⚠️ 以下所有内容总结都来自于 大语言模型的能力,如有错误,仅供参考,谨慎使用
🔴 请注意:千万不要用于严肃的学术场景,只能用于论文阅读前的初筛!
💗 如果您觉得我们的项目对您有帮助 ChatPaperFree ,还请您给我们一些鼓励!⭐️ HuggingFace免费体验
2025-01-23 更新
Early Detection and Classification of Breast Cancer Using Deep Learning Techniques
Authors:Mst. Mumtahina Labonno, D. M. Asadujjaman, Md. Mahfujur Rahman, Abdullah Tamim, Mst. Jannatul Ferdous, Rafi Muttaki Mahi
Breast cancer is one of the deadliest cancers causing about massive number of patients to die annually all over the world according to the WHO. It is a kind of cancer that develops when the tissues of the breast grow rapidly and unboundly. This fatality rate can be prevented if the cancer is detected before it gets malignant. Using automation for early-age detection of breast cancer, Artificial Intelligence and Machine Learning technologies can be implemented for the best outcome. In this study, we are using the Breast Cancer Image Classification dataset collected from the Kaggle depository, which comprises 9248 Breast Ultrasound Images and is classified into three categories: Benign, Malignant, and Normal which refers to non-cancerous, cancerous, and normal images.This research introduces three pretrained model featuring custom classifiers that includes ResNet50, MobileNet, and VGG16, along with a custom CNN model utilizing the ReLU activation function.The models ResNet50, MobileNet, VGG16, and a custom CNN recorded accuracies of 98.41%, 97.91%, 98.19%, and 92.94% on the dataset, correspondingly, with ResNet50 achieving the highest accuracy of 98.41%.This model, with its deep