⚠️ 以下所有内容总结都来自于 大语言模型的能力,如有错误,仅供参考,谨慎使用
🔴 请注意:千万不要用于严肃的学术场景,只能用于论文阅读前的初筛!
💗 如果您觉得我们的项目对您有帮助 ChatPaperFree ,还请您给我们一些鼓励!⭐️ HuggingFace免费体验
2025-01-23 更新
MMVU: Measuring Expert-Level Multi-Discipline Video Understanding
Authors:Yilun Zhao, Lujing Xie, Haowei Zhang, Guo Gan, Yitao Long, Zhiyuan Hu, Tongyan Hu, Weiyuan Chen, Chuhan Li, Junyang Song, Zhijian Xu, Chengye Wang, Weifeng Pan, Ziyao Shangguan, Xiangru Tang, Zhenwen Liang, Yixin Liu, Chen Zhao, Arman Cohan
We introduce MMVU, a comprehensive expert-level, multi-discipline benchmark for evaluating foundation models in video understanding. MMVU includes 3,000 expert-annotated questions spanning 27 subjects across four core disciplines: Science, Healthcare, Humanities & Social Sciences, and Engineering. Compared to prior benchmarks, MMVU features three key advancements. First, it challenges models to apply domain-specific knowledge and perform expert-level reasoning to analyze specialized-domain videos, moving beyond the basic visual perception typically assessed in current video benchmarks. Second, each example is annotated by human experts from scratch. We implement strict data quality controls to ensure the high quality of the dataset. Finally, each