⚠️ 以下所有内容总结都来自于 大语言模型的能力,如有错误,仅供参考,谨慎使用
🔴 请注意:千万不要用于严肃的学术场景,只能用于论文阅读前的初筛!
💗 如果您觉得我们的项目对您有帮助 ChatPaperFree ,还请您给我们一些鼓励!⭐️ HuggingFace免费体验
2025-01-28 更新
Training-Free Style and Content Transfer by Leveraging U-Net Skip Connections in Stable Diffusion 2.*
Authors:Ludovica Schaerf, Andrea Alfarano, Fabrizio Silvestri, Leonardo Impett
Despite significant recent advances in image generation with diffusion models, their internal latent representations remain poorly understood. Existing works focus on the bottleneck layer (h-space) of Stable Diffusion’s U-Net or leverage the cross-attention, self-attention, or decoding layers. Our model, SkipInject takes advantage of U-Net’s skip connections. We conduct thorough analyses on the role of the skip connections and find that the residual connections passed by the third encoder block carry most of the spatial information of the reconstructed image, splitting the content from the style. We show that injecting the representations from this block can be used for text-based editing, precise modifications, and style transfer. We compare our methods state-of-the-art style transfer and image editing methods and demonstrate that our method obtains the best content alignment and optimal structural preservation tradeoff.
