⚠️ 以下所有内容总结都来自于 大语言模型的能力,如有错误,仅供参考,谨慎使用
🔴 请注意:千万不要用于严肃的学术场景,只能用于论文阅读前的初筛!
💗 如果您觉得我们的项目对您有帮助 ChatPaperFree ,还请您给我们一些鼓励!⭐️ HuggingFace免费体验
2025-01-23 更新
EfficientVITON: An Efficient Virtual Try-On Model using Optimized Diffusion Process
Authors:Mostafa Atef, Mariam Ayman, Ahmed Rashed, Ashrakat Saeed, Abdelrahman Saeed, Ahmed Fares
Would not it be much more convenient for everybody to try on clothes by only looking into a mirror ? The answer to that problem is virtual try-on, enabling users to digitally experiment with outfits. The core challenge lies in realistic image-to-image translation, where clothing must fit diverse human forms, poses, and figures. Early methods, which used 2D transformations, offered speed, but image quality was often disappointing and lacked the nuance of deep learning. Though GAN-based techniques enhanced realism, their dependence on paired data proved limiting. More adaptable methods offered great visuals but demanded significant computing power and time. Recent advances in diffusion models have shown promise for high-fidelity translation, yet the current crop of virtual try-on tools still struggle with detail loss and warping issues. To tackle these challenges, this paper proposes EfficientVITON, a new virtual try-on system leveraging the impressive pre-trained Stable Diffusion model for better images and deployment feasibility. The system includes a spatial encoder to maintain clothings finer details and zero cross-attention blocks to capture the subtleties of how clothes fit a human body. Input images are carefully prepared, and the diffusion process has been tweaked to significantly cut generation time without image quality loss. The training process involves two distinct stages of fine-tuning, carefully incorporating a balance of loss functions to ensure both accurate try-on results and high-quality visuals. Rigorous testing on the VITON-HD dataset, supplemented with real-world examples, has demonstrated that EfficientVITON achieves state-of-the-art results.
