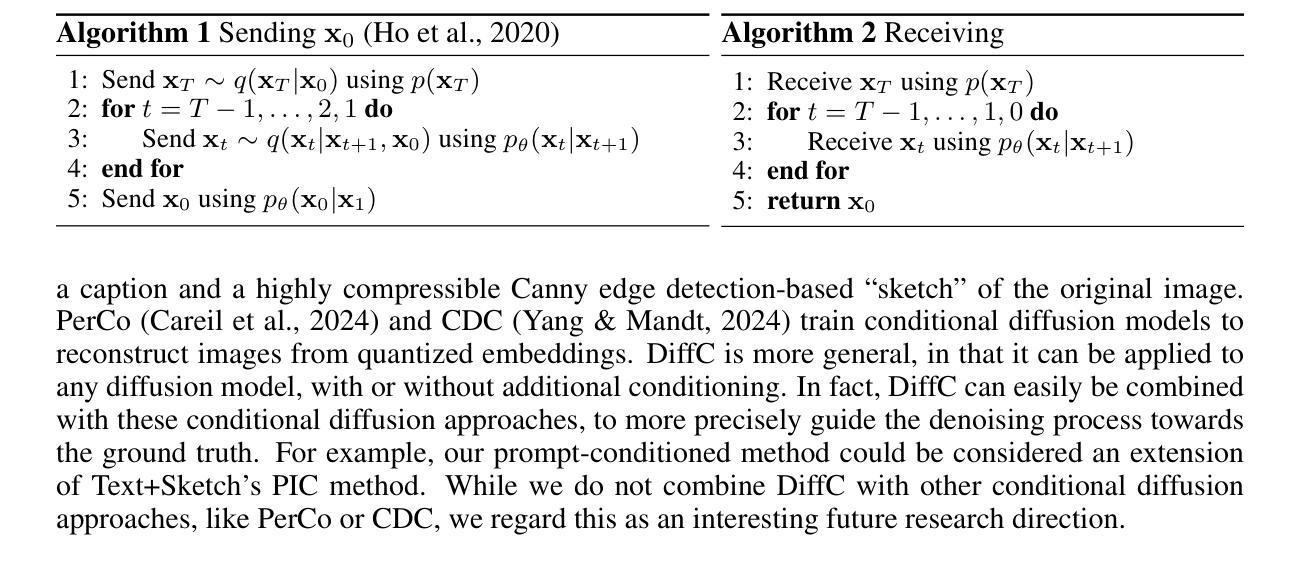
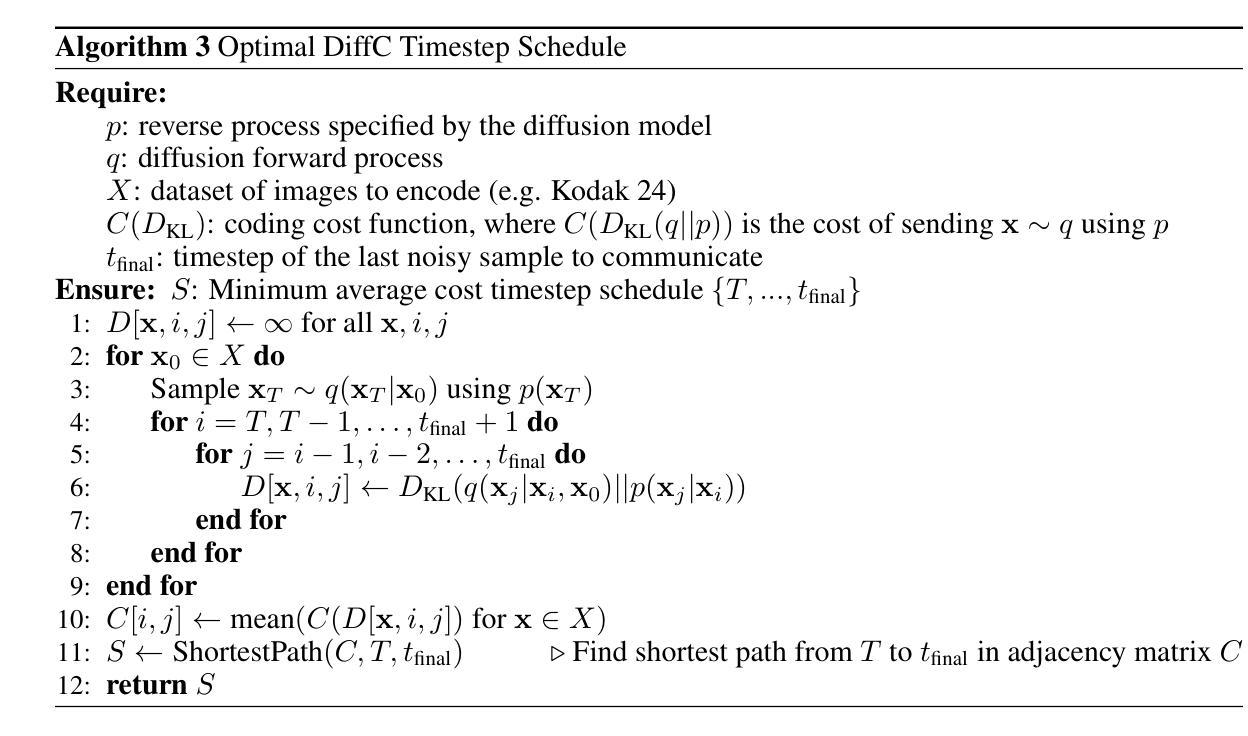
NeurOp-Diff:Continuous Remote Sensing Image Super-Resolution via Neural Operator Diffusion
Authors:Zihao Xu, Yuzhi Tang, Bowen Xu, Qingquan Li
Most publicly accessible remote sensing data suffer from low resolution, limiting their practical applications. To address this, we propose a diffusion model guided by neural operators for continuous remote sensing image super-resolution (NeurOp-Diff). Neural operators are used to learn resolution representations at arbitrary scales, encoding low-resolution (LR) images into high-dimensional features, which are then used as prior conditions to guide the diffusion model for denoising. This effectively addresses the artifacts and excessive smoothing issues present in existing super-resolution (SR) methods, enabling the generation of high-quality, continuous super-resolution images. Specifically, we adjust the super-resolution scale by a scaling factor s, allowing the model to adapt to different super-resolution magnifications. Furthermore, experiments on multiple datasets demonstrate the effectiveness of NeurOp-Diff. Our code is available at https://github.com/zerono000/NeurOp-Diff.
大部分公开可访问的遥感数据存在分辨率低的问题,这限制了它们的实际应用。为解决这一问题,我们提出了一种由神经算子引导的扩散模型,用于连续遥感图像超分辨率(NeurOp-Diff)。神经算子用于学习任意尺度的分辨率表示,将低分辨率(LR)图像编码为高维特征,然后将其作为先验条件,引导扩散模型进行去噪。这有效地解决了现有超分辨率(SR)方法中出现的伪影和过度平滑问题,能够生成高质量、连续的超高分辨率图像。具体来说,我们通过缩放因子s调整超分辨率尺度,使模型能够适应不同的超分辨率放大倍数。此外,多个数据集上的实验证明了NeurOp-Diff的有效性。我们的代码可在https://github.com/zerono000/NeurOp-Diff上获取。
论文及项目相关链接
摘要
针对现有遥感数据分辨率低的问题,提出了一种基于神经算子和扩散模型的连续遥感图像超分辨率方法(NeurOp-Diff)。该方法利用神经算子学习任意尺度的分辨率表示,将低分辨率图像编码为高维特征,并作为先验条件引导扩散模型进行去噪。这解决了现有超分辨率方法中的伪影和过度平滑问题,能够生成高质量、连续的超级分辨率图像。通过调整超分辨率尺度缩放因子s,该模型可适应不同的超分辨率放大倍数。在多个数据集上的实验验证了NeurOp-Diff的有效性。
要点
- 提出了一种基于神经算子和扩散模型的遥感图像超分辨率方法NeurOp-Diff。
- 神经算子用于学习任意尺度的分辨率表示,并将低分辨率图像转化为高维特征。
- 高维特征作为先验条件引导扩散模型进行去噪,解决了伪影和过度平滑问题。
