⚠️ 以下所有内容总结都来自于 大语言模型的能力,如有错误,仅供参考,谨慎使用
🔴 请注意:千万不要用于严肃的学术场景,只能用于论文阅读前的初筛!
💗 如果您觉得我们的项目对您有帮助 ChatPaperFree ,还请您给我们一些鼓励!⭐️ HuggingFace免费体验
2025-01-24 更新
DWTNeRF: Boosting Few-shot Neural Radiance Fields via Discrete Wavelet Transform
Authors:Hung Nguyen, Blark Runfa Li, Truong Nguyen
Neural Radiance Fields (NeRF) has achieved superior performance in novel view synthesis and 3D scene representation, but its practical applications are hindered by slow convergence and reliance on dense training views. To this end, we present DWTNeRF, a unified framework based on Instant-NGP’s fast-training hash encoding. It is coupled with regularization terms designed for few-shot NeRF, which operates on sparse training views. Our DWTNeRF includes a novel Discrete Wavelet loss that allows explicit prioritization of low frequencies directly in the training objective, reducing few-shot NeRF’s overfitting on high frequencies in earlier training stages. We additionally introduce a model-based approach, based on multi-head attention, that is compatible with INGP-based models, which are sensitive to architectural changes. On the 3-shot LLFF benchmark, DWTNeRF outperforms Vanilla NeRF by 15.07% in PSNR, 24.45% in SSIM and 36.30% in LPIPS. Our approach encourages a re-thinking of current few-shot approaches for INGP-based models.
神经辐射场(NeRF)在新型视图合成和3D场景表示方面取得了卓越的性能,但其实际应用受到了收敛速度慢和依赖密集训练视图的影响。为此,我们提出了DWTNeRF,一个基于Instant-NGP快速训练哈希编码的统一框架。它与针对少量射击NeRF设计的正则化术语相结合,在稀疏训练视图上运行。我们的DWTNeRF包括一种新型离散小波损失,允许在训练目标中直接明确优先低频,减少早期训练阶段中少量射击NeRF对高频的过拟合。此外,我们还引入了一种基于多头注意力机制的模型方法,这种方法与敏感的架构变化兼容的INGP模型兼容。在3次射击的LLFF基准测试中,DWTNeRF在PSNR上优于普通NeRF 15.07%,在SSIM上高出24.45%,在LPIPS上高出36.30%。我们的方法鼓励对当前的基于INGP模型的少量射击方法进行重新思考。
论文及项目相关链接
PDF 10 pages, 6 figures
Summary
NeRF技术已在新型视图合成和3D场景表示方面取得了卓越性能,但其实际应用受到缓慢收敛和依赖密集训练视图的限制。为此,我们提出了DWTNeRF,一个基于Instant-NGP快速训练哈希编码的统一框架,与针对稀疏训练视图设计的正则化术语相结合。DWTNeRF包括一种新型离散小波损失,允许直接在训练目标中明确优先处理低频信息,减少早期训练阶段中few-shot NeRF对高频的过拟合。