试穿衣物时,如果只需要照镜子,对每个人来说不是更方便吗?这个问题的答案是虚拟试穿,它让用户能够数字化地尝试不同的服装。核心挑战在于实现真实的图像到图像的转换,其中服装必须适应各种人类形态、姿势和体型。早期使用2D转换的方法虽然速度很快,但图像质量往往令人失望,缺乏深度学习的细微差别。虽然基于GAN的技术增强了现实感,但它们对配对数据的依赖证明了其局限性。更灵活的方法提供了很好的视觉效果,但需要大量的计算能力和时间。扩散模型的最新进展显示出高保真翻译的希望,但目前的虚拟试穿工具仍然面临细节损失和变形问题。为了应对这些挑战,本文提出了EfficientVITON,这是一种新的虚拟试穿系统,它利用令人印象深刻的预训练Stable Diffusion模型,以更好地实现图像和部署的可行性。该系统包括一个空间编码器,以保留衣物的细节和零交叉注意力块,以捕捉衣物如何贴合人体身的细微差别。输入图像经过精心准备,扩散过程经过了调整,以显著减少生成时间而不会损失图像质量。训练过程包括两个精细调整的独特阶段,精心平衡损失函数以确保准确的试穿结果和高质量的视觉效果。在VITON-HD数据集上进行严格测试,并结合现实世界案例,已证明EfficientVITON达到了最先进的成果。
论文及项目相关链接
PDF 7 pages
摘要
文本提出了一种新型的虚拟试衣系统EfficientVITON,它利用预训练的Stable Diffusion模型,旨在解决现有虚拟试衣技术在图像质量和计算效率方面的挑战。该系统包括空间编码器和零交叉注意力块,以提高衣物细节的保留和人体与衣物贴合度的捕捉。通过精心准备输入图像和调整扩散过程,实现了在不影响图像质量的情况下大幅缩短生成时间。训练过程包括两个精细调整阶段,通过平衡损失函数确保试衣结果的准确性和高质感的视觉体验。在VITON-HD数据集上的严格测试以及现实世界的实例表明,EfficientVITON取得了业界最佳成果。
关键见解
- 虚拟试衣技术的核心挑战在于实现真实的图像到图像转换,需要适应各种人类形态、姿势和体型。
- 早期方法主要依赖2D转换,虽然速度较快,但图像质量常常不尽人意。
- GAN技术增强了现实感,但依赖于配对数据,有一定的局限性。
- 扩散模型的最新进展显示出高保真翻译的希望,但当前虚拟试衣工具仍存在细节损失和变形问题。
- EfficientVITON系统利用预训练的Stable Diffusion模型,旨在提高图像质量和部署的可行性。
- EfficientVITON包括空间编码器和零交叉注意力块,以更好地捕捉衣物的细节和贴合度。
点此查看论文截图
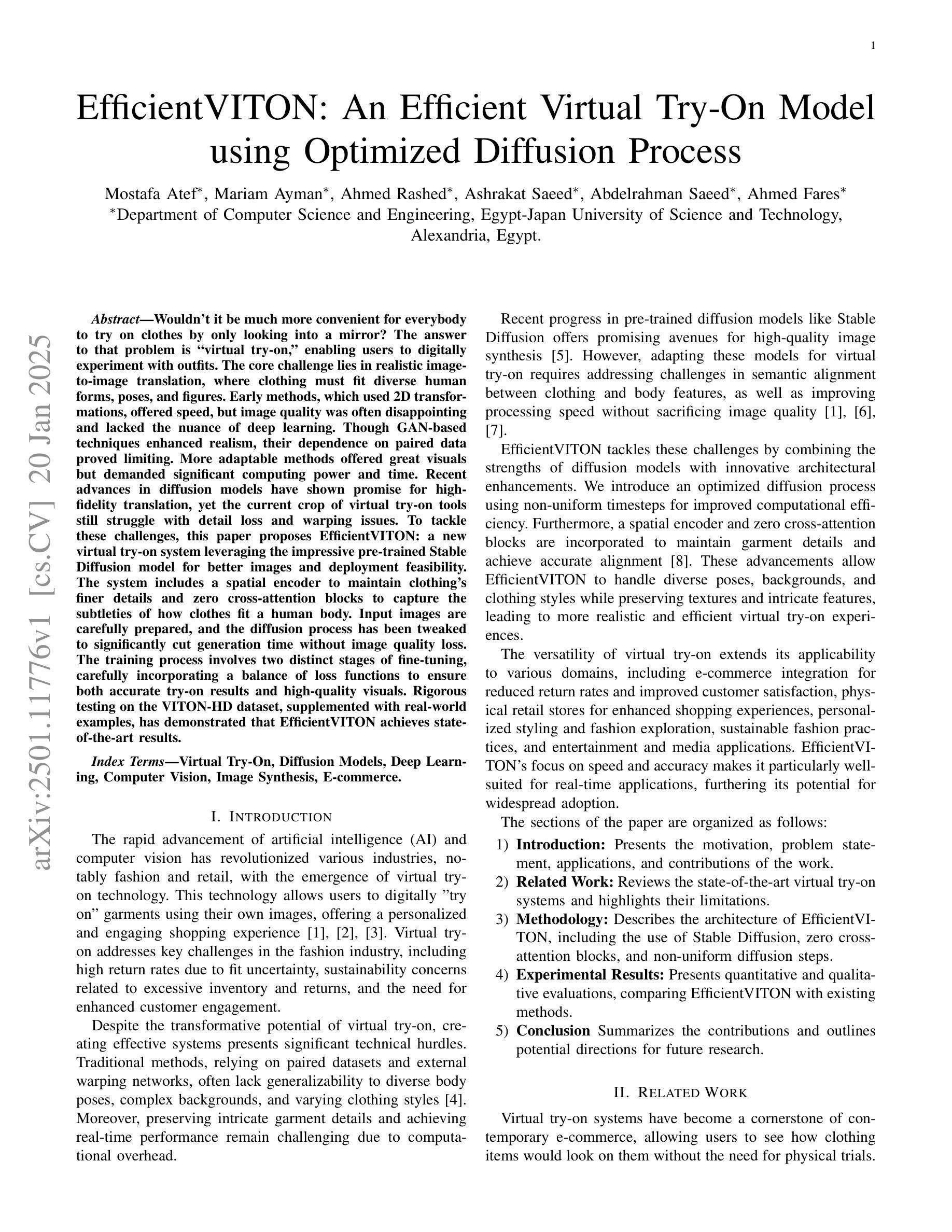
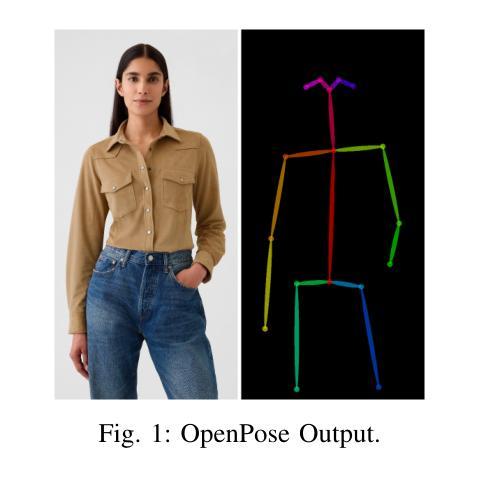
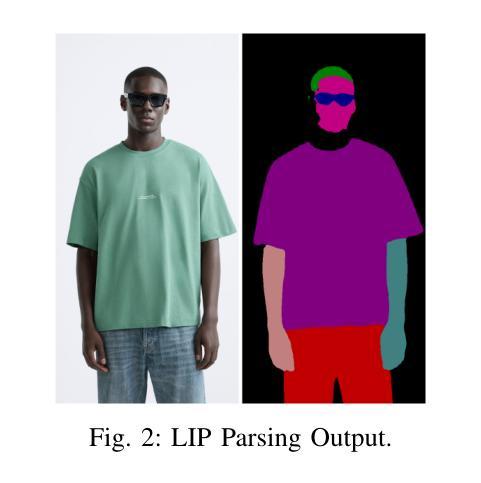
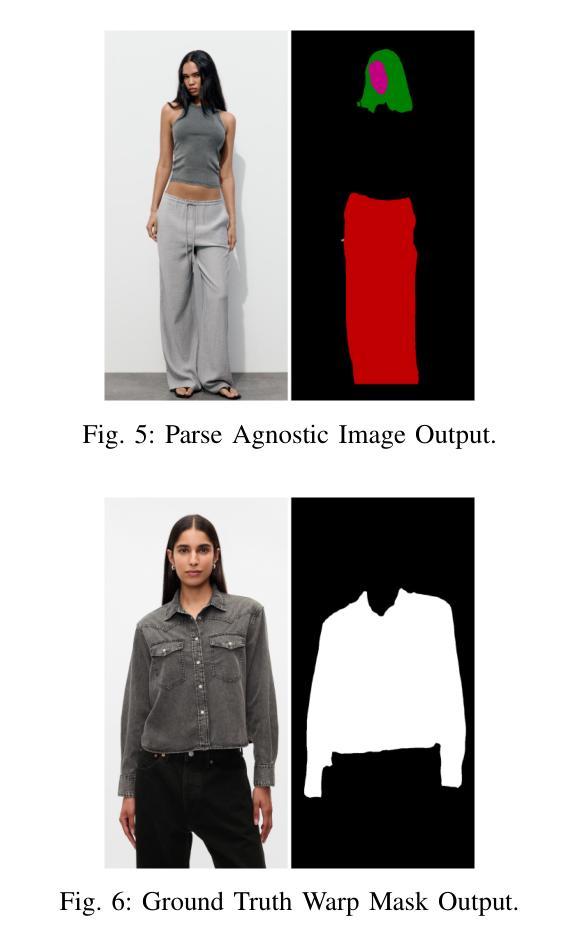
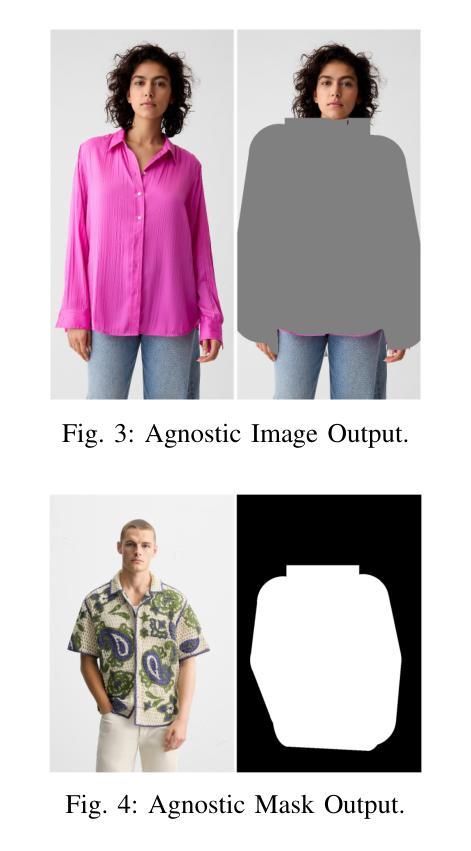
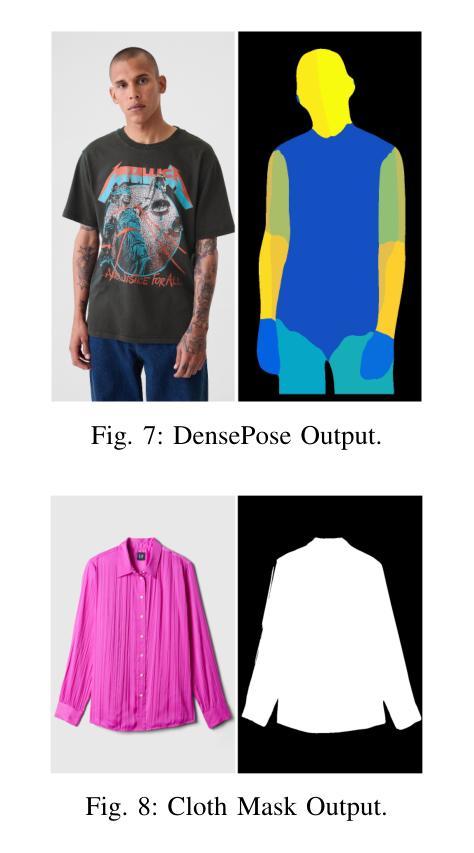
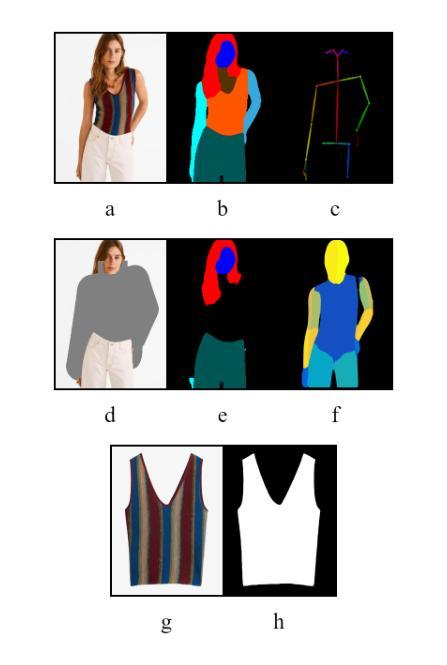
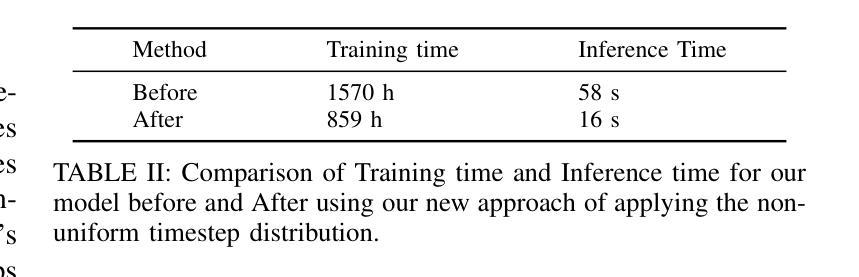
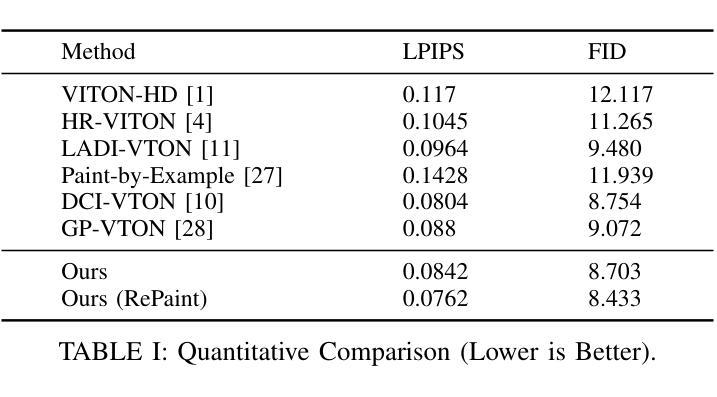
Nested Annealed Training Scheme for Generative Adversarial Networks