- 通过调整缩放因子s,模型可适应不同的超分辨率放大需求。
- NeurOp-Diff在多个数据集上的实验验证了其有效性。
- 该模型的代码已公开可用。
- 该方法能够生成高质量的连续超级分辨率图像。
点此查看论文截图
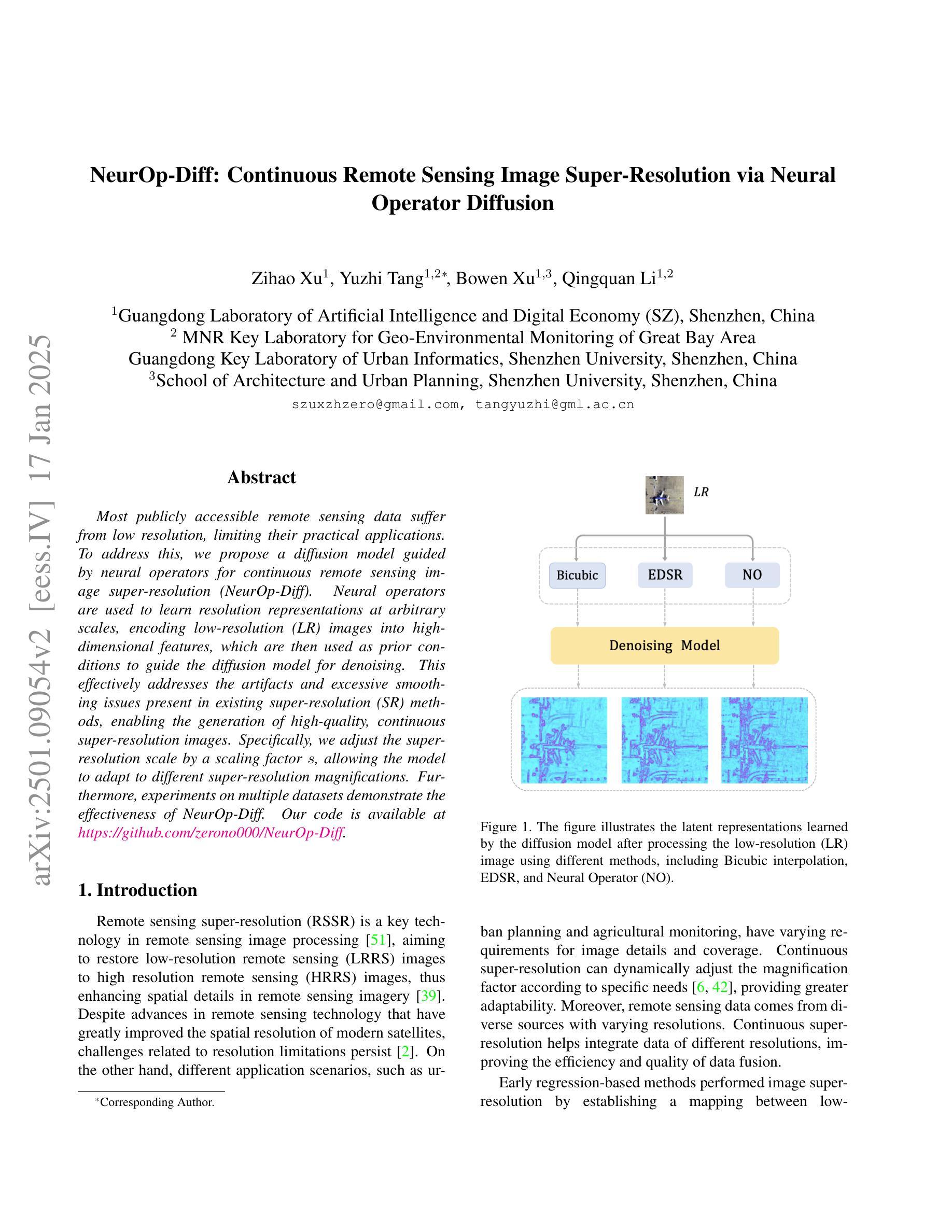
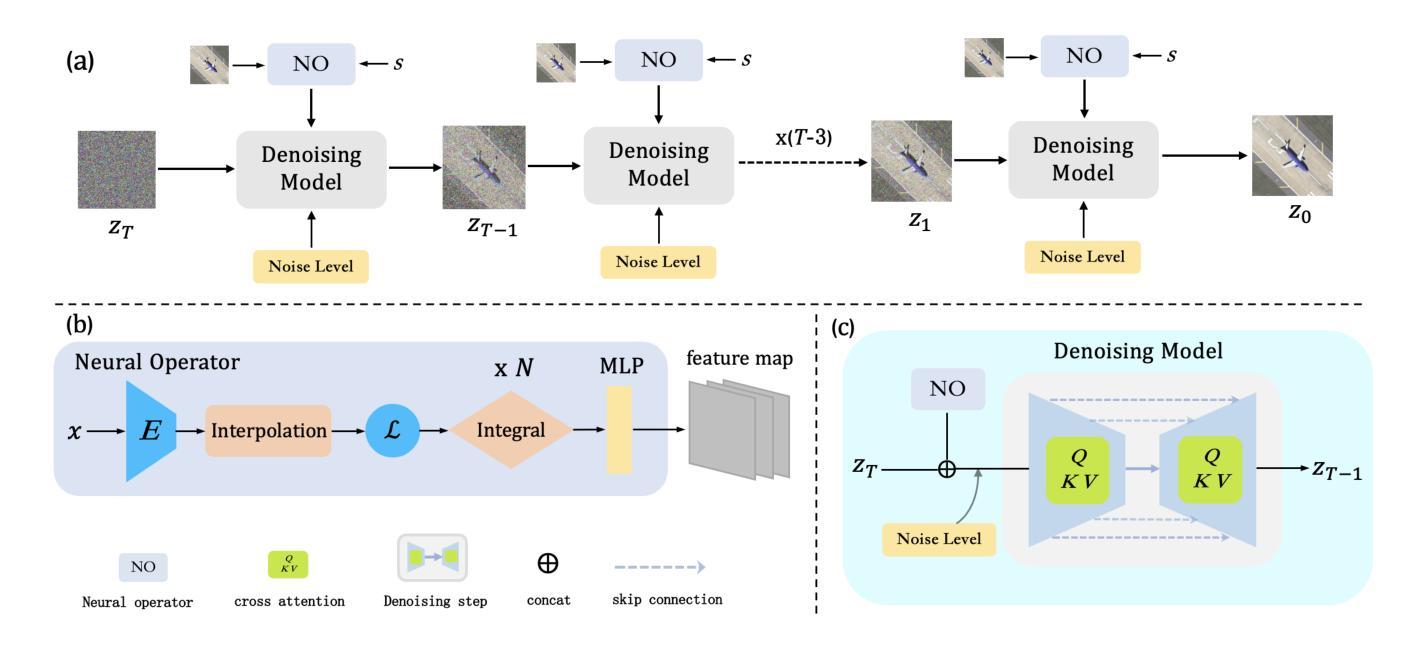
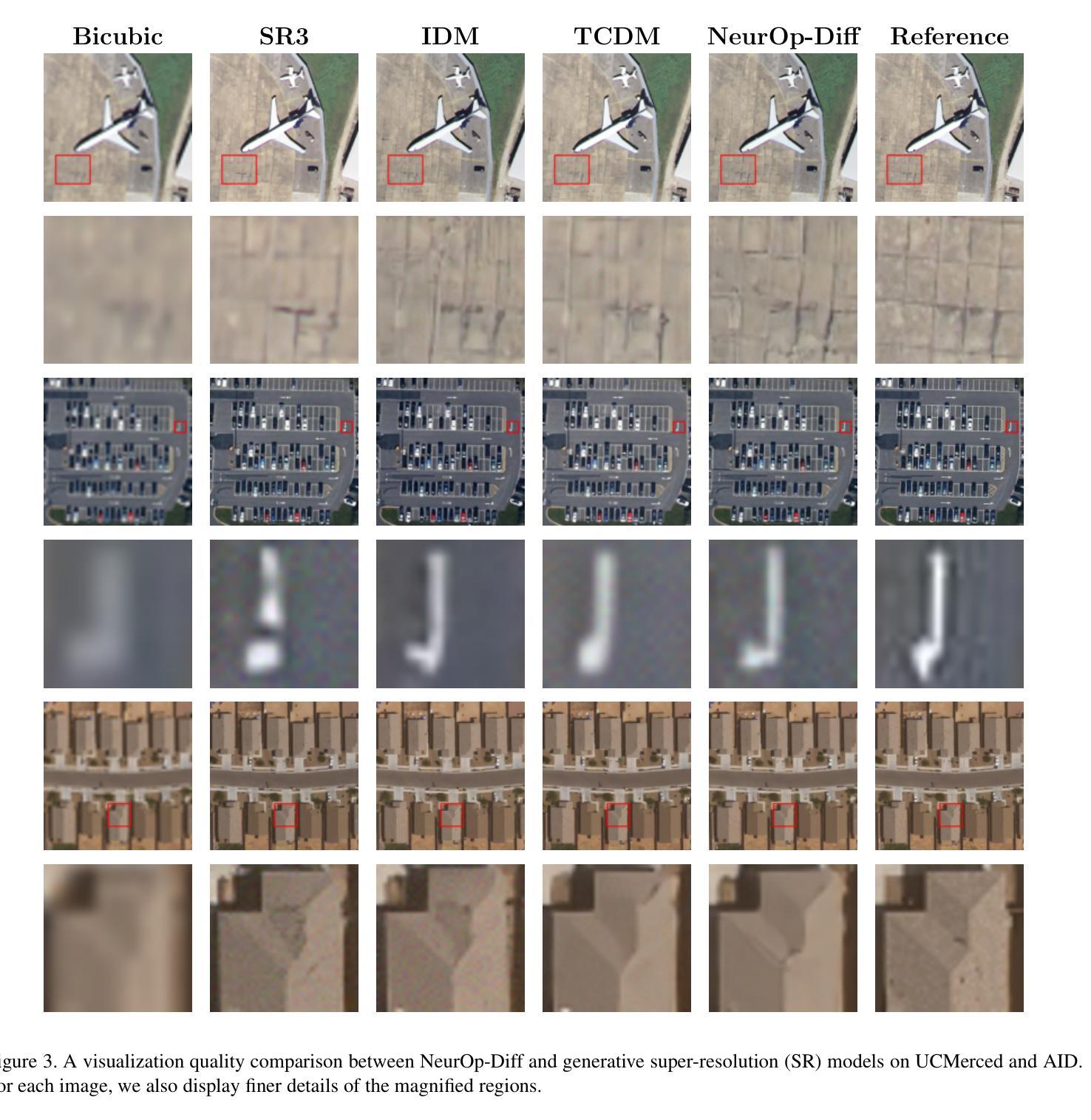
DPCL-Diff: The Temporal Knowledge Graph Reasoning Based on Graph Node Diffusion Model with Dual-Domain Periodic Contrastive Learning
Authors:Yukun Cao, Lisheng Wang, Luobin Huang
Temporal knowledge graph (TKG) reasoning that infers future missing facts is an essential and challenging task. Predicting future events typically relies on closely related historical facts, yielding more accurate results for repetitive or periodic events. However, for future events with sparse historical interactions, the effectiveness of this method, which focuses on leveraging high-frequency historical information, diminishes. Recently, the capabilities of diffusion models in image generation have opened new opportunities for TKG reasoning. Therefore, we propose a graph node diffusion model with dual-domain periodic contrastive learning (DPCL-Diff). Graph node diffusion model (GNDiff) introduces noise into sparsely related events to simulate new events, generating high-quality data that better conforms to the actual distribution. This generative mechanism significantly enhances the model’s ability to reason about new events. Additionally, the dual-domain periodic contrastive learning (DPCL) maps periodic and non-periodic event entities to Poincar'e and Euclidean spaces, leveraging their characteristics to distinguish similar periodic events effectively. Experimental results on four public datasets demonstrate that DPCL-Diff significantly outperforms state-of-the-art TKG models in event prediction, demonstrating our approach’s effectiveness. This study also investigates the combined effectiveness of GNDiff and DPCL in TKG tasks.
