⚠️ 以下所有内容总结都来自于 大语言模型的能力,如有错误,仅供参考,谨慎使用
🔴 请注意:千万不要用于严肃的学术场景,只能用于论文阅读前的初筛!
💗 如果您觉得我们的项目对您有帮助 ChatPaperFree ,还请您给我们一些鼓励!⭐️ HuggingFace免费体验
2025-01-23 更新
Finding Reproducible and Prognostic Radiomic Features in Variable Slice Thickness Contrast Enhanced CT of Colorectal Liver Metastases
Authors:Jacob J. Peoples, Mohammad Hamghalam, Imani James, Maida Wasim, Natalie Gangai, Hyunseon Christine Kang, X. John Rong, Yun Shin Chun, Richard K. G. Do, Amber L. Simpson
Establishing the reproducibility of radiomic signatures is a critical step in the path to clinical adoption of quantitative imaging biomarkers; however, radiomic signatures must also be meaningfully related to an outcome of clinical importance to be of value for personalized medicine. In this study, we analyze both the reproducibility and prognostic value of radiomic features extracted from the liver parenchyma and largest liver metastases in contrast enhanced CT scans of patients with colorectal liver metastases (CRLM). A prospective cohort of 81 patients from two major US cancer centers was used to establish the reproducibility of radiomic features extracted from images reconstructed with different slice thicknesses. A publicly available, single-center cohort of 197 preoperative scans from patients who underwent hepatic resection for treatment of CRLM was used to evaluate the prognostic value of features and models to predict overall survival. A standard set of 93 features was extracted from all images, with a set of eight different extractor settings. The feature extraction settings producing the most reproducible, as well as the most prognostically discriminative feature values were highly dependent on both the region of interest and the specific feature in question. While the best overall predictive model was produced using