此外,我们还引入了一种基于多头注意力的模型方法,与INGP模型兼容,INGP模型对架构变化敏感。在3次拍摄的LLFF基准测试中,DWTNeRF较普通NeRF在PSNR上提高了15.07%,在SSIM上提高了24.45%,在LPIPS上提高了36.30%。我们的方法鼓励重新思考当前的INGP模型的few-shot方法。
Key Takeaways
- DWTNeRF是基于Instant-NGP快速训练哈希编码的统一框架,适用于稀疏训练视图。
- DWTNeRF引入了一种新型离散小波损失,能够减少早期训练阶段中few-shot NeRF对高频的过拟合。
- 通过在训练目标中明确优先处理低频信息,DWTNeRF提高了模型的性能。
- DWTNeRF提出了一种基于多头注意力的模型方法,与INGP模型兼容。
- 在LLFF基准测试中,DWTNeRF显著优于普通NeRF。
- DWTNeRF的方法为重新思考当前的few-shot方法在INGP模型中的应用提供了新的视角。
点此查看论文截图
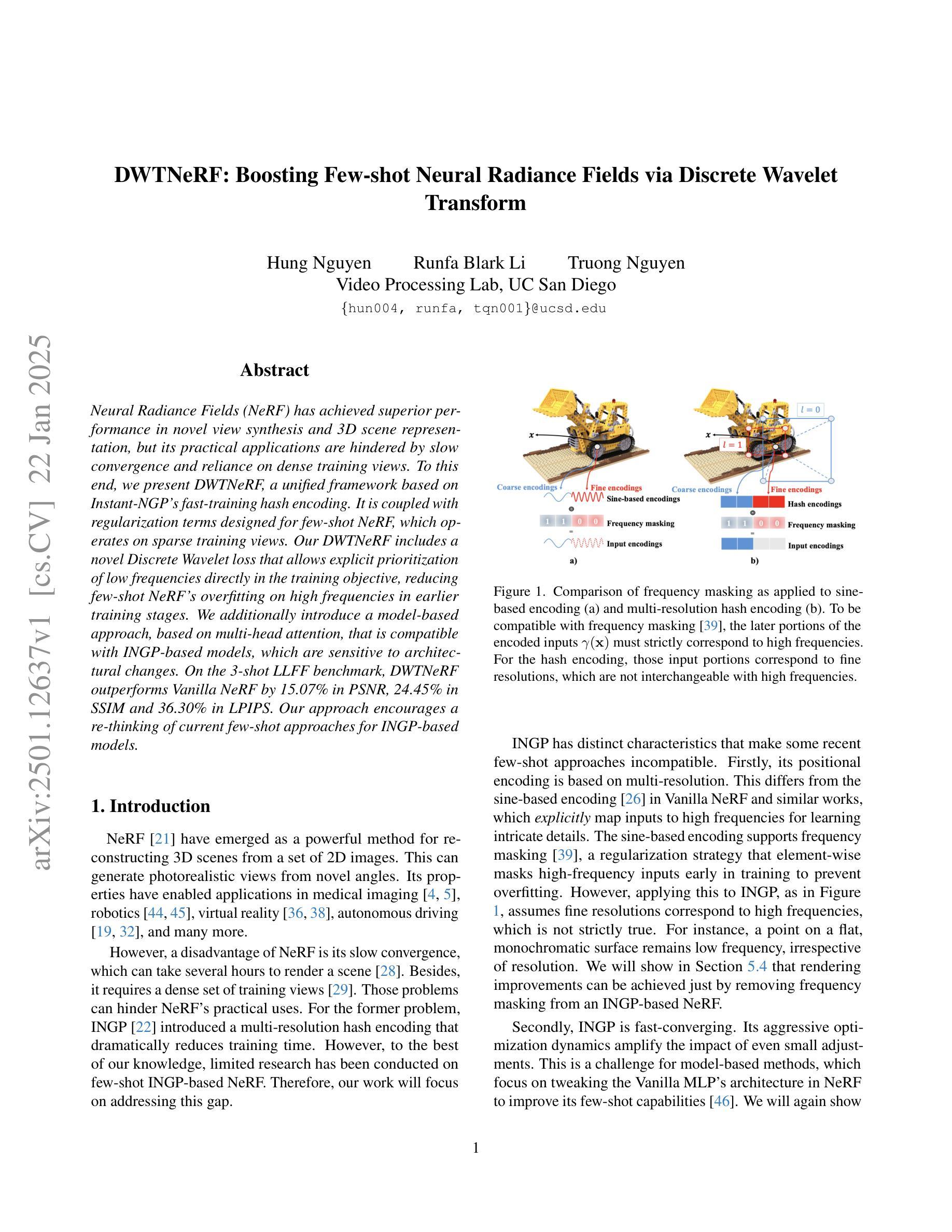
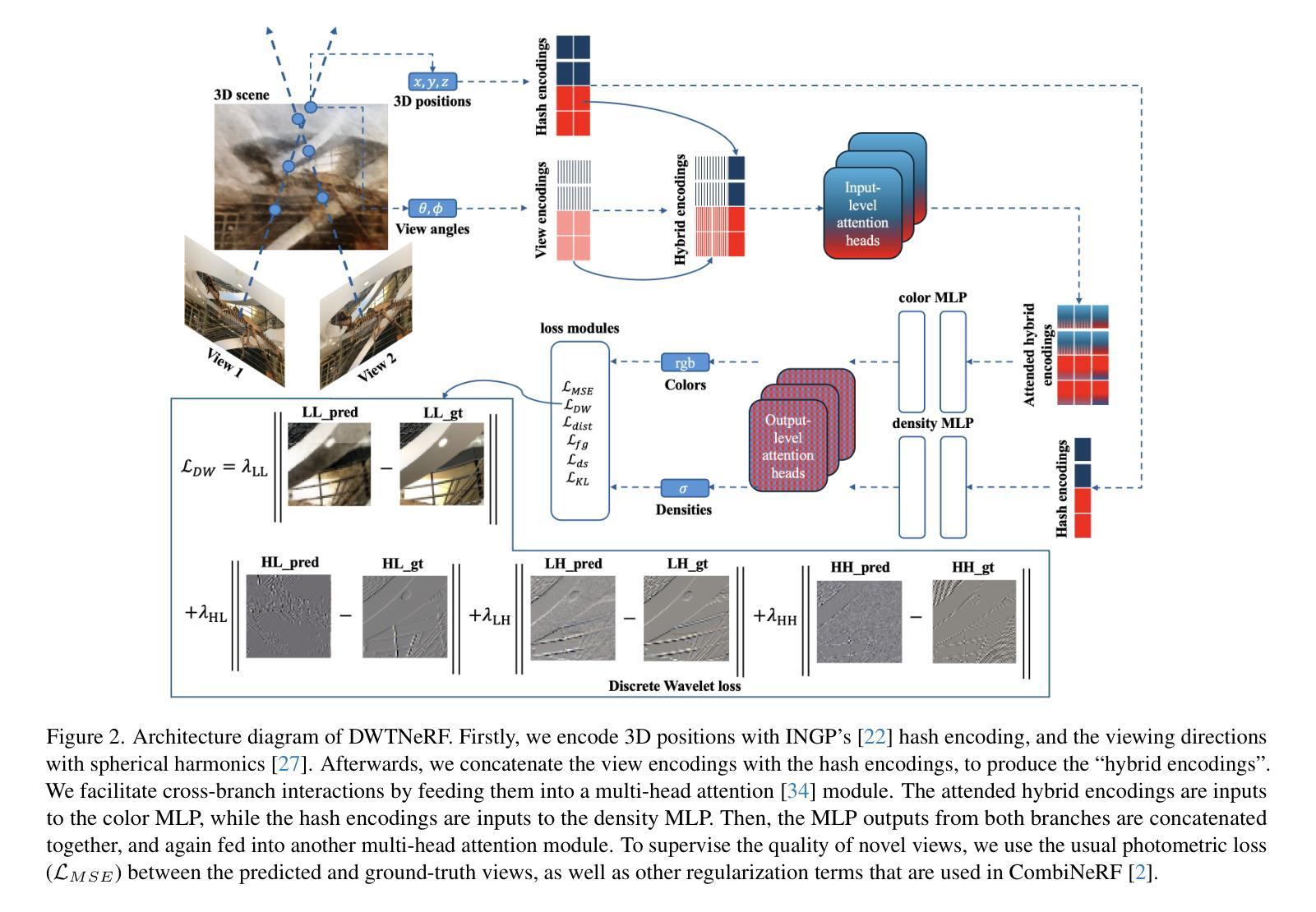
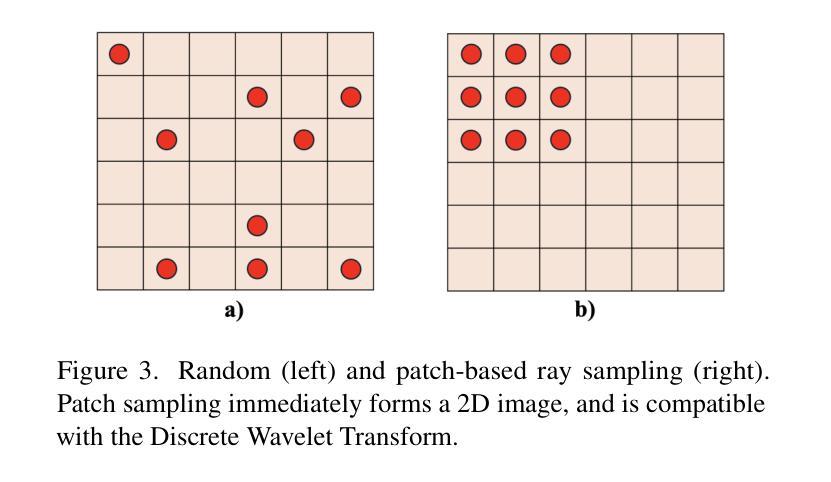
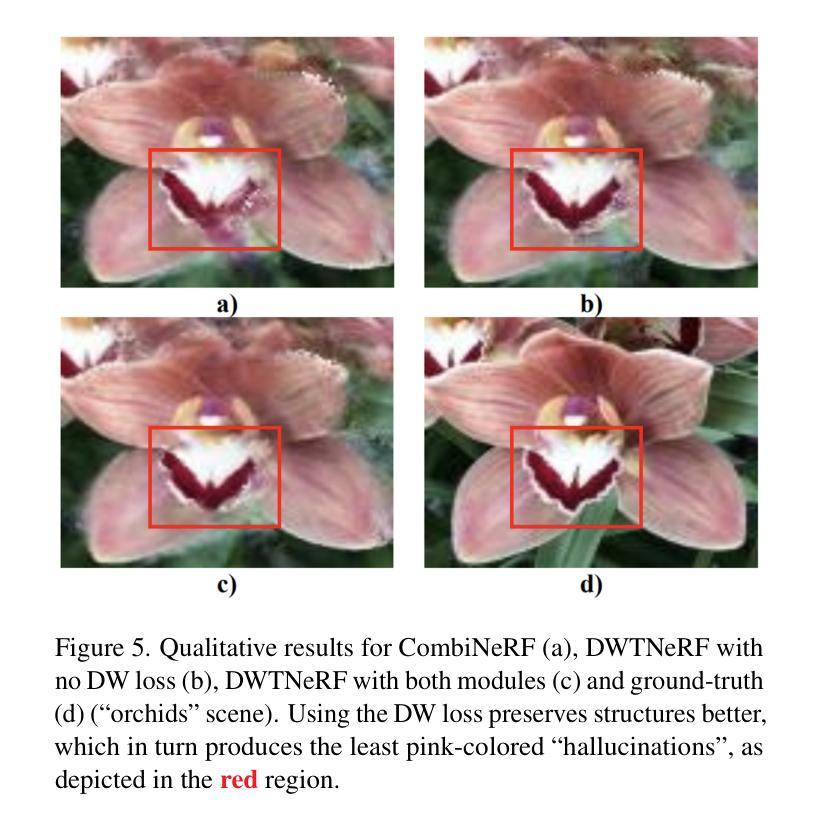
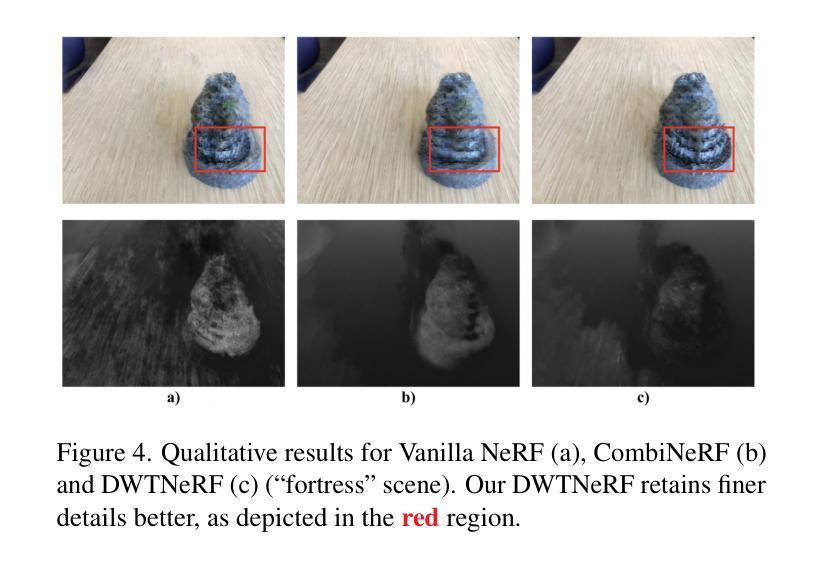
Developing Cryptocurrency Trading Strategy Based on Autoencoder-CNN-GANs Algorithms
Authors:Zhuohuan Hu, Richard Yu, Zizhou Zhang, Haoran Zheng, Qianying Liu, Yining Zhou
This paper leverages machine learning algorithms to forecast and analyze financial time series. The process begins with a denoising autoencoder to filter out random noise fluctuations from the main contract price data. Then, one-dimensional convolution reduces the dimensionality of the filtered data and extracts key information. The filtered and dimensionality-reduced price data is fed into a GANs network, and its output serve as input of a fully connected network. Through cross-validation, a model is trained to capture features that precede large price fluctuations. The model predicts the likelihood and direction of significant price changes in real-time price sequences, placing trades at moments of high prediction accuracy. Empirical results demonstrate that using autoencoders and convolution to filter and denoise financial data, combined with GANs, achieves a certain level of predictive performance, validating the capabilities of machine learning algorithms to discover underlying patterns in financial sequences. Keywords - CNN;GANs; Cryptocurrency; Prediction.