时序知识图谱(TKG)推理,推断未来缺失的事实,是一项重要且具有挑战性的任务。预测未来事件通常依赖于密切相关的历史事实,对于重复性或周期性事件,能带来更准确的结果。然而,对于历史交互稀疏的未来事件,这种方法的有效性会降低,因为它主要侧重于利用高频历史信息。最近,扩散模型在图像生成方面的能力为TKG推理提供了新的机会。因此,我们提出了一种具有双域周期性对比学习(DPCL)的图节点扩散模型(DPCL-Diff)。图节点扩散模型(GNDiff)将噪声引入稀疏相关的事件中,以模拟新事件,生成高质量的数据,更好地符合实际分布。这种生成机制显著提高了模型对新事件的推理能力。此外,双域周期性对比学习(DPCL)将周期性和非周期性事件实体映射到Poincaré和欧几里得空间,利用其特性来有效地区分相似的周期性事件。在四个公共数据集上的实验结果表明,DPCL-Diff在事件预测方面显著优于最先进的TKG模型,证明了我们的方法的有效性。本研究还探讨了GNDiff和DPCL在TKG任务中的组合效果。
论文及项目相关链接
PDF 11 pages, 2 figures
Summary
基于时序知识图谱(TKG)推理预测未来缺失事实是一项重要且具挑战性的任务。现有方法主要依赖历史事实进行未来事件预测,对于重复或周期性事件效果较好,但对于历史交互稀疏的未来事件则表现不足。本文提出了结合图节点扩散模型和双域周期性对比学习(DPCL-Diff)的方法,通过引入噪声模拟新事件,提高模型对新事件的推理能力。同时,DPCL将周期性和非周期性事件实体映射到不同的空间,以区分相似的周期性事件。在四个公开数据集上的实验结果表明,DPCL-Diff在事件预测方面显著优于现有TKG模型。
Key Takeaways
- 时序知识图谱(TKG)推理预测未来缺失事实很重要且具挑战性。
- 现有方法主要依赖历史事实进行预测,对稀疏历史交互的未来事件效果有限。
- 提出的图节点扩散模型(GNDiff)通过引入噪声模拟新事件,提高模型对新事件的推理能力。
- 双域周期性对比学习(DPCL)能有效区分周期性事件和非周期性事件实体。
- DPCL-Diff方法结合了GNDiff和DPCL,显著提高了在四个公开数据集上的事件预测性能。
- GNDiff和DPCL的联合效果在TKG任务中得到了调查验证。
点此查看论文截图
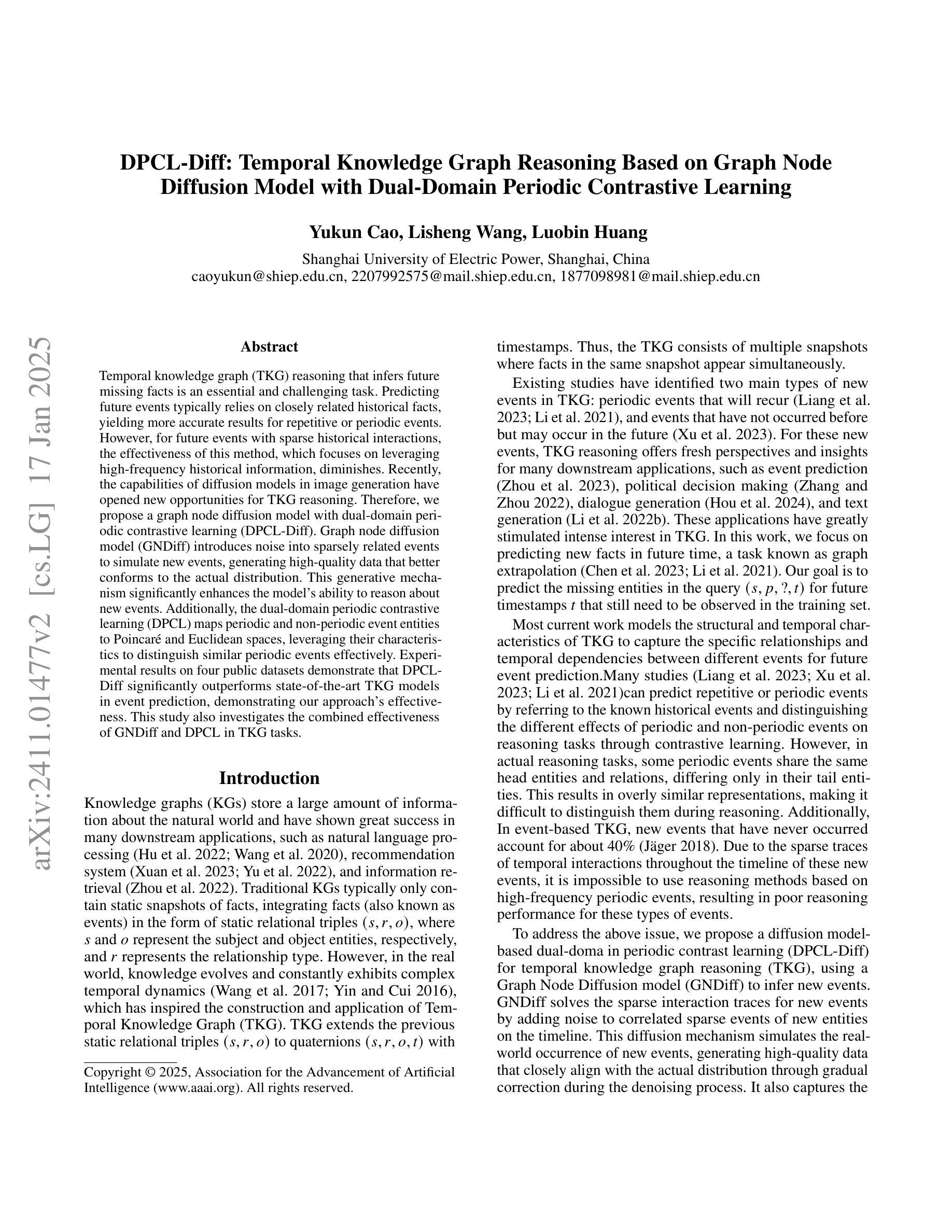
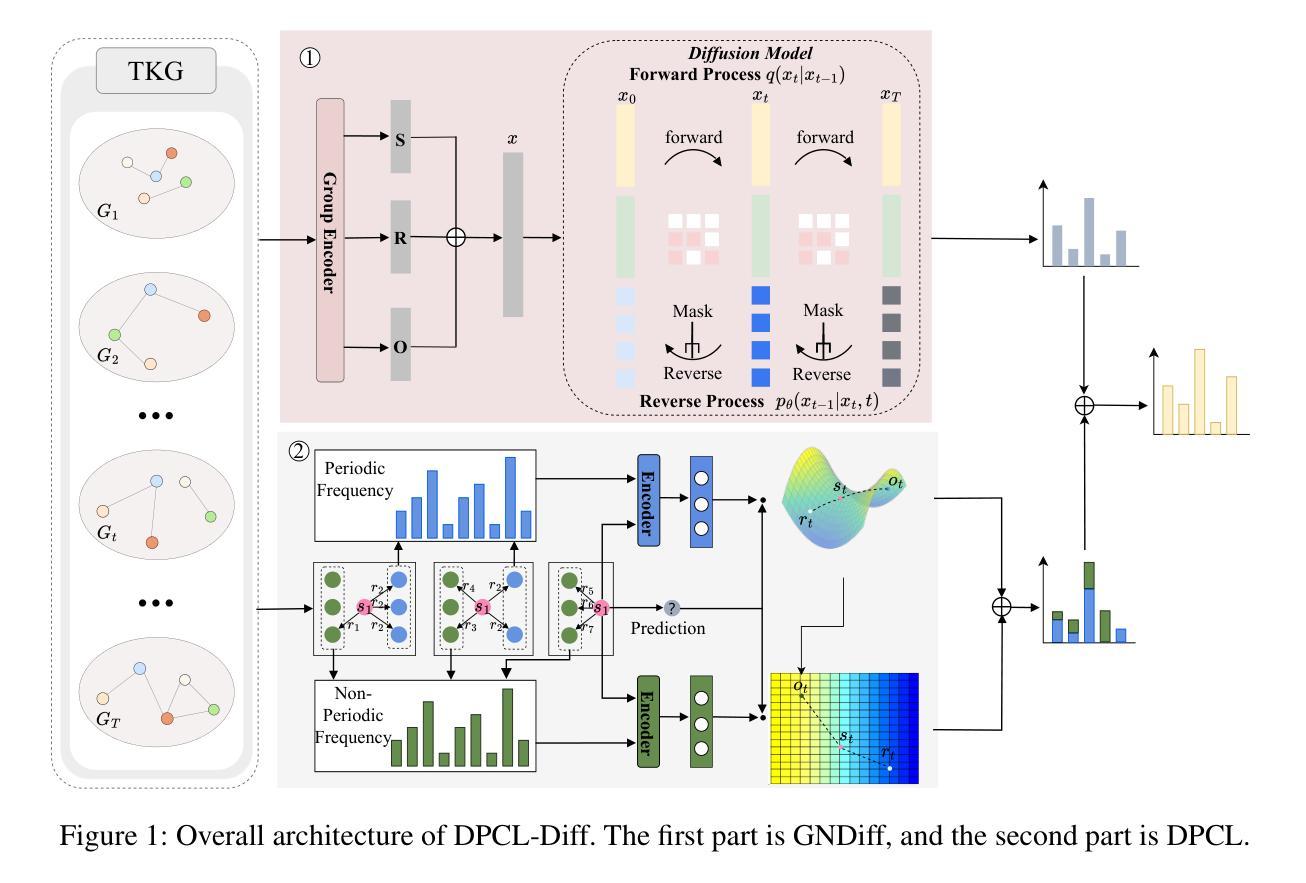
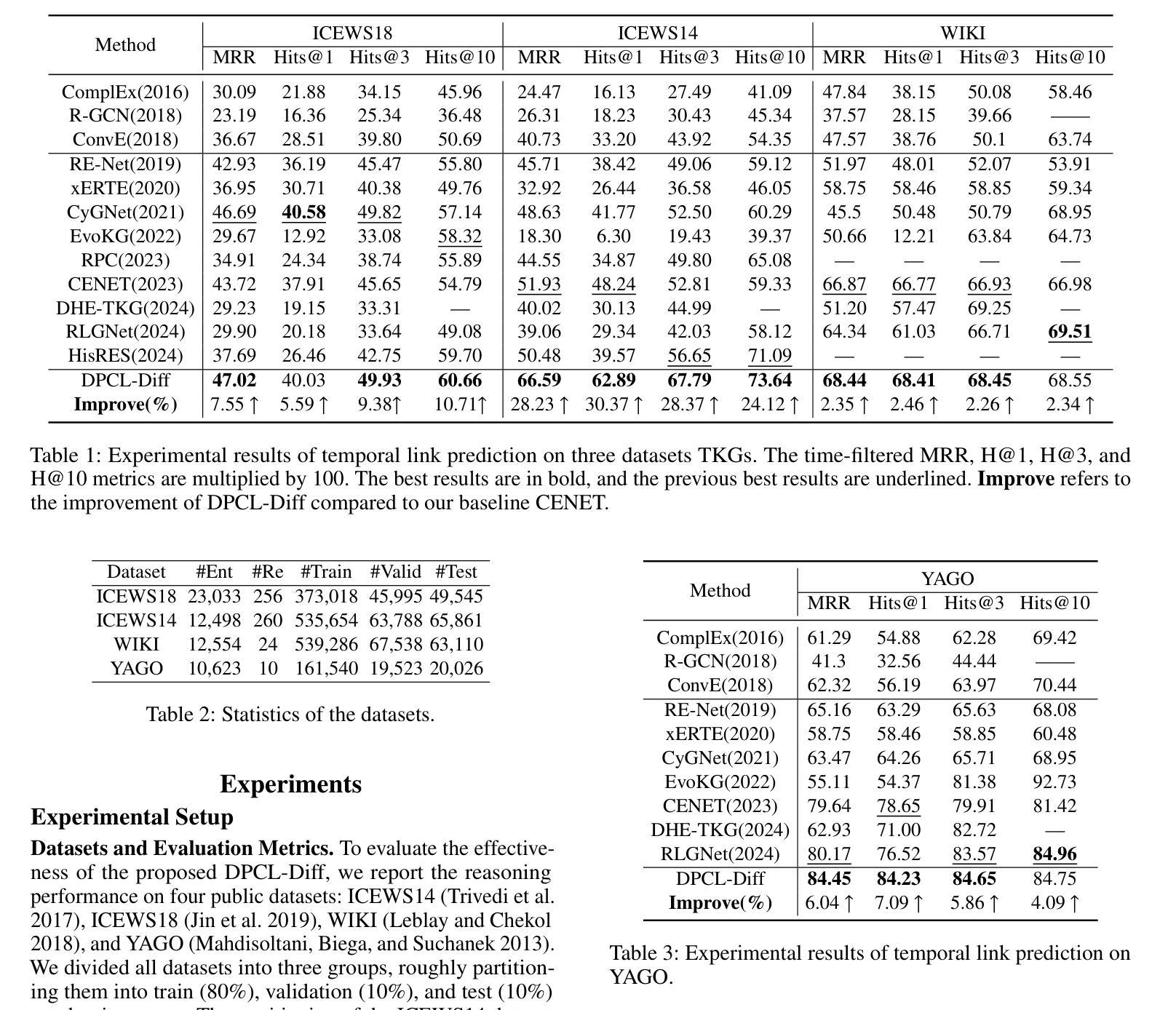
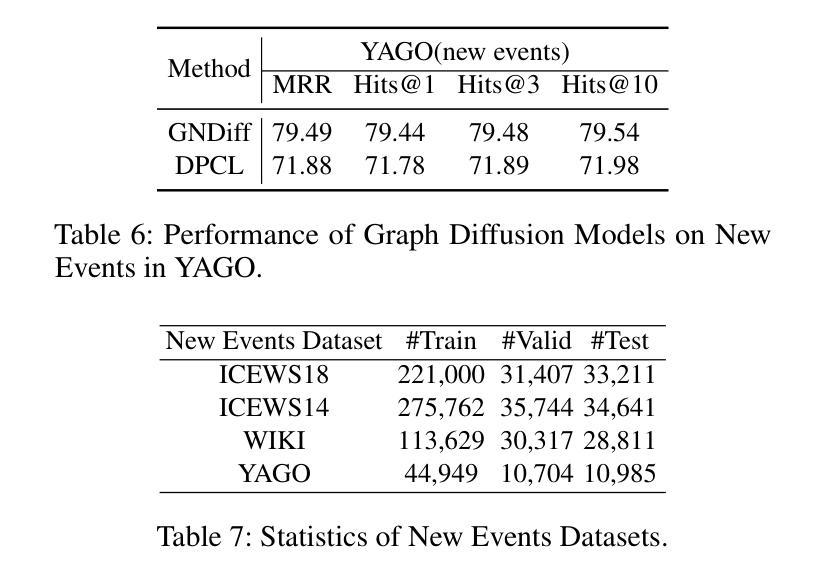
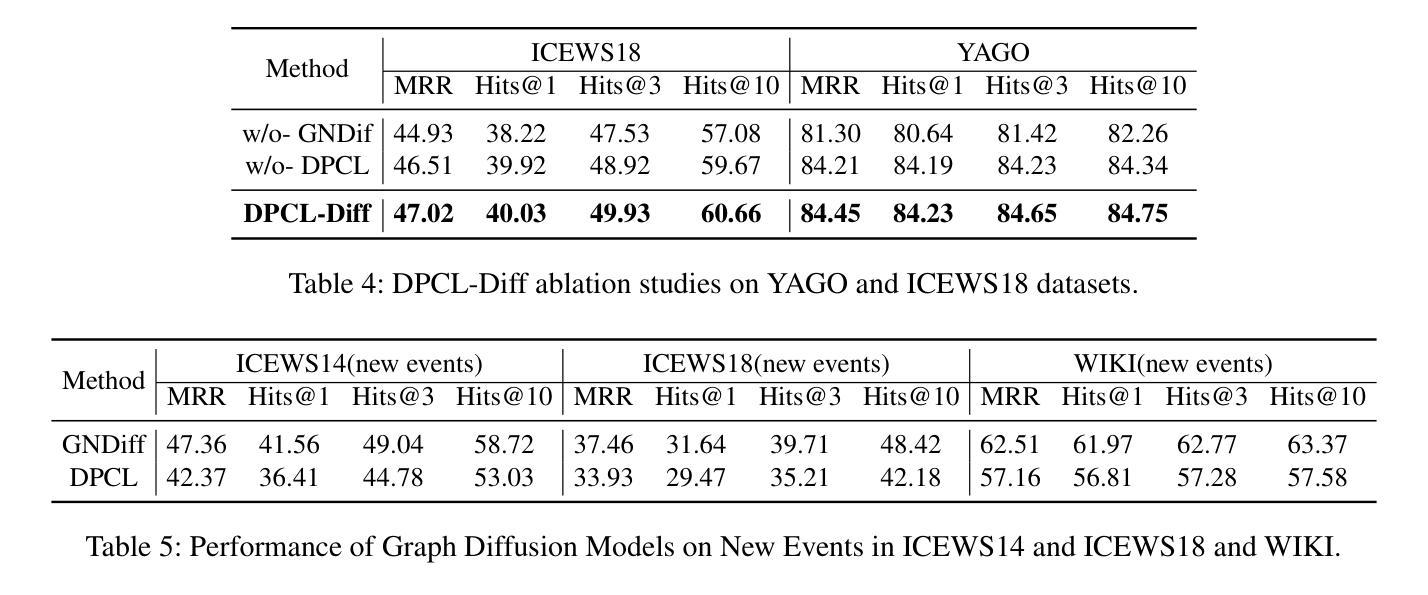
DX2CT: Diffusion Model for 3D CT Reconstruction from Bi or Mono-planar 2D X-ray(s)
Authors:Yun Su Jeong, Hye Bin Yoo, Il Yong Chun
Computational tomography (CT) provides high-resolution medical imaging, but it can expose patients to high radiation. X-ray scanners have low radiation exposure, but their resolutions are low. This paper proposes a new conditional diffusion