尽管扩散模型在图像生成方面取得了重大进展,但其内部潜在表示仍然鲜为人知。现有工作主要关注Stable Diffusion的U-Net的瓶颈层(h空间),或利用交叉注意力、自注意力或解码层。我们的模型SkipInject利用U-Net的跳跃连接。我们对跳跃连接的作用进行了深入分析,发现通过第三个编码器块的残差连接携带了重建图像的大部分空间信息,将内容和风格分开。我们展示了从这个块注入表示可以用于基于文本编辑、精确修改和风格转换。我们将我们的方法与最先进的风格转换和图像编辑方法进行了比较,并证明我们的方法在内容对齐和结构保持方面取得了最佳的权衡。
论文及项目相关链接
Summary
本文探讨了扩散模型在图像生成中的最新进展,并指出尽管已有显著进步,但对扩散模型内部潜在表示的理解仍然有限。本文提出了一种新的模型SkipInject,它利用U-Net的跳跃连接进行图像编辑和风格转换。通过深入分析跳跃连接的作用,发现第三个编码器块传递的残差连接包含了重建图像的大部分空间信息,能将内容和风格分开。注入此块的表示可用于基于文本编辑、精确修改和风格转换。与其他先进的风格转换和图像编辑方法相比,本文提出的方法在内容对齐和最佳结构保留方面表现出优势。
Key Takeaways
- 扩散模型在图像生成领域取得了显著进展,但对内部潜在表示的理解仍然有限。
- SkipInject模型利用U-Net的跳跃连接进行图像编辑和风格转换。
- 第三个编码器块的残差连接包含重建图像的大部分空间信息。
- 跳跃连接能将图像的内容和风格分开。
- 注入特定块的表示可用于基于文本的编辑、精确修改和风格转换。
- 与其他方法相比,SkipInject在内容对齐方面表现出优势。
点此查看论文截图
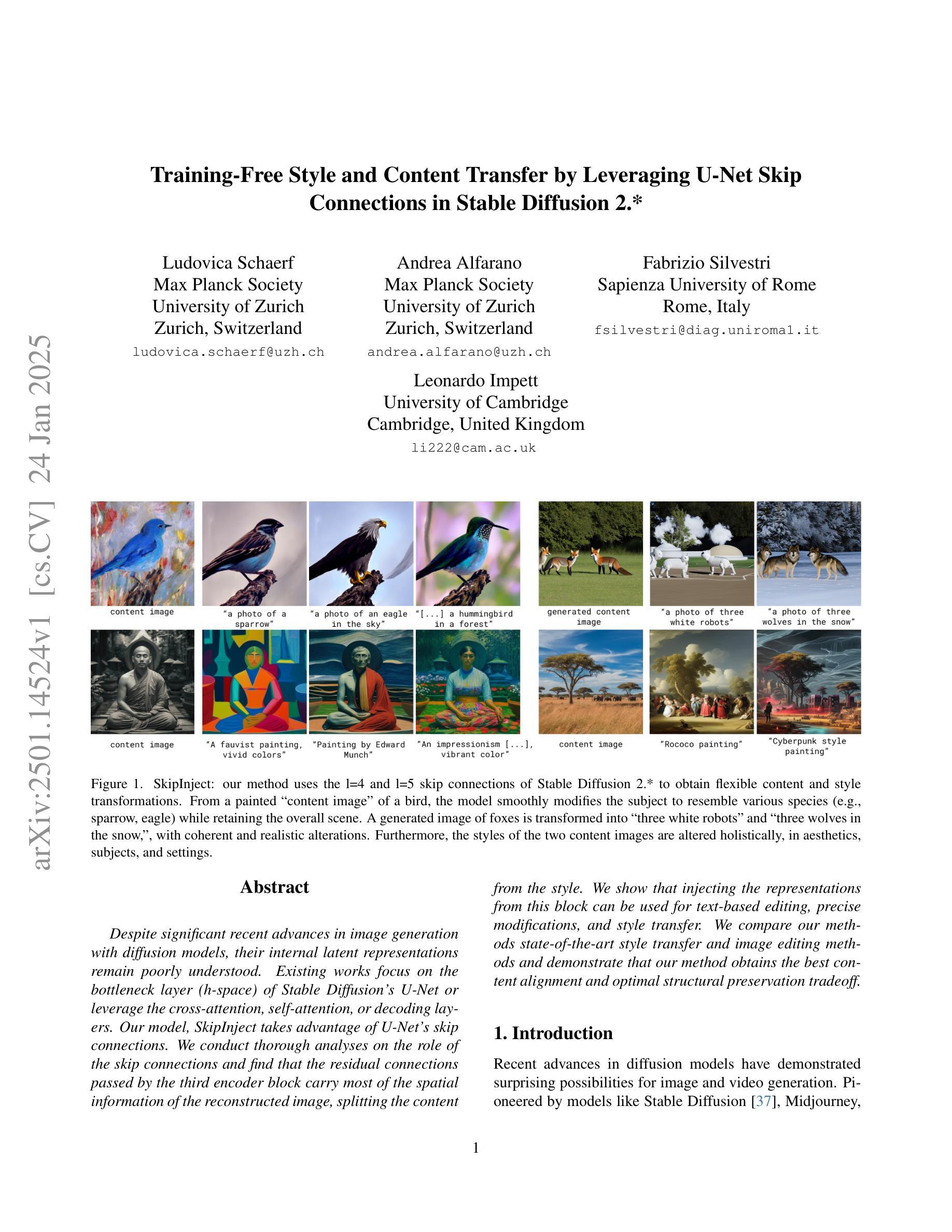
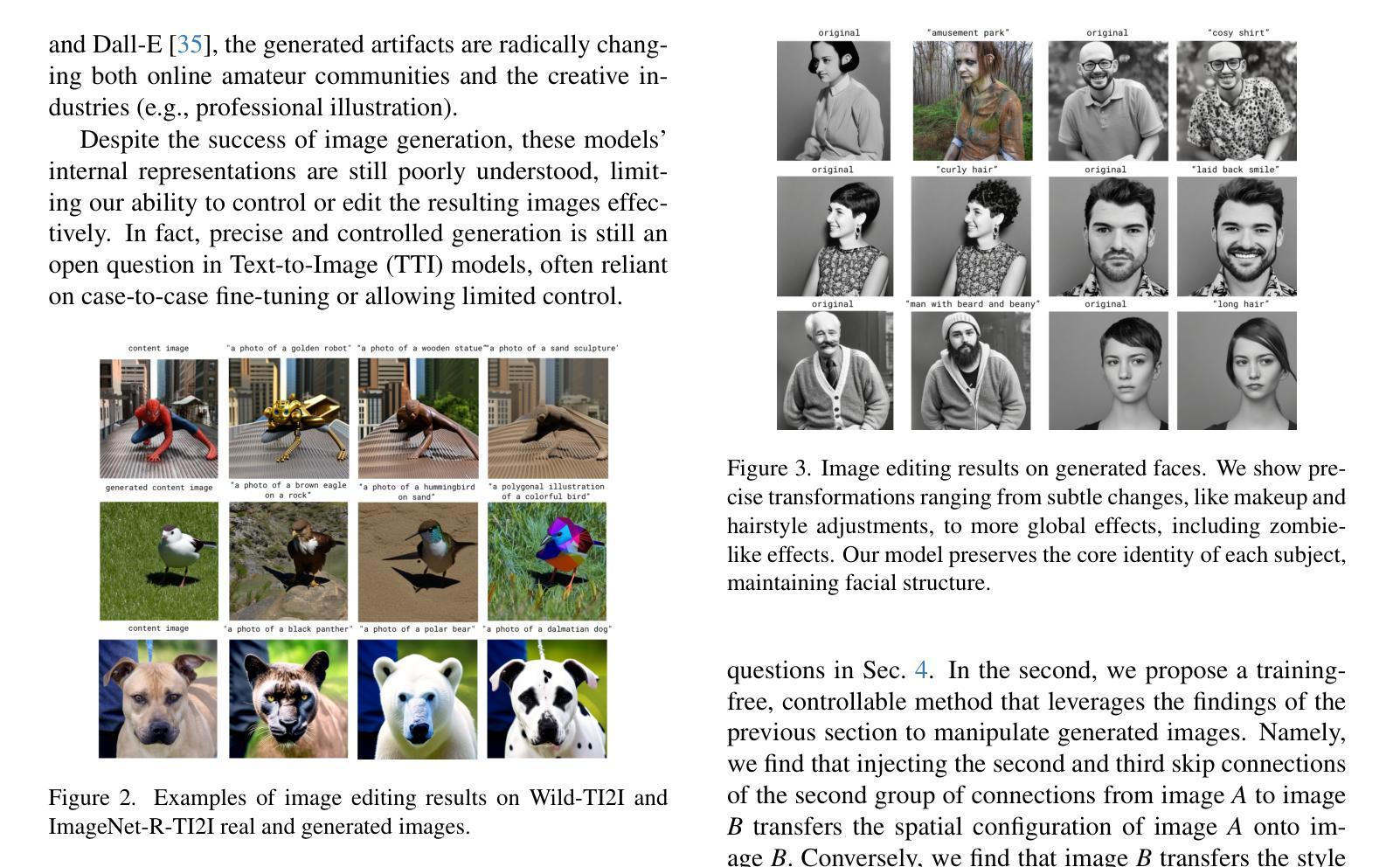
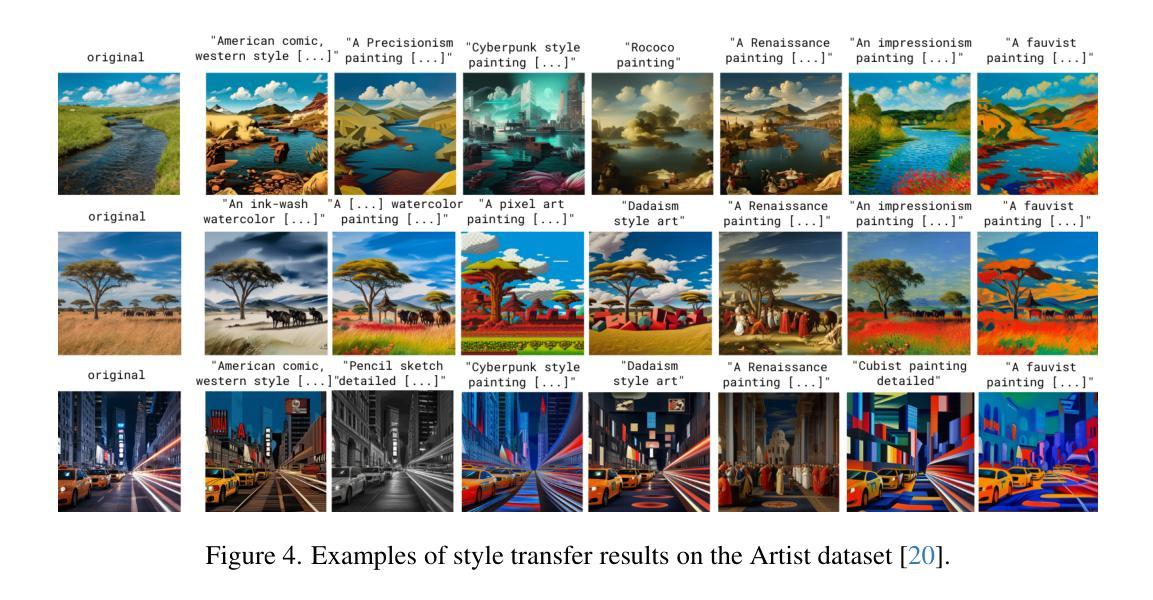
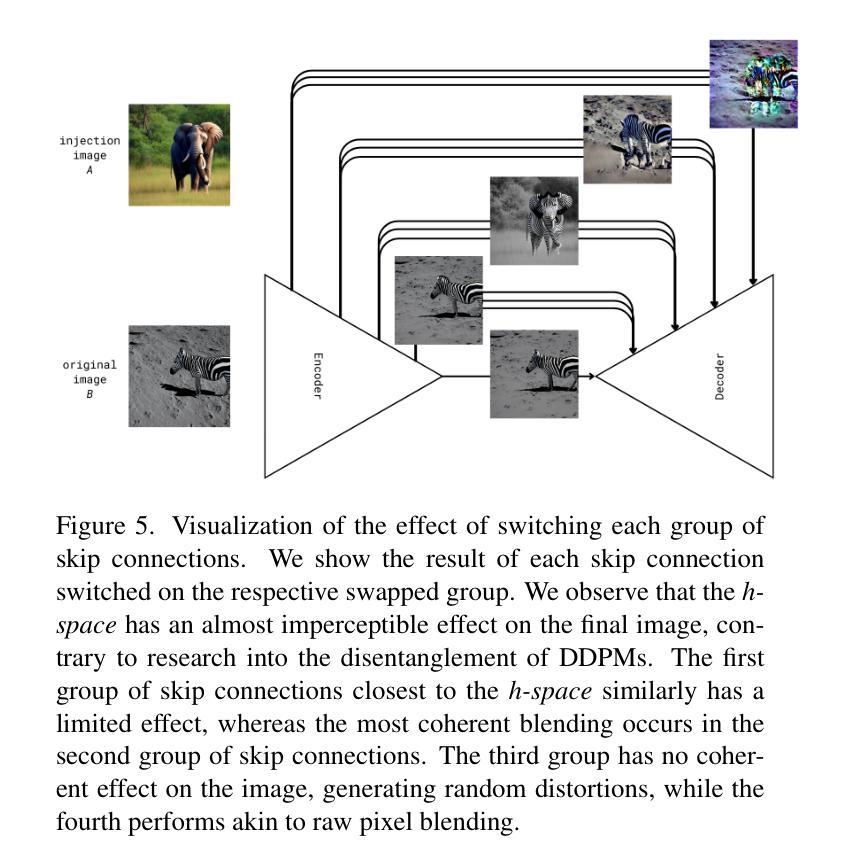
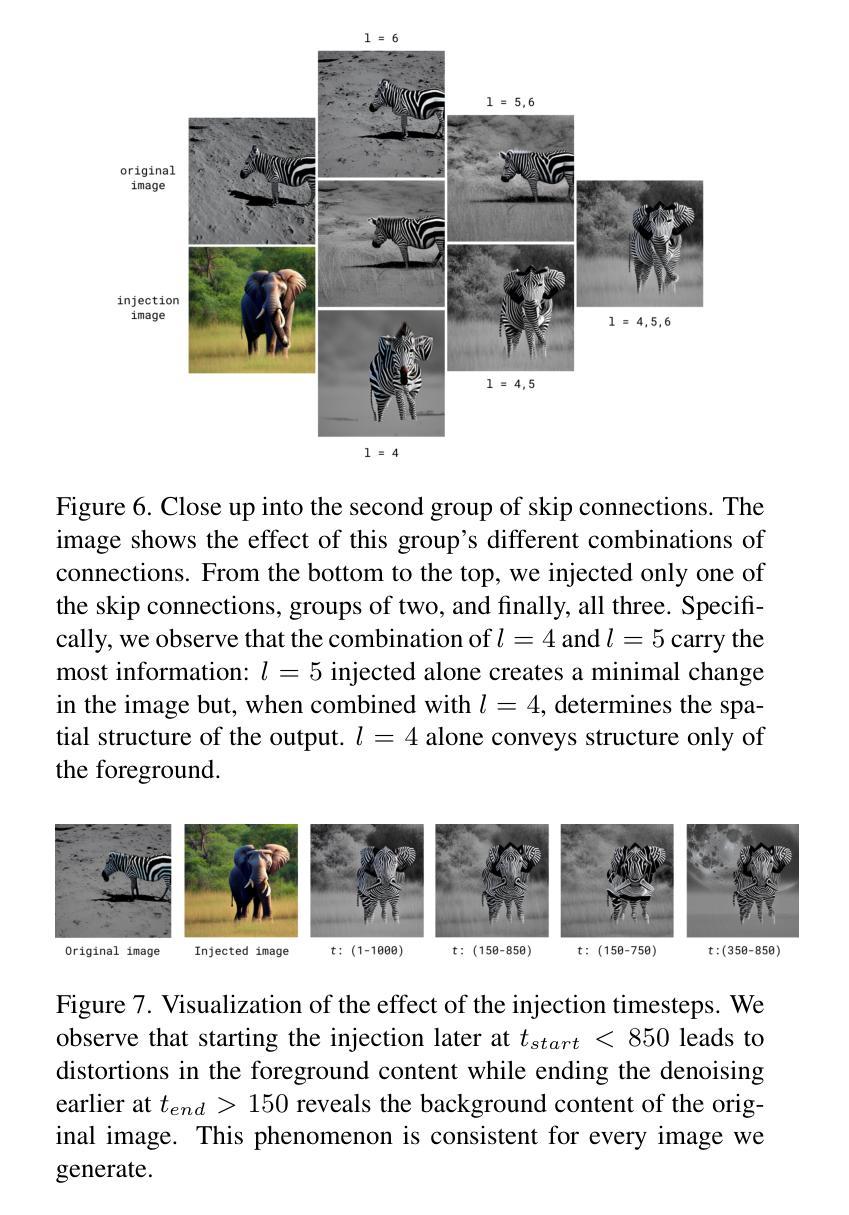
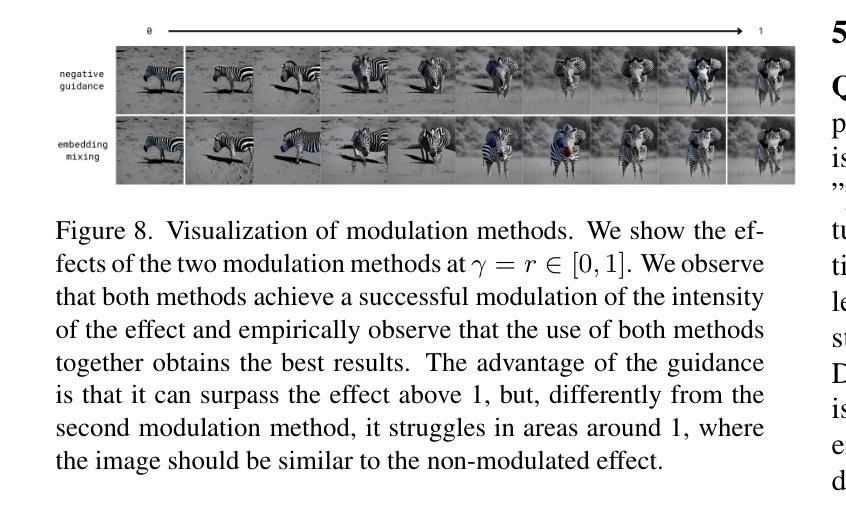
One-Prompt-One-Story: Free-Lunch Consistent Text-to-Image Generation Using a Single Prompt
Authors:Tao Liu, Kai Wang, Senmao Li, Joost van de Weijer, Fahad Shahbaz Khan, Shiqi Yang, Yaxing Wang, Jian Yang, Ming-Ming Cheng
Text-to-image generation models can create high-quality images from input prompts. However, they struggle to support the consistent generation of identity-preserving requirements for storytelling. Existing approaches to this problem typically require extensive training in large datasets or additional modifications to the original model architectures. This limits their applicability across different domains and diverse diffusion model configurations. In this paper, we first observe the inherent capability of language models, coined context consistency, to comprehend identity through context with a single prompt. Drawing inspiration from the inherent context consistency, we propose a novel training-free method for consistent text-to-image (T2I) generation, termed “One-Prompt-One-Story” (1Prompt1Story). Our approach 1Prompt1Story concatenates all prompts into a single input for T2I diffusion models, initially preserving character identities. We then refine the generation process using two novel techniques: Singular-Value Reweighting and Identity-Preserving Cross-Attention, ensuring better alignment with the input description for each frame. In our experiments, we compare our method against various existing consistent T2I generation approaches to demonstrate its effectiveness through quantitative metrics and qualitative assessments. Code is available at https://github.com/byliutao/1Prompt1Story.
