⚠️ 以下所有内容总结都来自于 大语言模型的能力,如有错误,仅供参考,谨慎使用
🔴 请注意:千万不要用于严肃的学术场景,只能用于论文阅读前的初筛!
💗 如果您觉得我们的项目对您有帮助 ChatPaperFree ,还请您给我们一些鼓励!⭐️ HuggingFace免费体验
2025-01-24 更新
Condition-Invariant Semantic Segmentation
Authors:Christos Sakaridis, David Bruggemann, Fisher Yu, Luc Van Gool
Adaptation of semantic segmentation networks to different visual conditions is vital for robust perception in autonomous cars and robots. However, previous work has shown that most feature-level adaptation methods, which employ adversarial training and are validated on synthetic-to-real adaptation, provide marginal gains in condition-level adaptation, being outperformed by simple pixel-level adaptation via stylization. Motivated by these findings, we propose to leverage stylization in performing feature-level adaptation by aligning the internal network features extracted by the encoder of the network from the original and the stylized view of each input image with a novel feature invariance loss. In this way, we encourage the encoder to extract features that are already invariant to the style of the input, allowing the decoder to focus on parsing these features and not on further abstracting from the specific style of the input. We implement our method, named Condition-Invariant Semantic Segmentation (CISS), on the current state-of-the-art domain adaptation architecture and achieve outstanding results on condition-level adaptation. In particular, CISS sets the new state of the art in the popular daytime-to-nighttime Cityscapes$\to$Dark Zurich benchmark. Furthermore, our method achieves the second-best performance on the normal-to-adverse Cityscapes$\to$ACDC benchmark. CISS is shown to generalize well to domains unseen during training, such as BDD100K-night and ACDC-night. Code is publicly available at https://github.com/SysCV/CISS .
对于自动驾驶汽车和机器人中的稳健感知,将语义分割网络适应不同的视觉条件至关重要。然而,先前的工作表明,大多数采用对抗训练并在合成到现实适应中进行验证的特征级适应方法,在条件级适应上的收益甚微,被通过风格化实现的简单像素级适应所超越。