⚠️ 以下所有内容总结都来自于 大语言模型的能力,如有错误,仅供参考,谨慎使用
🔴 请注意:千万不要用于严肃的学术场景,只能用于论文阅读前的初筛!
💗 如果您觉得我们的项目对您有帮助 ChatPaperFree ,还请您给我们一些鼓励!⭐️ HuggingFace免费体验
2025-01-25 更新
PhotoGAN: Generative Adversarial Neural Network Acceleration with Silicon Photonics
Authors:Tharini Suresh, Salma Afifi, Sudeep Pasricha
Generative Adversarial Networks (GANs) are at the forefront of AI innovation, driving advancements in areas such as image synthesis, medical imaging, and data augmentation. However, the unique computational operations within GANs, such as transposed convolutions and instance normalization, introduce significant inefficiencies when executed on traditional electronic accelerators, resulting in high energy consumption and suboptimal performance. To address these challenges, we introduce PhotoGAN, the first silicon-photonic accelerator designed to handle the specialized operations of GAN models. By leveraging the inherent high throughput and energy efficiency of silicon photonics, PhotoGAN offers an innovative, reconfigurable architecture capable of accelerating transposed convolutions and other GAN-specific layers. The accelerator also incorporates a sparse computation optimization technique to reduce redundant operations, improving computational efficiency. Our experimental results demonstrate that PhotoGAN achieves at least 4.4x higher GOPS and 2.18x lower energy-per-bit (EPB) compared to state-of-the-art accelerators, including GPUs and TPUs. These findings showcase PhotoGAN as a promising solution for the next generation of GAN acceleration, providing substantial gains in both performance and energy efficiency.
生成对抗网络(GANs)是人工智能创新的前沿,推动着图像合成、医学影像和数据增强等领域的进步。然而,GANs中的独特计算操作,如转置卷积和实例归一化,在传统电子加速器上执行时会出现显著的低效情况,导致能耗高和性能不佳。为了应对这些挑战,我们推出了PhotoGAN,这是首款设计用于处理GAN模型特殊操作的硅光子加速器。通过利用硅光子学固有的高吞吐量和能效优势,PhotoGAN提供了一种创新的可重构架构,能够加速转置卷积和其他GAN特定层。该加速器还采用稀疏计算优化技术,减少冗余操作,提高计算效率。我们的实验结果表明,与包括GPU和TPU在内的最新加速器相比,PhotoGAN至少实现了4.4倍的更高GOPs和2.18倍更低的每比特能耗(EPB)。这些发现展示了PhotoGAN作为下一代GAN加速器的有前途的解决方案,在性能和能源效率方面都实现了显著的提升。
论文及项目相关链接
Summary
GAN因其在图像合成、医学影像、数据增强等领域的卓越表现,已成为人工智能创新的前沿。然而,其在传统电子加速器上执行时,由于特殊运算操作如转置卷积和实例归一化,存在高能耗和性能不佳的问题。为解决这些问题,我们推出了PhotoGAN——首款用于处理GAN模型特殊运算的硅光子加速器。借助硅光子学的高吞吐量和能效优势,PhotoGAN提供可重构架构,可加速转置卷积等GAN特定层。加速器还采用稀疏计算优化技术减少冗余操作,提高计算效率。实验结果显示,相较于GPU和TPU等现有加速器,PhotoGAN至少实现4.4倍更高的GOPs和2.18倍更低的每比特能耗(EPB)。这为下一代GAN加速提供了极具潜力的解决方案。