文本到图像生成模型可以从输入提示中创建高质量的图像。然而,它们在支持故事叙述中的身份一致性要求的一致生成方面存在困难。现有解决此问题的方法通常需要在大型数据集上进行广泛训练或对原始模型架构进行额外修改。这限制了它们在不同领域和多样化扩散模型配置中的应用性。在本文中,我们首先观察到语言模型的固有能力,称为上下文一致性,即通过一个单一提示理解身份的能力。从固有的上下文一致性中汲取灵感,我们提出了一种新型的无需训练即可实现一致文本到图像(T2I)生成的方法,称为“One-Prompt-One-Story”(1Prompt1Story)。我们的1Prompt1Story方法将所有提示合并为一个单一输入,用于T2I扩散模型,最初保留角色身份。然后,我们使用两种新颖的技术来完善生成过程:奇异值重加权和身份保留交叉注意力,确保与每个帧的输入描述更好地对齐。在我们的实验中,我们将我们的方法与各种现有的一致T2I生成方法进行比较,通过定量指标和定性评估来证明其有效性。代码可在https://github.com/byliutao/1Prompt1Story找到。
论文及项目相关链接
PDF 28 pages, 22 figures, ICLR2025 conference
Summary
文本到图像生成模型能够从输入提示创建高质量图像。然而,它们在支持故事叙述的身份一致性生成方面存在挑战。现有解决此问题的方法通常需要在大型数据集上进行广泛训练或对原始模型架构进行额外修改,这限制了它们在不同领域和多样化扩散模型配置中的应用性。本文首先观察到语言模型的内在能力,称为上下文一致性,能够通过单个提示理解身份。受内在上下文一致性的启发,我们提出了一种新颖的无需训练的一致文本到图像(T2I)生成方法,称为“One-Prompt-One-Story”(1Prompt1Story)。我们的方法将所有提示合并为一个单一输入,最初保留角色身份。然后,我们使用两种新技术完善生成过程:奇异值重加权和身份保留交叉注意力,确保与每个帧的输入描述更好对齐。
Key Takeaways
- 文本到图像生成模型能够创建高质量图像,但在支持故事叙述的身份一致性生成方面存在挑战。
- 现有解决此问题的方法具有广泛的应用限制,需要大规模训练和模型结构修改。
- 语言模型具有通过单个提示理解身份的内在能力,称为上下文一致性。
- 提出了新颖的无需训练的一致文本到图像(T2I)生成方法——“One-Prompt-One-Story”(1Prompt1Story)。
- 1Prompt1Story方法将所有提示合并为一个单一输入,以保留角色身份为核心。
- 使用了奇异值重加权和身份保留交叉注意力两种新技术,以提高生成图像与输入描述的对齐度。
点此查看论文截图
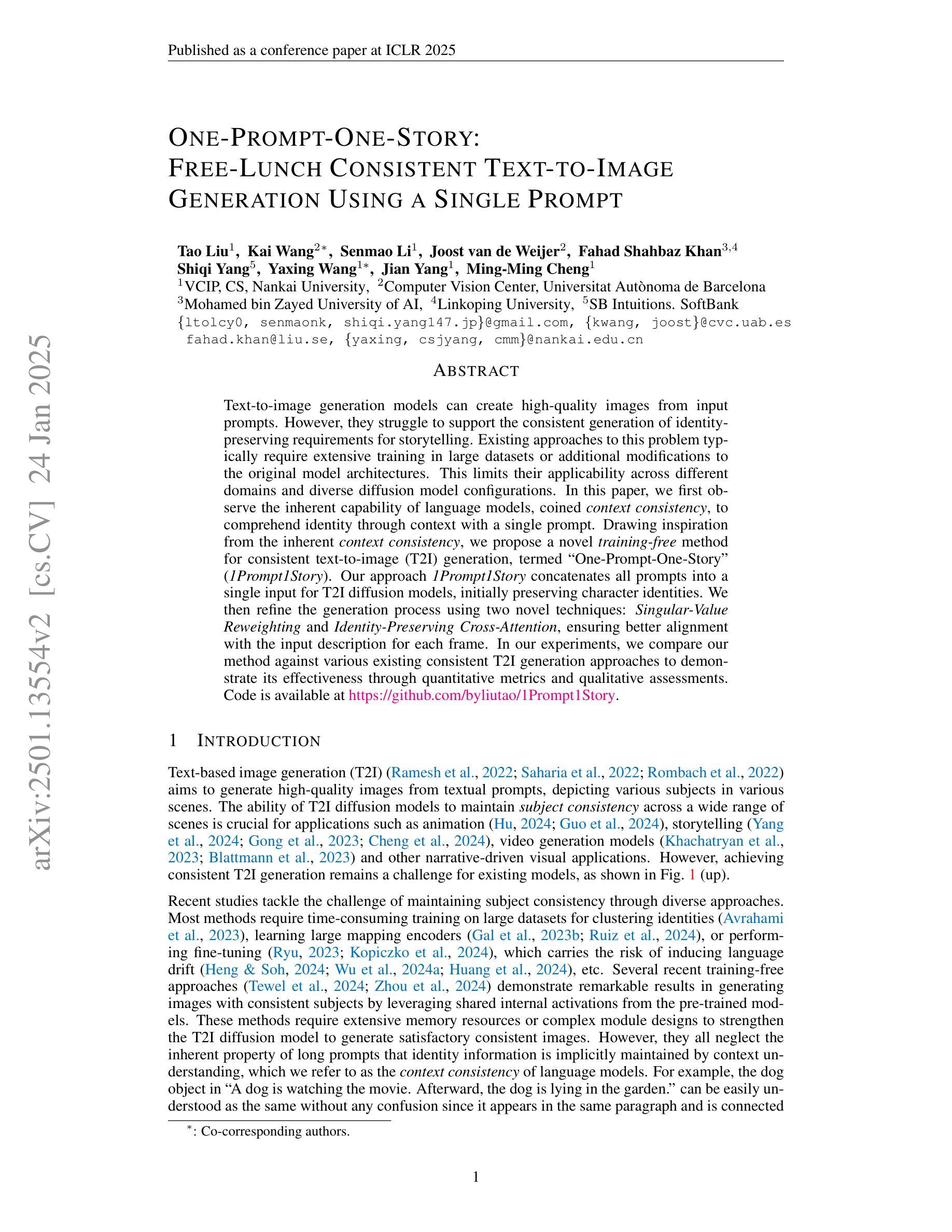
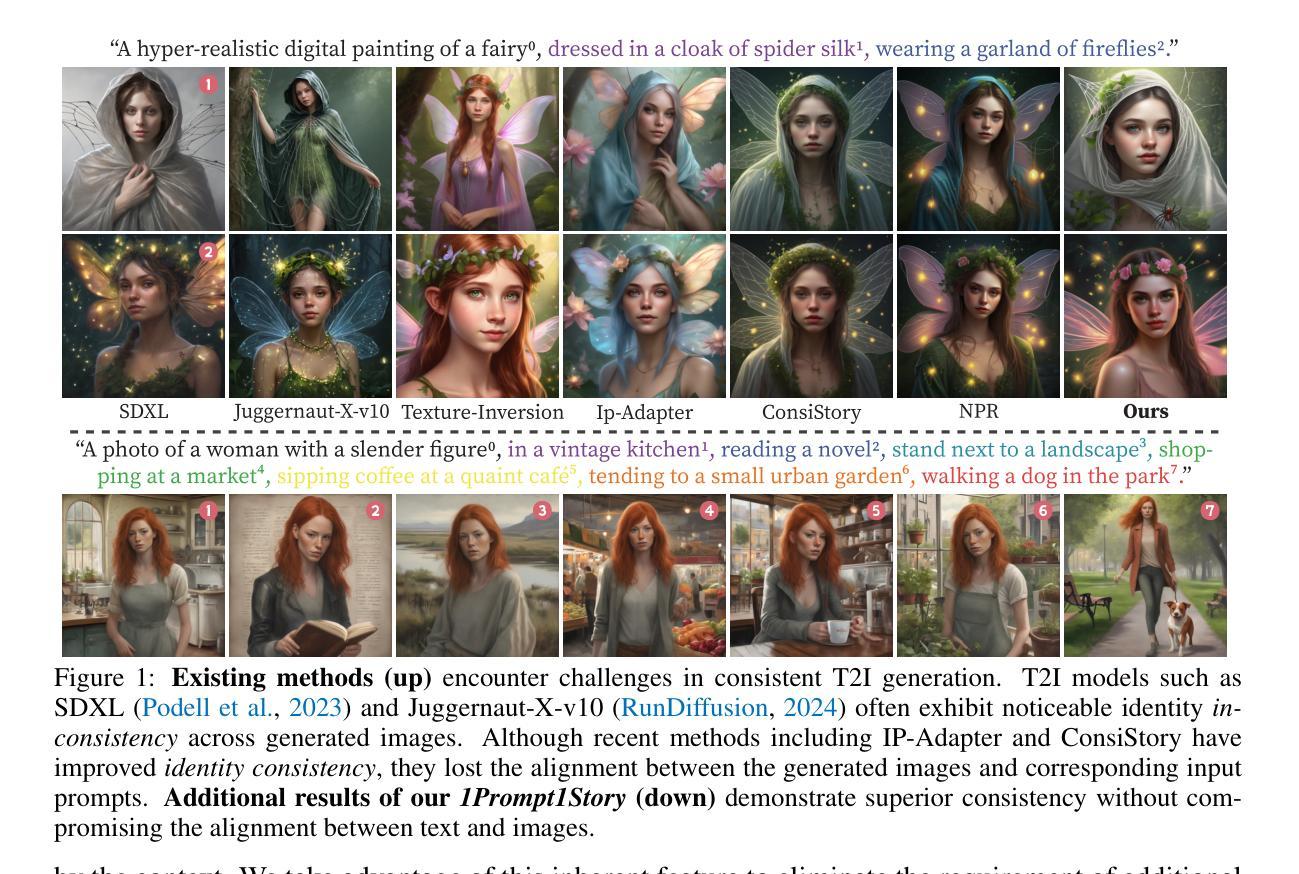
