⚠️ 以下所有内容总结都来自于 大语言模型的能力,如有错误,仅供参考,谨慎使用
🔴 请注意:千万不要用于严肃的学术场景,只能用于论文阅读前的初筛!
💗 如果您觉得我们的项目对您有帮助 ChatPaperFree ,还请您给我们一些鼓励!⭐️ HuggingFace免费体验
2025-01-28 更新
Relightable Full-Body Gaussian Codec Avatars
Authors:Shaofei Wang, Tomas Simon, Igor Santesteban, Timur Bagautdinov, Junxuan Li, Vasu Agrawal, Fabian Prada, Shoou-I Yu, Pace Nalbone, Matt Gramlich, Roman Lubachersky, Chenglei Wu, Javier Romero, Jason Saragih, Michael Zollhoefer, Andreas Geiger, Siyu Tang, Shunsuke Saito
We propose Relightable Full-Body Gaussian Codec Avatars, a new approach for modeling relightable full-body avatars with fine-grained details including face and hands. The unique challenge for relighting full-body avatars lies in the large deformations caused by body articulation and the resulting impact on appearance caused by light transport. Changes in body pose can dramatically change the orientation of body surfaces with respect to lights, resulting in both local appearance changes due to changes in local light transport functions, as well as non-local changes due to occlusion between body parts. To address this, we decompose the