example is enriched with expert-annotated reasoning rationals and relevant domain knowledge, facilitating in-depth analysis. We conduct an extensive evaluation of 32 frontier multimodal foundation models on MMVU. The latest System-2-capable models, o1 and Gemini 2.0 Flash Thinking, achieve the highest performance among the tested models. However, they still fall short of matching human expertise. Through in-depth error analyses and case studies, we offer actionable insights for future advancements in expert-level, knowledge-intensive video understanding for specialized domains.
我们介绍了MMVU,这是一个全面的专家级跨学科基准测试,用于评估视频理解中的基础模型。MMVU包含3000个专家注释的问题,涵盖四个核心学科的27个主题:科学、医疗、人文与社会科学和工程。与之前的基准测试相比,MMVU有三个关键进展。首先,它挑战模型应用特定领域的知识并进行专家级的推理,以分析专业领域视频,超越了当前视频基准测试中通常评估的基本视觉感知。其次,每个示例都是由人类专家从头开始进行注释的。我们实施严格的数据质量控制措施,以确保数据集的高质量。最后,每个示例都辅以专家注释的推理理由和相关领域知识,便于进行深入分析。我们在MMVU上对32个前沿的多模式基础模型进行了广泛评估。最新的具备System-2能力的模型,即o1和双子座2.0快速思考,在测试模型中表现最佳。然而,它们仍然未能达到人类专家的水平。通过深入的误差分析和案例研究,我们为未来在专家级知识密集型视频理解方面的专业领域的进步提供了可操作的见解。
论文及项目相关链接
Summary
本文介绍了MMVU,这是一个全面的专家级跨学科基准测试,用于评估视频理解中的基础模型。MMVU包含3000个专家标注的问题,涵盖四个核心学科的27个科目。相比之前的基准测试,MMVU有三个关键进步:应用领域专业知识、进行专家级别的分析评估特定领域的视频、每个例子均由人类专家从头开始标注,并实施了严格的数据质量控制以确保数据集的高质量。我们对前沿的32个多媒体基础模型进行了广泛评估,发现系统2能力模型以及Gemini 2.0 Flash Thinking表现最佳,但仍未达到人类专家水平。通过深入的错误分析和案例研究,为未来的专家级、知识密集型的视频理解提供了可操作见解。