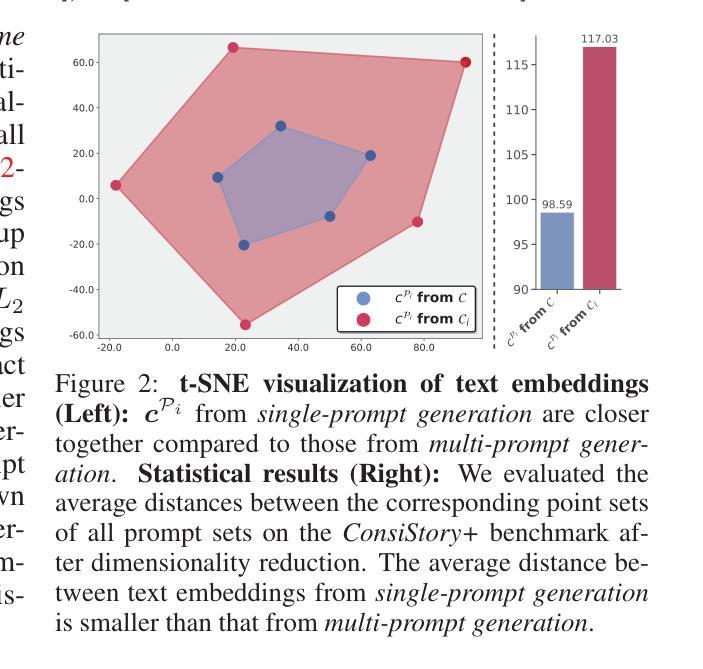
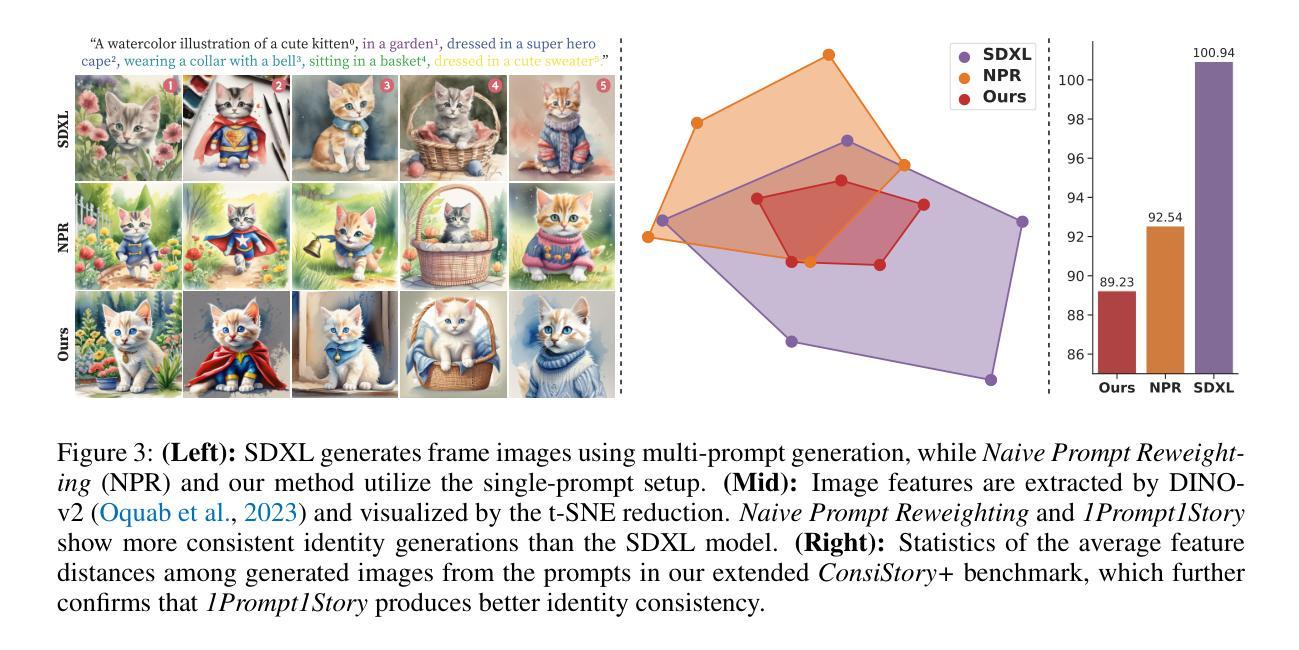
Solving Blind Inverse Problems: Adaptive Diffusion Models for Motion-corrected Sparse-view 4DCT
Authors:Antoine De Paepe, Alexandre Bousse, Clémentine Phung-Ngoc, Dimitris Visvikis
Four-dimensional computed tomography (4DCT) is essential for medical imaging applications like radiotherapy, which demand precise respiratory motion representation. Traditional methods for reconstructing 4DCT data suffer from artifacts and noise, especially in sparse-view, low-dose contexts. Motion-corrected (MC) reconstruction is a blind inverse problem that we propose to solve with a novel diffusion model (DM) framework that calibrates an adaptive unknown forward model for motion correction. Furthermore, we used a wavelet diffusion model (WDM) to address computational cost and memory usage. By leveraging the prior probability distribution function (PDF) from the DMs, we enhance the joint reconstruction and motion estimation (JRM) process, improving image quality and preserving resolution. Experiments on extended cardiac-torso (XCAT) phantom data demonstrate that our method outperforms existing techniques, yielding artifact-free, high-resolution reconstructions even under irregular breathing conditions. These results showcase the potential of combining DMs with motion correction to advance sparse-view 4DCT imaging.
