⚠️ 以下所有内容总结都来自于 大语言模型的能力,如有错误,仅供参考,谨慎使用
🔴 请注意:千万不要用于严肃的学术场景,只能用于论文阅读前的初筛!
💗 如果您觉得我们的项目对您有帮助 ChatPaperFree ,还请您给我们一些鼓励!⭐️ HuggingFace免费体验
2025-01-23 更新
EfficientVITON: An Efficient Virtual Try-On Model using Optimized Diffusion Process
Authors:Mostafa Atef, Mariam Ayman, Ahmed Rashed, Ashrakat Saeed, Abdelrahman Saeed, Ahmed Fares
Would not it be much more convenient for everybody to try on clothes by only looking into a mirror ? The answer to that problem is virtual try-on, enabling users to digitally experiment with outfits. The core challenge lies in realistic image-to-image translation, where clothing must fit diverse human forms, poses, and figures. Early methods, which used 2D transformations, offered speed, but image quality was often disappointing and lacked the nuance of deep learning. Though GAN-based techniques enhanced realism, their dependence on paired data proved limiting. More adaptable methods offered great visuals but demanded significant computing power and time. Recent advances in diffusion models have shown promise for high-fidelity translation, yet the current crop of virtual try-on tools still struggle with detail loss and warping issues. To tackle these challenges, this paper proposes EfficientVITON, a new virtual try-on system leveraging the impressive pre-trained Stable Diffusion model for better images and deployment feasibility. The system includes a spatial encoder to maintain clothings finer details and zero cross-attention blocks to capture the subtleties of how clothes fit a human body. Input images are carefully prepared, and the diffusion process has been tweaked to significantly cut generation time without image quality loss. The training process involves two distinct stages of fine-tuning, carefully incorporating a balance of loss functions to ensure both accurate try-on results and high-quality visuals. Rigorous testing on the VITON-HD dataset, supplemented with real-world examples, has demonstrated that EfficientVITON achieves state-of-the-art results.
如果每个人都只是通过照镜子来试穿衣服,那将更加方便不是吗?这个问题的答案是虚拟试衣,它让用户能够数字化地尝试各种服装。核心挑战在于真实的图像到图像的转换,其中服装必须适应各种人类形态、姿势和体型。早期使用2D转换的方法虽然速度很快,但图像质量往往令人失望,缺乏深度学习的细微差别。虽然基于GAN的技术增强了现实感,但它们对配对数据的依赖证明是有限的。