and powerful architecture, is particularly successful in detecting aberrant cells as well as cancerous or non-cancerous tumors. These accuracies show that the Machine Learning methods are more compatible for the classification and early detection of breast cancer.
乳腺癌是致死率较高的癌症之一,世界卫生组织(WHO)的数据显示,全球每年都有大量患者因乳腺癌离世。乳腺癌是一种乳房组织快速且无序增长形成的癌症。在癌症恶化之前如能检测出来,则可以预防这种高死亡率。为了早期发现乳腺癌,可以采用自动化技术,应用人工智能和机器学习技术以达到最佳效果。本研究中,我们使用了来自Kaggle存储库的乳腺癌图像分类数据集,其中包含9248张乳腺超声图像,分为三类:良性、恶性和正常(分别指非癌性、癌性和正常图像)。本研究介绍了三种预训练模型,其中包括ResNet50、MobileNet和VGG16,以及使用ReLU激活函数的自定义CNN模型。ResNet50、MobileNet、VGG16和自定义CNN在数据集上的准确率分别为98.41%、97.91%、98.19%和92.94%,其中ResNet50的准确率最高,达到98.41%。该模型具有深度和强大的架构,特别擅长检测异常细胞以及癌性或非癌性肿瘤。这些准确率表明,机器学习方法更适用于乳腺癌的分类和早期检测。
论文及项目相关链接
Summary