Authors:Chang Wan, Ming-Hsuan Yang, Minglu Li, Yunliang Jiang, Zhonglong Zheng
Recently, researchers have proposed many deep generative models, including generative adversarial networks(GANs) and denoising diffusion models. Although significant breakthroughs have been made and empirical success has been achieved with the GAN, its mathematical underpinnings remain relatively unknown. This paper focuses on a rigorous mathematical theoretical framework: the composite-functional-gradient GAN (CFG)[1]. Specifically, we reveal the theoretical connection between the CFG model and score-based models. We find that the training objective of the CFG discriminator is equivalent to finding an optimal D(x). The optimal gradient of D(x) differentiates the integral of the differences between the score functions of real and synthesized samples. Conversely, training the CFG generator involves finding an optimal G(x) that minimizes this difference. In this paper, we aim to derive an annealed weight preceding the weight of the CFG discriminator. This new explicit theoretical explanation model is called the annealed CFG method. To overcome the limitation of the annealed CFG method, as the method is not readily applicable to the SOTA GAN model, we propose a nested annealed training scheme (NATS). This scheme keeps the annealed weight from the CFG method and can be seamlessly adapted to various GAN models, no matter their structural, loss, or regularization differences. We conduct thorough experimental evaluations on various benchmark datasets for image generation. The results show that our annealed CFG and NATS methods significantly improve the quality and diversity of the synthesized samples. This improvement is clear when comparing the CFG method and the SOTA GAN models.