model, DX2CT, that reconstructs three-dimensional (3D) CT volumes from bi or mono-planar X-ray image(s). Proposed DX2CT consists of two key components: 1) modulating feature maps extracted from two-dimensional (2D) X-ray(s) with 3D positions of CT volume using a new transformer and 2) effectively using the modulated 3D position-aware feature maps as conditions of DX2CT. In particular, the proposed transformer can provide conditions with rich information of a target CT slice to the conditional diffusion model, enabling high-quality CT reconstruction. Our experiments with the bi or mono-planar X-ray(s) benchmark datasets show that proposed DX2CT outperforms several state-of-the-art methods. Our codes and model will be available at: https://www.github.com/intyeger/DX2CT.
计算机断层扫描(CT)提供高分辨率医学成像,但它会使患者暴露在较高的辐射之下。X射线扫描仪的辐射暴露较低,但其分辨率也较低。本文提出了一种新的条件扩散模型DX2CT,能够从双平面或单平面X射线图像重建三维(3D)CT体积。提出的DX2CT由两个关键部分组成:1)使用新变压器将二维(2D)X射线特征图与CT体积的三维位置进行调制,以及2)有效地利用调制后的三维位置感知特征图作为DX2CT的条件。特别是,所提出的变压器可以为条件扩散模型提供目标CT切片的丰富信息,从而实现高质量的CT重建。我们在双平面或单平面X射线基准数据集上的实验表明,所提出的DX2CT优于几种最新方法。我们的代码和模型将在https://www.github.com/intyeger/DX2CT上提供。
论文及项目相关链接
Summary
本文提出了一种新型的条件扩散模型DX2CT,可从二维X-ray图像重建出三维CT体积。该模型由两个关键部分组成:一是使用新变压器调制来自二维X-ray的特征图,并结合CT体积的三维位置;二是有效地利用调制后的三维位置感知特征图作为DX2CT的条件。实验表明,DX2CT在二维X-ray基准数据集上优于其他最先进的方法。
Key Takeaways
- 论文提出了一种新型条件扩散模型DX2CT,可从二维X-ray图像重建出高质量的三维CT体积。
- DX2CT包含两个核心组件:调制特征图的变压器和用于条件扩散模型的三维位置感知特征图。
- 变压器能够从二维X-ray图像中提取特征图,并结合CT体积的三维位置进行调制。
- 调制后的三维位置感知特征图被用作DX2CT的条件,以提高重建质量。
- 实验表明,DX2CT在二维X-ray图像基准数据集上的表现优于其他最先进的方法。
- 该模型的代码和将公开在GitHub上供公众使用。
点此查看论文截图
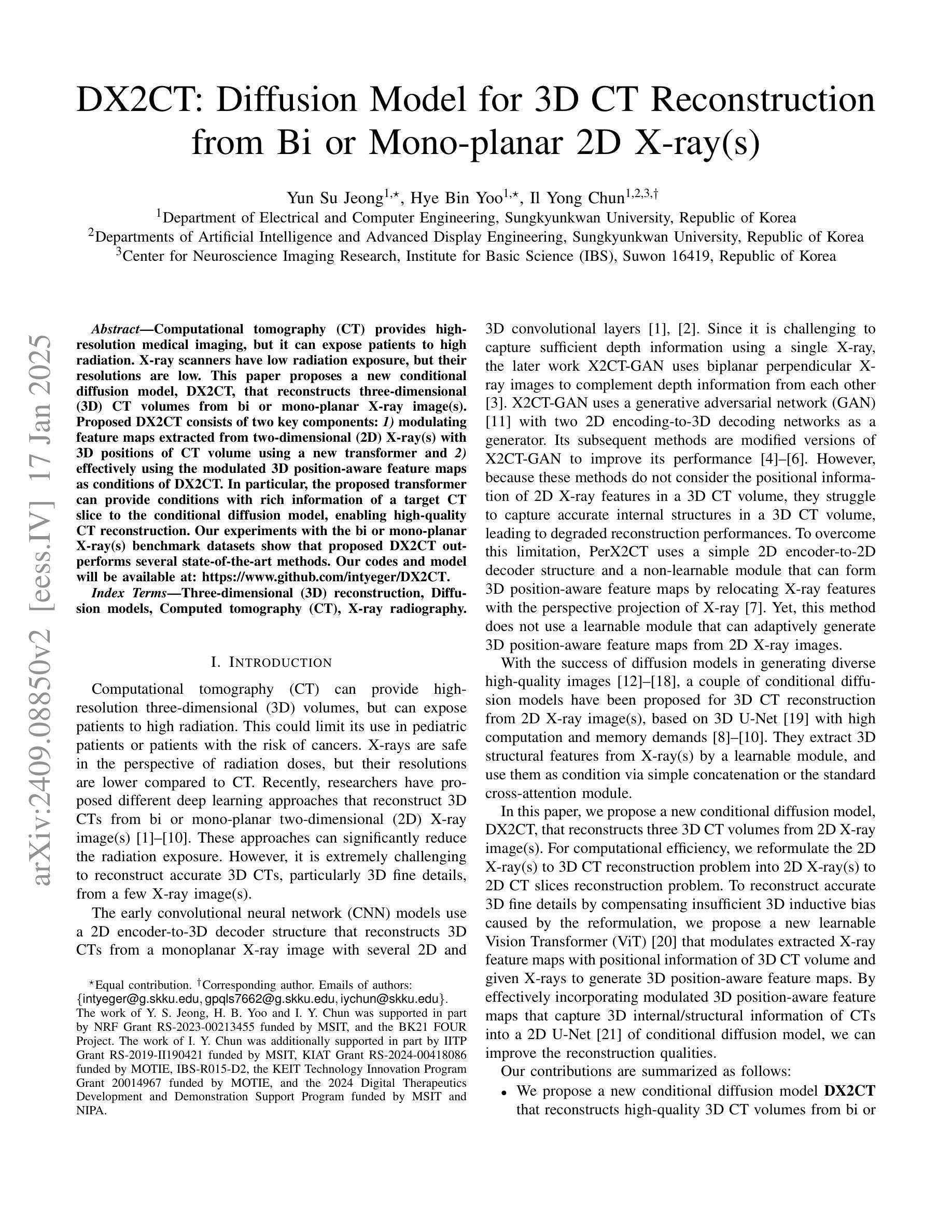