Key Takeaways
- MMVU是一个用于评估视频理解模型的新基准测试,包括涉及多个学科的广泛问题集。
- MMVU挑战模型在特定领域进行专家级别的视频分析,超越当前视频基准测试通常评估的基本视觉感知。
- 每个例子均由人类专家从头开始标注,并实施了严格的数据质量控制。
- MMVU包含专家标注的推理理由和相关领域知识,便于深入分析。
- 最新系统如System-2和Gemini 2.0 Flash Thinking在测试中表现良好,但仍未达人类专家水平。
- 通过深入错误分析和案例研究,提供了对模型不足的深入理解。
点此查看论文截图
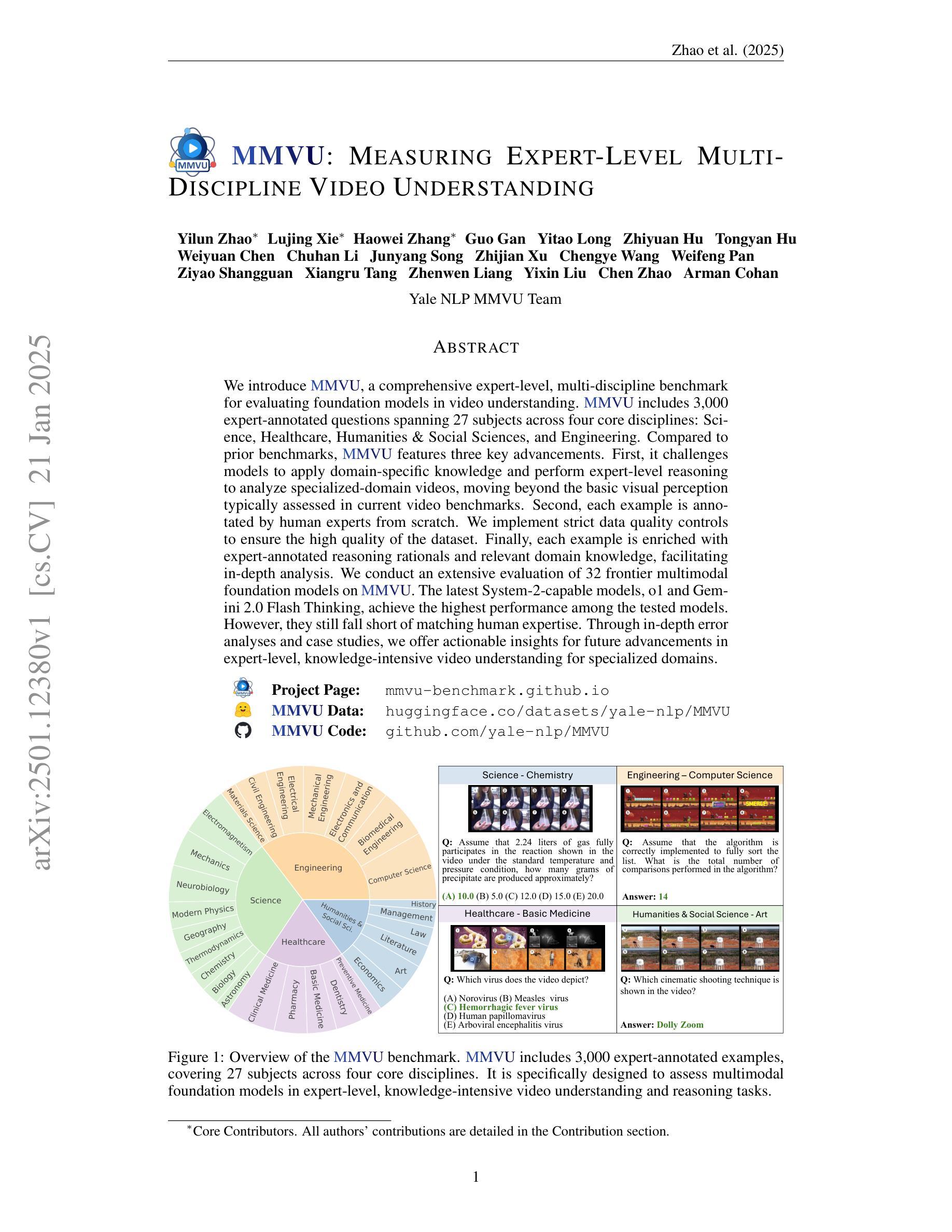
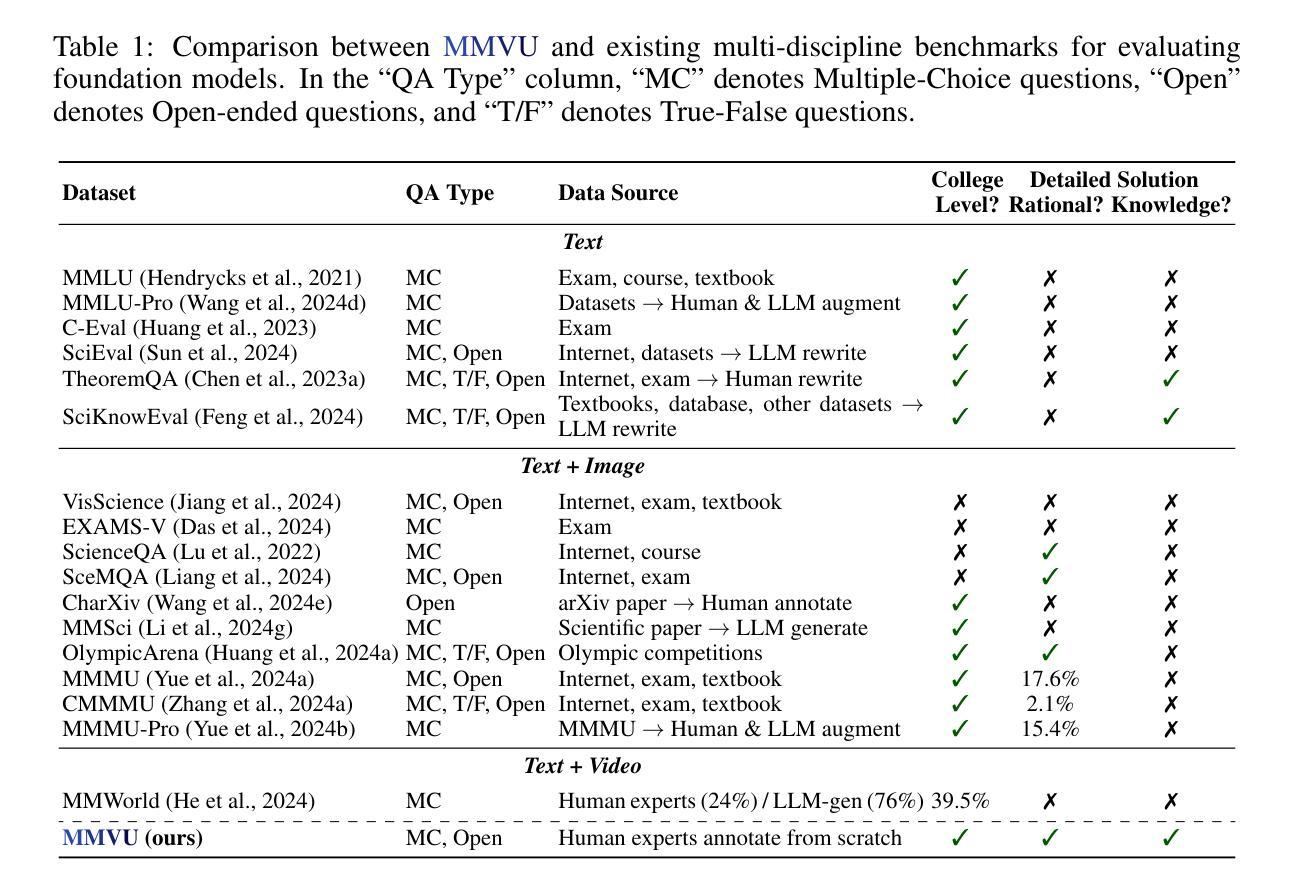
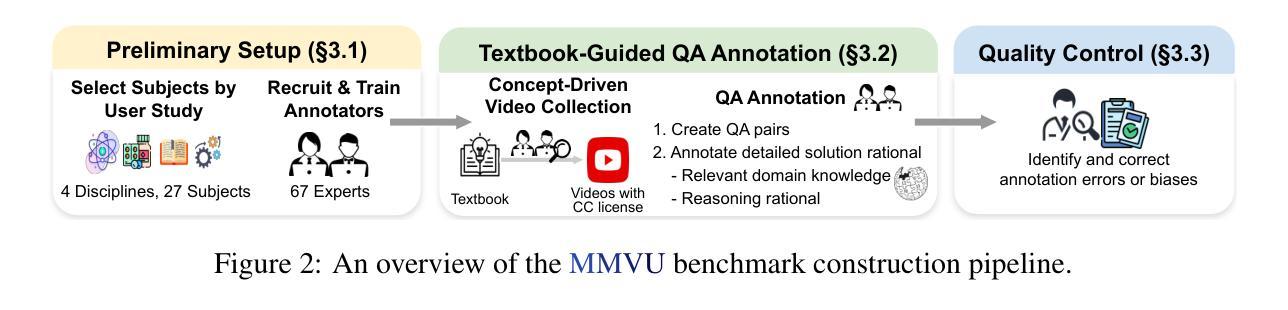

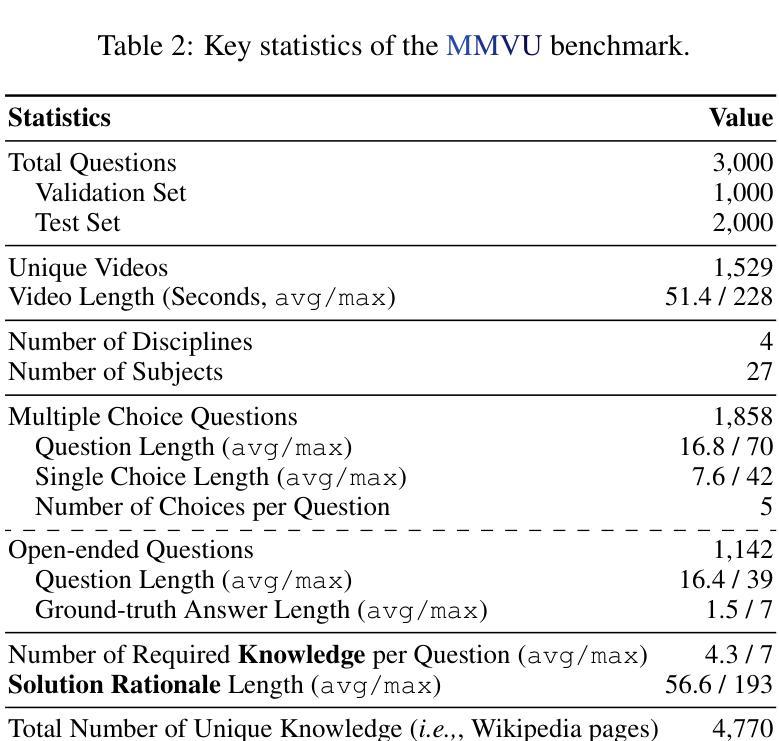
Neptune: The Long Orbit to Benchmarking Long Video Understanding
Authors:Arsha Nagrani, Mingda Zhang, Ramin Mehran, Rachel Hornung, Nitesh Bharadwaj Gundavarapu, Nilpa Jha, Austin Myers, Xingyi Zhou, Boqing Gong, Cordelia Schmid, Mikhail Sirotenko, Yukun Zhu, Tobias Weyand
We introduce Neptune, a benchmark for long video understanding that requires reasoning over long time horizons and across different modalities. Many existing video datasets and models are focused on short clips (10s-30s). While some long video datasets do exist, they can often be solved by powerful image models applied per frame (and often to very few frames) in a video, and are usually manually