features extracted with a particular setting, without accounting for reproducibility, (C-index = 0.630 (0.603–0.649)) an equivalent-performing model (C-index = 0.629 (0.605–0.645)) was produced by pooling features from all extraction settings, and thresholding features with low reproducibility ($\mathrm{CCC} \geq 0.85$), prior to feature selection. Our findings support a data-driven approach to feature extraction and selection, preferring the inclusion of many features, and narrowing feature selection based on reproducibility when relevant data is available.
建立放射学特征的重复性是在临床采用定量影像学标志物道路上的关键一步。然而,放射学特征还必须与具有临床重要性的结果有实际意义的关系,才能对个性化医疗有价值。在这项研究中,我们分析了从结直肠癌肝转移(CRLM)患者的增强CT扫描中提取的肝实质和最大肝转移灶的放射学特征的重复性和预后价值。使用来自美国两个主要癌症中心的81名患者的前瞻性队列,来建立从不同切片厚度重建的图像中提取的放射学特征的重复性。还使用了来自接受CRLM肝切除术患者的197例术前扫描的公开可用单中心队列,以评估特征的预后价值,并构建预测总体生存的模型。从所有图像中提取了93个标准特征集,使用了八种不同的提取器设置。产生最可重复和最具预后鉴别力的特征值的特征提取设置高度依赖于感兴趣区域和特定特征。虽然使用特定设置提取的特征产生了最佳的预测模型,但在不考虑可重复性的情况下,(C指数= 0.630(0.603–0.649))通过合并所有提取设置的特征,并对低可重复性的特征(CCC≥ 0.85)进行阈值处理,也产生了表现相当的模型(C指数= 0.629(0.605–0.645))。我们的研究结果支持数据驱动的特征提取和选择方法,更倾向于包含许多特征,并在有可用相关数据时进行基于可重复性的特征选择。
论文及项目相关链接
PDF Accepted for publication at the Journal of Machine Learning for Biomedical Imaging (MELBA) https://melba-journal.org/2024:032
摘要
本文研究了基于对比学习的无监督与半监督学习技术在放射组学特征的可重复性和预后价值分析中的应用。通过对来自两个美国主要癌症中心的81位患者的队列进行前瞻性研究,本文分析了放射组学特征的图像重建不同切片厚度下的可重复性。同时,使用公开的单中心队列中的197位患者的术前扫描图像,评估了放射组学特征预测总体生存率的预后价值。本文发现,最佳预测模型依赖于特定的特征提取设置,同时也考虑到了特征的可重复性。通过整合多种设置下的特征并基于可重复性进行特征选择,可以构建性能相当的模型。
关键见解
- 放射组学特征的可重复性和预后价值对于其在个性化医学中的价值至关重要。
- 通过前瞻性研究,验证了不同切片厚度下放射组学特征的可重复性。
- 在评估放射组学特征的预后价值时,使用了来自公开队列的大规模患者数据。
- 最佳预测模型依赖于特定的特征提取设置,同时也考虑到了特征的可重复性。
- 通过整合多种特征提取设置下的特征并基于高可重复性进行阈值和选择,可以构建性能相当的模型。
- 研究支持数据驱动的特征提取和选择方法,倾向于包含更多特征,并根据可重复性进行特征筛选。
点此查看论文截图
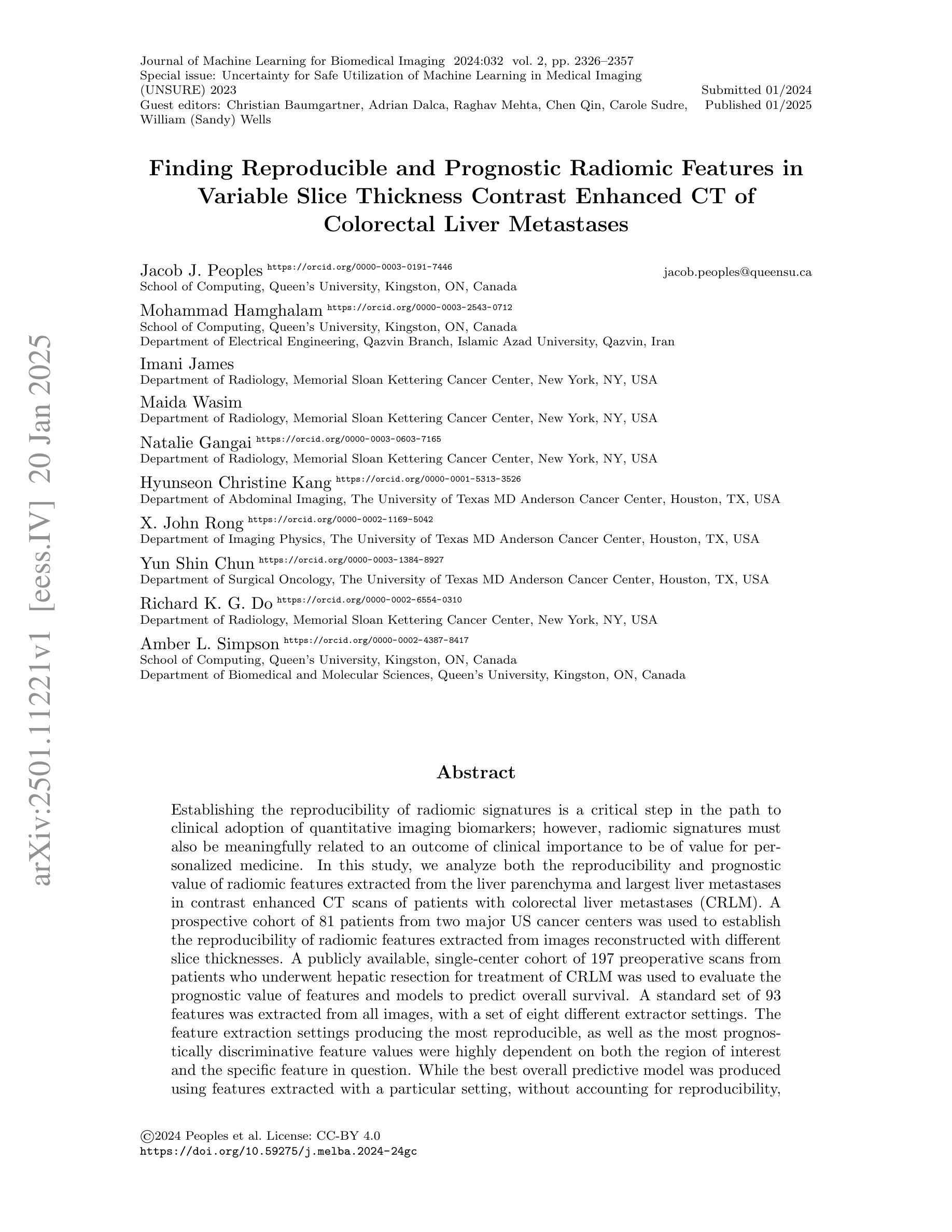
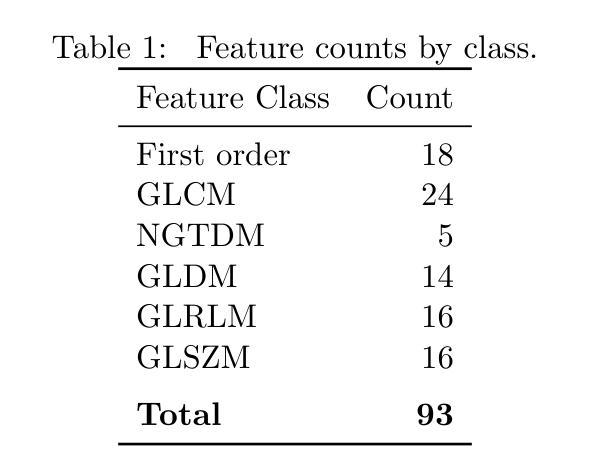