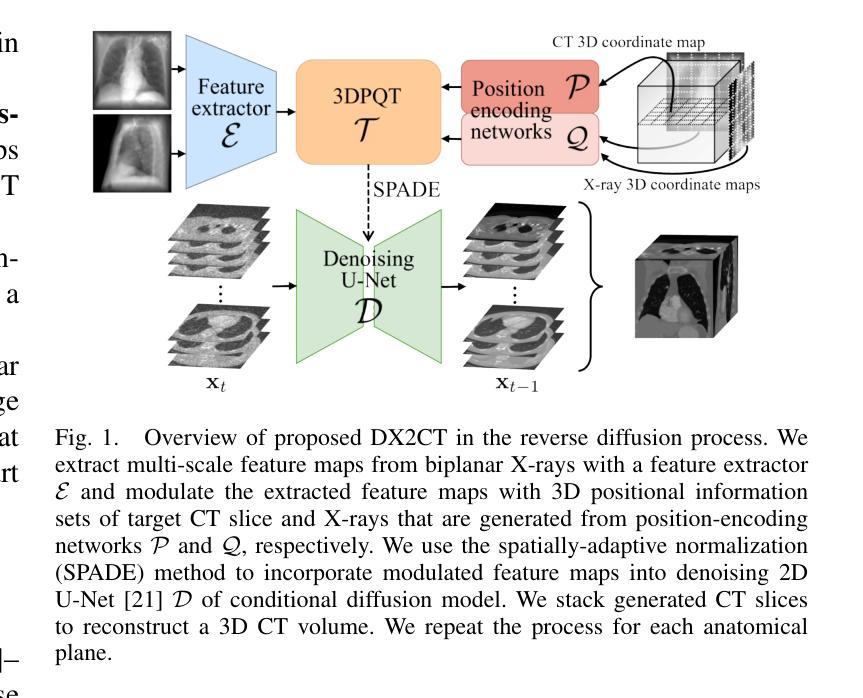
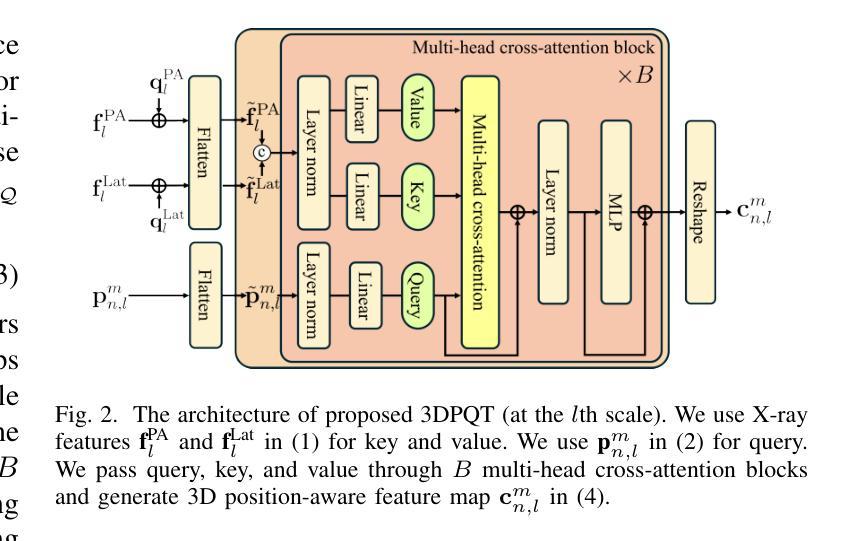
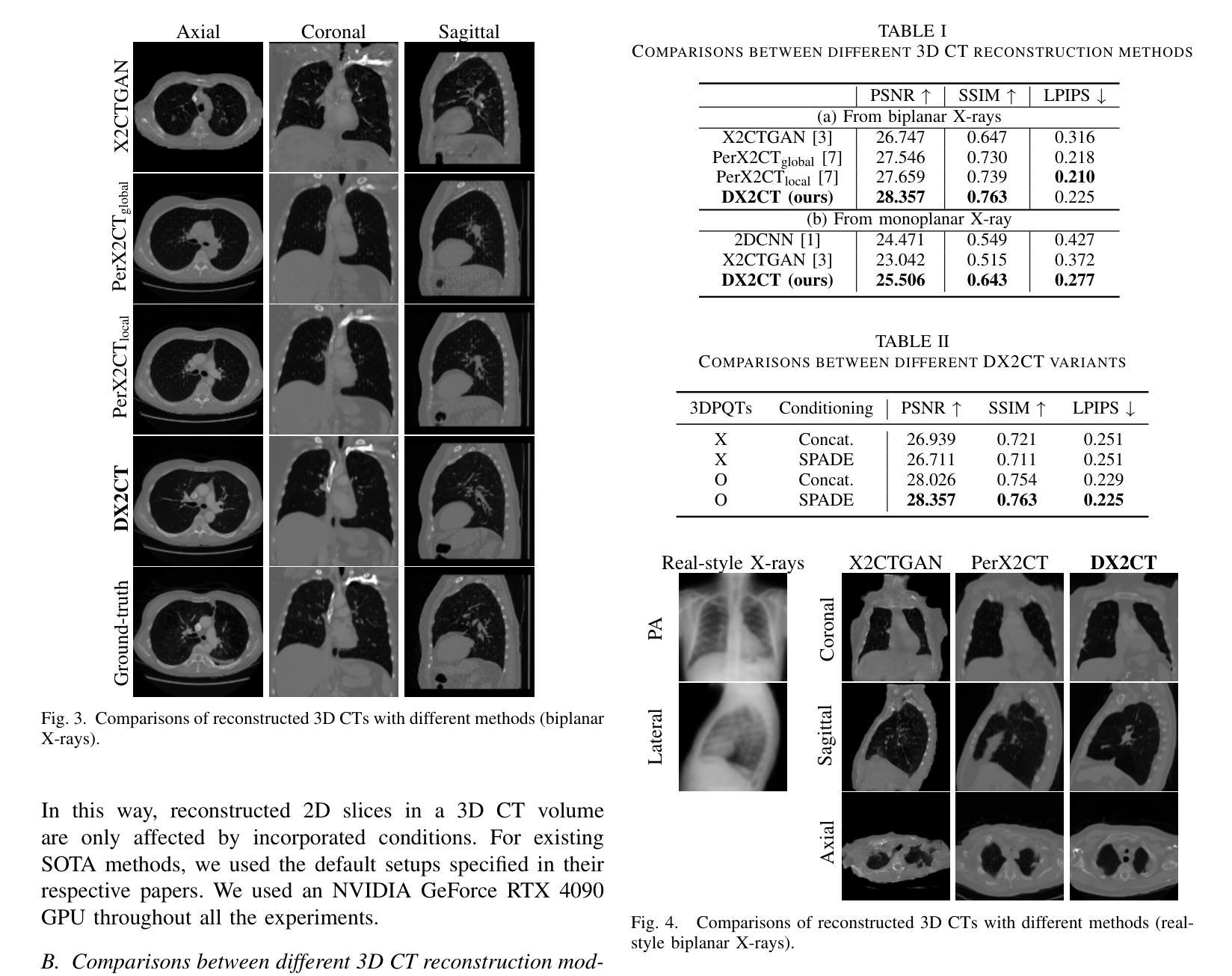
Isolated Diffusion: Optimizing Multi-Concept Text-to-Image Generation Training-Freely with Isolated Diffusion Guidance
Authors:Jingyuan Zhu, Huimin Ma, Jiansheng Chen, Jian Yuan
Large-scale text-to-image diffusion models have achieved great success in synthesizing high-quality and diverse images given target text prompts. Despite the revolutionary image generation ability, current state-of-the-art models still struggle to deal with multi-concept generation accurately in many cases. This phenomenon is known as ``concept bleeding” and displays as the unexpected overlapping or merging of various concepts. This paper presents a general approach for text-to-image diffusion models to address the mutual interference between different subjects and their attachments in complex scenes, pursuing better text-image consistency. The core idea is to isolate the synthesizing processes of different concepts. We propose to bind each attachment to corresponding subjects separately with split text prompts. Besides, we introduce a revision method to fix the concept bleeding problem in multi-subject synthesis. We first depend on pre-trained object detection and segmentation models to obtain the layouts of subjects. Then we isolate and resynthesize each subject individually with corresponding text prompts to avoid mutual interference. Overall, we achieve a training-free strategy, named Isolated Diffusion, to optimize multi-concept text-to-image