最近,研究人员提出了许多深度生成模型,包括生成对抗网络(GANs)和去噪扩散模型。尽管GAN取得了重大突破和实证成功,但其数学基础仍然相对未知。本文重点关注严格的数学理论框架:复合功能梯度GAN(CFG)[1]。具体来说,我们揭示了CFG模型与基于分数的模型之间的理论联系。我们发现CFG判别器的训练目标是找到最优的D(x)。D(x)的最优梯度区分了真实样本和合成样本分数函数之间的差异积分。相反,训练CFG生成器是寻找一个最优的G(x),以最小化这种差异。在本文中,我们的目标是推导出退火权重,该权重位于CFG判别器权重之前。这种新的显式理论解释模型被称为退火CFG方法。为了克服退火CFG方法的局限性(该方法不适用于SOTA GAN模型),我们提出了一种嵌套退火训练方案(NATS)。该方案保留了CFG方法的退火权重,并能无缝适应各种GAN模型,无论其结构、损失或正则化差异如何。我们在图像生成的各种基准数据集上进行了全面的实验评估。结果表明,我们的退火CFG和NATS方法显著提高了合成样本的质量和多样性。在与SOTA GAN模型比较时,这种改进更为明显。
论文及项目相关链接
Summary
本文介绍了复合功能梯度生成对抗网络(CFG)的理论框架,并揭示了其与基于分数的模型之间的理论联系。研究团队提出了退火权重前置的CFG鉴别器,并构建了退火CFG方法。为克服其局限性,提出了一种嵌套退火训练方案(NATS),可无缝适应各种GAN模型。实验评估表明,退火CFG和NATS方法能显著提高合成样本的质量和多样性。
Key Takeaways
- 介绍了复合功能梯度生成对抗网络(CFG)的理论框架。
- 揭示了CFG模型与基于分数的模型之间的理论联系。
- 提出了退火权重前置的CFG鉴别器。
- 构建了一种新的理论解释模型——退火CFG方法。
- 为克服退火CFG方法的局限性,提出了一种嵌套退火训练方案(NATS)。
- NATS方案可无缝适应各种GAN模型,无论其结构、损失或正则化差异如何。
点此查看论文截图
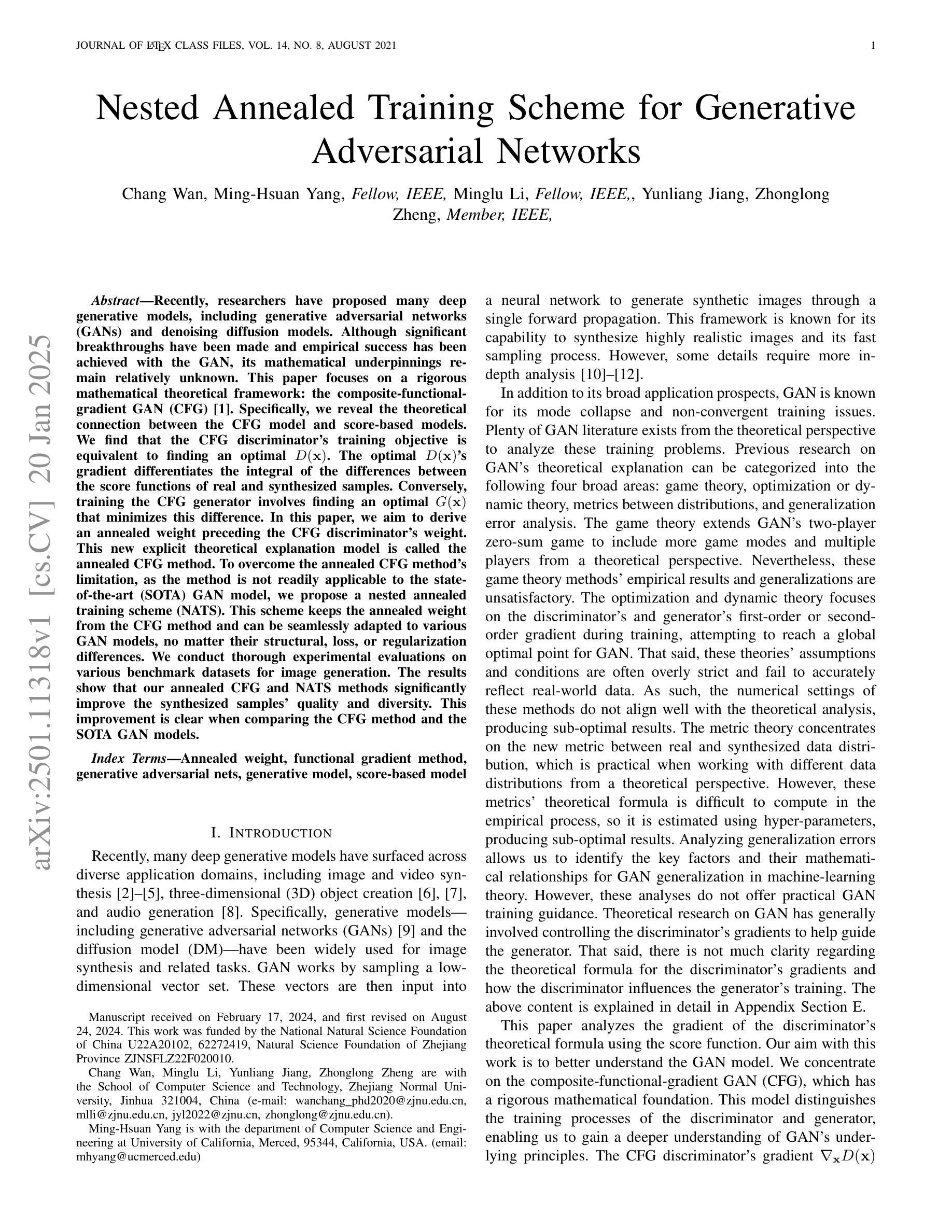
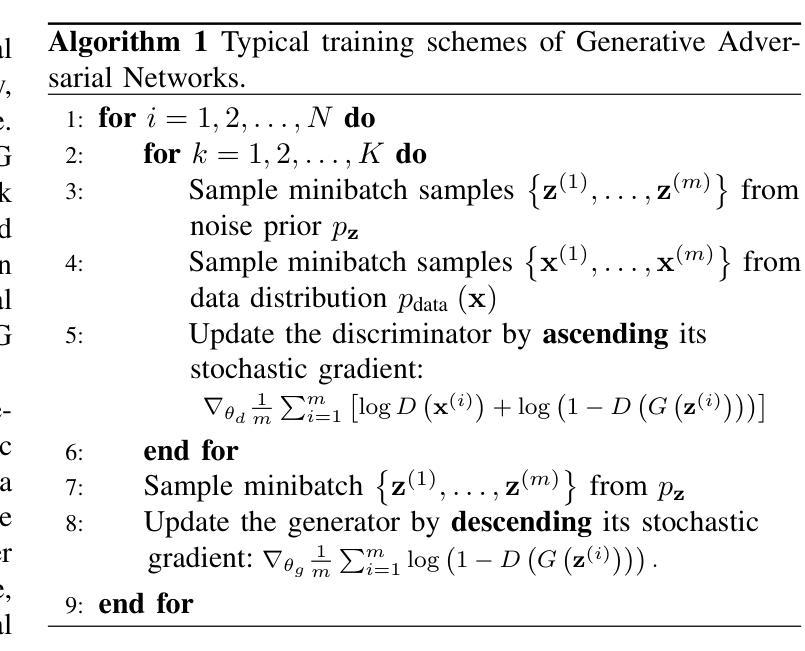
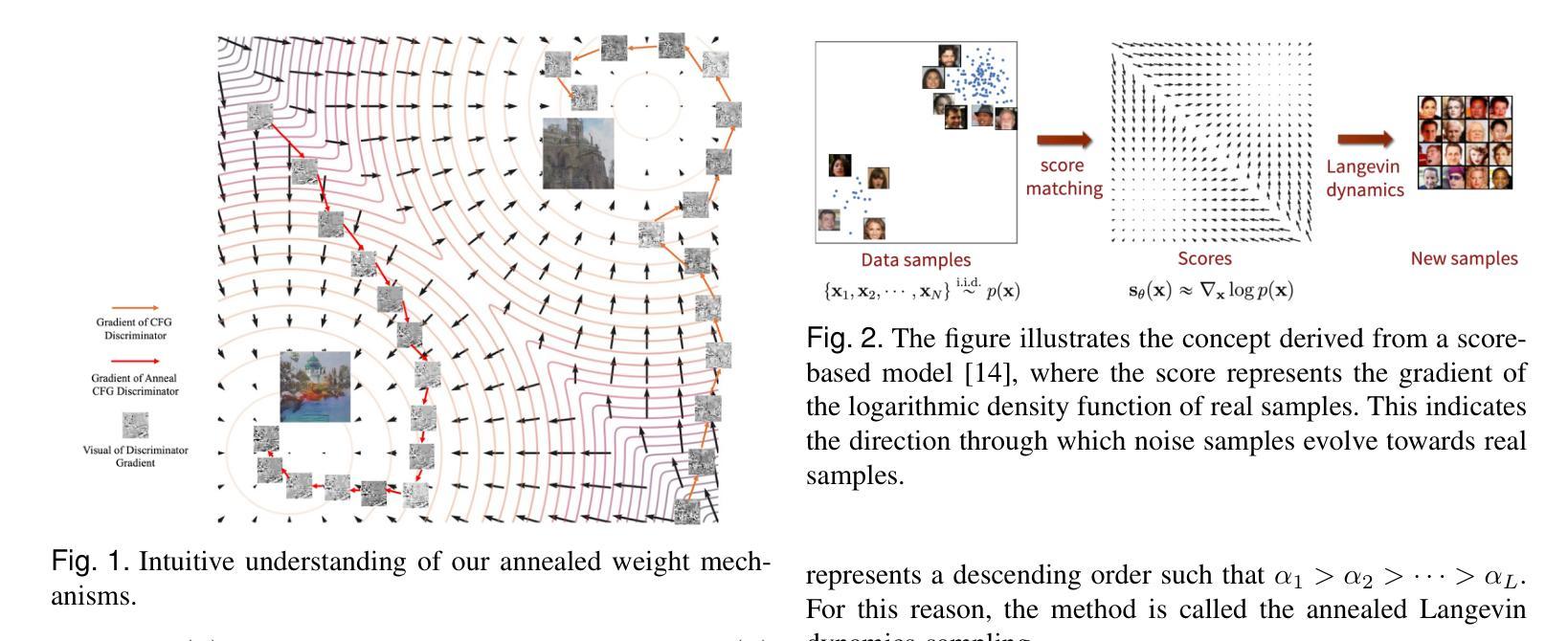
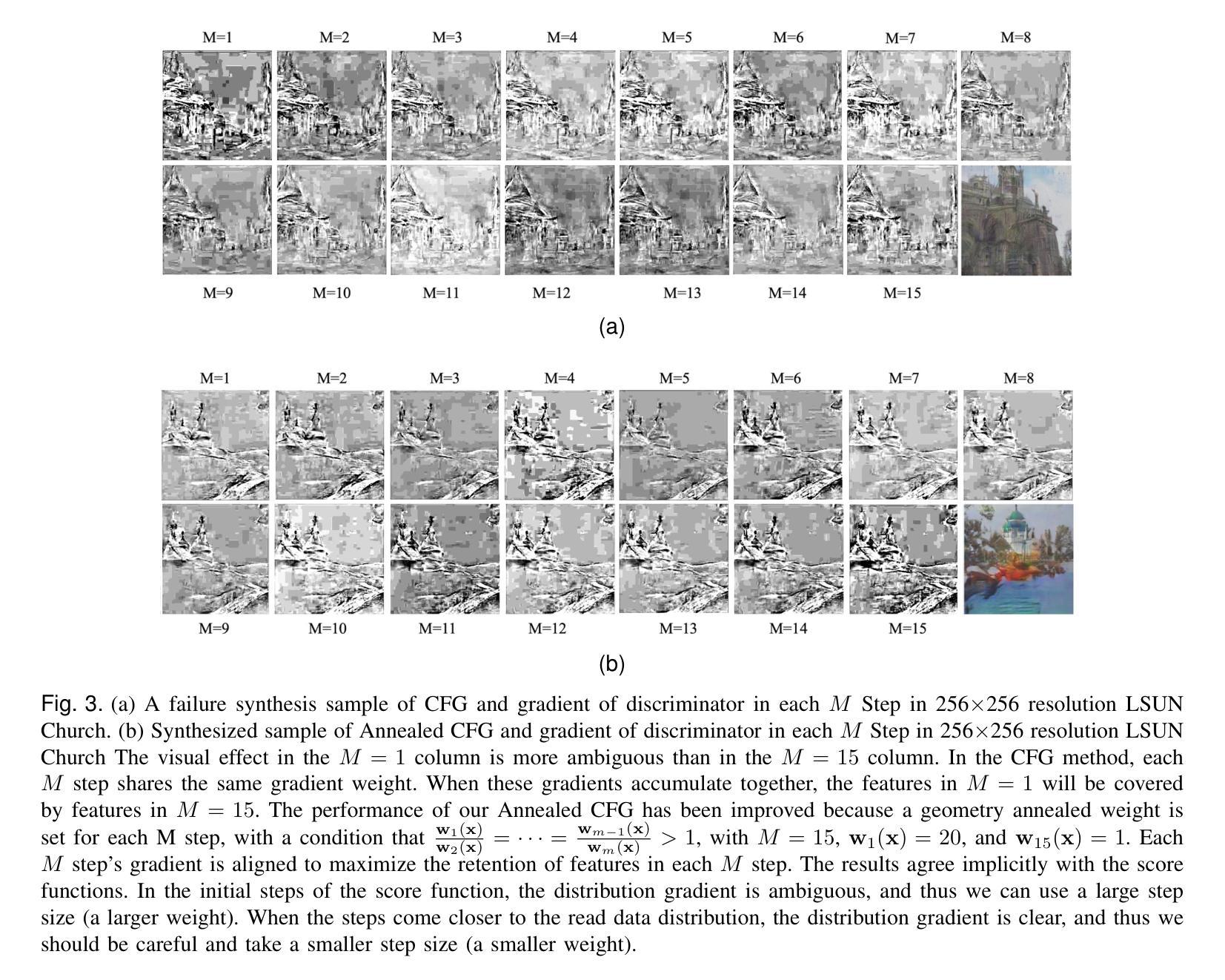
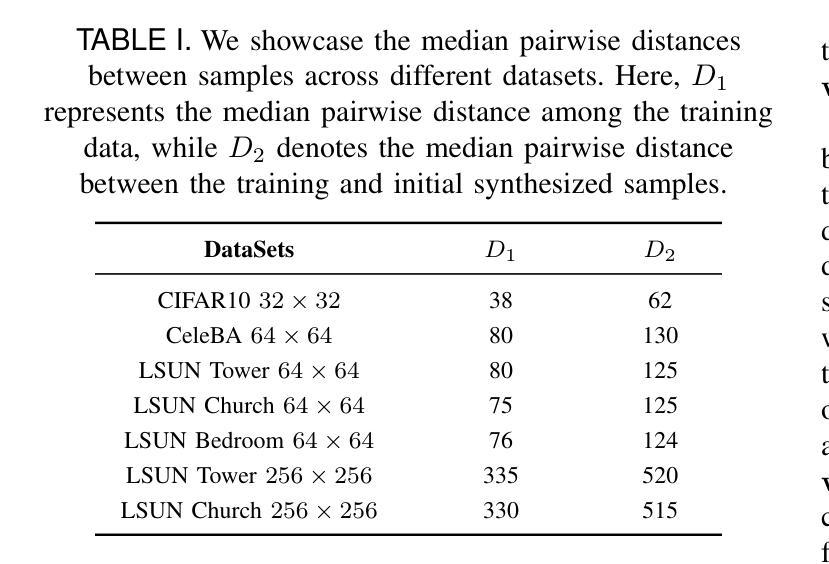