适应性更强的方法在视觉上很棒,但需要大量的计算能力和时间。最近扩散模型方面的进展显示出高保真翻译的前景,但目前的虚拟试衣工具仍然面临细节丢失和变形问题。为了应对这些挑战,本文提出了EfficientVITON,一个新的虚拟试衣系统,利用令人印象深刻的预训练Stable Diffusion模型,以获取更好的图像和更高的部署可行性。该系统包括一个空间编码器,以保留服装的精细细节和零交叉注意力块,以捕捉服装如何贴合人体细微之处。输入图像经过精心准备,扩散过程经过调整,以显著减少生成时间而不损失图像质量。训练过程包括两个精细调整的独特阶段,精心平衡损失函数,以确保准确的试穿结果和高质量的视觉效果。在VITON-HD数据集上进行严格测试,并结合现实世界示例进行补充,证明了EfficientVITON达到了最先进的结果。
论文及项目相关链接
PDF 7 pages
Summary
基于文本内容,生成了摘要:“虚拟试衣技术旨在让用户数字化地尝试不同服装搭配。面临的挑战在于实现真实图像到图像的转换,以确保衣物能够贴合多样的人体形态和姿态。文章提出新的虚拟试衣系统EfficientVITON,采用预训练的Stable Diffusion模型以改善图像质量和部署可行性。通过空间编码器和零交叉注意力块等技术细节调整,以准确捕捉衣物与人体之间的微妙关系。系统测试表明,EfficientVITON达到了最新的技术成果。”
Key Takeaways
以下是七个关于给定文本的关键见解:
- 虚拟试衣技术允许用户数字化地尝试不同服装搭配,通过镜子进行试穿更加便捷。
- 实现真实图像到图像的转换是虚拟试衣技术的核心挑战,需要确保衣物贴合多样的人体形态和姿态。
- 早期方法主要使用2D转换,虽然速度较快但图像质量常常不尽人意,缺乏深度学习所带来的细微差别。
- 基于GAN的技术增强了现实感,但它们对配对数据的依赖证明其局限性。
- 最近扩散模型的发展显示出高保真翻译的希望,但当前的虚拟试穿工具仍然存在细节损失和变形问题。
- EfficientVITON是一个新的虚拟试穿系统,利用预训练的Stable Diffusion模型改善图像质量和部署可行性。
点此查看论文截图
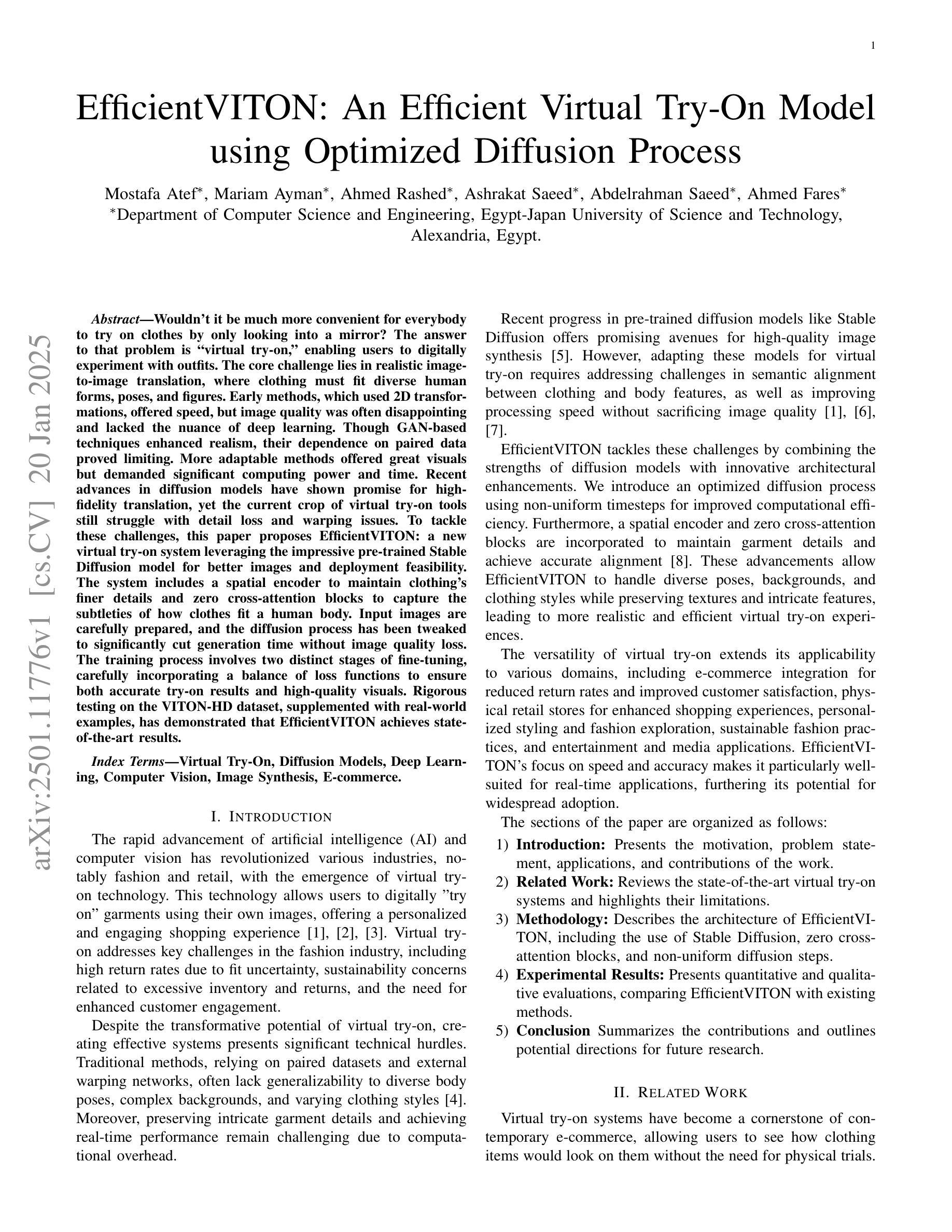
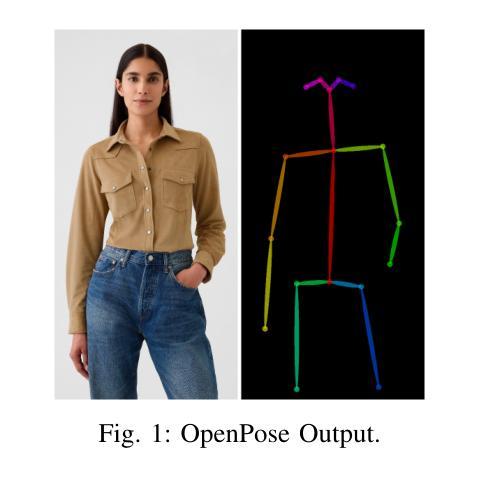
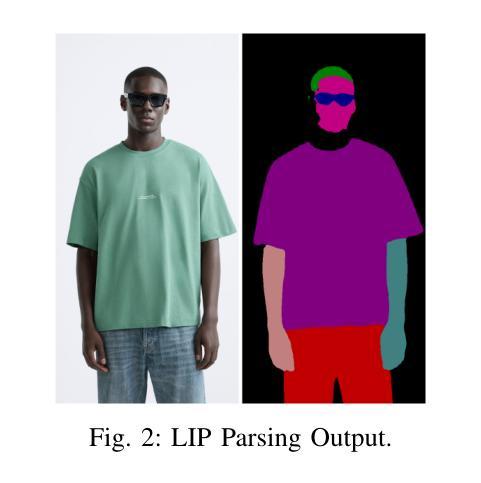
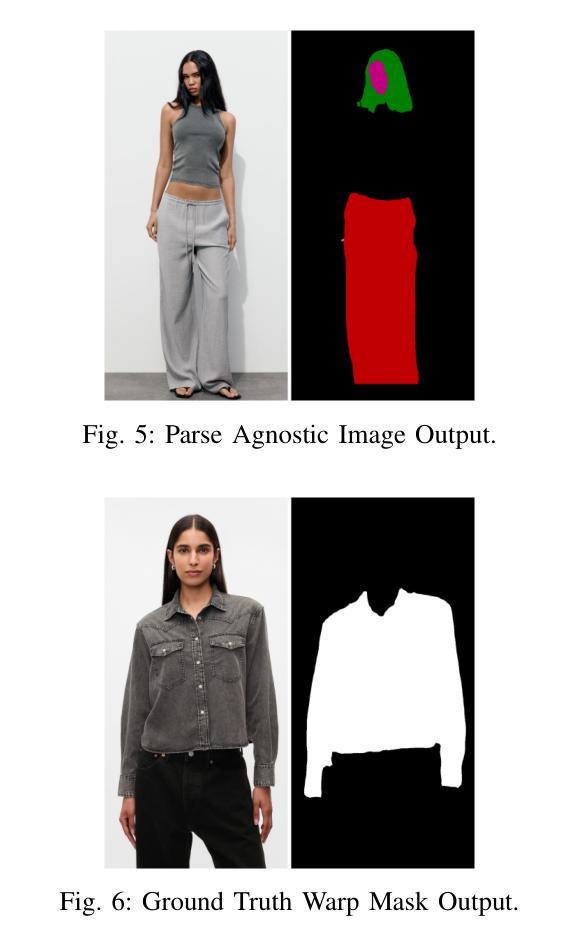