light transport into local and non-local effects. Local appearance changes are modeled using learnable zonal harmonics for diffuse radiance transfer. Unlike spherical harmonics, zonal harmonics are highly efficient to rotate under articulation. This allows us to learn diffuse radiance transfer in a local coordinate frame, which disentangles the local radiance transfer from the articulation of the body. To account for non-local appearance changes, we introduce a shadow network that predicts shadows given precomputed incoming irradiance on a base mesh. This facilitates the learning of non-local shadowing between the body parts. Finally, we use a deferred shading approach to model specular radiance transfer and better capture reflections and highlights such as eye glints. We demonstrate that our approach successfully models both the local and non-local light transport required for relightable full-body avatars, with a superior generalization ability under novel illumination conditions and unseen poses.
我们提出了重光全身高斯编码化身(Relightable Full-Body Gaussian Codec Avatars)新方法,用于构建具有精细细节(包括面部和手部)的全身重光化身。为全身化身重新照明所面临的独特挑战在于身体关节活动产生的大变形以及由此导致的光照传输对外观的影响。身体姿态的改变会显著改变身体表面相对于光源的方向,从而导致局部光照传输功能的改变引起的局部外观变化,以及由于身体部位之间的遮挡引起的非局部变化。为了解决这个问题,我们将光照传输分解为局部和非局部效应。局部外观变化使用可学习的区域谐波对漫反射辐射传输进行建模。与球面谐波不同,区域谐波在关节活动时旋转非常高效。这使我们能够在局部坐标系中学习漫反射辐射传输,从而将局部辐射传输与身体的关节活动分开。为了解释非局部外观变化,我们引入了一个阴影网络,该网络根据基础网格上的预计算入射辐射来预测阴影。这有助于学习身体部位之间的非局部阴影。最后,我们使用延迟着色方法来模拟镜面辐射传输,以更好地捕捉反射和高光,例如眼睛闪烁。我们证明,我们的方法成功地模拟了全身重光化身所需的局部和非局部光照传输,并在新型照明条件和未见姿态下具有出色的泛化能力。
论文及项目相关链接
PDF 14 pages, 9 figures. Project page: https://neuralbodies.github.io/RFGCA
Summary
本文提出一种名为“Relightable Full-Body Gaussian Codec Avatars”的新方法,用于创建具有精细细节的可重新照明的全身虚拟角色,包括面部和手部。该方法通过分解光照传输为局部和非局部效应来解决全身虚拟角色的重新照明挑战。局部外观变化采用可学习的区域谐波来模拟漫反射传输;非局部外观变化则通过阴影网络预测基于基础网格的预计算入射辐射来实现。最后,使用延迟着色方法来模拟镜面反射并更好地捕捉反射和高光。此方法成功模拟了可重新照明的全身虚拟角色的局部和非局部光照传输,并在新型照明条件和未见姿态下具有出色的泛化能力。
Key Takeaways
- 提出了一种新的全身虚拟角色建模方法——“Relightable Full-Body Gaussian Codec Avatars”。
- 解决了全身虚拟角色重新照明中的大变形和光照传输影响问题。
- 通过分解光照传输为局部和非局部效应,处理了局部和非局部外观变化。
- 采用可学习的区域谐波模拟漫反射传输,效率高于球形谐波。
- 引入阴影网络预测基础网格上的预计算入射辐射,以处理非局部阴影变化。
- 使用延迟着色方法模拟镜面反射,更好地捕捉反射和高光。
点此查看论文截图
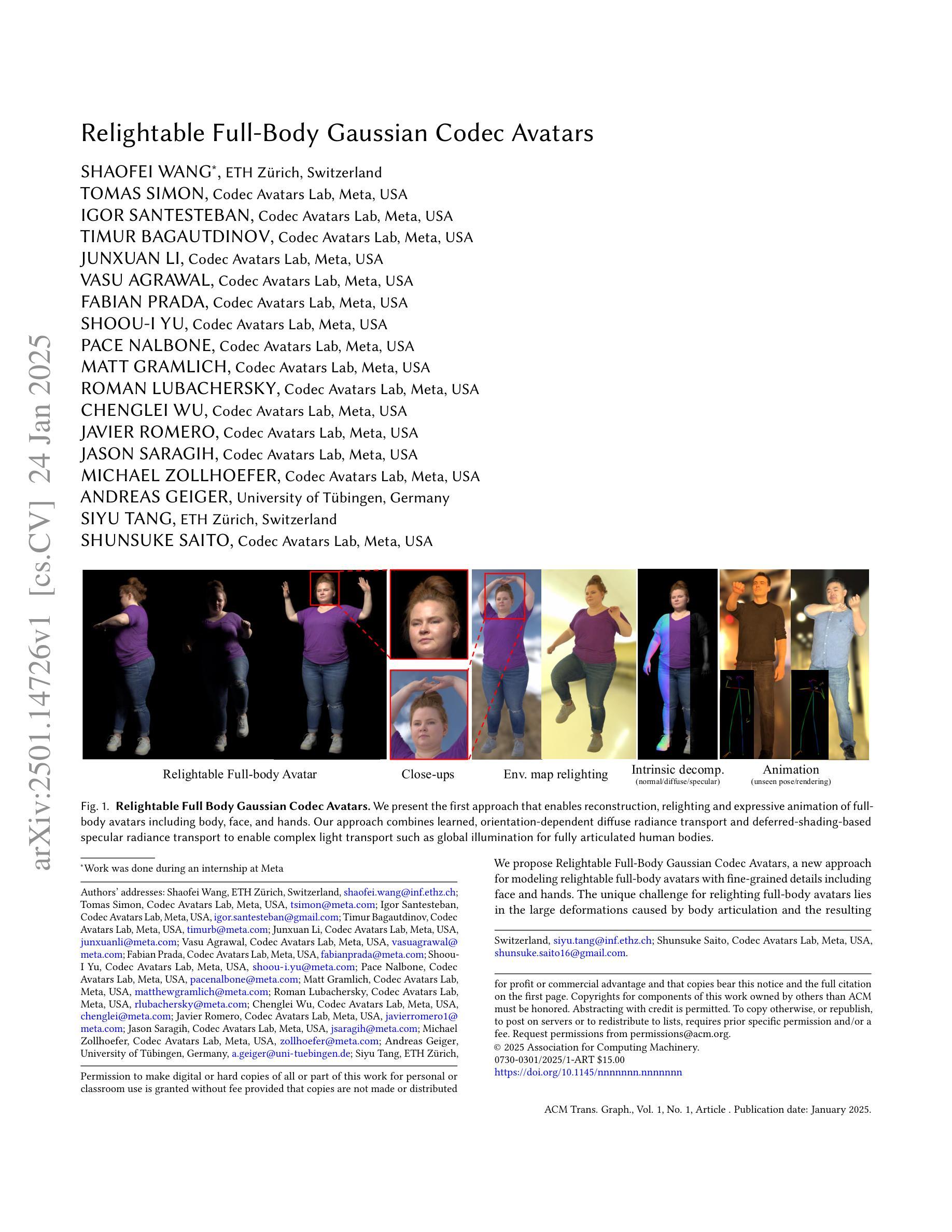
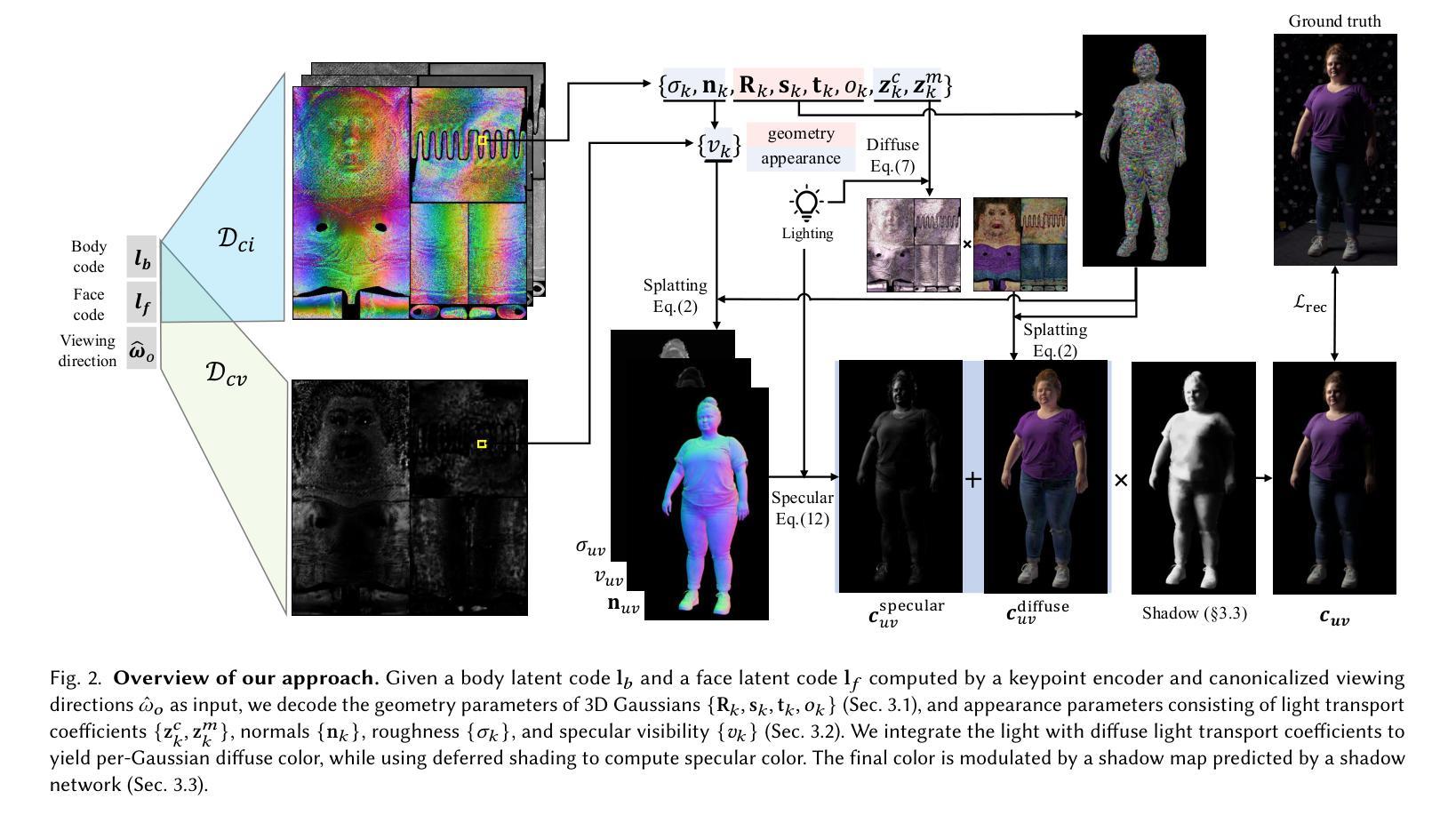