Key Takeaways
- GANs在多个领域表现卓越,但仍面临传统电子加速器上的性能挑战。
- PhotoGAN是首款硅光子加速器,专为处理GAN模型的特殊运算设计。
- PhotoGAN利用硅光子学的高吞吐量和能效优势。
- PhotoGAN提供可重构架构,加速转置卷积等GAN特定层运算。
- 加速器采用稀疏计算优化技术以提高计算效率。
- 实验结果显示PhotoGAN在性能与能效上均显著优于现有加速器。
点此查看论文截图
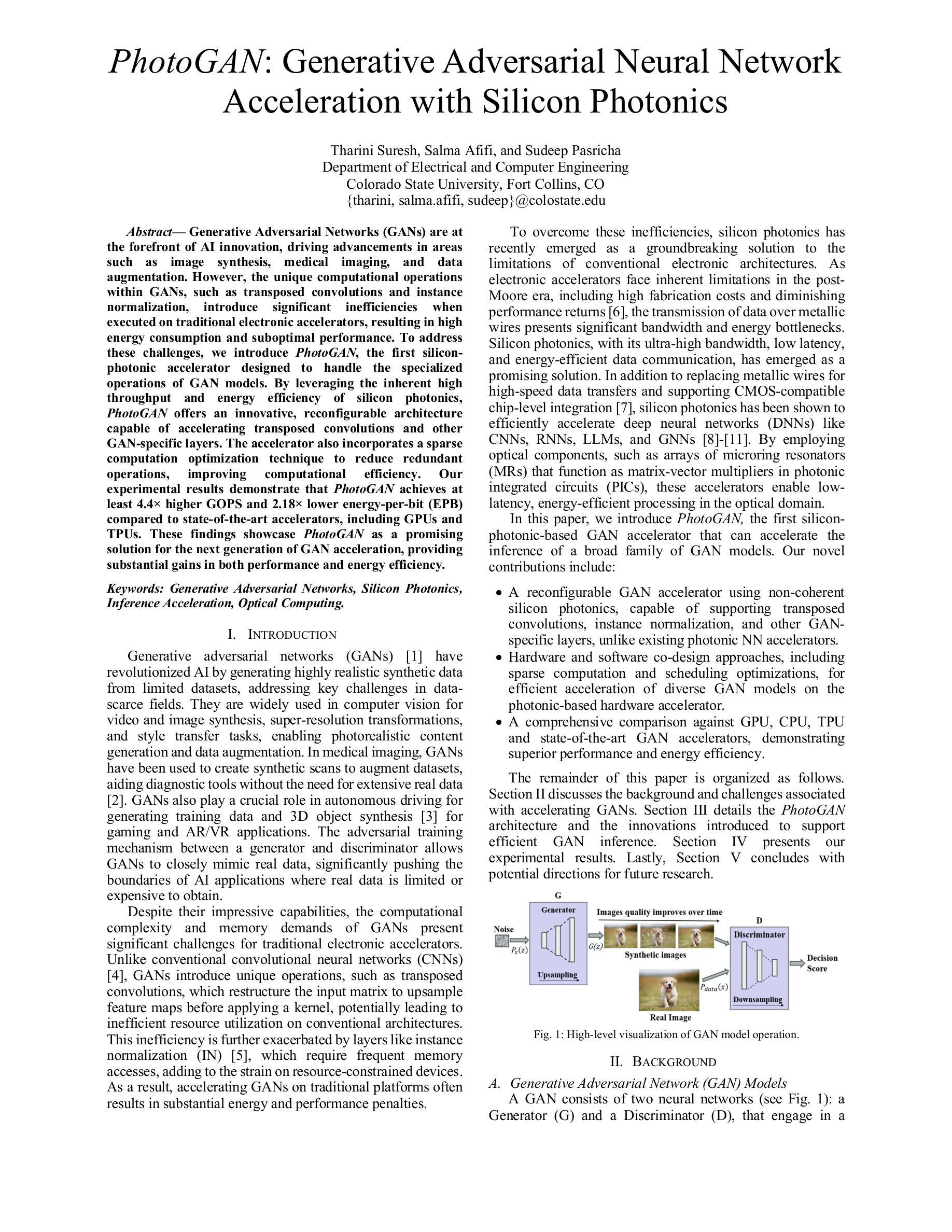
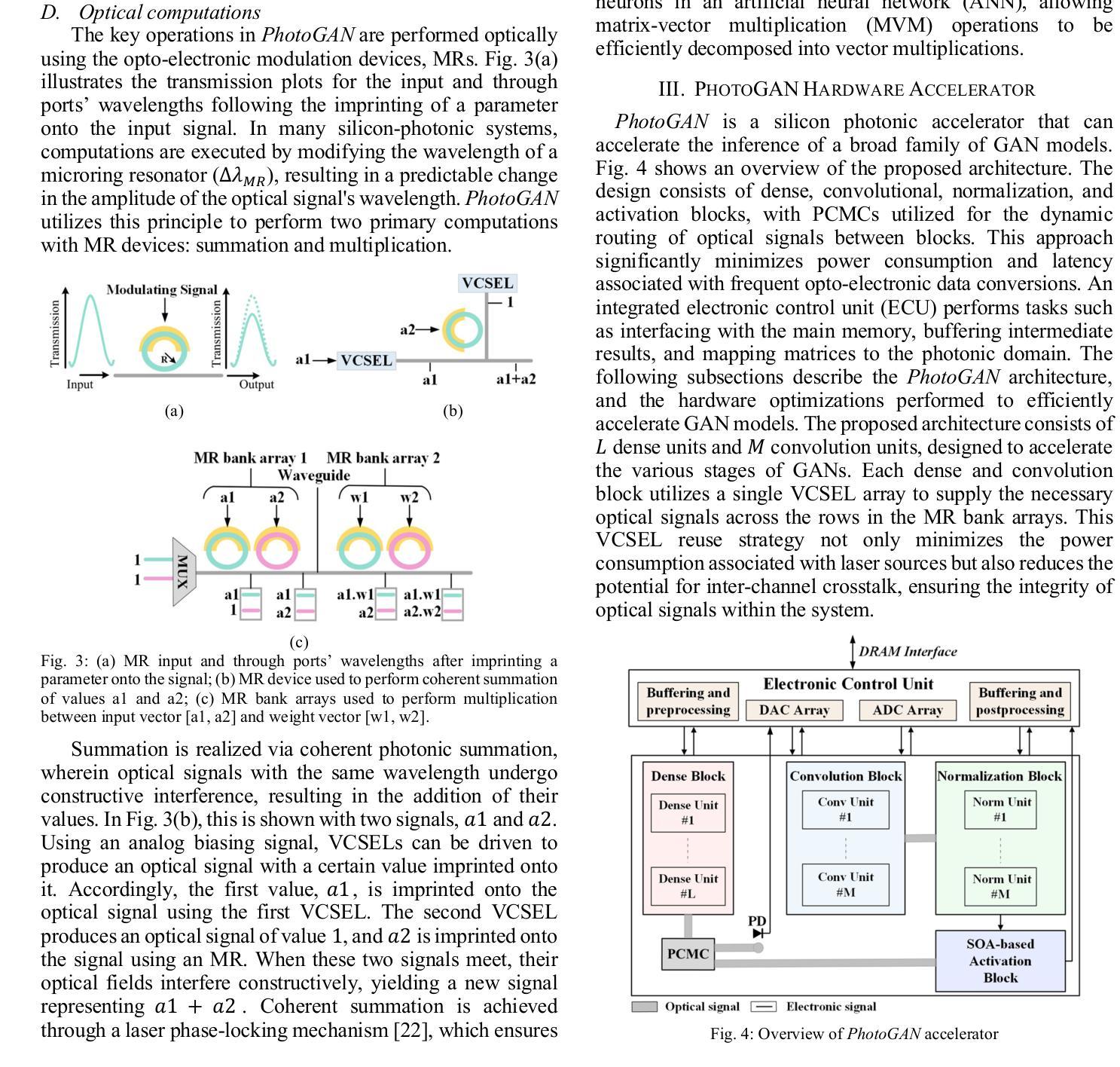
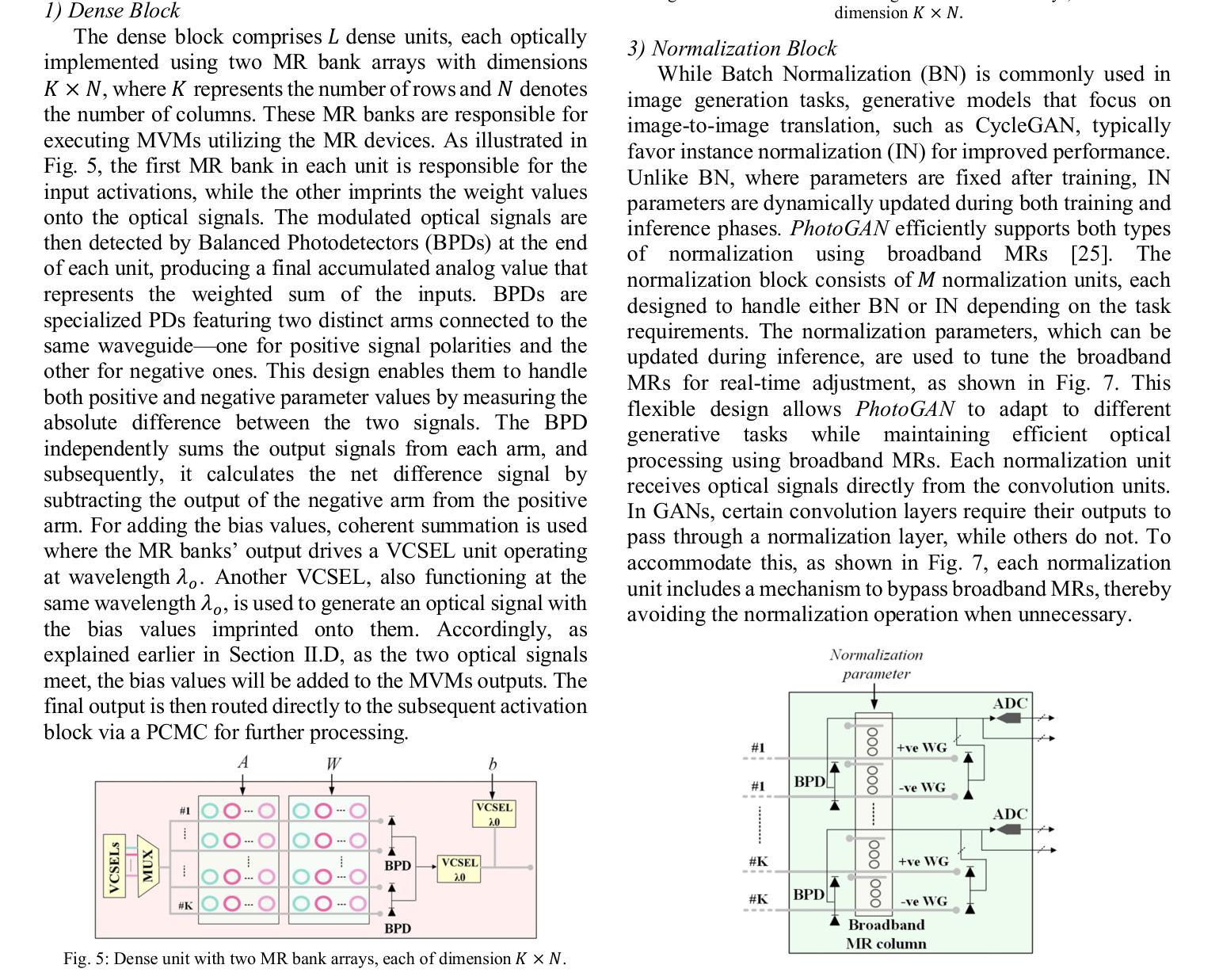
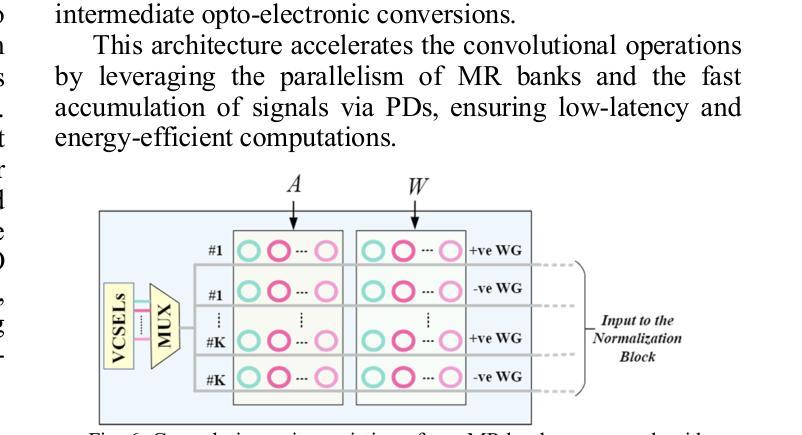
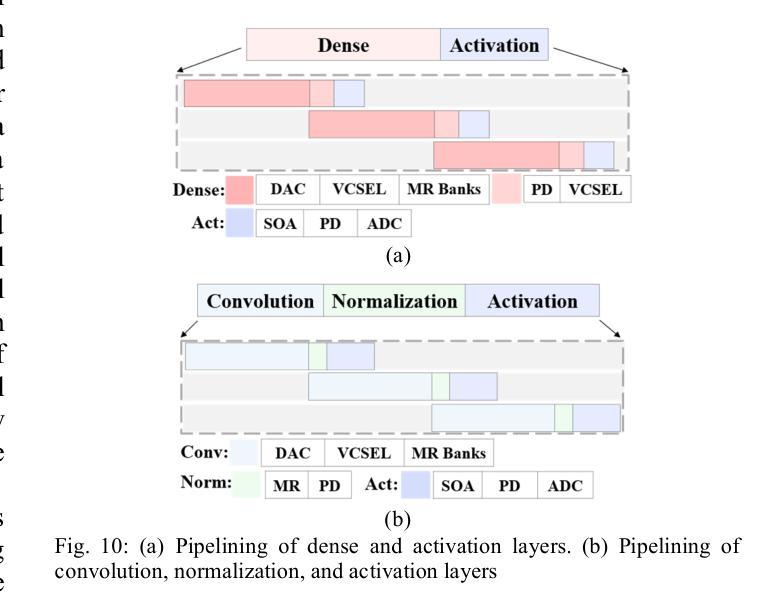
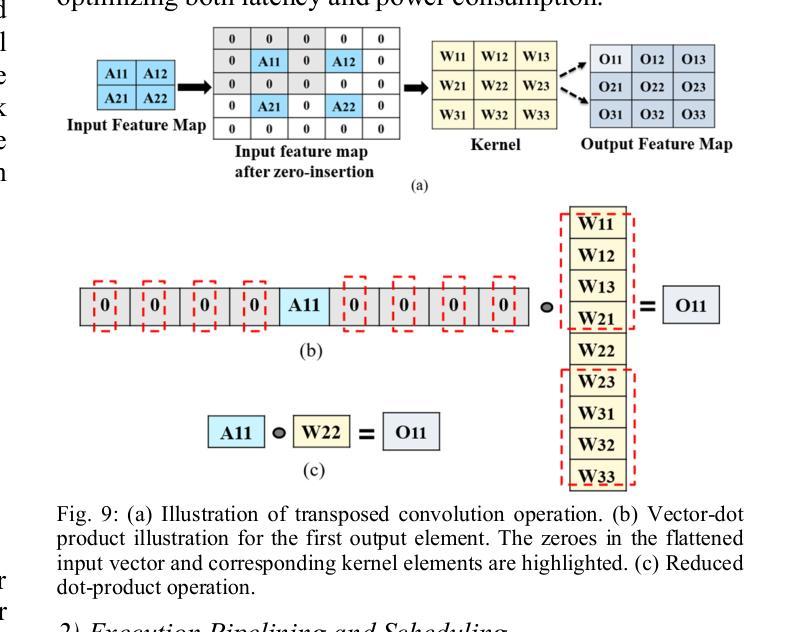
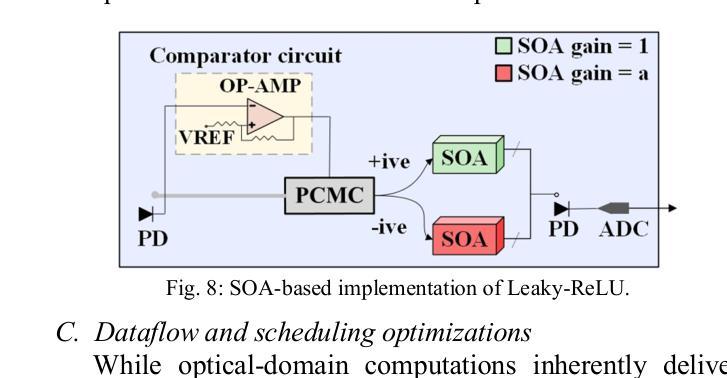
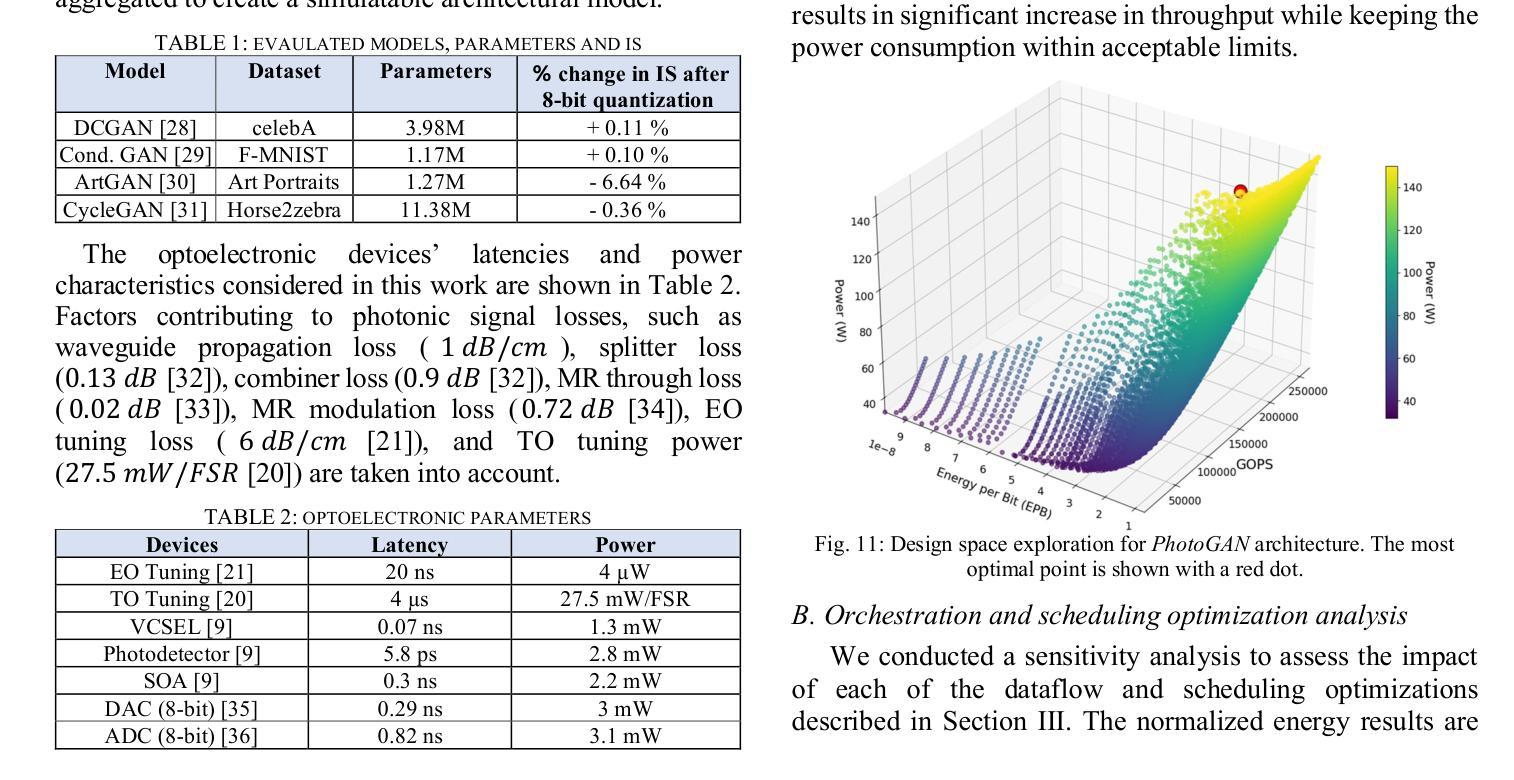
LDR-Net: A Novel Framework for AI-generated Image Detection via Localized Discrepancy Representation
Authors:JiaXin Chen, Miao Hu, DengYong Zhang, Yun Song, Xin Liao
With the rapid advancement of generative models, the visual quality of generated images has become nearly indistinguishable from the real ones, posing challenges to content authenticity verification. Existing methods for detecting AI-generated images primarily focus on specific forgery clues, which are often tailored to particular generative models like GANs or diffusion models. These approaches struggle to generalize across architectures. Building on the observation that generative images often exhibit local anomalies, such as excessive smoothness, blurred textures, and unnatural pixel variations in small regions, we propose the localized discrepancy representation network (LDR-Net), a novel approach for detecting AI-generated images. LDR-Net captures smoothing artifacts and texture irregularities, which are common but often overlooked. It integrates two complementary modules: local gradient autocorrelation (LGA) which models local smoothing anomalies to detect smoothing anomalies, and local variation pattern (LVP) which captures unnatural regularities by modeling the complexity of image patterns. By merging LGA and LVP features, a comprehensive representation of localized discrepancies can be provided. Extensive experiments demonstrate that our LDR-Net achieves state-of-the-art performance in detecting generated images and exhibits satisfactory generalization across unseen generative models. The code will be released upon acceptance of this paper.