Leveraging GANs For Active Appearance Models Optimized Model Fitting
Authors:Anurag Awasthi
Generative Adversarial Networks (GANs) have gained prominence in refining model fitting tasks in computer vision, particularly in domains involving deformable models like Active Appearance Models (AAMs). This paper explores the integration of GANs to enhance the AAM fitting process, addressing challenges in optimizing nonlinear parameters associated with appearance and shape variations. By leveraging GANs’ adversarial training framework, the aim is to minimize fitting errors and improve convergence rates. Achieving robust performance even in cases with high appearance variability and occlusions. Our approach demonstrates significant improvements in accuracy and computational efficiency compared to traditional optimization techniques, thus establishing GANs as a potent tool for advanced image model fitting.
生成对抗网络(GANs)在计算机视觉的模型拟合任务中,特别是在涉及可变形模型(如主动外观模型(AAMs))的领域,已经获得了显著的地位。本文探讨了GANs与AAM拟合过程的集成,解决了优化与外观和形状变化相关的非线性参数方面的挑战。通过利用GANs的对抗训练框架,旨在减小拟合误差并提高收敛速率。即使在外观变化大和有遮挡的情况下也能实现稳健的性能。我们的方法与传统优化技术相比,在准确性和计算效率方面表现出显著改进,从而确立了GANs作为高级图像模型拟合的强大工具的地位。