synthesis. It is compatible with the latest Stable Diffusion XL (SDXL) and prior Stable Diffusion (SD) models. We compare our approach with alternative methods using a variety of multi-concept text prompts and demonstrate its effectiveness with clear advantages in text-image consistency and user study.
大规模文本到图像的扩散模型在给定目标文本提示的情况下,已成功合成高质量和多样化的图像。尽管这些革命性的图像生成能力令人印象深刻,但当前最先进的模型仍然在许多情况下难以准确处理多概念生成。这种现象被称为“概念出血”,表现为各种概念的意外重叠或合并。本文提出了一种通用的文本到图像扩散模型方法,以解决复杂场景中不同主题及其附件之间的相互干扰,以追求更好的文本-图像一致性。核心思想是隔离不同概念的合成过程。我们提出通过拆分文本提示来将每个附件与相应的主题分别绑定。此外,我们引入了一种修正方法来解决多主题合成中的概念出血问题。我们首先依赖于预训练的目标检测和分割模型来获得主题布局。然后,我们分别使用相应的文本提示来隔离并重新合成每个主题,以避免相互干扰。总的来说,我们实现了一种名为“孤立扩散”的非训练策略,以优化多概念文本到图像的合成。它与最新的Stable Diffusion XL(SDXL)和之前的Stable Diffusion(SD)模型兼容。我们通过使用多种多概念文本提示将我们的方法与替代方法进行比较,并在文本-图像一致性和用户研究方面显示出其有效性及明显优势。
论文及项目相关链接
PDF Accepted by IEEE Transactions on Visualization and Computer Graphics
Summary
本文提出了一种针对文本到图像扩散模型的新方法,用于解决复杂场景中不同主题及其附件之间的相互干扰问题,从而提高文本与图像的一致性。通过分离不同概念的合成过程,采用分割文本提示将每个附件绑定到相应的主题上。同时,引入了一种修正方法来解决多主题合成中的概念混淆问题。通过依赖于预训练的目标检测和分割模型来获取主题布局,然后孤立并重新合成每个主题,以提高文本与图像的一致性。总体而言,这是一种名为“孤立扩散”的无训练策略,可优化多概念文本到图像的综合生成,与最新的Stable Diffusion XL和之前的Stable Diffusion模型兼容。
Key Takeaways
- 文本到图像扩散模型在合成高质量和多样化的图像方面取得了巨大成功,但在多概念生成方面仍存在挑战。
- 当前模型面临的概念混淆问题被称为“概念出血”,表现为各种概念的意外重叠或合并。
- 提出了一种新的方法来解决复杂场景中不同主题及其附件之间的干扰问题,以提高文本与图像的一致性。
- 通过分离不同概念的合成过程并采用分割文本提示来绑定每个附件到相应的主题。
- 引入了一种修正方法来处理多主题合成中的概念混淆问题,依赖于预训练的目标检测和分割模型来获取主题布局。
- 提出了一种名为“孤立扩散”的无训练策略,优化了多概念文本到图像的综合生成。
点此查看论文截图
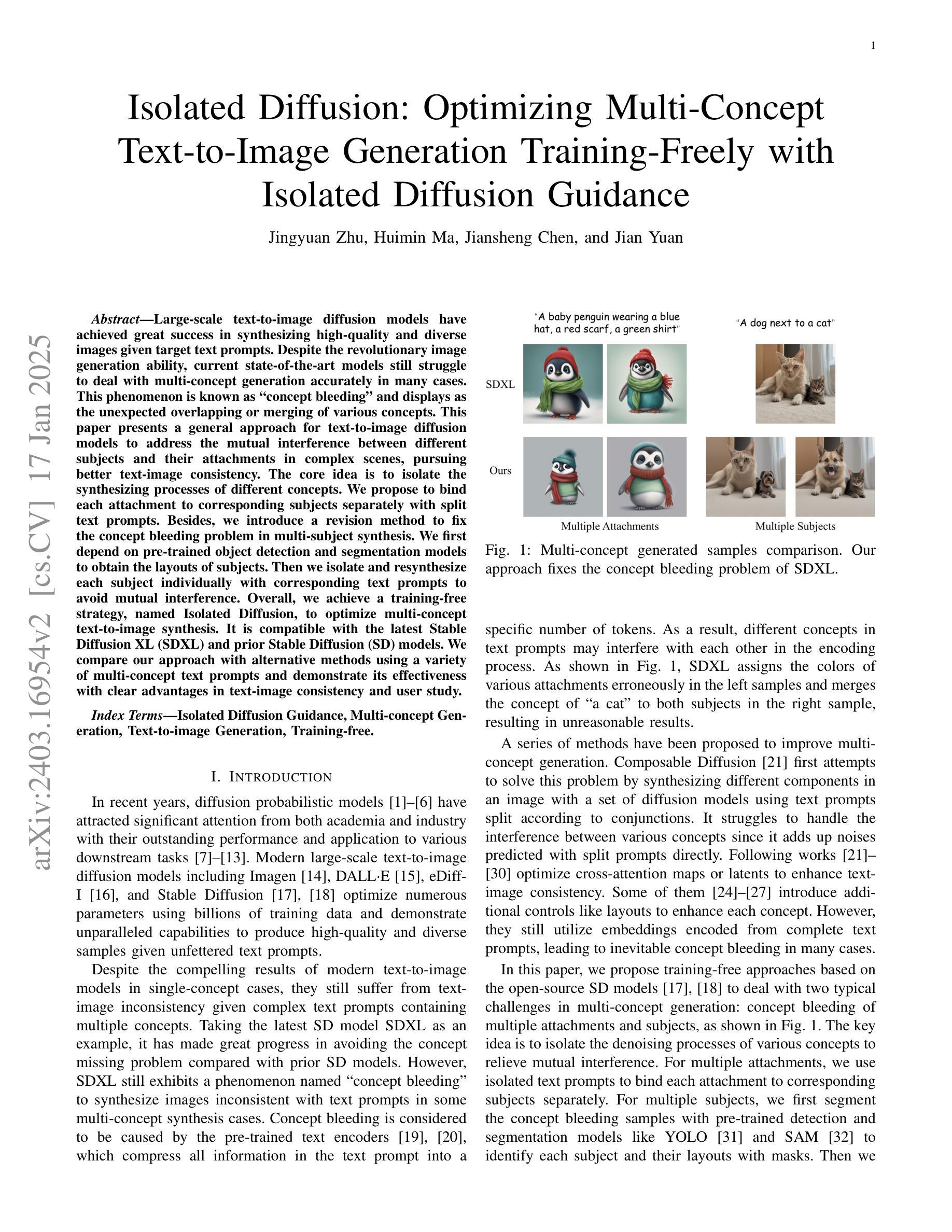
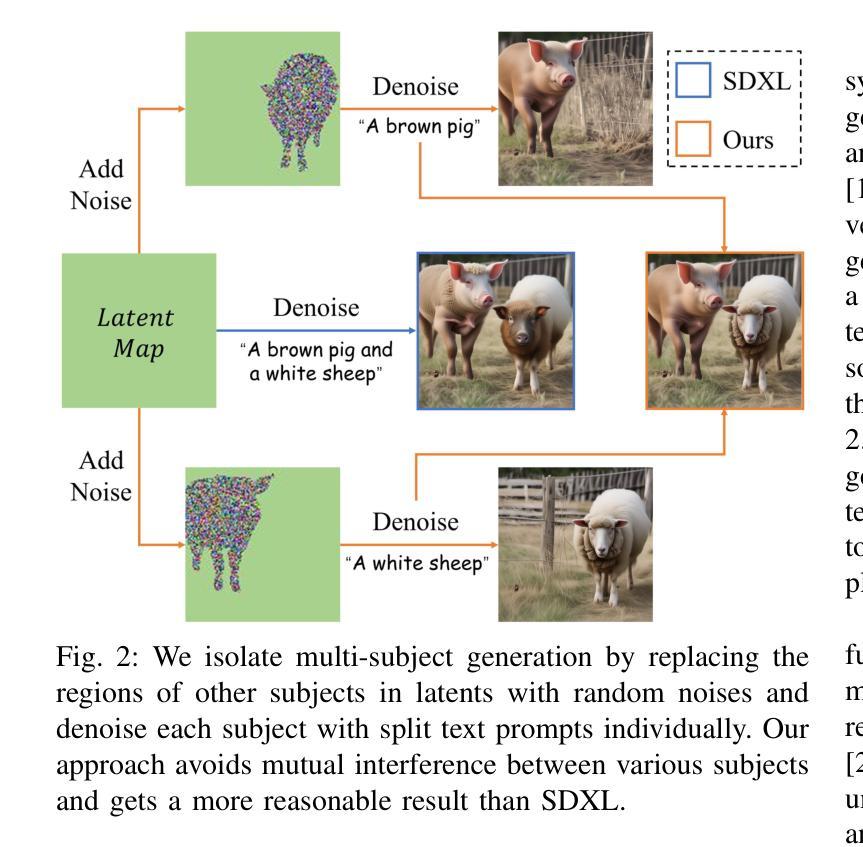
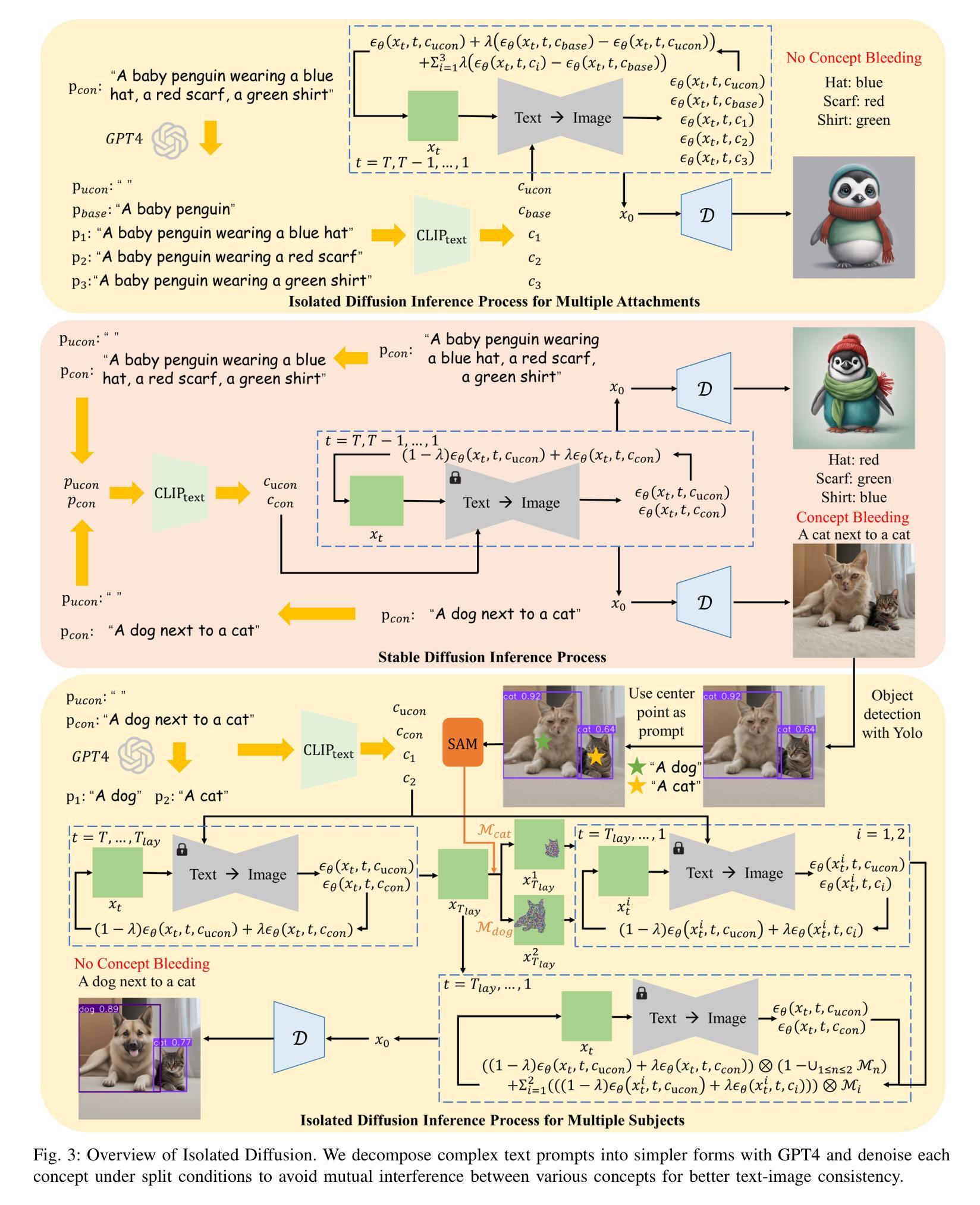