annotated at high cost. In order to mitigate both these problems, we propose a scalable dataset creation pipeline which leverages large models (VLMs and LLMs), to automatically generate dense, time-aligned video captions, as well as tough question answer decoy sets for video segments (up to 15 minutes in length). Our dataset Neptune covers a broad range of long video reasoning abilities and consists of a subset that emphasizes multimodal reasoning. Since existing metrics for open-ended question answering are either rule-based or may rely on proprietary models, we provide a new open source model-based metric GEM to score open-ended responses on Neptune. Benchmark evaluations reveal that most current open-source long video models perform poorly on Neptune, particularly on questions testing temporal ordering, counting and state changes. Through Neptune, we aim to spur the development of more advanced models capable of understanding long videos. The dataset is available at https://github.com/google-deepmind/neptune
我们介绍了Neptune,这是一个针对长视频理解的基准测试,它需要在长时间范围和不同模式之间进行推理。许多现有的视频数据集和模型都专注于短视频片段(10秒至30秒)。虽然也有一些长视频数据集存在,但它们通常可以通过应用在视频的每一帧(通常是很少的帧)上的强大图像模型来解决,并且通常是高成本的手动标注。为了解决这两个问题,我们提出了一种可扩展的数据集创建流程,它利用大型模型(视觉语言模型和大型语言模型)自动生成密集、时间对齐的视频字幕,以及为视频片段(长达15分钟)自动生成问题答案的干扰集。我们的数据集Neptune涵盖了广泛的长期视频理解能力,并包含了一个强调多模式推理的子集。由于现有的开放式问答指标要么是规则基础的,要么可能依赖于专有模型,因此我们提供了一个新的开源模型指标GEM来对Neptune上的开放式答案进行评分。基准评估显示,大多数当前的开源长视频模型在Neptune上的表现不佳,特别是在测试时间顺序、计数和状态更改的问题方面。通过Neptune,我们旨在促进更先进模型的发展,使其能够理解长视频。数据集可在https://github.com/google-deepmind/neptune找到。
论文及项目相关链接
Summary:
海王星是一个针对长视频理解的新基准测试集,要求跨不同模态进行长时间推理。现有视频数据集和模型大多聚焦于短片段(10秒至30秒)。虽然也存在一些长视频数据集,但它们可以通过应用于视频的每一帧的强大图像模型来解决,且通常人工标注成本高昂。为了缓解这两个问题,我们提出了一个可扩展的数据集创建流程,利用大型模型自动生成密集、时间对齐的视频字幕,以及针对视频片段(长达15分钟)的难题问答选项集。海王星数据集涵盖了广泛的长视频推理能力,并包含强调多模态推理的子集。由于现有的开放问答指标要么是规则基础的,要么可能依赖于专有模型,我们提供新的开源模型基础度量GEM来评估海王星上的开放式回答。基准评估表明,大多数当前的开源长视频模型在海王星上的表现不佳,特别是在测试时间顺序、计数和状态变化的问题方面。通过海王星,我们旨在促进更先进模型的发展,使其能够理解和处理长视频。
Key Takeaways:
- 海王星是一个针对长视频理解的新基准测试集。
- 现有视频数据集和模型大多局限于短片段。
- 我们提出了一个可扩展的数据集创建流程来自动生成密集的视频字幕和问答选项集。
- 海王星数据集涵盖广泛的长视频推理能力,包括多模态推理。
- 提供新的开源模型基础度量GEM来评估开放式回答。
- 大多数现有模型在海王星上的表现不佳,特别是在时间顺序、计数和状态变化方面的测试。
点此查看论文截图
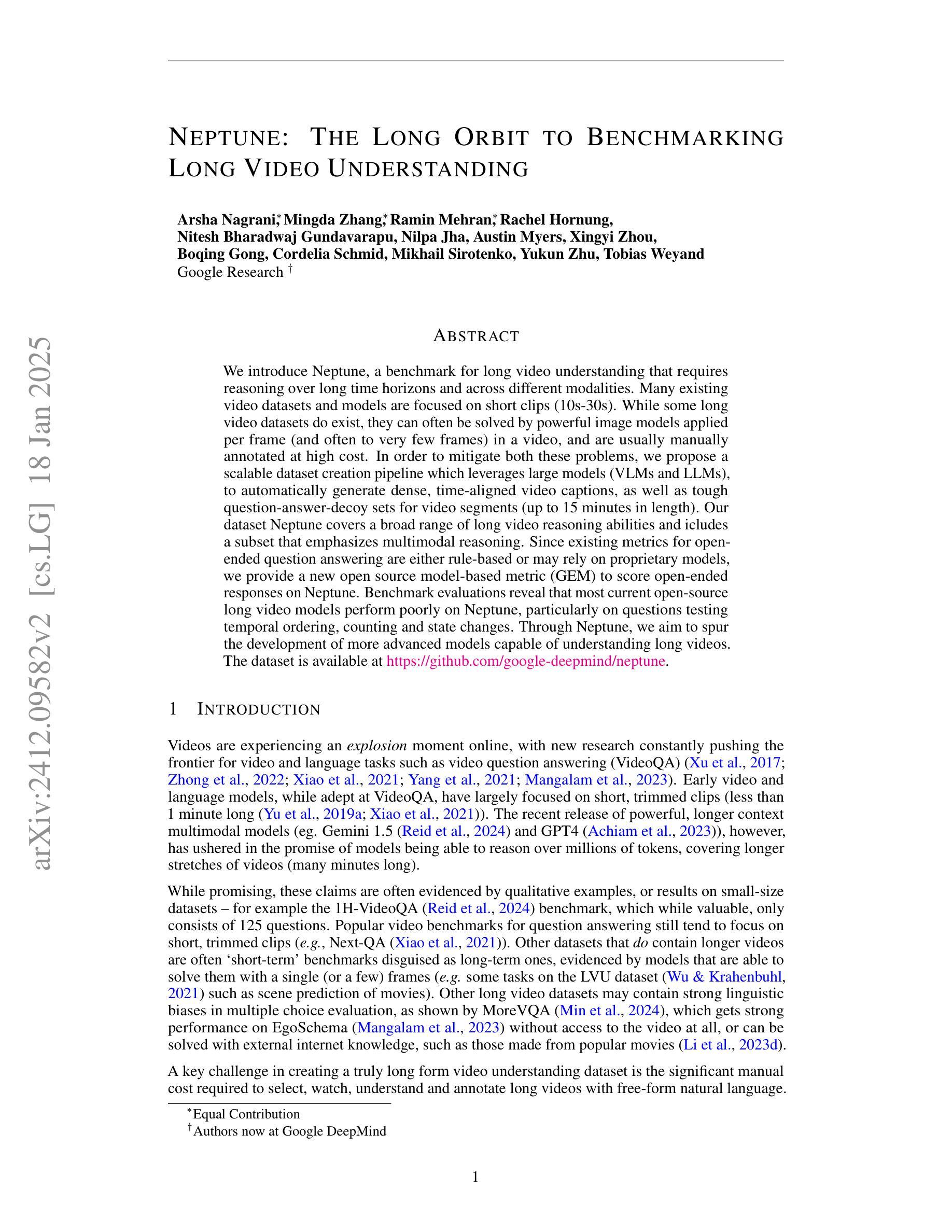
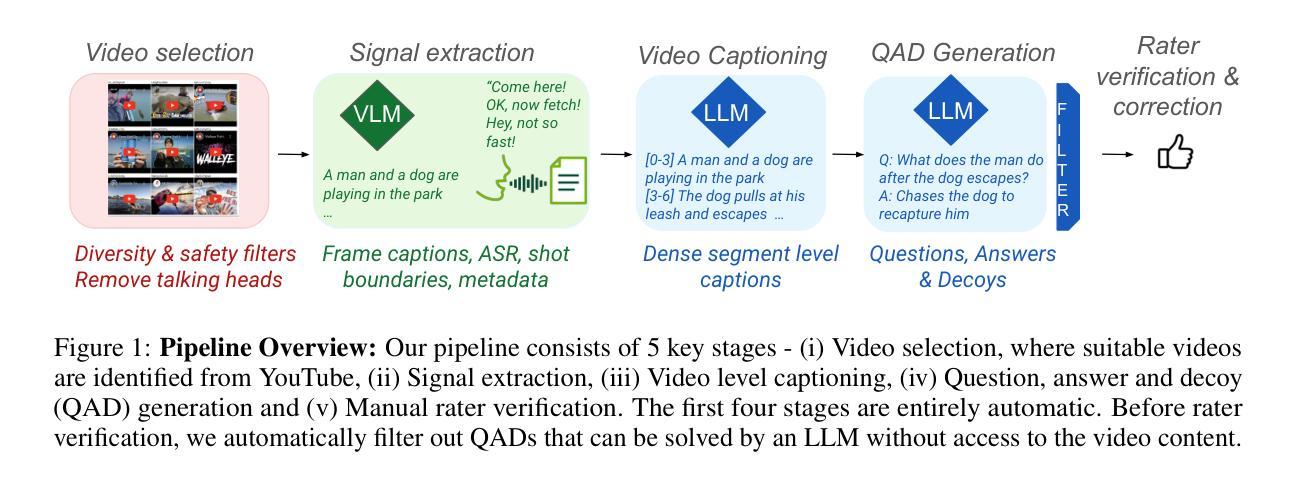
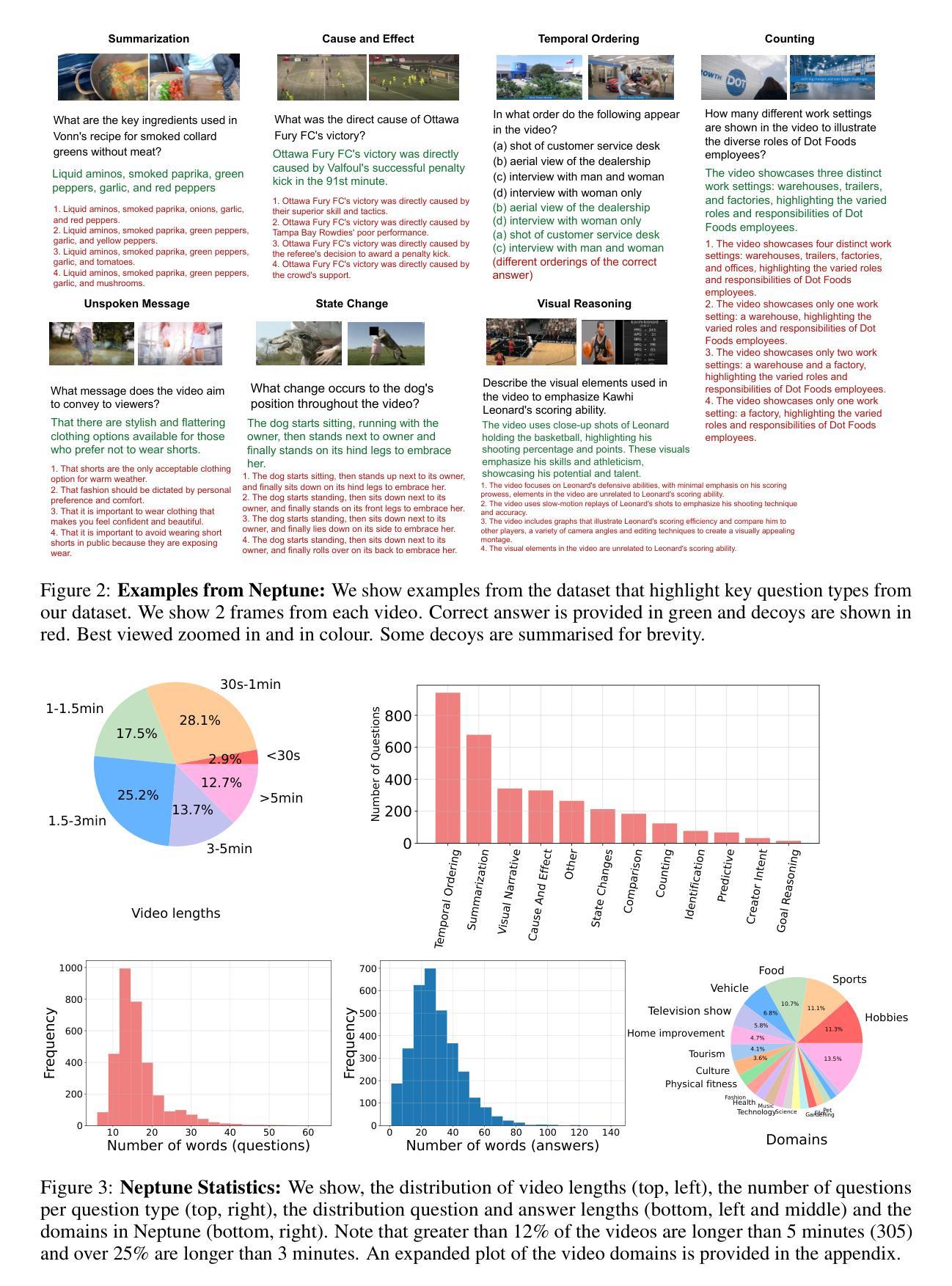
Transformer-Based Model for Monocular Visual Odometry: A Video Understanding Approach
Authors:André O. Françani, Marcos R. O. A. Maximo
Estimating the camera’s pose given images from a single camera is a traditional task in mobile robots and autonomous vehicles. This problem is called monocular visual odometry and often relies on geometric approaches that require considerable engineering effort for a specific scenario. Deep learning methods have been shown to be generalizable after proper training and with a large amount of available data. Transformer-based architectures have dominated the state-of-the-art in natural language processing and computer vision tasks, such as image and video understanding. In this work, we deal with the monocular visual odometry as a video understanding task to estimate the 6 degrees of freedom of a camera’s pose. We contribute by presenting the TSformer-VO model based on spatio-temporal self-attention mechanisms to extract features from clips and estimate the motions in an end-to-end manner. Our approach achieved competitive state-of-the-art performance compared with geometry-based and deep learning-based methods on the KITTI visual odometry dataset, outperforming the DeepVO implementation highly accepted in the visual odometry community. The code is publicly available at https://github.com/aofrancani/TSformer-VO.