随着生成模型的快速发展,生成图像的视觉质量已经变得与真实图像几乎无法区分,这给内容真实性验证带来了挑战。现有的检测AI生成图像的方法主要关注特定的伪造线索,这些线索通常针对特定的生成模型,如GAN或扩散模型。这些方法在跨架构方面的推广能力较弱。基于观察到生成图像通常表现出局部异常,如过度平滑、纹理模糊以及小区域中的不自然像素变化,我们提出了局部差异表示网络(LDR-Net),这是一种检测AI生成图像的新方法。LDR-Net捕捉了常见的但常被忽略的平滑伪影和纹理不规则。它集成了两个互补的模块:局部梯度自相关(LGA),它模拟局部平滑异常来检测平滑异常;以及局部变化模式(LVP),它通过模拟图像模式的复杂性来捕捉不自然的规律性。通过合并LGA和LVP特征,可以提供对局部差异的综合表示。大量实验表明,我们的LDR-Net在检测生成图像方面达到了最先进的性能,并且在未见过的生成模型之间表现出令人满意的泛化能力。论文被接受后,代码将予以公开。
论文及项目相关链接
Summary
基于生成模型(如GANs和扩散模型)的快速发展,生成的图像与现实中的图像几乎无法区分,这给内容真实性验证带来了挑战。现有的检测AI生成图像的方法主要关注特定的伪造线索,但很难在不同架构之间进行推广。本文提出一种新型网络——局部差异表示网络(LDR-Net),用于捕捉生成图像中常见的局部异常,如过度平滑、纹理模糊和区域性像素变异。该方法整合了两个互补模块,实现高效准确的检测。
Key Takeaways
- 生成模型的快速发展使得生成的图像与现实中的图像难以区分,内容真实性验证面临挑战。
- 现有检测AI生成图像的方法主要关注特定伪造线索,但缺乏跨架构的通用性。
- LDR-Net通过捕捉生成图像中的局部异常(如过度平滑和纹理模糊)来检测图像是否生成。
- LDR-Net包含两个互补模块:局部梯度自相关(LGA)和局部变化模式(LVP)。
- LGA模块通过建模局部平滑异常来检测平滑异常。
- LVP模块通过建模图像模式的复杂性来捕捉不自然的规律性。