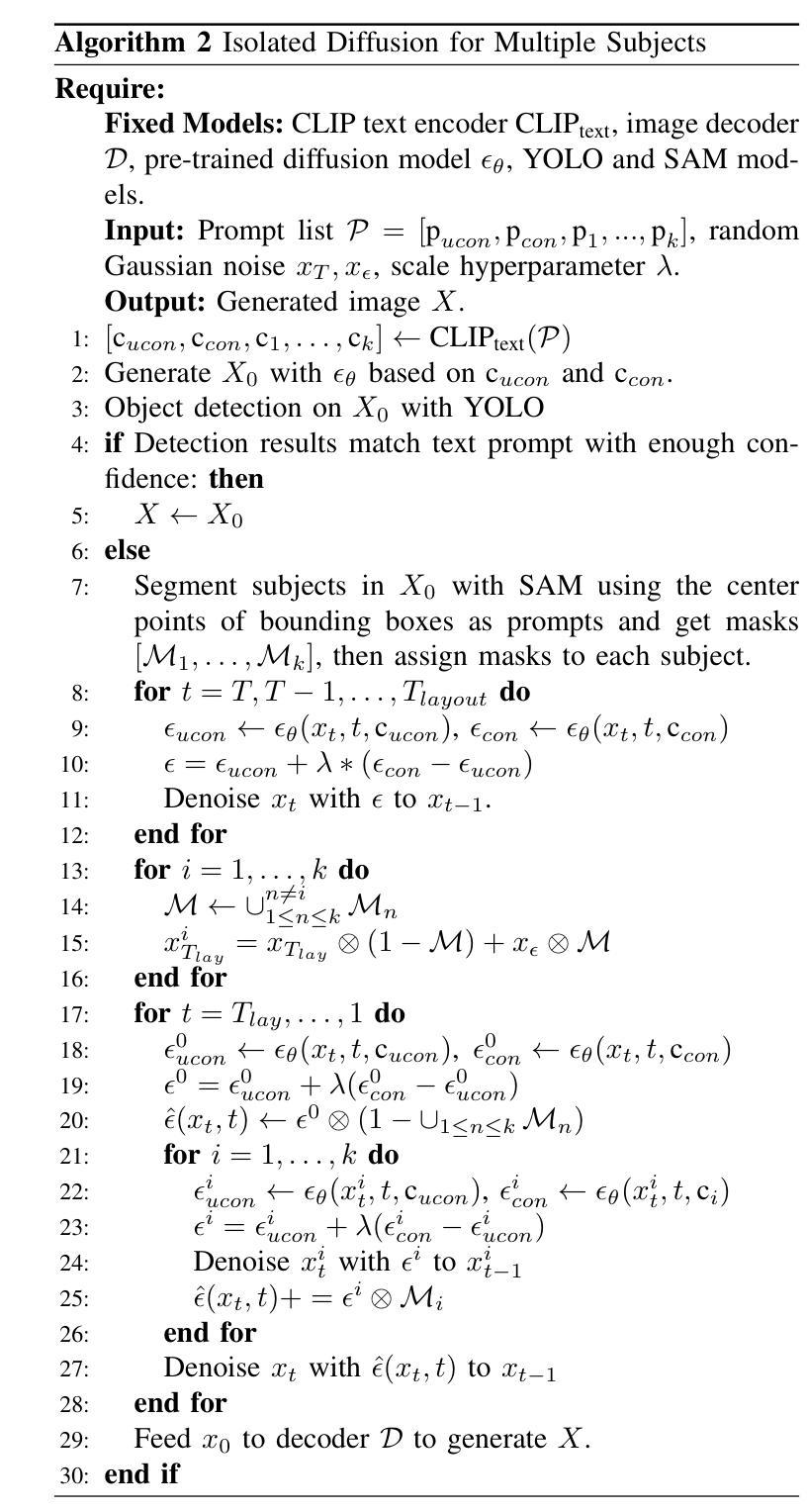
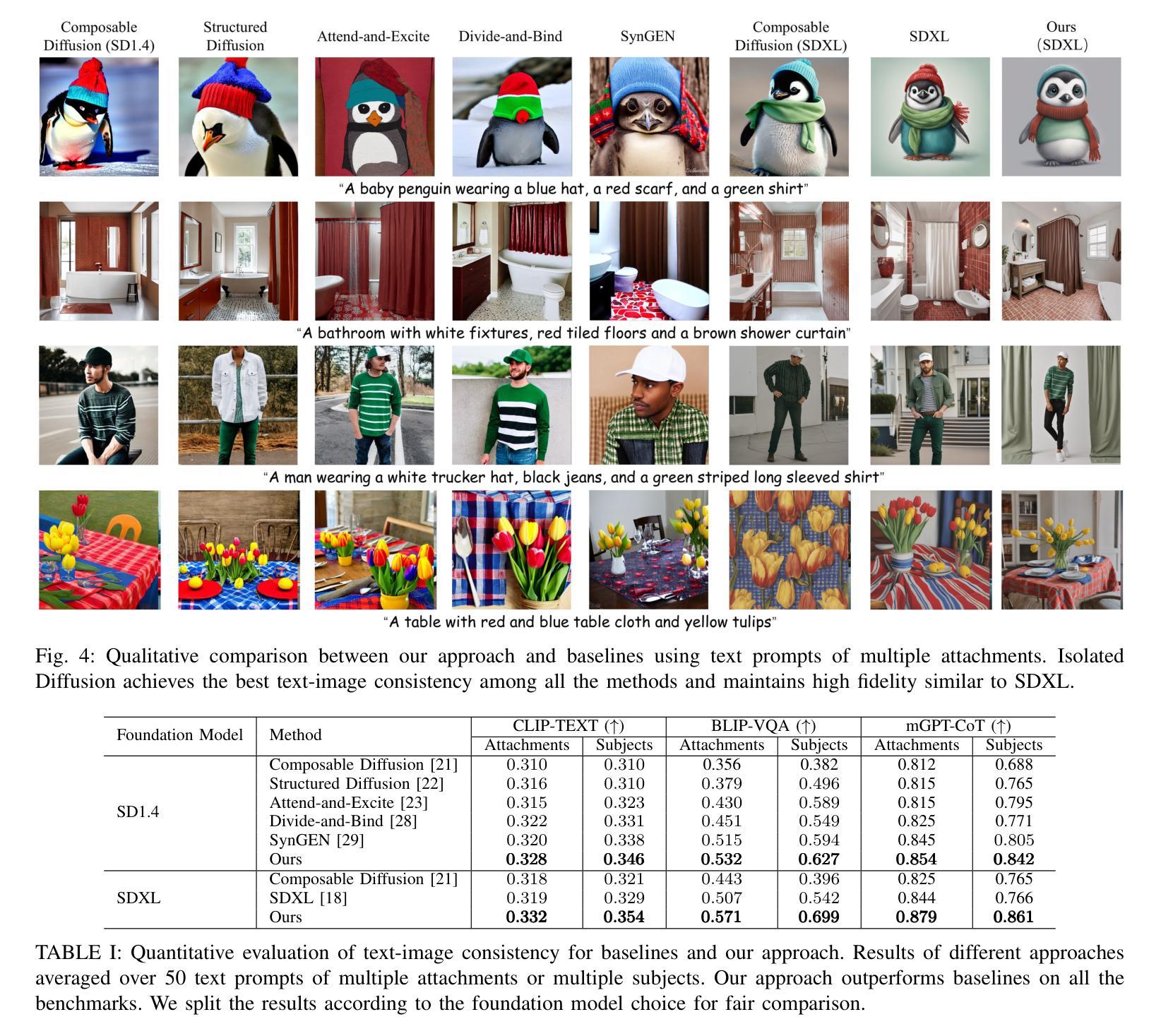
Generate E-commerce Product Background by Integrating Category Commonality and Personalized Style
Authors:Haohan Wang, Wei Feng, Yaoyu Li, Zheng Zhang, Jingjing Lv, Junjie Shen, Zhangang Lin, Jingping Shao
The state-of-the-art methods for e-commerce product background generation suffer from the inefficiency of designing product-wise prompts when scaling up the production, as well as the ineffectiveness of describing fine-grained styles when customizing personalized backgrounds for some specific brands. To address these obstacles, we integrate the category commonality and personalized style into diffusion models. Concretely, we propose a Category-Wise Generator to enable large-scale background generation with only one model for the first time. A unique identifier in the prompt is assigned to each category, whose attention is located on the background by a mask-guided cross attention layer to learn the category-wise style. Furthermore, for products with specific and fine-grained requirements in layout, elements, etc, a Personality-Wise Generator is devised to learn such personalized style directly from a reference image to resolve textual ambiguities, and is trained in a self-supervised manner for more efficient training data usage. To advance research in this field, the first large-scale e-commerce product background generation dataset BG60k is constructed, which covers more than 60k product images from over 2k categories. Experiments demonstrate that our method could generate high-quality backgrounds for different categories, and maintain the personalized background style of reference images. BG60k will be available at \url{https://github.com/Whileherham/BG60k}.