本文利用机器学习算法对金融时间序列进行预测和分析。流程始于使用降噪自编码器过滤主合约价格数据中的随机噪声波动。然后,一维卷积降低了过滤数据的维度并提取了关键信息。经过过滤和降维的价格数据被输入到GANs网络中,其输出作为全连接网络的输入。通过交叉验证,训练模型以捕获先于大价格波动的特征。该模型预测实时价格序列中重大价格变动的可能性和方向,在高预测准确性的时刻进行交易。实证结果表明,结合使用自编码器和卷积进行金融数据的过滤和降噪以及使用GANs达到了一定的预测性能,验证了机器学习算法在发现金融序列中潜在模式方面的能力。关键词——卷积神经网络;生成对抗网络;加密货币;预测。
论文及项目相关链接
PDF The paper was accepted by 2024 4th International Conference on Artificial Intelligence, Robotics, and Communication(ICAIRC 2024)
Summary:本文利用机器学习算法对金融时间序列进行预测与分析。通过降噪自编码器过滤原始合约价格数据中的随机噪声波动,一维卷积降低数据维度并提取关键信息。经过处理的数据输入生成对抗网络,其输出再进入全连接网络。通过交叉验证,训练模型捕捉预示大价格波动的特征,实时预测价格变动的可能性和方向,并在预测准确率高的时刻进行交易。实证结果表明,结合自编码器、卷积和生成对抗网络,对金融数据具有一定的预测性能,验证了机器学习算法在发现金融序列潜在模式方面的能力。