点此查看论文截图
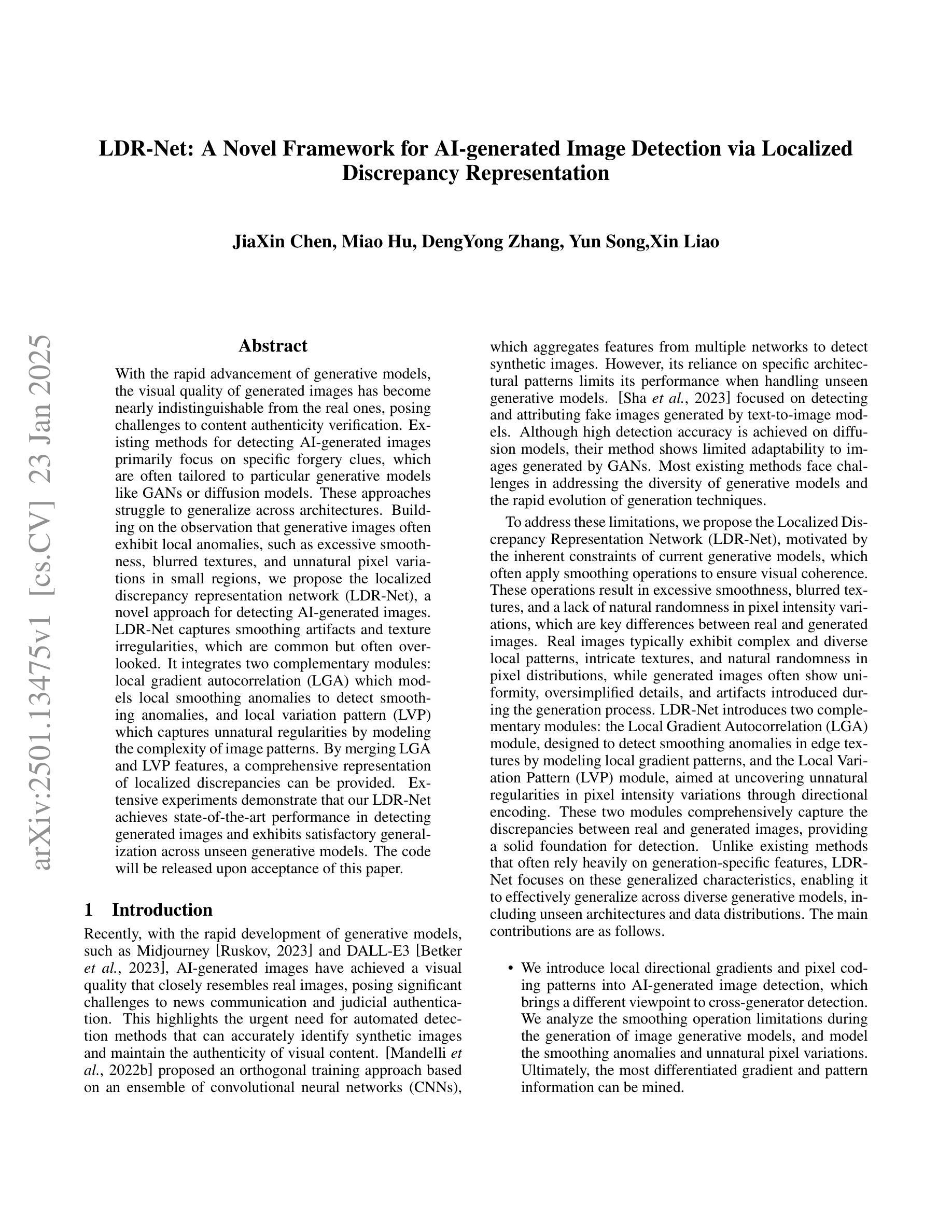
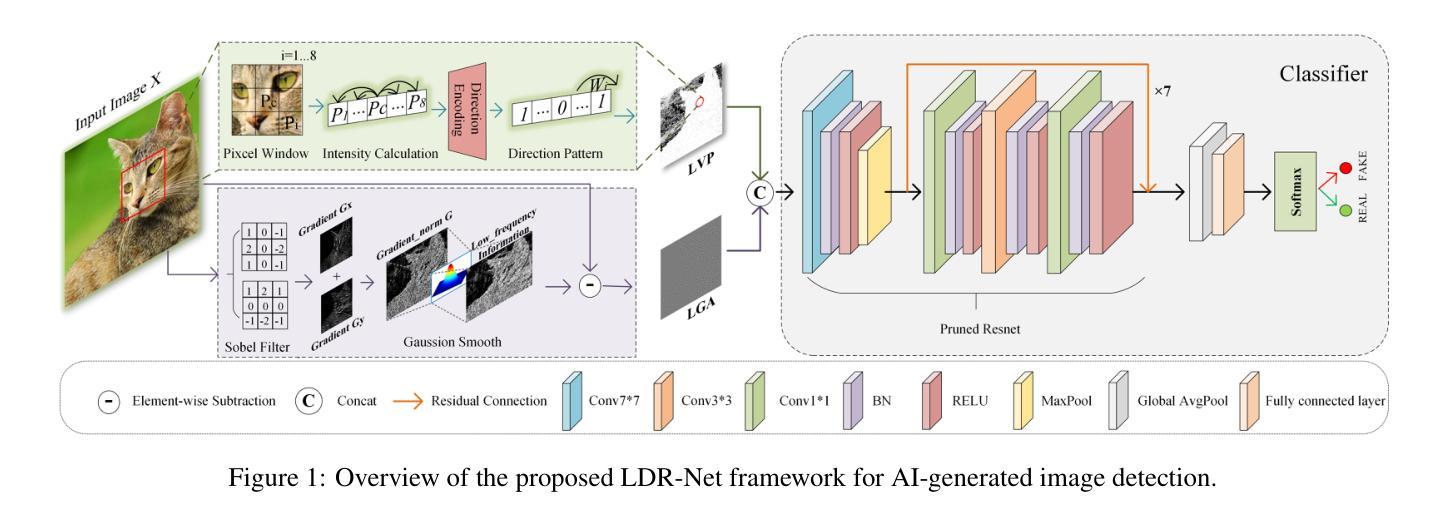
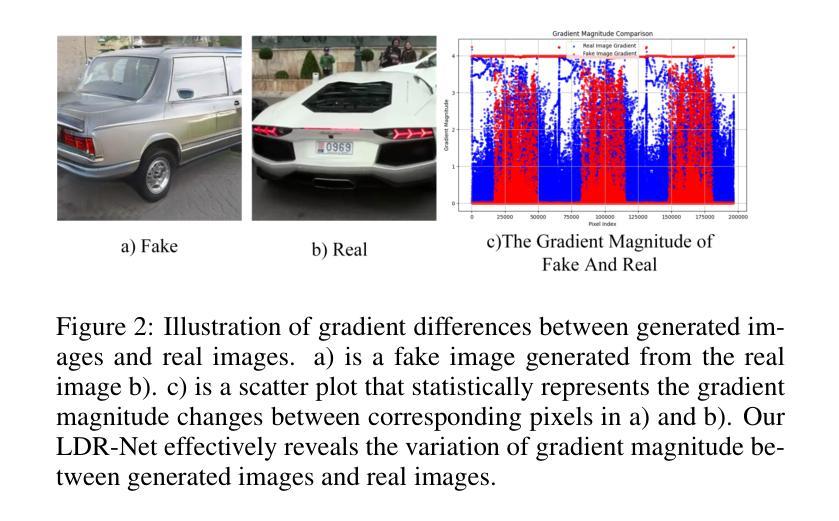
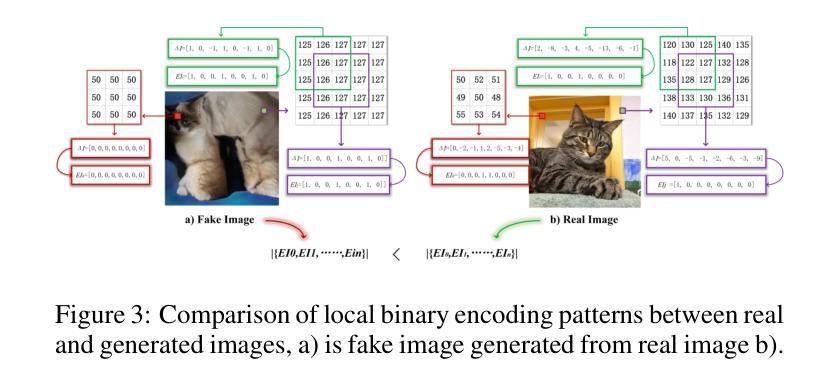
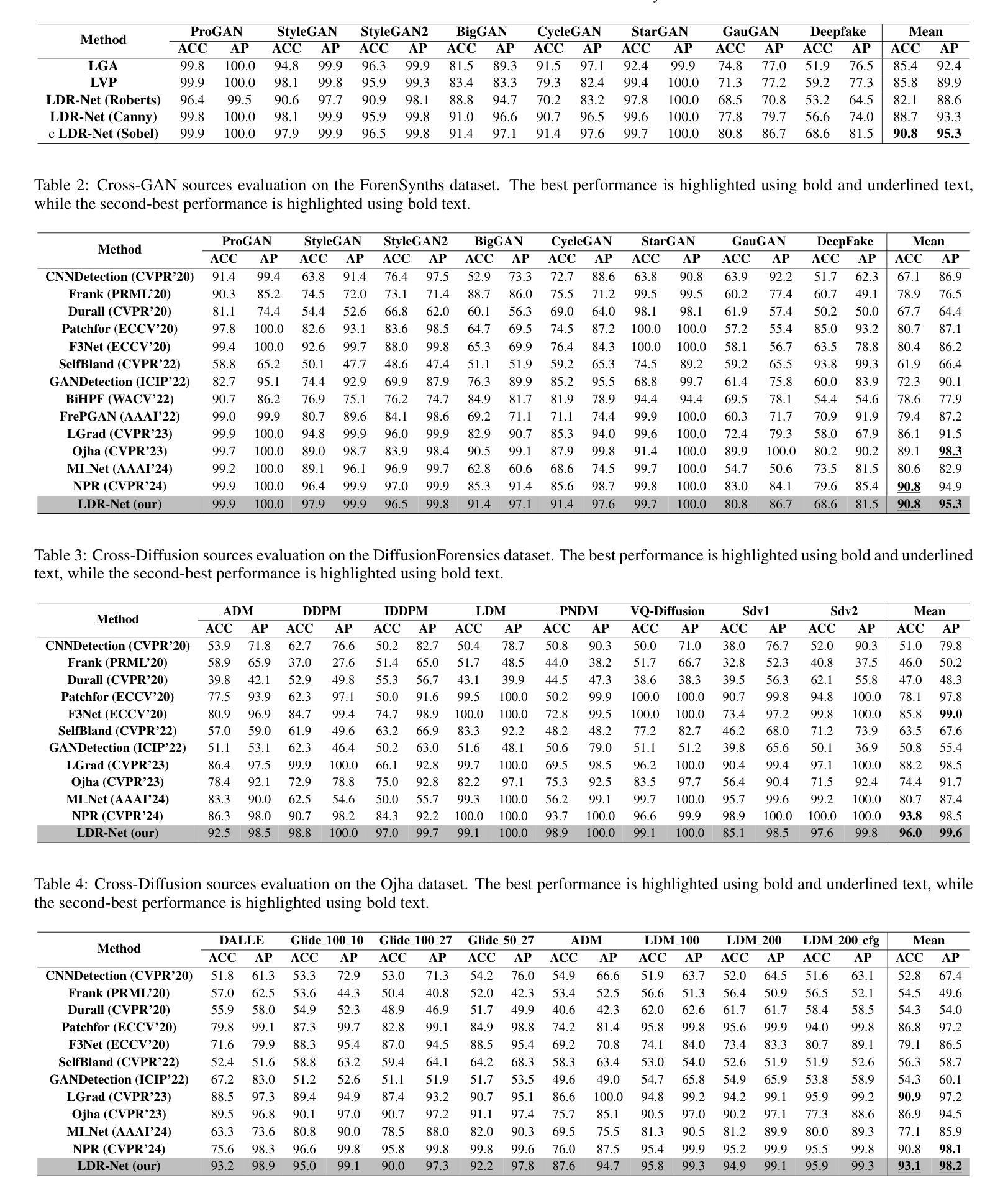
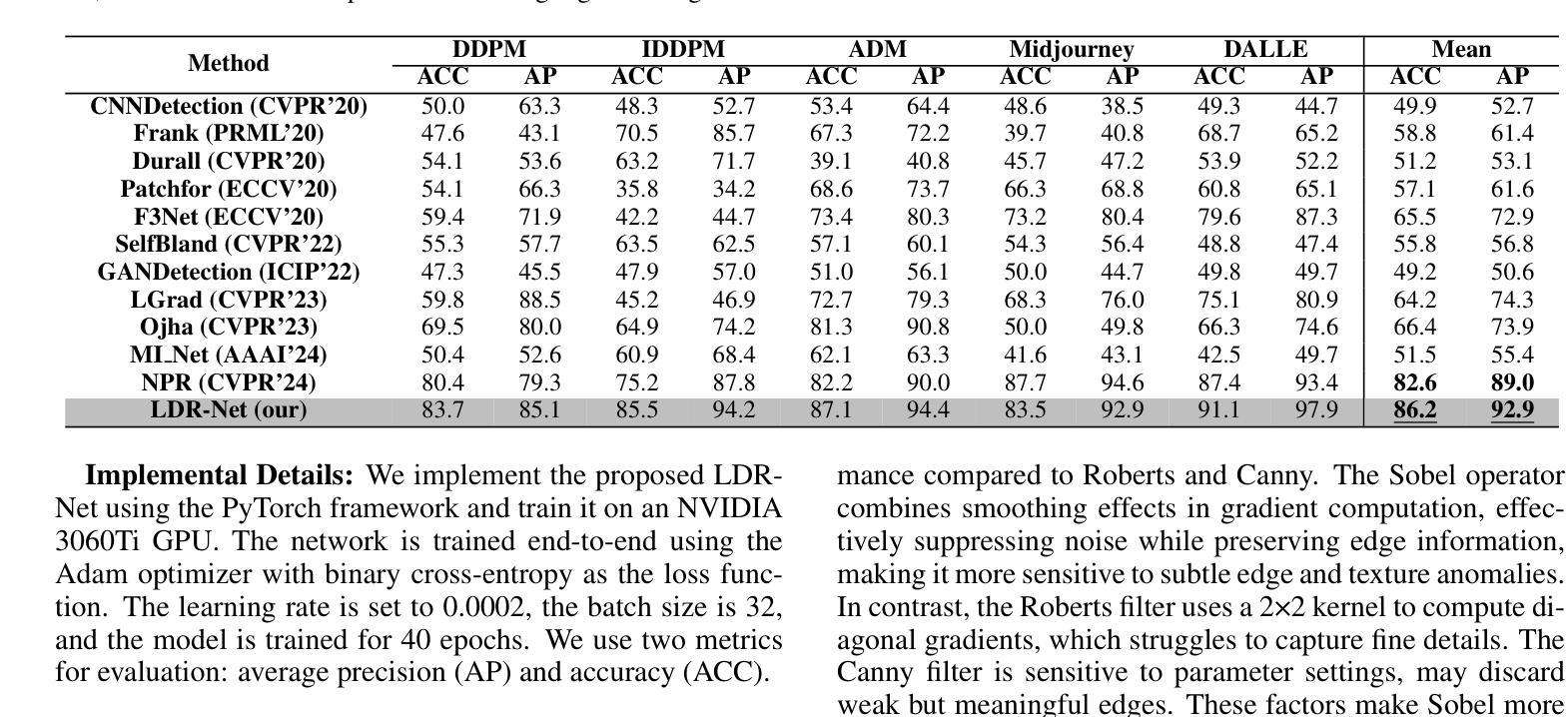
Graph Representation Learning with Diffusion Generative Models
Authors:Daniel Wesego
Diffusion models have established themselves as state-of-the-art generative models across various data modalities, including images and videos, due to their ability to accurately approximate complex data distributions. Unlike traditional generative approaches such as VAEs and GANs, diffusion models employ a progressive denoising process that transforms noise into meaningful data over multiple iterative steps. This gradual approach enhances their expressiveness and generation quality. Not only that, diffusion models have also been shown to extract meaningful representations from data while learning to generate samples. Despite their success, the application of diffusion models to graph-structured data remains relatively unexplored, primarily due to the discrete nature of graphs, which necessitates discrete diffusion processes distinct from the continuous methods used in other domains. In this work, we leverage the representational capabilities of diffusion models to learn meaningful embeddings for graph data. By training a discrete diffusion model within an autoencoder framework, we enable both effective autoencoding and representation learning tailored to the unique characteristics of graph-structured data. We only need the encoder at the end to extract representations. Our approach demonstrates the potential of discrete diffusion models to be used for graph representation learning.