乳腺癌是一种致死率较高的癌症,世界卫生组织数据显示其每年导致大量患者死亡。早期检测是降低死亡率的关键。本研究利用Kaggle存储库中的乳腺癌图像分类数据集,采用ResNet50、MobileNet、VGG16等预训练模型和自定义分类器,以及使用ReLU激活函数的自定义CNN模型。其中ResNet50模型准确率最高,达到98.41%,可有效检测异常细胞及癌或非癌肿瘤。研究结果表明,机器学习方法在乳腺癌分类和早期检测方面更具优势。
Key Takeaways
- 乳腺癌是一种致死率高的癌症,早期检测对降低死亡率至关重要。
- 研究使用了包含良性、恶性及正常乳腺超声图像的乳腺癌图像分类数据集。
- 研究采用了ResNet50、MobileNet、VGG16等预训练模型和自定义分类器以及自定义CNN模型。
- ResNet50模型在研究中表现出最高准确率,达到98.41%。
- ResNet50模型能够成功检测异常细胞以及癌或非癌肿瘤。
- 研究结果表明,机器学习方法在乳腺癌分类和早期检测方面更具优势。
点此查看论文截图
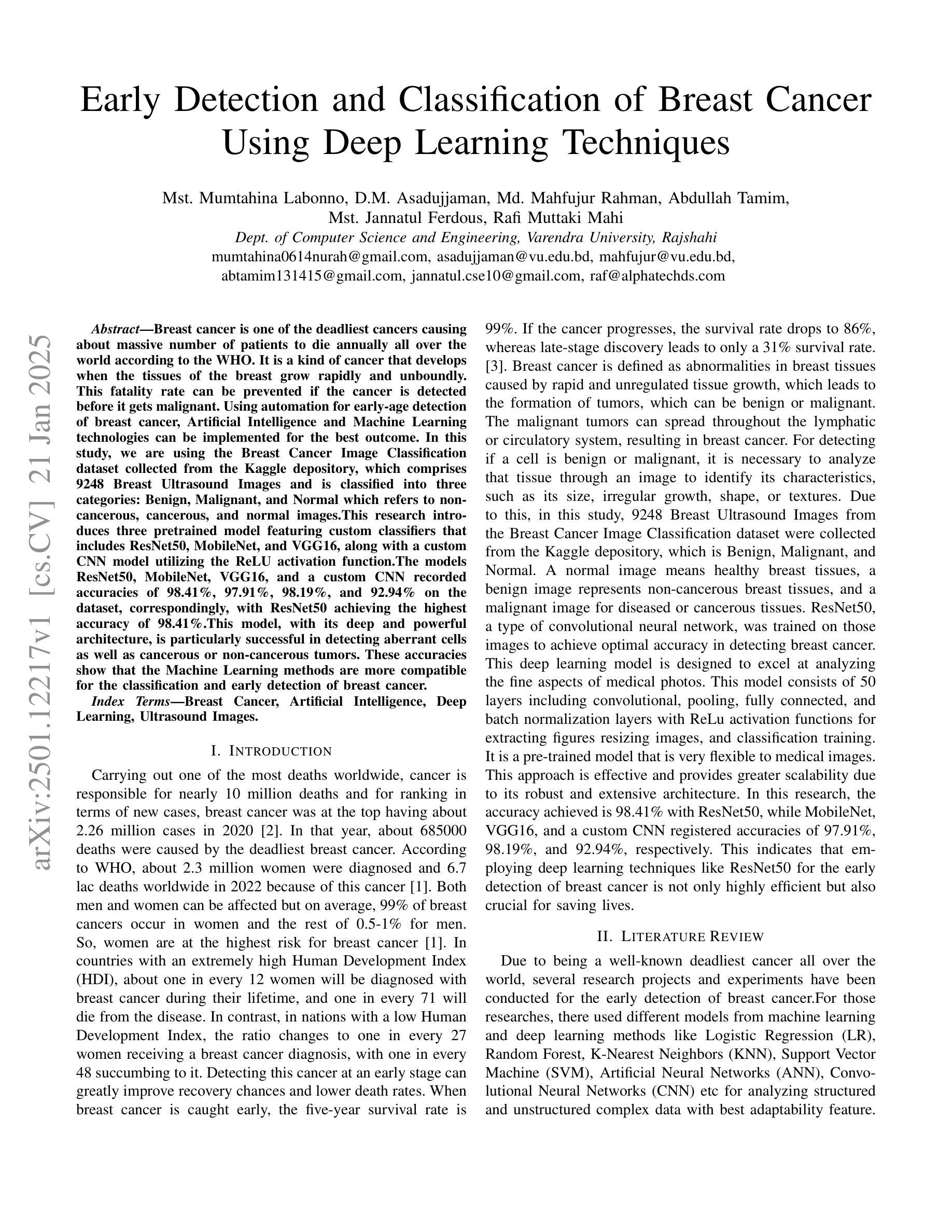
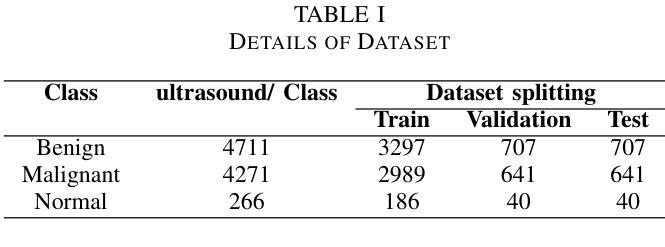
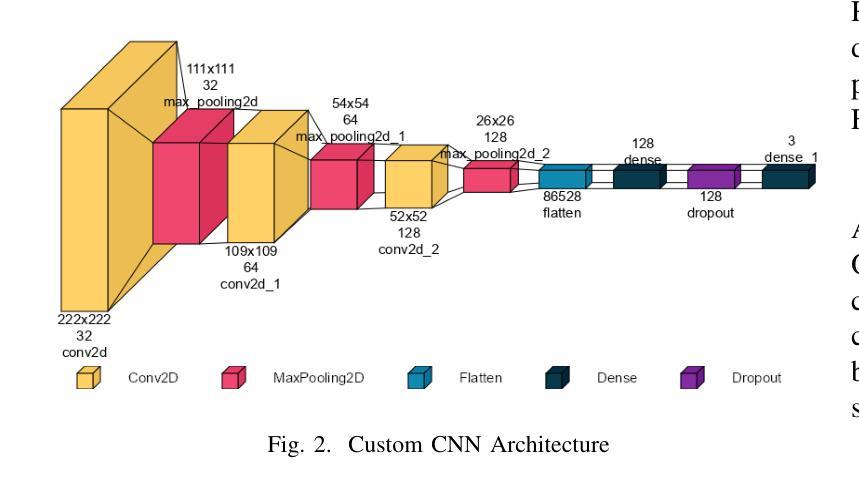
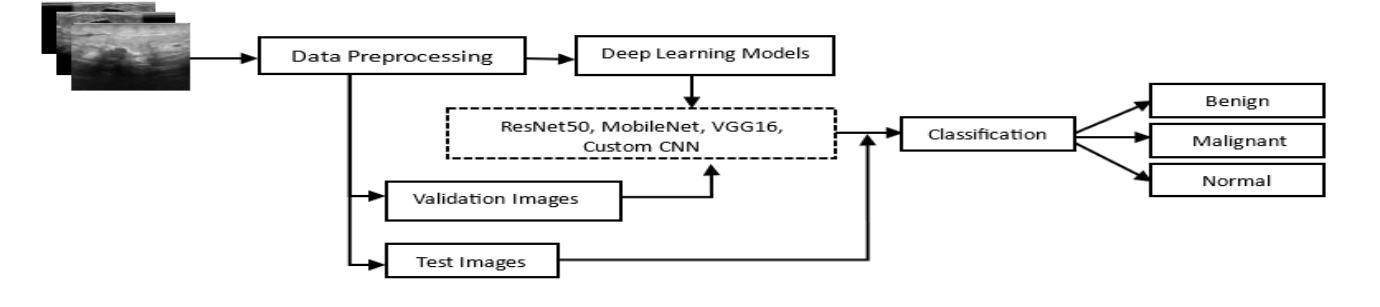
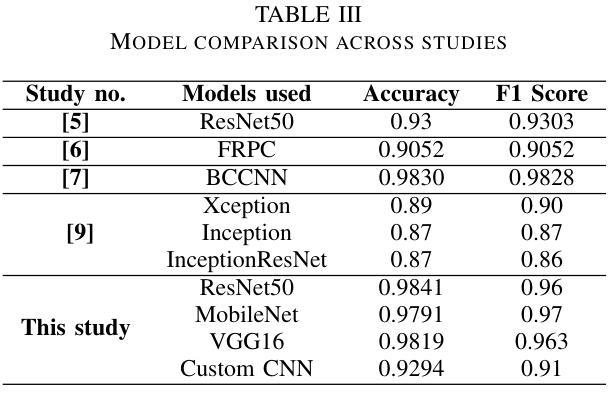
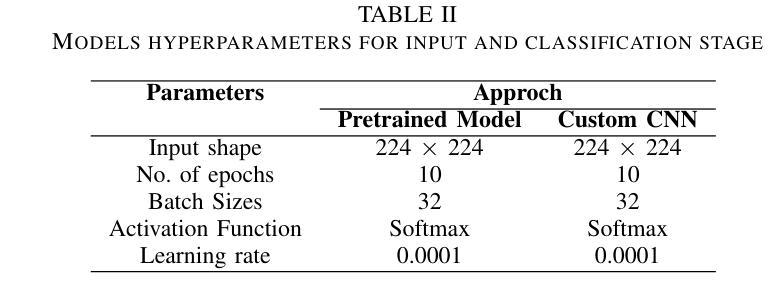
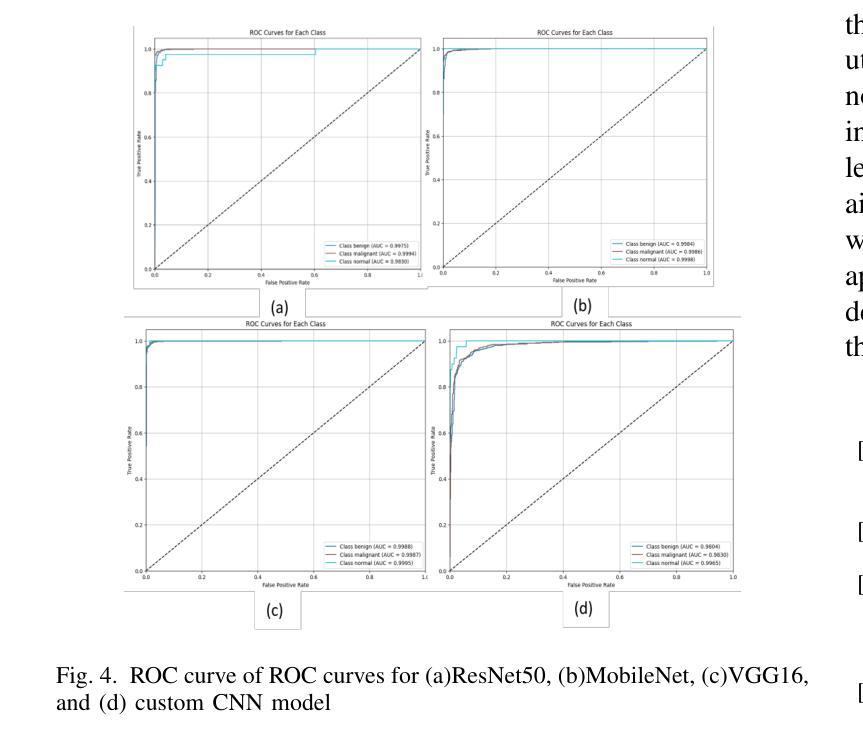
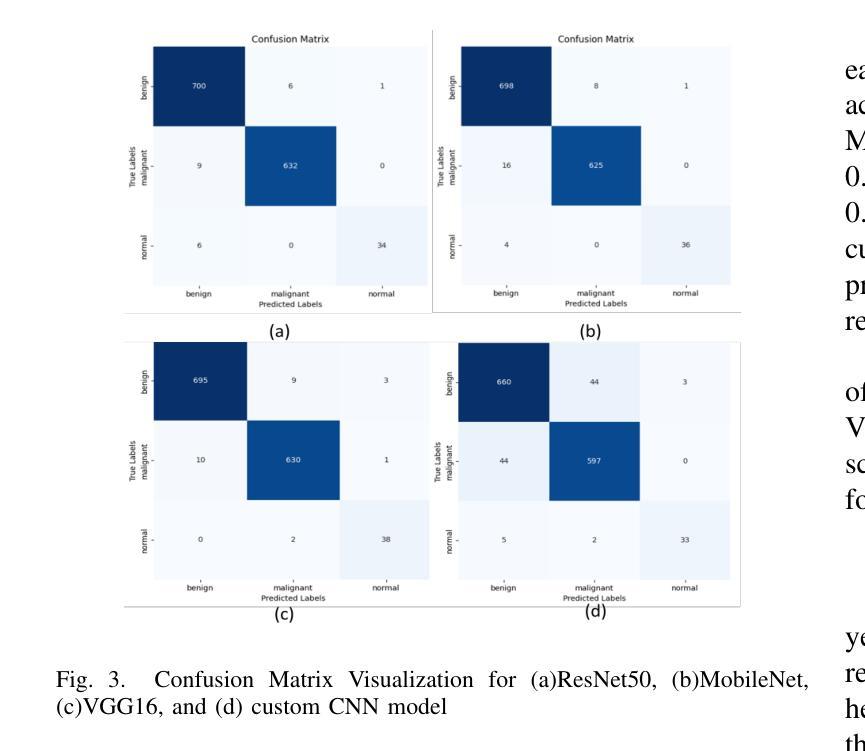
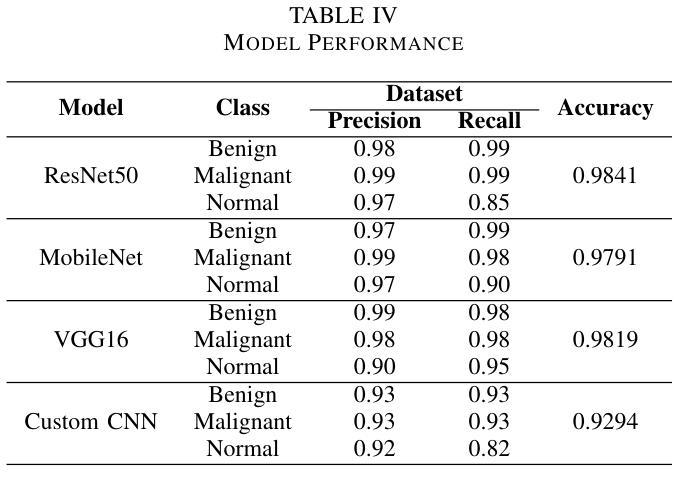