四维计算机断层扫描(4DCT)在放射治疗等医学成像应用中至关重要,这些应用需要精确表示呼吸运动。传统的重建4DCT数据的方法在稀疏视角、低剂量情况下会出现伪影和噪声。运动校正(MC)重建是一个盲反问题,我们提出通过一种新的扩散模型(DM)框架来解决这个问题,该框架校准了用于运动校正的自适应未知前向模型。此外,我们使用小波扩散模型(WDM)来解决计算成本和内存使用问题。通过利用扩散模型的先验概率分布函数(PDF),我们增强了联合重建和运动估计(JRM)过程,提高了图像质量并保持分辨率。在扩展心脏体模(XCAT)幻影数据上的实验表明,我们的方法优于现有技术,即使在不规则呼吸条件下也能产生无伪影、高分辨率的重建结果。这些结果展示了将扩散模型与运动校正相结合在稀疏视角4DCT成像中的潜力。
论文及项目相关链接
PDF 4 pages, 2 figures, 1 table
Summary
本文介绍了四维计算机断层扫描(4DCT)在医疗成像应用中的重要性,特别是在放射治疗领域。针对传统重建方法在处理稀疏视角和低剂量环境下的数据时出现的伪影和噪声问题,提出了一种基于扩散模型(DM)的校正自适应未知前向模型的运动校正(MC)重建方法。通过利用扩散模型的先验概率分布函数(PDF),优化了联合重建和运动估计(JRM)过程,提高了图像质量和分辨率。在XCAT Phantom数据上的实验表明,该方法优于现有技术,即使在不规则呼吸条件下也能实现无伪影、高分辨率的重建,展示了扩散模型与运动校正相结合在稀疏视角4DCT成像中的潜力。
Key Takeaways
- 四维计算机断层扫描(4DCT)在医疗成像中至关重要,尤其在需要精确表示呼吸运动的放射治疗领域。
- 传统重建方法在稀疏视角和低剂量环境下处理数据时存在伪影和噪声问题。
- 提出了一种基于扩散模型(DM)的新方法来解决运动校正(MC)重建中的盲逆问题。
- 利用扩散模型的先验概率分布函数(PDF)优化联合重建和运动估计(JRM)过程。
- 实验证明,该方法在XCAT Phantom数据上的表现优于现有技术。
- 该方法能够实现无伪艺、高分辨率的重建,即使在不规则的呼吸条件下。
点此查看论文截图
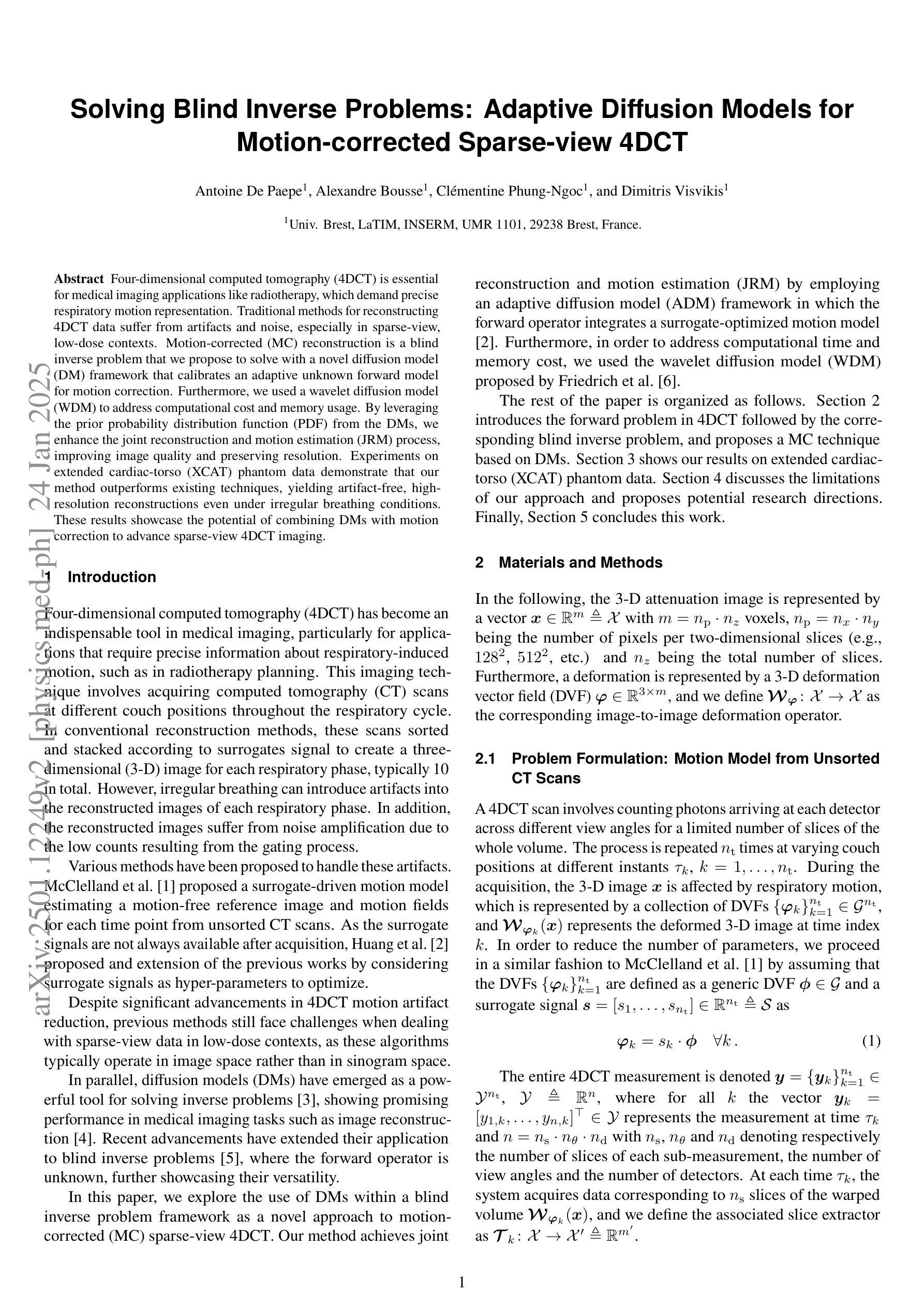
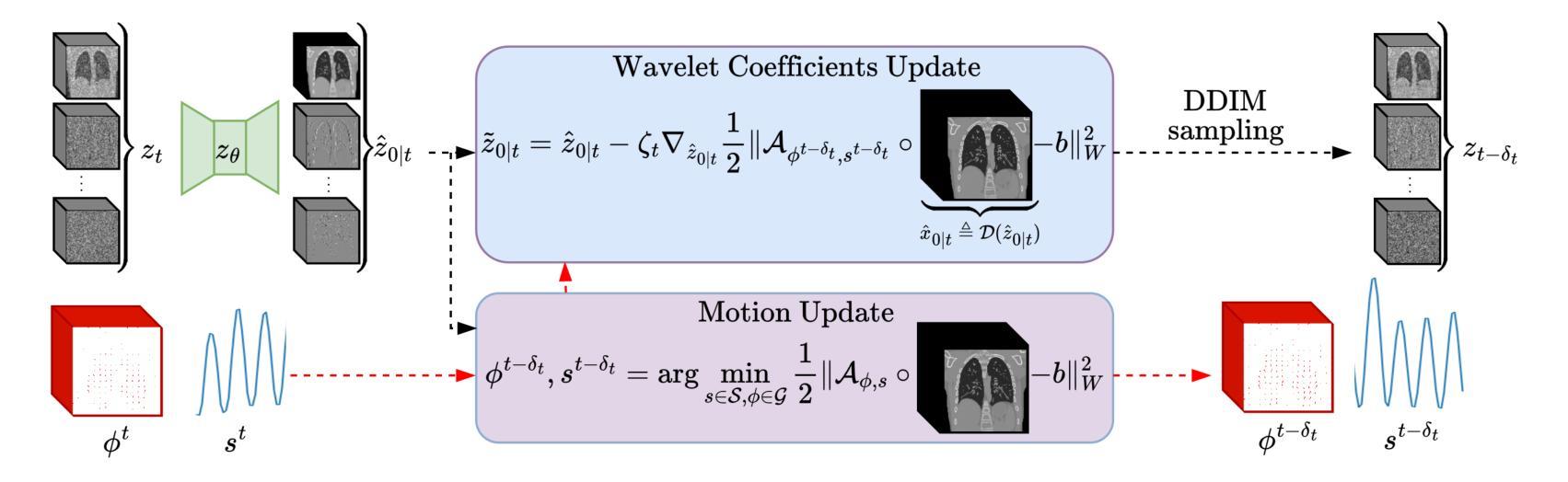
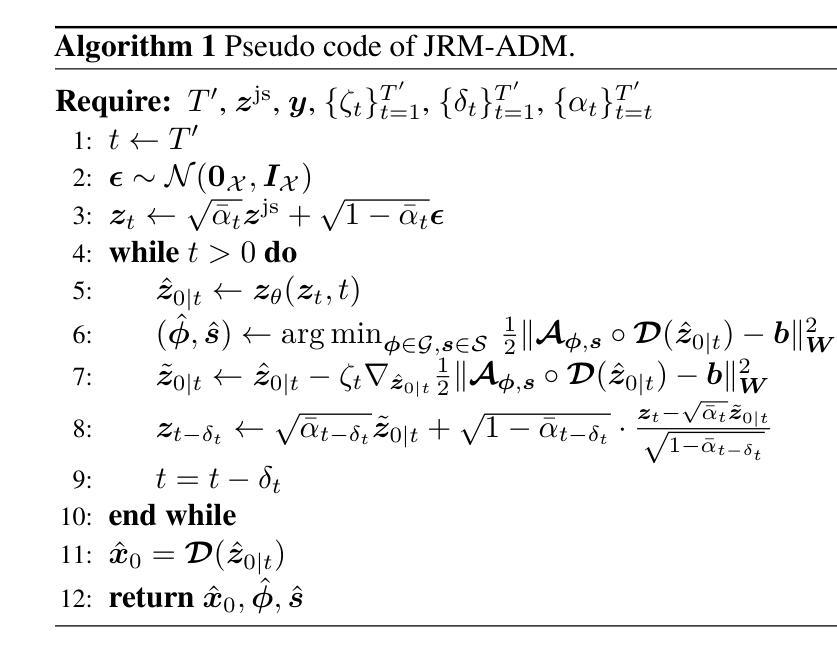
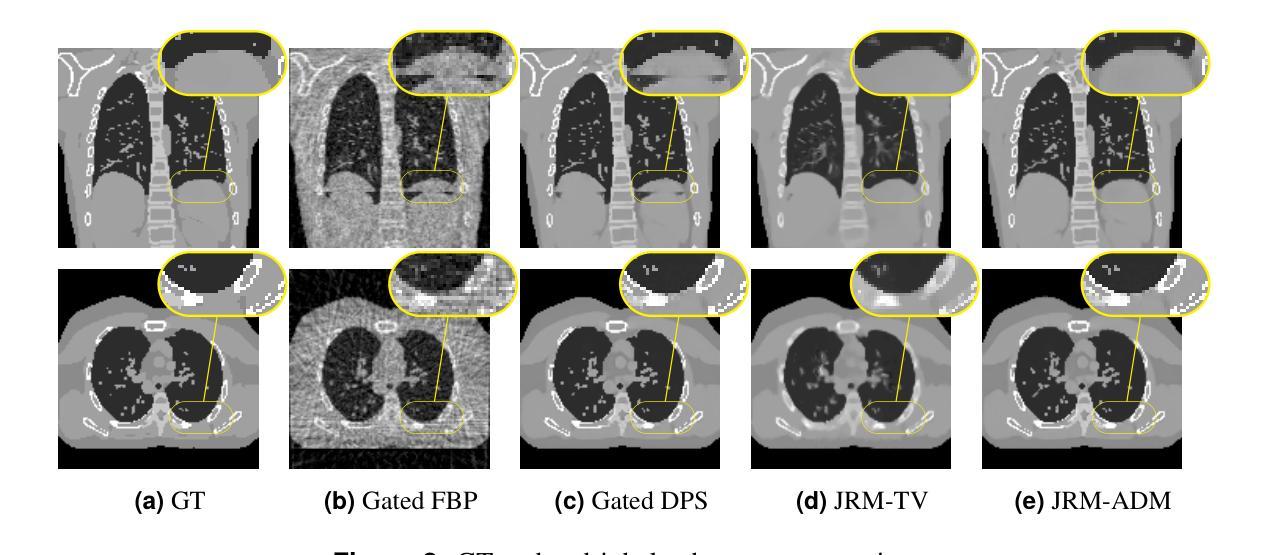