Key Takeaways:
- 利用降噪自编码器过滤金融数据中的随机噪声。
- 一维卷积用于降低数据维度并提取关键信息。
- 生成对抗网络用于训练模型,以捕捉预示大价格波动的特征。
- 全连接网络用于实时预测价格变动的可能性和方向。
- 通过交叉验证训练模型,提高预测准确率。
- 实证结果表明,结合自编码器、卷积和GANs对金融数据具有预测性能。
- 机器学习算法在发现金融序列潜在模式方面具能力。
点此查看论文截图
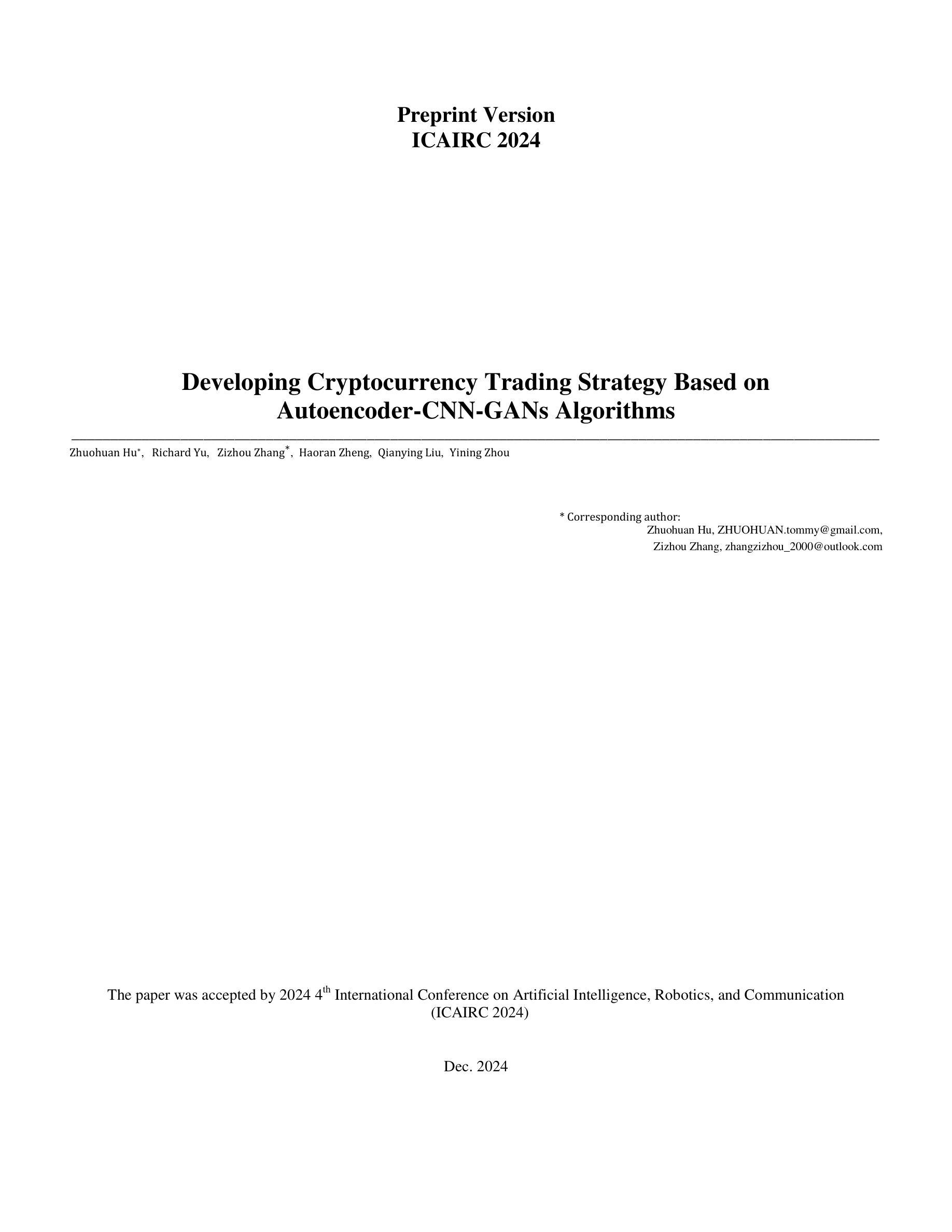
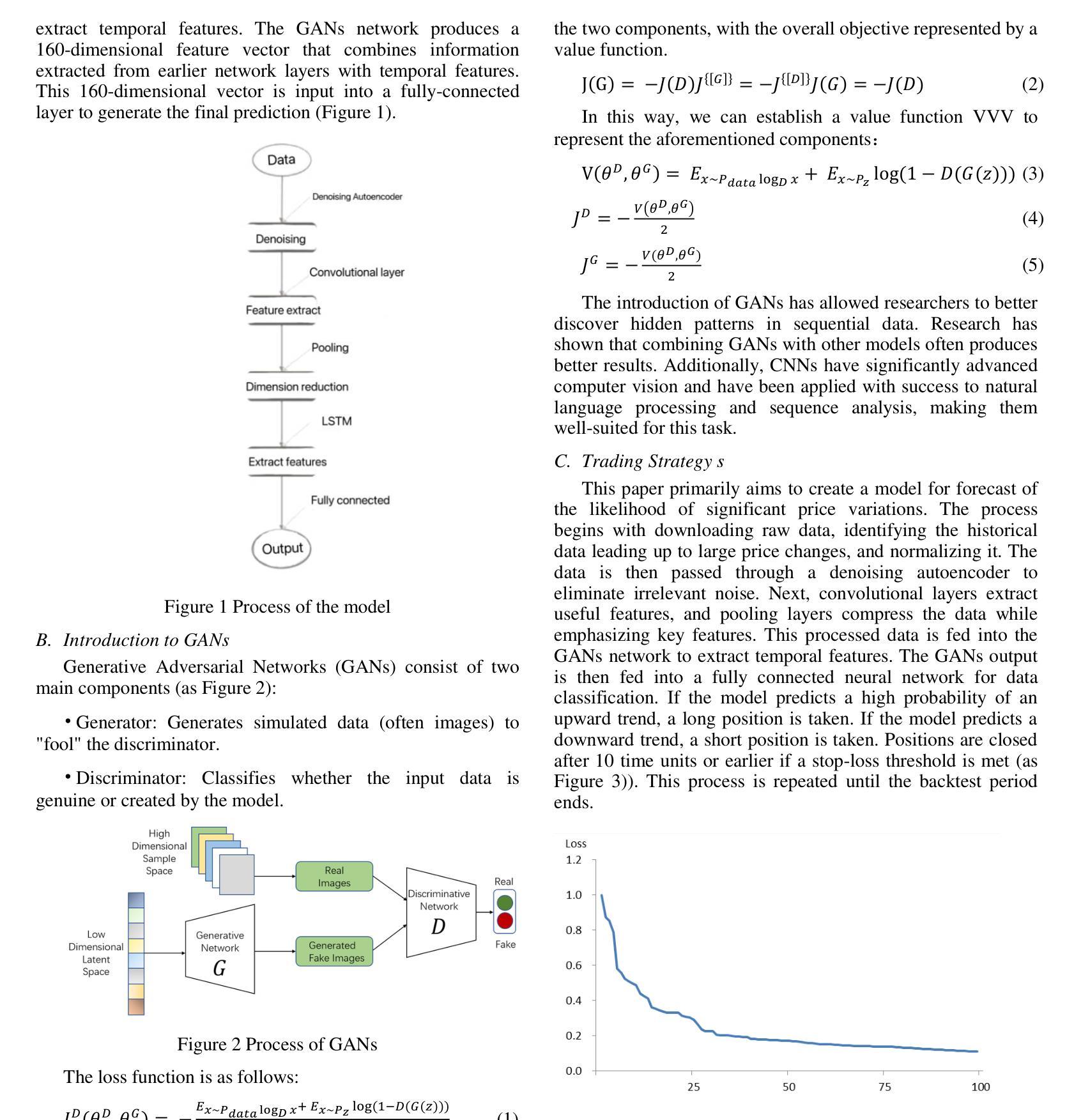
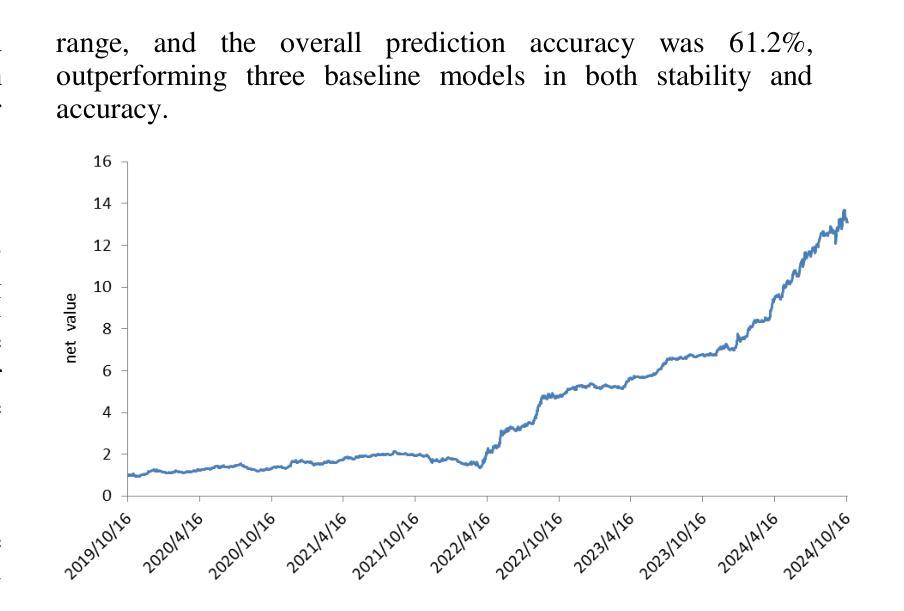