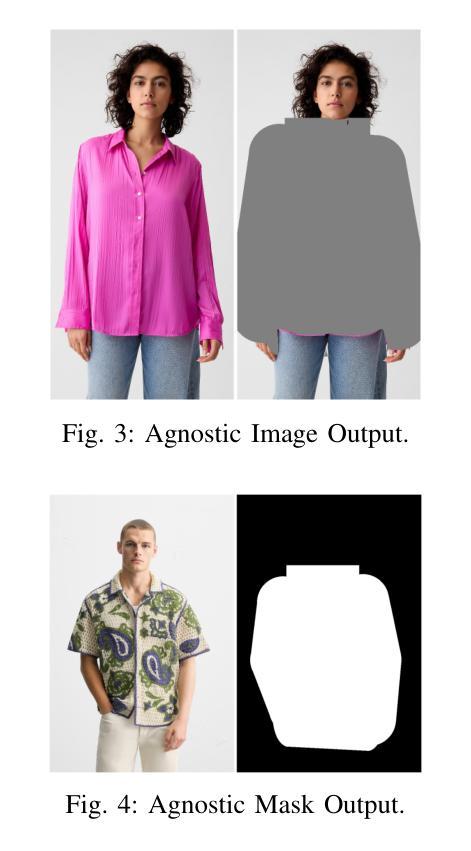
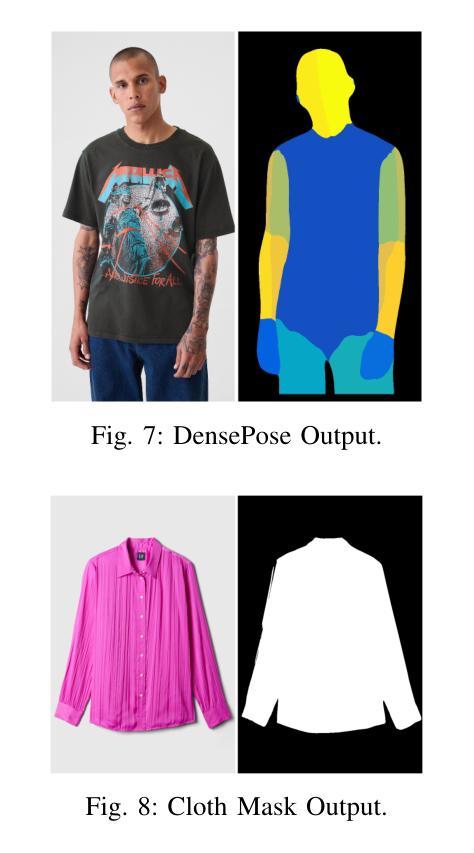
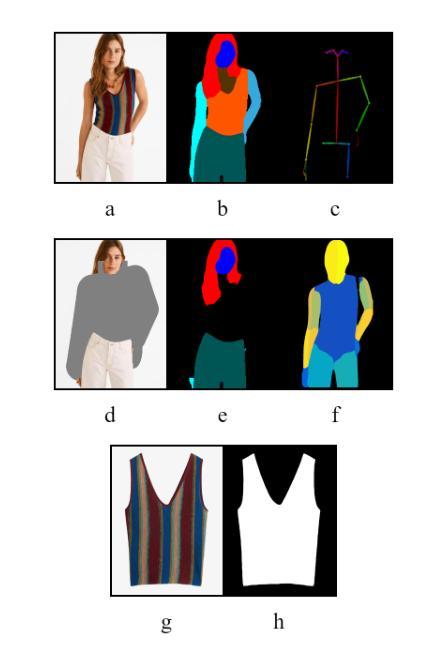
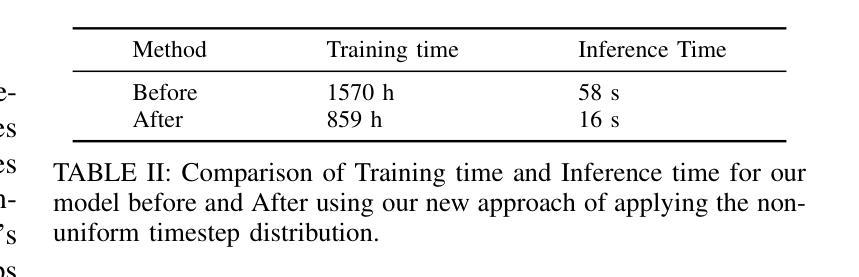
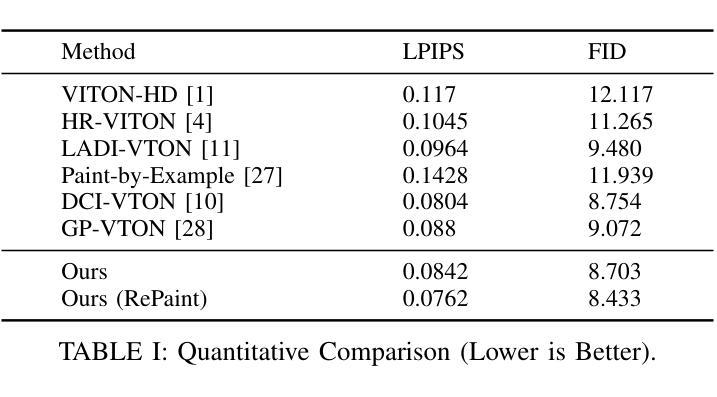
Untrained Perceptual Loss for image denoising of line-like structures in MR images
Authors:Elisabeth Pfaehler, Daniel Pflugfelder, Hanno Scharr
In the acquisition of Magnetic Resonance (MR) images shorter scan times lead to higher image noise. Therefore, automatic image denoising using deep learning methods is of high interest. MR images containing line-like structures such as roots or vessels yield special characteristics as they display connected structures and yield sparse information. For this kind of data, it is important to consider voxel neighborhoods when training a denoising network. In this paper, we translate the Perceptual Loss to 3D data by comparing feature maps of untrained networks in the loss function as done previously for 2D data. We tested the performance of untrained Perceptual Loss (uPL) on 3D image denoising of MR images displaying brain vessels (MR angiograms - MRA) and images of plant roots in soil. We investigate the impact of various uPL characteristics such as weight initialization, network depth, kernel size, and pooling operations on the results. We tested the performance of the uPL loss on four Rician noise levels using evaluation metrics such as the Structural Similarity Index Metric (SSIM). We observe, that our uPL outperforms conventional loss functions such as the L1 loss or a loss based on the Structural Similarity Index Metric (SSIM). The uPL network’s initialization is not important, while network depth and pooling operations impact denoising performance. E.g. for both datasets a network with five convolutional layers led to the best performance while a network with more layers led to a performance drop. We also find that small uPL networks led to better or comparable results than using large networks such as VGG. We observe superior performance of our loss for both datasets, all noise levels, and three network architectures. In conclusion, for images containing line-like structures, uPL is an alternative to other loss functions for 3D image denoising.