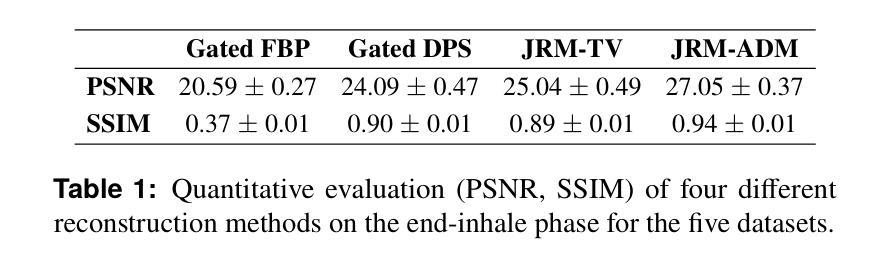
A Survey on Diffusion Models for Anomaly Detection
Authors:Jing Liu, Zhenchao Ma, Zepu Wang, Yang Liu, Zehua Wang, Peng Sun, Liang Song, Bo Hu, Azzedine Boukerche, Victor C. M. Leung
Diffusion models (DMs) have emerged as a powerful class of generative AI models, showing remarkable potential in anomaly detection (AD) tasks across various domains, such as cybersecurity, fraud detection, healthcare, and manufacturing. The intersection of these two fields, termed diffusion models for anomaly detection (DMAD), offers promising solutions for identifying deviations in increasingly complex and high-dimensional data. In this survey, we review recent advances in DMAD research. We begin by presenting the fundamental concepts of AD and DMs, followed by a comprehensive analysis of classic DM architectures including DDPMs, DDIMs, and Score SDEs. We further categorize existing DMAD methods into reconstruction-based, density-based, and hybrid approaches, providing detailed examinations of their methodological innovations. We also explore the diverse tasks across different data modalities, encompassing image, time series, video, and multimodal data analysis. Furthermore, we discuss critical challenges and emerging