论文及项目相关链接
PDF 9 pages, 2 figures, in proceeding at conference
Summary
本文探讨了将生成对抗网络(GANs)应用于增强Active Appearance Models(AAMs)拟合过程的研究。该研究利用GANs的对抗训练框架,针对外观和形状变化的非线性参数优化挑战,旨在减少拟合误差并提高收敛速度,在高外观变化和遮挡的情况下实现稳健性能。与传统优化技术相比,该方法在精度和计算效率方面显示出显著改进,从而证明了GANs在高级图像模型拟合中的潜力。
Key Takeaways
- GANs在模型拟合任务中的应用:本文主要探讨了生成对抗网络(GANs)在模型拟合任务中的应用,特别是在涉及可变模型(如Active Appearance Models)的计算机视觉领域。
- GANs增强AAM拟合过程:通过利用GANs的对抗训练框架,研究旨在增强Active Appearance Models(AAMs)的拟合过程。
- 优化非线性参数:针对外观和形状变化的非线性参数优化挑战,该研究提出解决方案。
- 减少拟合误差和提高收敛速度:利用GANs的对抗训练,研究旨在最小化拟合误差并提高收敛速度。
- 稳健性能的实现:在高外观可变性和遮挡的情况下,研究证明了该方法的稳健性能。
- 与传统技术的对比:与传统优化技术相比,该方法在精度和计算效率方面表现出显著改进。
点此查看论文截图
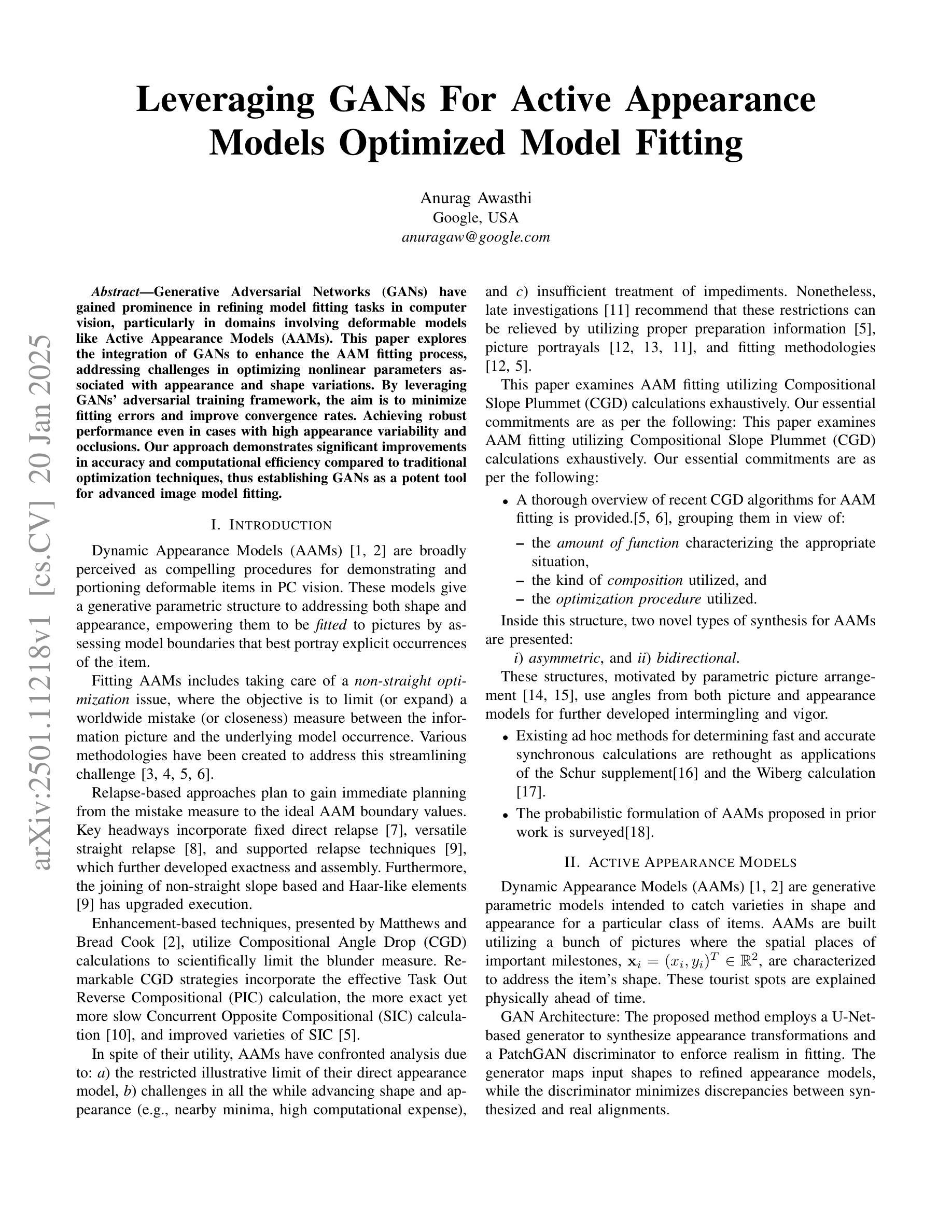
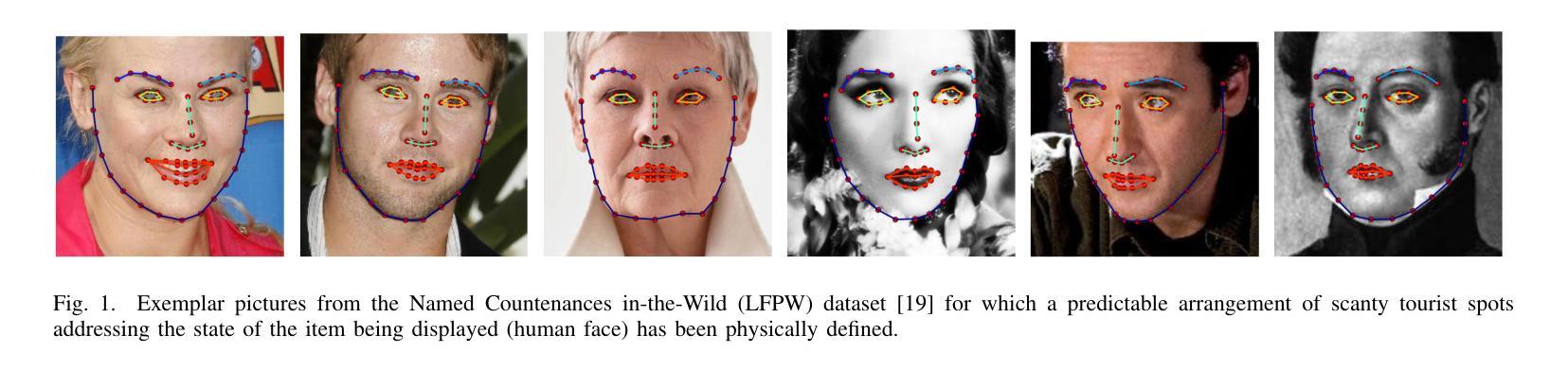
Multi-Scale Texture Loss for CT denoising with GANs
Authors:Francesco Di Feola, Lorenzo Tronchin, Valerio Guarrasi, Paolo Soda