在获取磁共振(MR)图像时,较短的扫描时间会导致图像噪声较高。因此,使用深度学习方法进行自动图像去噪具有很高兴趣。MR图像中包含类似线条的结构,如根部或血管,表现出特殊特性,因为它们显示出连接的结构并产生稀疏信息。对于此类数据,在训练去噪网络时考虑体素邻域很重要。在本文中,我们通过将感知损失转化为三维数据,通过比较损失函数中的未训练网络特征图,如先前对二维数据所做的那样。我们在MR图像的三维图像去噪上测试了未训练的感知损失(uPL)的性能,这些图像显示脑血管(MR血管造影-MRA)和土壤中的植物根系图像。我们研究了各种uPL特性(如权重初始化,网络深度,内核大小和池操作)对结果的影响。我们在四个Rician噪声水平上测试了uPL损失的性能,使用结构相似性指数度量(SSIM)等评估指标。我们观察到,我们的uPL优于传统的损失函数,如L1损失或基于结构相似性指数度量(SSIM)的损失。uPL网络的初始化并不重要,而网络深度和池操作会影响去噪性能。例如,对于这两个数据集,具有五个卷积层的网络表现最佳,而具有更多层的网络则导致性能下降。我们还发现,使用小型uPL网络比使用大型网络(如VGG)获得更好或相当的结果。我们在所有数据集、所有噪声水平和三种网络架构中都观察到我们损失的优势性能。总之,对于包含线状结构的图像,uPL是适用于三维图像去噪的替代损失函数。