受到这些发现的启发,我们提出利用风格化进行特征级适应,通过对网络中编码器提取的原始输入和每个输入图像风格化视图的内部网络特征进行对齐,采用新型的特征不变性损失。通过这种方式,我们鼓励编码器提取对输入风格已经不变的特性,让解码器专注于解析这些特性,而不是进一步从输入的特定风格中进行抽象。我们实现了名为条件不变语义分割(CISS)的方法,并应用于当前最先进的域适应架构上,在条件级适应上取得了卓越的结果。特别是,CISS在流行的白天到夜晚Cityscapes到Dark Zurich基准测试中树立了新的研究前沿。此外,我们的方法在正常的Cityscapes到ACDC基准测试中取得了第二好的性能。CISS被证明能够很好地泛化到训练期间未见过的领域,如BDD100K-night和ACDC-night。代码可在https://github.com/SysCV/CISS上公开获取。
论文及项目相关链接
PDF IEEE T-PAMI 2025
Summary
本文研究了语义分割网络在不同视觉条件下的适应性,对自主车辆和机器人的稳健感知至关重要。针对现有特征级别适应方法的局限性,提出了一种利用风格化进行特征级别适应的新方法,通过内部网络特征对齐,使编码器从原始和风格化视角提取的特征一致。实施名为条件不变语义分割(CISS)的方法,在条件级别适应上取得出色成果,特别是在日间到夜间的Cityscapes到Dark Zurich基准测试中表现突出。此外,该方法在正常的Cityscapes到ACDC基准测试中表现第二。CISS能够很好地泛化到训练时未见过的领域,如BDD100K-night和ACDC-night。
Key Takeaways
- 语义分割网络在不同视觉条件下的适应性对自主车辆和机器人至关重要。
- 特征级别适应方法面临局限性,需要新的策略来提升性能。
- 利用风格化进行特征级别适应是一种有效方法,通过对齐内部网络特征以提高适应性能。
- CISS方法在条件级别适应上表现突出,特别是在日间到夜间的基准测试中。
- CISS方法具有良好的泛化能力,适用于训练时未见过的领域。
点此查看论文截图
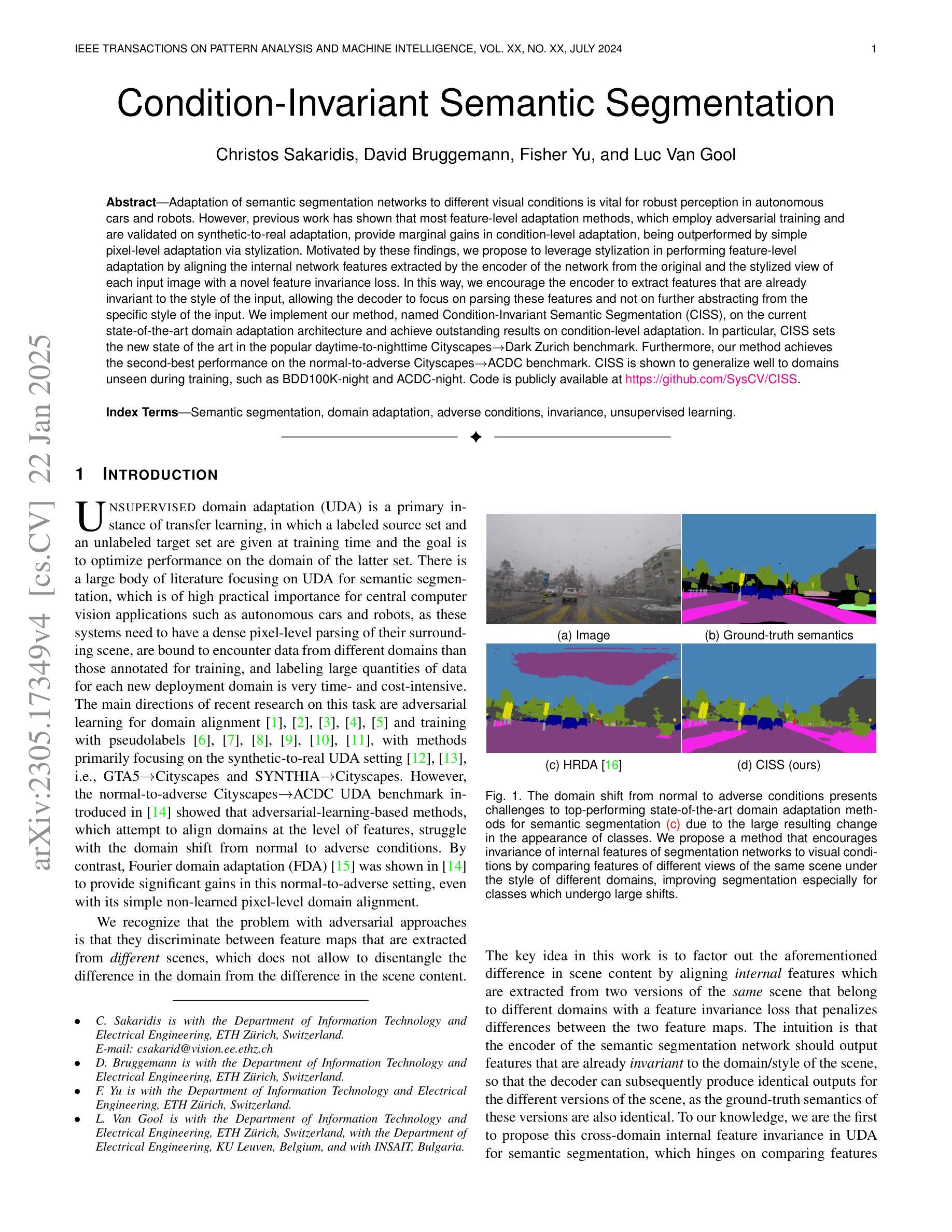
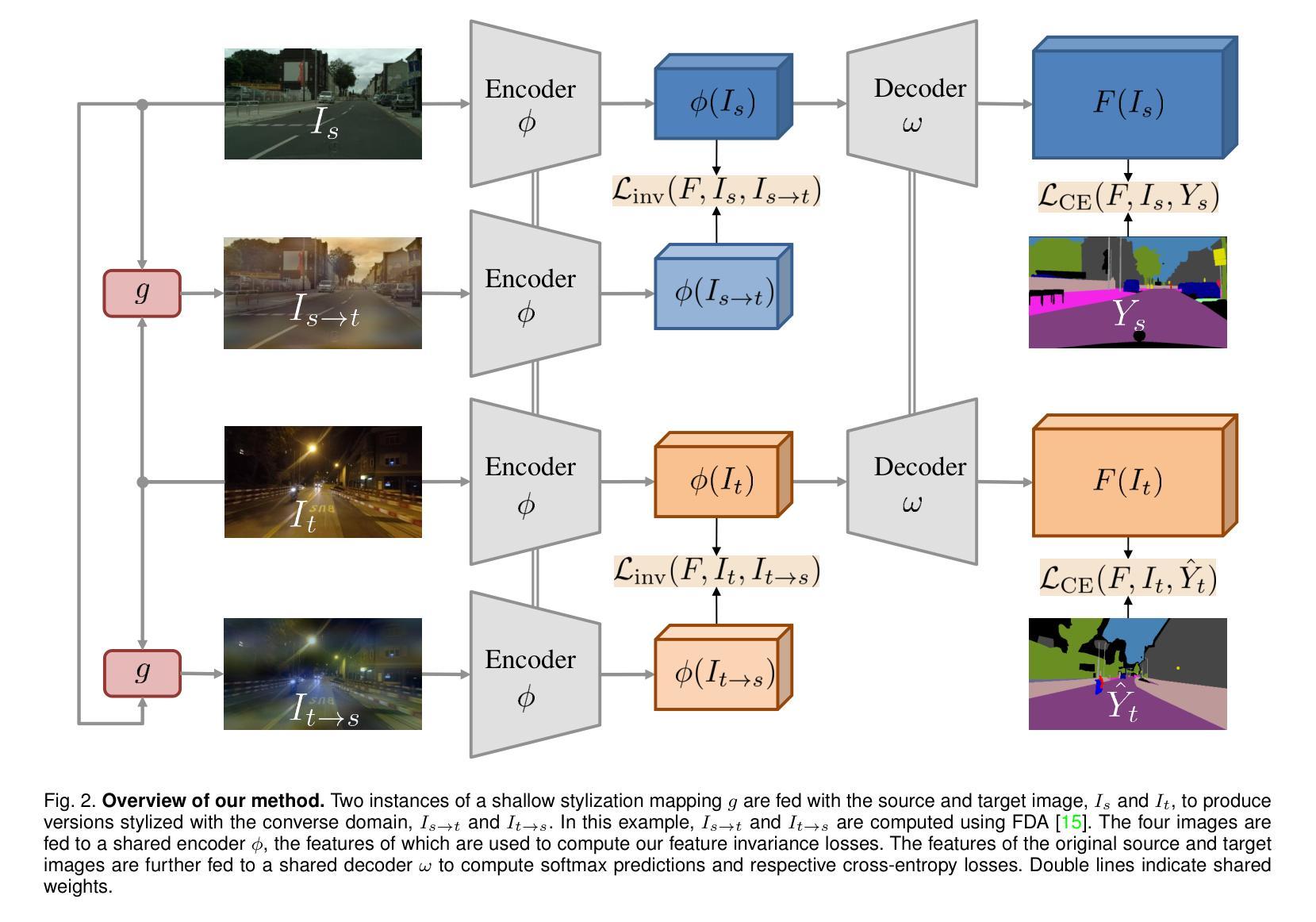
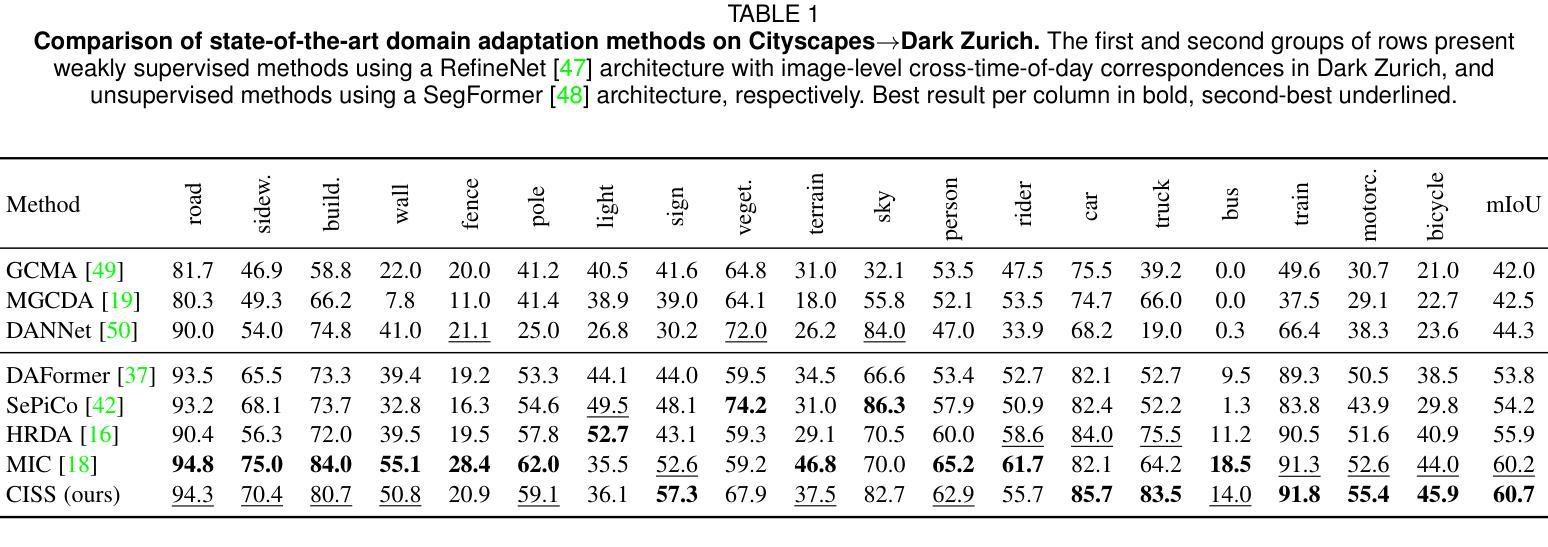