Generative Adversarial Networks (GANs) have proved as a powerful framework for denoising applications in medical imaging. However, GAN-based denoising algorithms still suffer from limitations in capturing complex relationships within the images. In this regard, the loss function plays a crucial role in guiding the image generation process, encompassing how much a synthetic image differs from a real image. To grasp highly complex and non-linear textural relationships in the training process, this work presents a novel approach to capture and embed multi-scale texture information into the loss function. Our method introduces a differentiable multi-scale texture representation of the images dynamically aggregated by a self-attention layer, thus exploiting end-to-end gradient-based optimization. We validate our approach by carrying out extensive experiments in the context of low-dose CT denoising, a challenging application that aims to enhance the quality of noisy CT scans. We utilize three publicly available datasets, including one simulated and two real datasets. The results are promising as compared to other well-established loss functions, being also consistent across three different GAN architectures. The code is available at: https://github.com/TrainLaboratory/MultiScaleTextureLoss-MSTLF
生成对抗网络(GANs)已证明在医学成像的去噪应用中是强大的框架。然而,基于GAN的去噪算法在捕捉图像内部复杂关系方面仍存在局限性。在这方面,损失函数在指导图像生成过程中起着至关重要的作用,包括合成图像与真实图像之间的差异程度。为了把握训练过程中的高度复杂和非线性纹理关系,这项工作提出了一种将多尺度纹理信息嵌入损失函数的新方法。我们的方法引入了一种可微分的多尺度纹理表示,通过自注意力层动态聚合图像,从而利用端到端的基于梯度的优化。我们通过低剂量计算机断层扫描去噪的情境进行了广泛的实验来验证我们的方法,这是一个旨在提高噪声CT扫描质量的具有挑战性的应用。我们使用了三个公开数据集,包括一个模拟数据集和两个真实数据集。与其他已建立损失函数相比,结果令人鼓舞,并且在三种不同GAN架构之间也是一致的。代码可在:https://github.com/TrainLaboratory/MultiScaleTextureLoss-MSTLF获取。
论文及项目相关链接
Summary
本文介绍了生成对抗网络(GANs)在医学成像去噪应用中的强大框架。针对GAN在去噪算法中捕捉图像复杂关系方面的局限性,提出了一种新的损失函数方法,通过嵌入多尺度纹理信息来捕捉高度复杂和非线性的纹理关系。新方法通过自注意力层动态聚合可微多尺度纹理表示,利用端到端梯度优化。在低剂量CT去噪等具有挑战性的应用中进行了广泛实验验证,与其他常用损失函数相比,结果令人鼓舞,且在三种不同的GAN架构中表现一致。
Key Takeaways
- GANs在医学成像去噪中表现出强大的框架能力。
- GANs在去噪算法中捕捉图像复杂关系方面存在局限性。
- 损失函数在捕捉高度复杂和非线性纹理关系中起着关键作用。
- 提出的损失函数方法通过嵌入多尺度纹理信息改进了GAN的性能。
- 新方法通过自注意力层动态聚合可微多尺度纹理表示。
- 方法在挑战性应用(如低剂量CT去噪)中进行了广泛实验验证。
点此查看论文截图
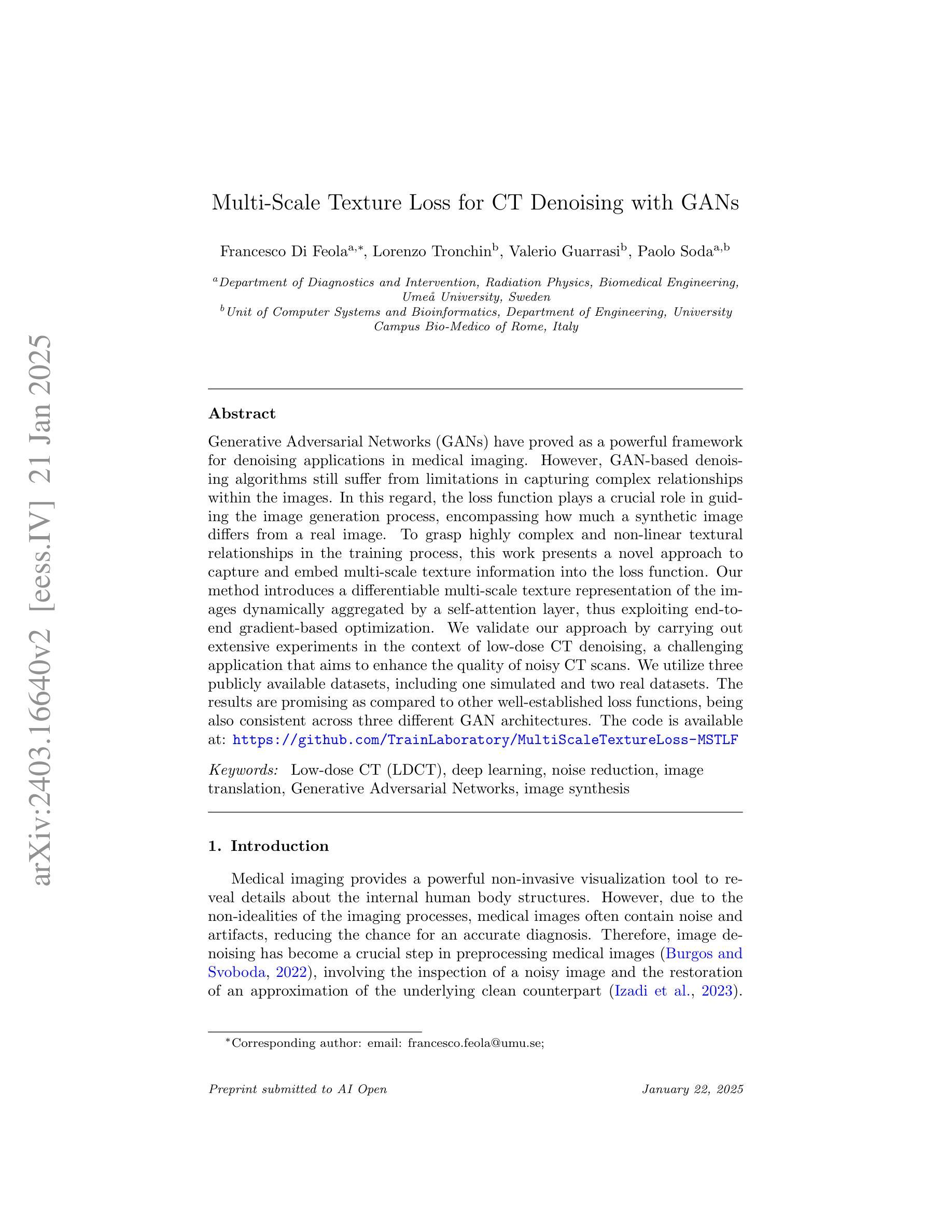
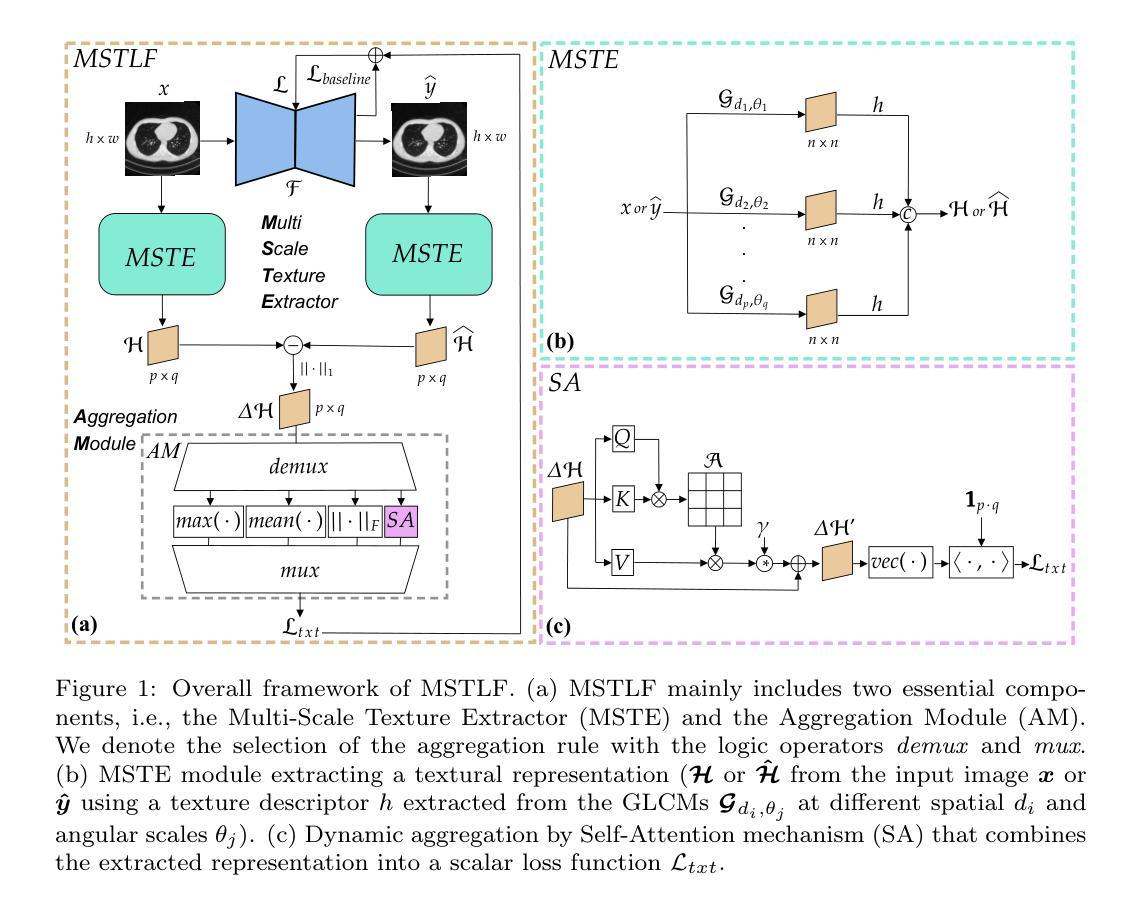