论文及项目相关链接
Summary
针对含有线状结构(如根部或血管)的MR图像,自动图像去噪是一个重要问题。本研究将感知损失(Perceptual Loss)应用到三维数据上,通过对未训练网络特征图的比较计算损失函数中的感知损失值。实验证明,该损失函数在去噪效果上优于传统的损失函数如L1损失或基于结构相似性指数度量的损失函数。网络深度与池化操作对去噪性能有影响,而网络初始化方式影响较小。此方法在包含线状结构的MR血管造影和植物根系图像上表现优异。
Key Takeaways
1. MR图像中扫描时间缩短会导致图像噪声增加,自动图像去噪方法尤为重要。
2. 对于含有线状结构的MR图像(如血管和植物根系),训练去噪网络时需考虑体素邻域信息。
3. 本研究成功将感知损失应用于三维数据,采用对比未训练网络特征图的方式计算损失。
4. 对比多种uPL特性(如权重初始化、网络深度、卷积核大小和池化操作)对结果的影响。
5. uPL损失函数在多种噪声水平下的性能优于传统损失函数,如L1损失和基于结构相似性指数度量的损失。
6. 网络深度与池化操作对去噪性能有影响,而网络初始化方式影响较小。
点此查看论文截图
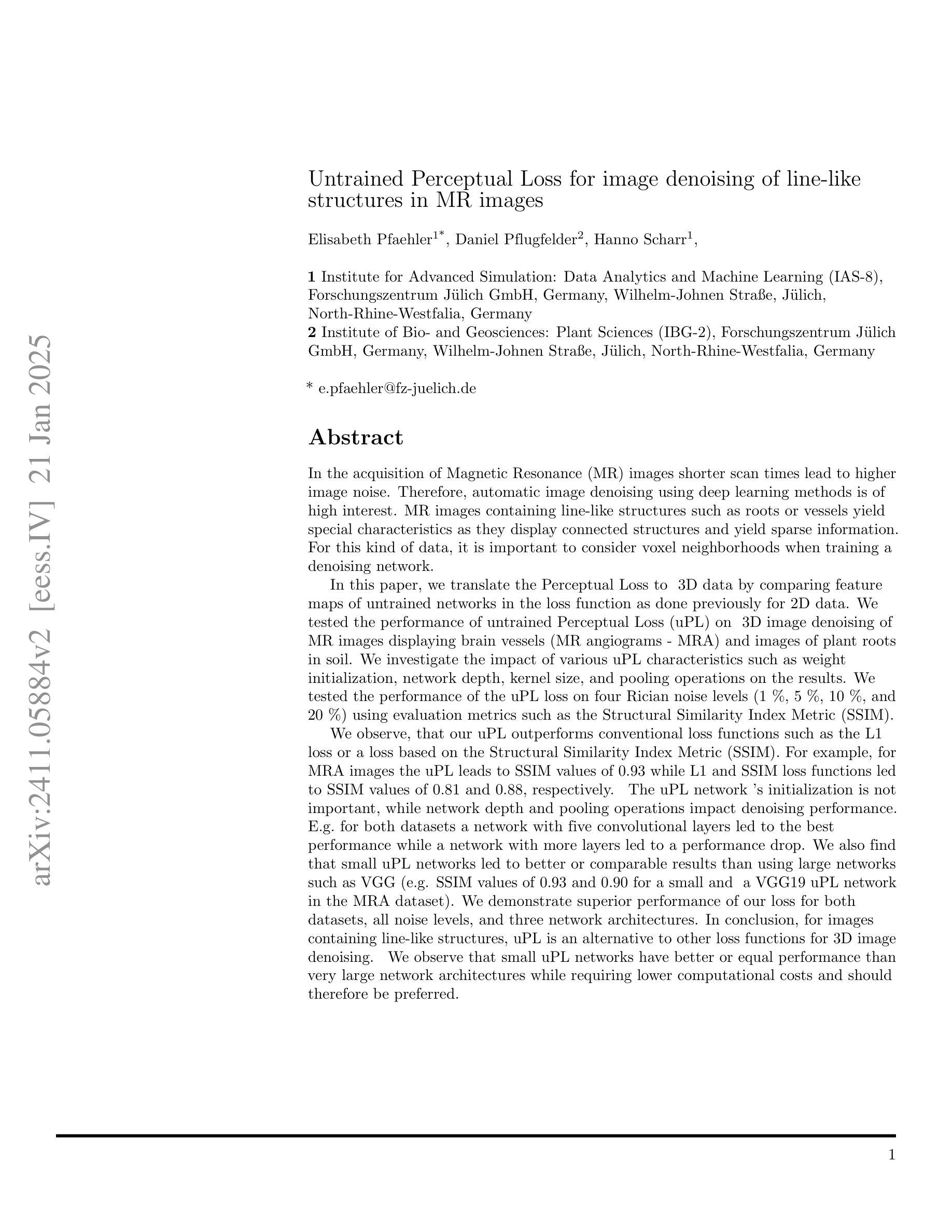
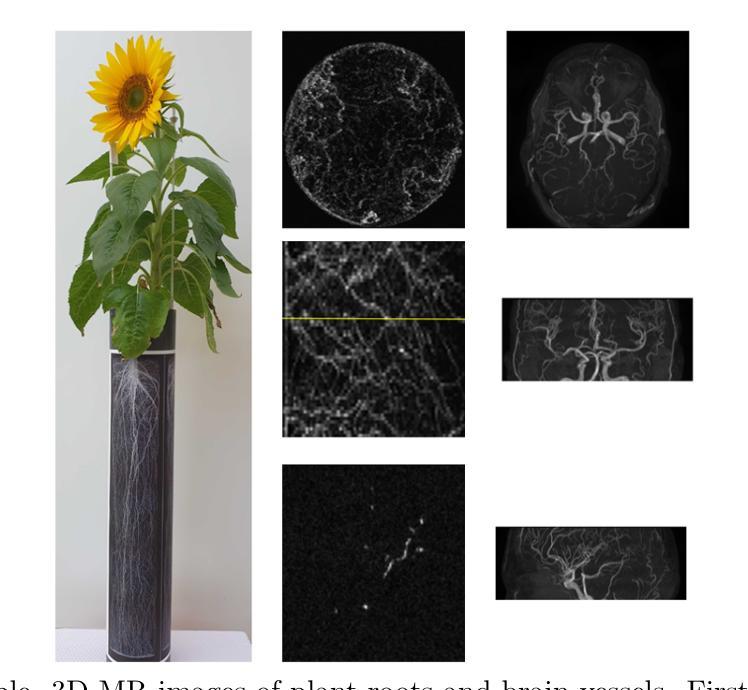