当前电子商务产品背景生成的最先进方法,在扩大生产规模时面临着产品设计提示效率低下的问题,以及在为某些特定品牌定制个性化背景时描述细微风格的不有效性。为了解决这些障碍,我们将类别共性与个性化风格融入扩散模型。具体来说,我们首次提出一种类别生成器,只需一个模型即可实现大规模的背景生成。每个类别在提示中都有一个唯一标识符,通过一个掩膜引导交叉注意力层将注意力集中在背景上,以学习类别风格。此外,对于在布局、元素等方面有特定和细微要求的产品,设计了一个个性化生成器,直接从参考图像学习个性化风格,以解决文本歧义问题,并以自我监督的方式进行训练,以更有效地利用训练数据。为了推动该领域的研究,构建了首个大规模电子商务产品背景生成数据集BG60k,该数据集涵盖了来自2k多个类别的超过6万张产品图像。实验表明,我们的方法能够为不同的类别生成高质量的背景,并保持参考图像的个性化背景风格。BG60k数据集将在\url{https://github.com/Whileherham/BG60k}上提供。
论文及项目相关链接
PDF Accepted by ICASSP 2025
Summary
在当前的电商产品背景生成方法中,随着产品数量的增长,为每一个产品设计专属提示语是非常低效的;同时为某些特定品牌定制精细风格背景时也存在描述不够精确的问题。为解决这些问题,研究团队结合扩散模型中的类别共性及个性化风格提出了创新的解决方案。首先,他们首次提出了一个类别导向生成器,只需一个模型就能实现大规模的背景生成。每个类别都有一个独特的标识符作为提示语,通过遮罩引导的交叉注意力层将其聚焦在背景上,以学习类别的风格特征。此外,为了满足具有特定细节要求的产品的布局和元素等需求,他们还设计了一个个性化生成器,可以从参考图像中学习个性化风格以解决文本歧义问题,并采用自我监督的方式进行训练以更有效地使用训练数据。为了推动该领域的研究进展,他们还构建了首个大规模的电商产品背景生成数据集BG60k,包含超过6万张来自超过两千个类别的产品图像。实验证明,该方法能够为不同类别的产品生成高质量背景,并保留参考图像的个性化背景风格。该数据集现已可在指定网站获取。
Key Takeaways
- 当前电商产品背景生成面临的挑战包括设计产品提示的低效性和描述精细风格的困难性。
- 研究团队通过结合扩散模型中的类别共性及个性化风格来解决上述问题。
- 提出类别导向生成器,实现大规模背景生成只需一个模型。每个类别有独特的标识符作为提示语。
- 设计个性化生成器以满足具有特定细节要求的产品的需求,从参考图像中学习个性化风格并解决文本歧义问题。
- 采用自我监督训练以提高训练数据使用效率。
- 研究团队构建了首个大规模的电商产品背景生成数据集BG60k,包含超过6万张来自两千个类别的产品图像。
点此查看论文截图
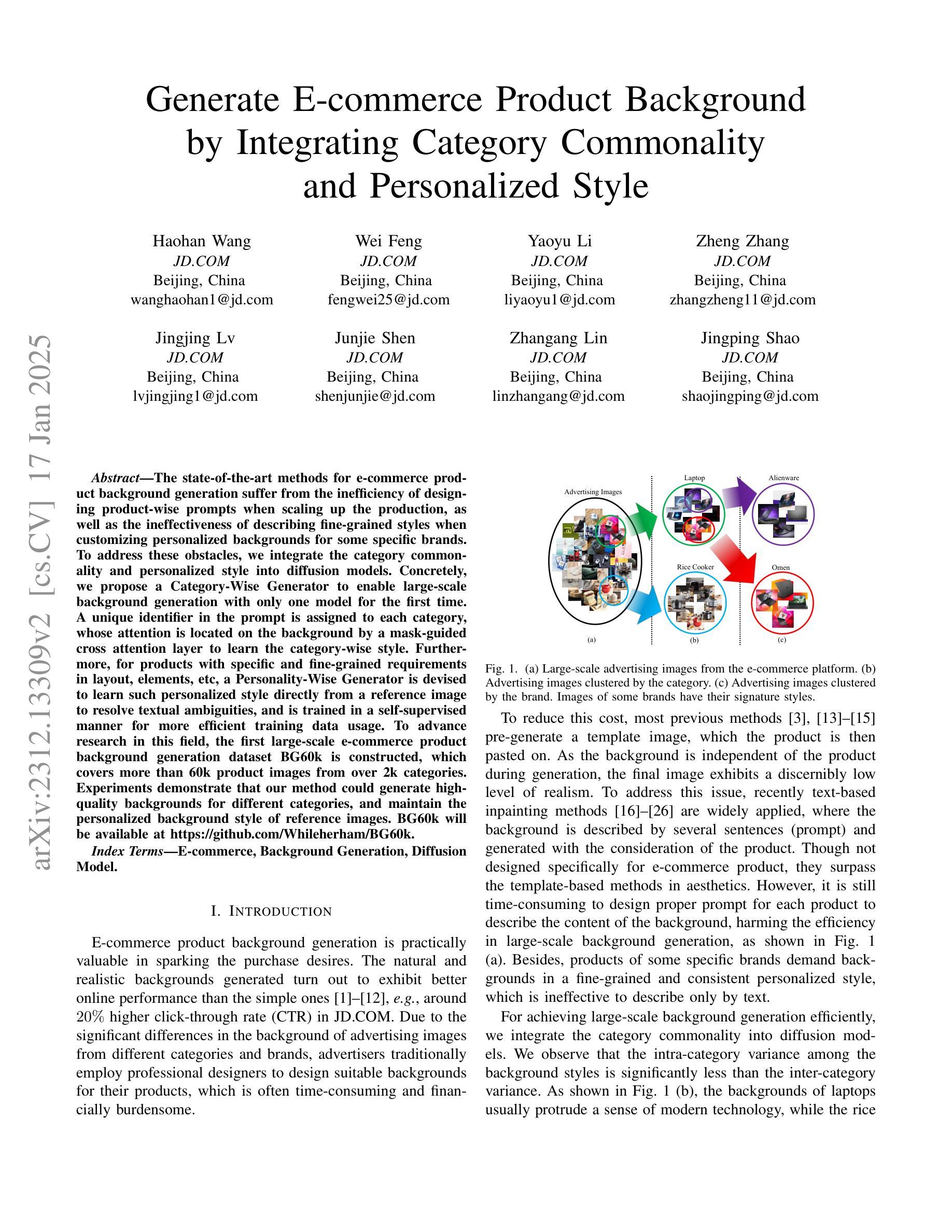
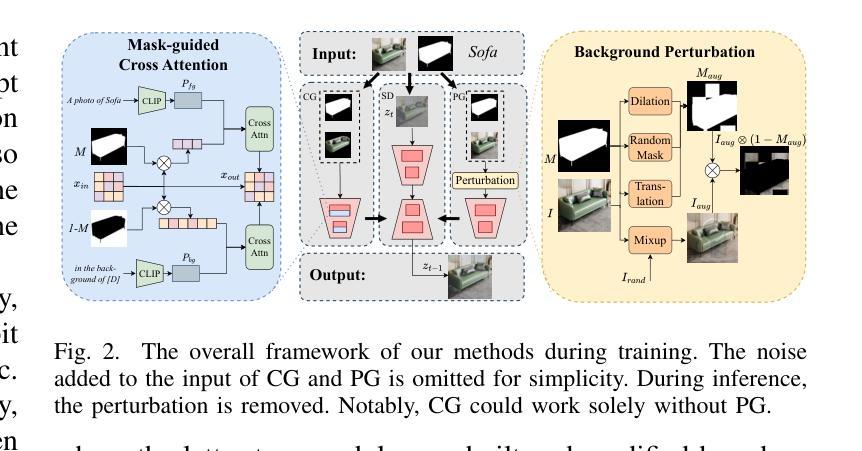
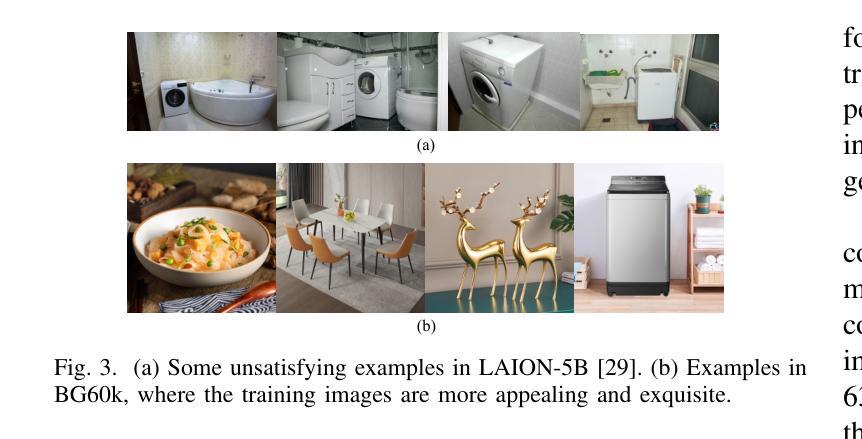
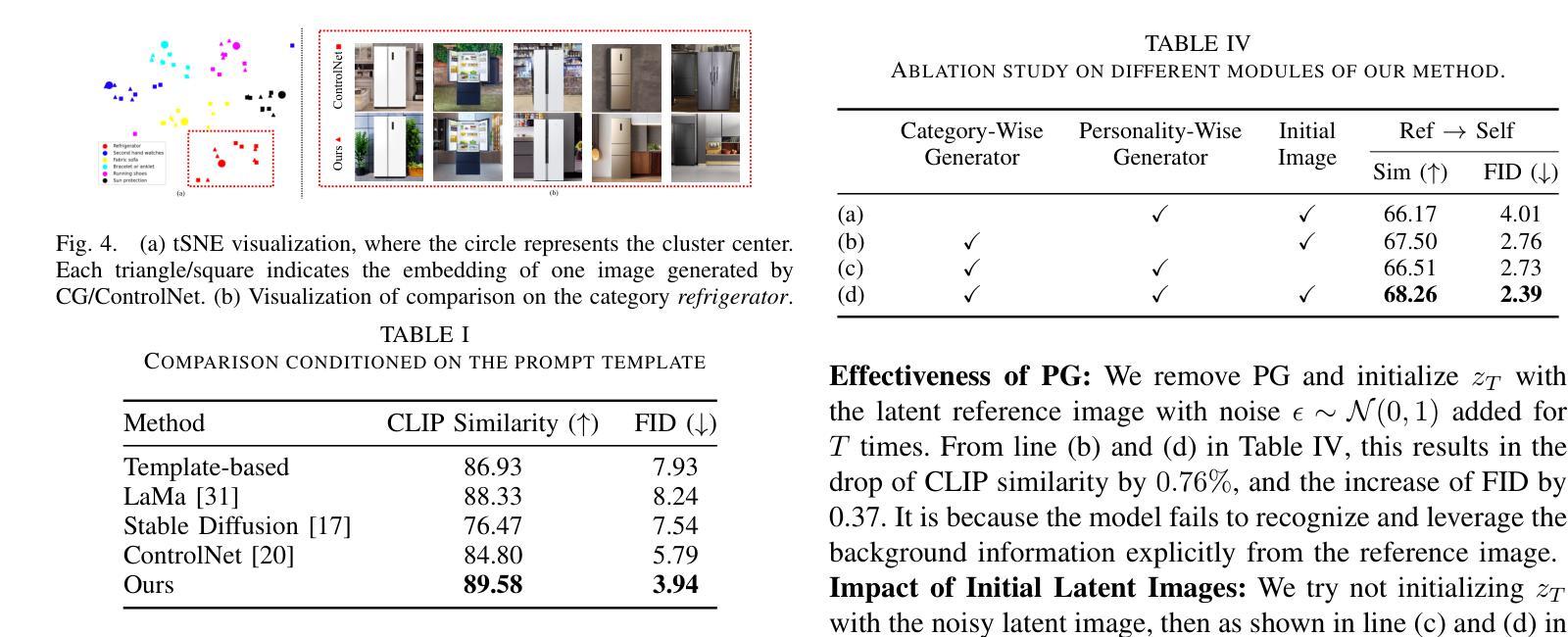
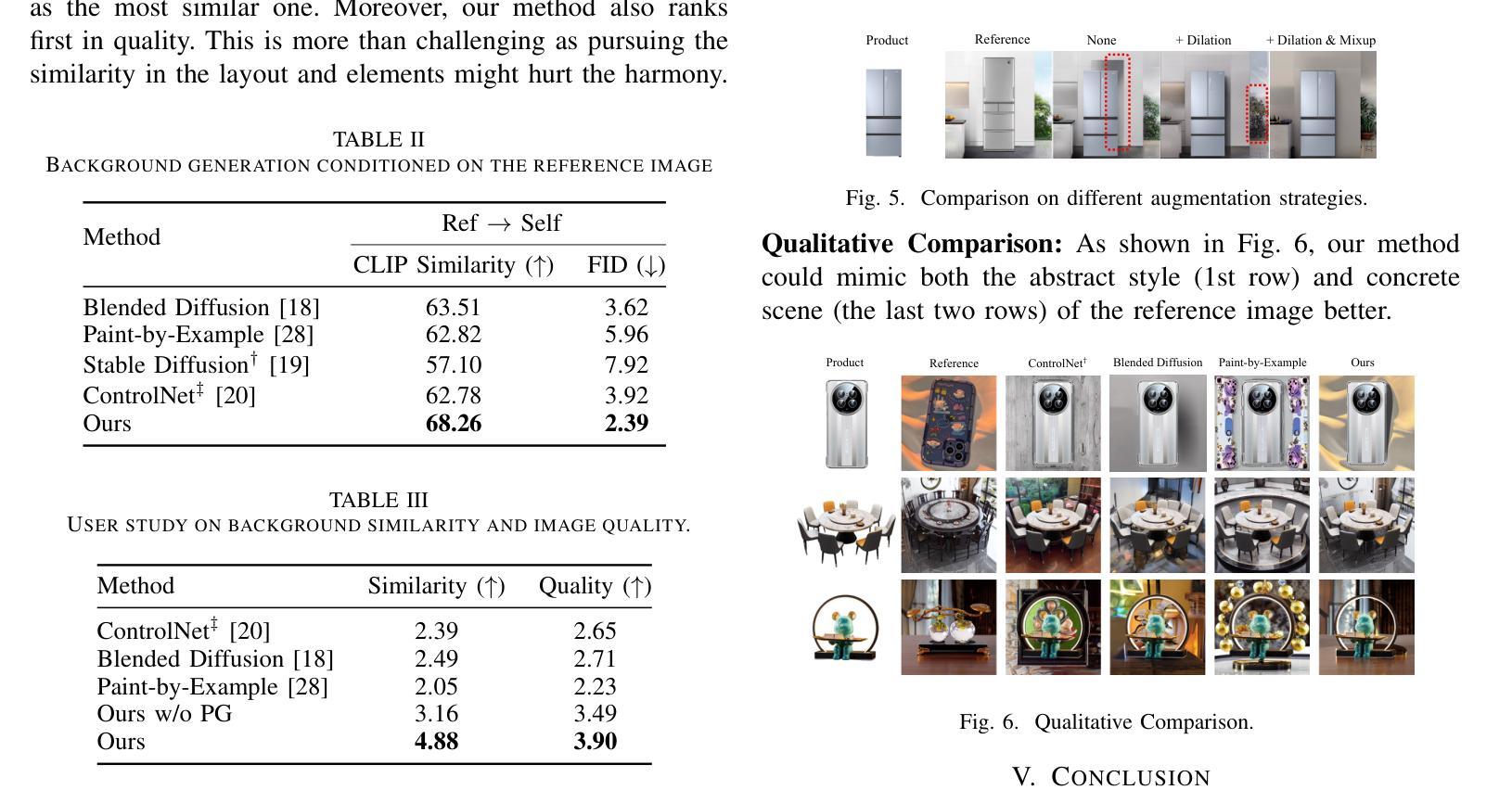