根据单一相机的图像估计相机的姿态是移动机器人和自动驾驶汽车中的一个传统任务。这个问题被称为单目视觉里程计,通常依赖于需要针对特定场景进行大量工程努力的几何方法。深度学习方法在适当的训练和大批量可用数据之后被证明具有良好的通用性。基于Transformer的架构在诸如图像和视频理解等自然语言处理和计算机视觉任务中占据了最先进的地位。在这项工作中,我们将单目视觉里程计作为视频理解任务来处理,以估计相机的六自由度姿态。我们提出了一种基于时空自注意力机制的TSformer-VO模型,该模型可以从片段中提取特征并以端到端的方式估计运动。我们的方法在KITTI视觉里程计数据集上与基于几何和基于深度学习方法相比,取得了具有竞争力的最先进的性能表现,并且超越了视觉里程计社区中广受欢迎的DeepVO实现。代码公开可访问于:https://github.com/aofrancani/TSformer-VO。
论文及项目相关链接
PDF This work has been accepted for publication in IEEE Access
Summary
本文介绍了基于Transformer架构的TSformer-VO模型,用于解决移动机器人和自动驾驶车辆中的单目视觉里程计问题。该模型利用时空自注意力机制从视频片段中提取特征,以端到端的方式估计相机姿态的六自由度运动。在KITTI视觉里程计数据集上,该模型相较于基于几何和深度学习的方法表现出竞争力,尤其是相较于视觉里程计社区广泛接受的DeepVO实现有更好的性能。
Key Takeaways
- TSformer-VO模型基于Transformer架构,用于解决单目视觉里程计问题。
- 模型利用时空自注意力机制从视频片段中提取特征。
- 该模型能端到端地估计相机姿态的六自由度运动。
- TSformer-VO在KITTI视觉里程计数据集上表现出竞争力。
- 该模型相较于视觉里程计社区广泛接受的DeepVO实现有更好的性能。
- TSformer-VO模型的代码已公开可访问。
点此查看论文截图
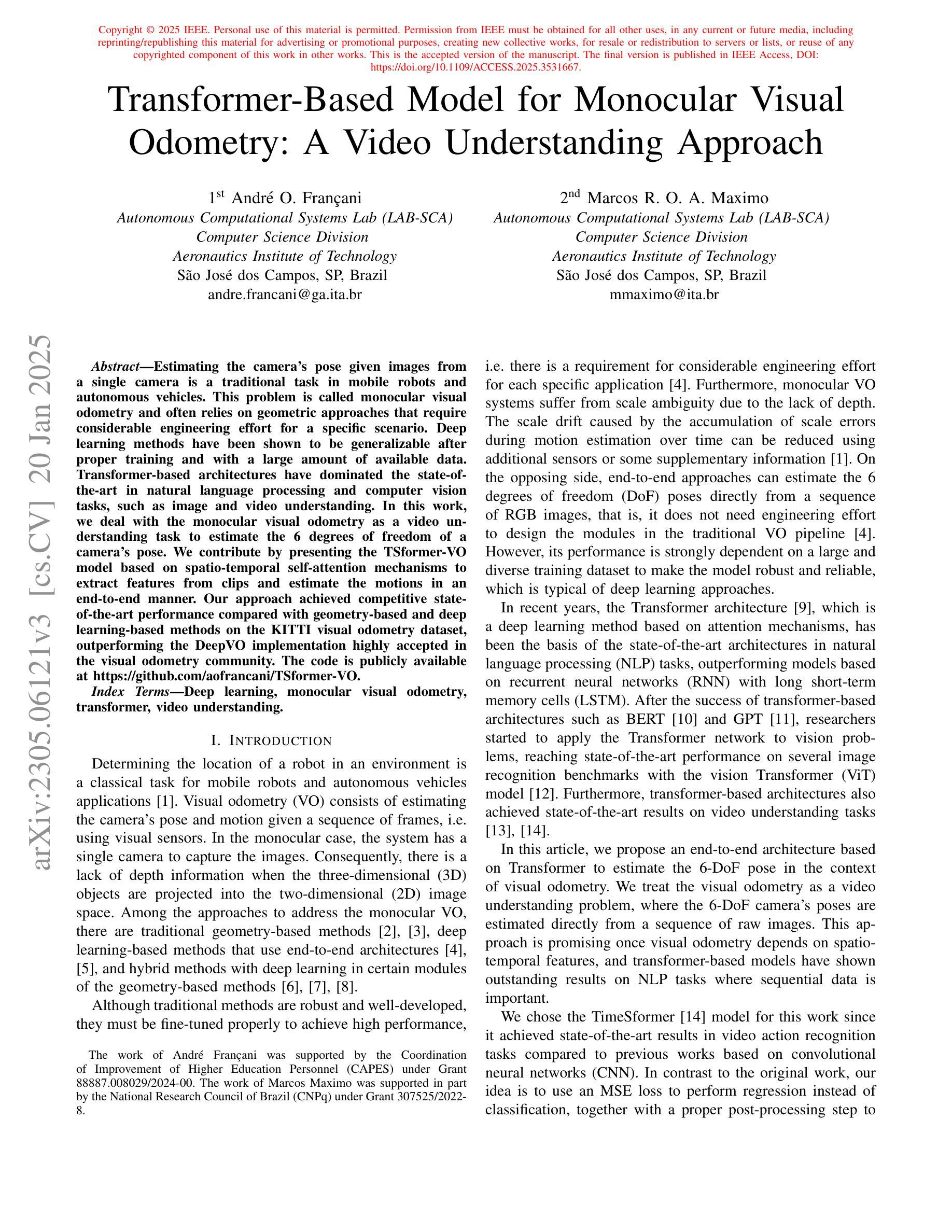
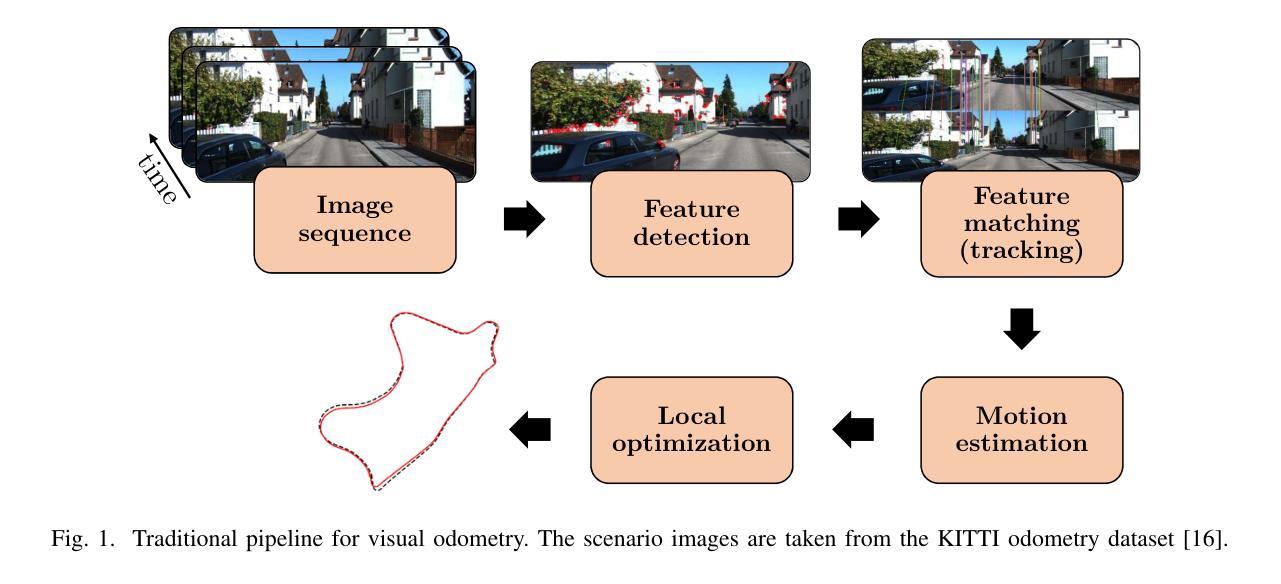
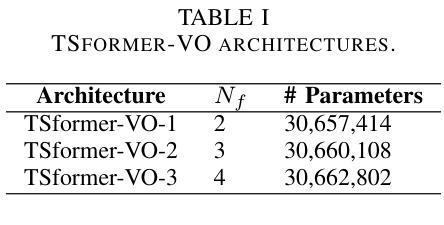

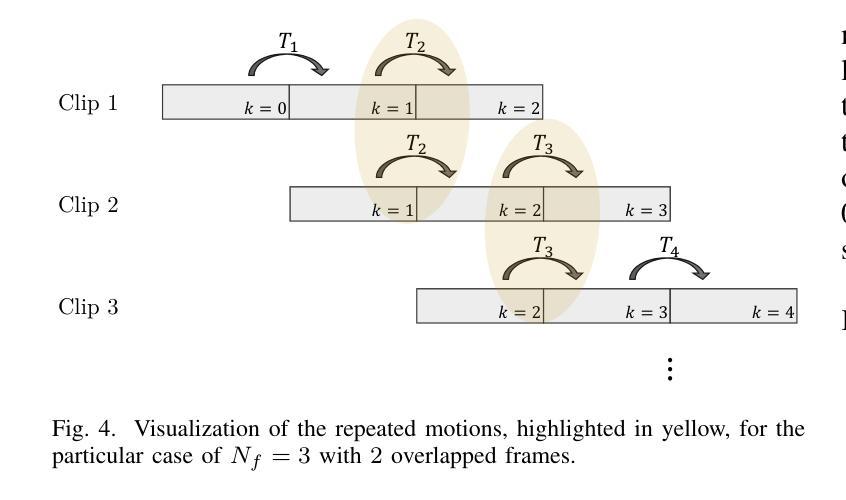