扩散模型凭借其准确逼近复杂数据分布的能力,已经在各种数据模式(包括图像和视频)中确立了自己作为最先进的生成模型的地位。与传统的生成方法(如变分自编码器(VAEs)和生成对抗网络(GANs))不同,扩散模型采用渐进的去噪过程,通过多个迭代步骤将噪声逐渐转化为有意义的数据。这种逐步的方法增强了其表达力和生成质量。不仅如此,扩散模型还显示出在生成样本的同时从数据中提取有意义表示的能力。尽管它们取得了成功,但将扩散模型应用于图结构数据的尝试仍然相对较少,这主要是因为图的离散性质需要一种不同于其他领域使用的连续方法的离散扩散过程。在这项工作中,我们利用扩散模型的表示能力来学习图数据的有意义嵌入。通过在自编码器框架内训练离散扩散模型,我们实现了针对图结构数据的独特特性进行的有效自编码和表示学习。我们最终只需要编码器来提取表示。我们的方法展示了离散扩散模型在图表示学习中的潜力。
论文及项目相关链接
Summary
扩散模型通过逐步去噪过程,能够在图像和视频等多种数据模态上实现最先进的生成模型表现,准确逼近复杂数据分布。不同于传统的生成方法如变分自编码器和生成对抗网络,扩散模型的逐步方法增强了其表达力和生成质量。此外,扩散模型还能在学习生成样本的同时从数据中提取有意义的表现。尽管其在图结构数据的应用上仍有待探索,但本研究利用扩散模型的表示能力来学习图数据的有意义嵌入。通过在一个自编码器框架内训练离散扩散模型,实现了对图结构数据的有效自编码和表示学习。最终只需要编码器来提取表现。这表明离散扩散模型在图形表示学习上的潜力。
Key Takeaways
- 扩散模型已成为多种数据模态的先进生成模型,因其准确逼近复杂数据分布的能力。
- 不同于传统生成方法,扩散模型采用逐步去噪过程,增强了表达力和生成质量。
- 扩散模型能从数据中提取有意义的表现,同时学习生成样本。
- 离散扩散模型在图结构数据的应用上仍有待探索,因为图的离散性质需要特殊的处理方法。
- 研究利用离散扩散模型的表示能力来学习图数据的嵌入。
- 通过在自编码器框架内训练离散扩散模型,实现了对图结构数据的有效自编码和表示学习。
点此查看论文截图
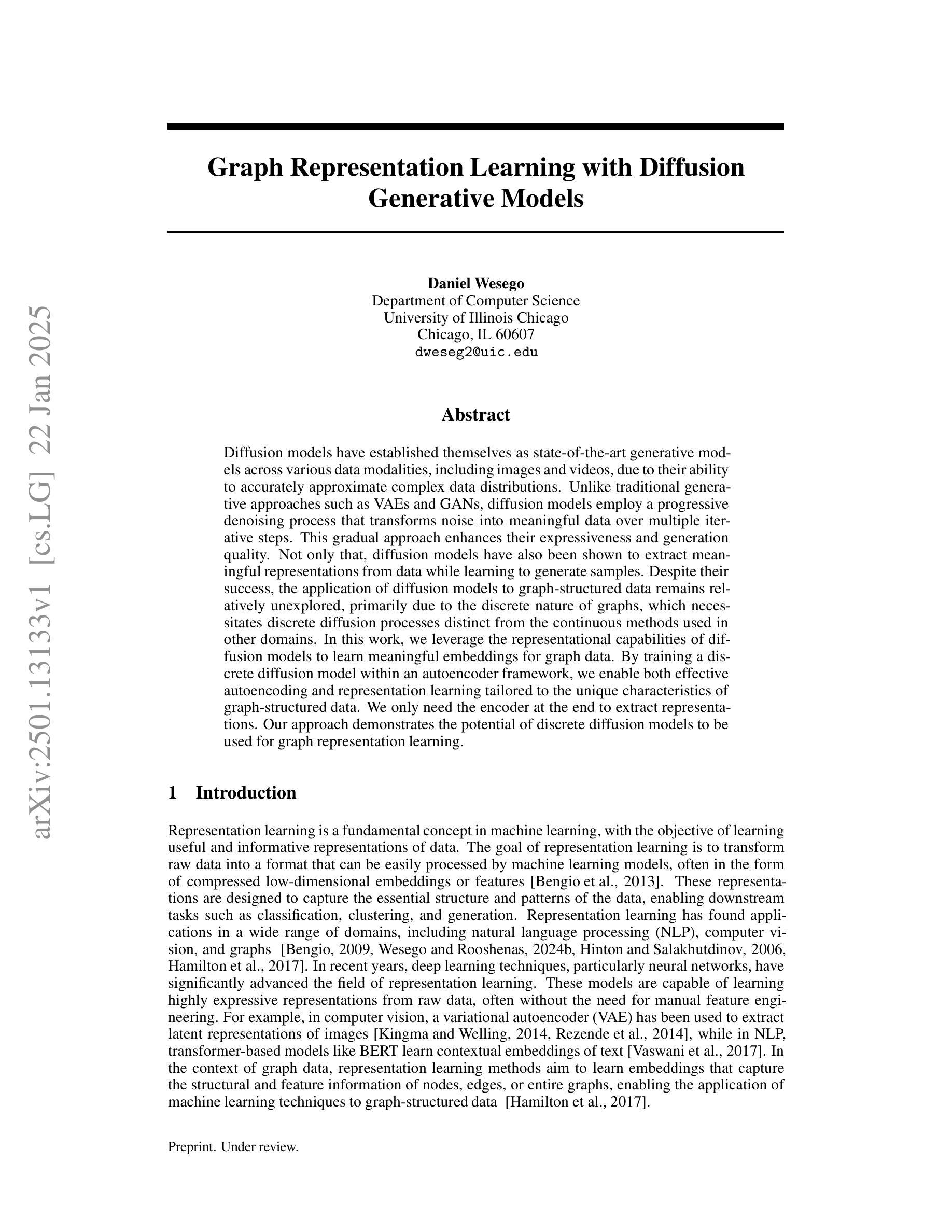
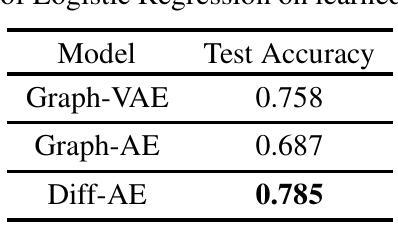