research directions, including computational efficiency, model interpretability, robustness enhancement, edge-cloud collaboration, and integration with large language models. The collection of DMAD research papers and resources is available at https://github.com/fdjingliu/DMAD.
扩散模型(DMs)作为一类强大的生成人工智能模型已经崭露头角,在异常检测(AD)任务中显示出显著潜力,广泛应用于网络安全、欺诈检测、医疗保健和制造等多个领域。这两个领域的交集,被称为用于异常检测的扩散模型(DMAD),为识别日益复杂和高维数据中的偏差提供了有前景的解决方案。在这篇综述中,我们回顾了DMAD研究的最新进展。首先介绍AD和DM的基本概念,然后全面分析经典的DM架构,包括DDPMs、DDIMS和Score SDEs。我们进一步将现有的DMAD方法分为基于重建的、基于密度的和混合方法,并对其方法创新进行详细检查。我们还探讨了不同数据模态的多样化任务,包括图像、时间序列、视频和多模态数据分析。此外,我们还讨论了关键的挑战和新兴的研究方向,包括计算效率、模型可解释性、增强稳健性、边缘云协作以及与大型语言模型的集成。DMAD研究论文和资源集可在https://github.com/fdjingliu/DMAD找到。
论文及项目相关链接
Summary
扩散模型(DMs)作为生成式人工智能模型的一种强大类别,在异常检测(AD)任务中展现出巨大的潜力,被广泛应用于网络安全、欺诈检测、医疗和制造等多个领域。本文综述了扩散模型在异常检测方面的最新研究进展,介绍了异常检测和扩散模型的基本概念,分析了经典的扩散模型架构,如DDPMs、DDIMs和Score SDEs。此外,本文还根据重建、密度和混合方法分类现有的DMAD方法,并对其方法论创新进行了详细的考察。文章还探讨了不同数据模式(如图像、时间序列、视频和多模态数据分析)下的各种任务,以及计算效率、模型可解释性、鲁棒性增强、边缘云协作和大型语言模型的集成等关键挑战和新兴研究方向。相关资源可访问:链接地址。
Key Takeaways
- 扩散模型(DMs)在异常检测(AD)领域具有广泛的应用潜力,尤其在网络安全、欺诈检测等领域。
- 文章综述了异常检测和扩散模型的基本概念。
- 经典的扩散模型架构包括DDPMs、DDIMs和Score SDEs。
- 现有的DMAD方法主要分为重建、密度和混合方法。
- 扩散模型可应用于多种数据模式,包括图像、时间序列、视频和多模态数据分析。
- 计算效率、模型可解释性和鲁棒性增强是当前面临的关键挑战。
点此查看论文截图
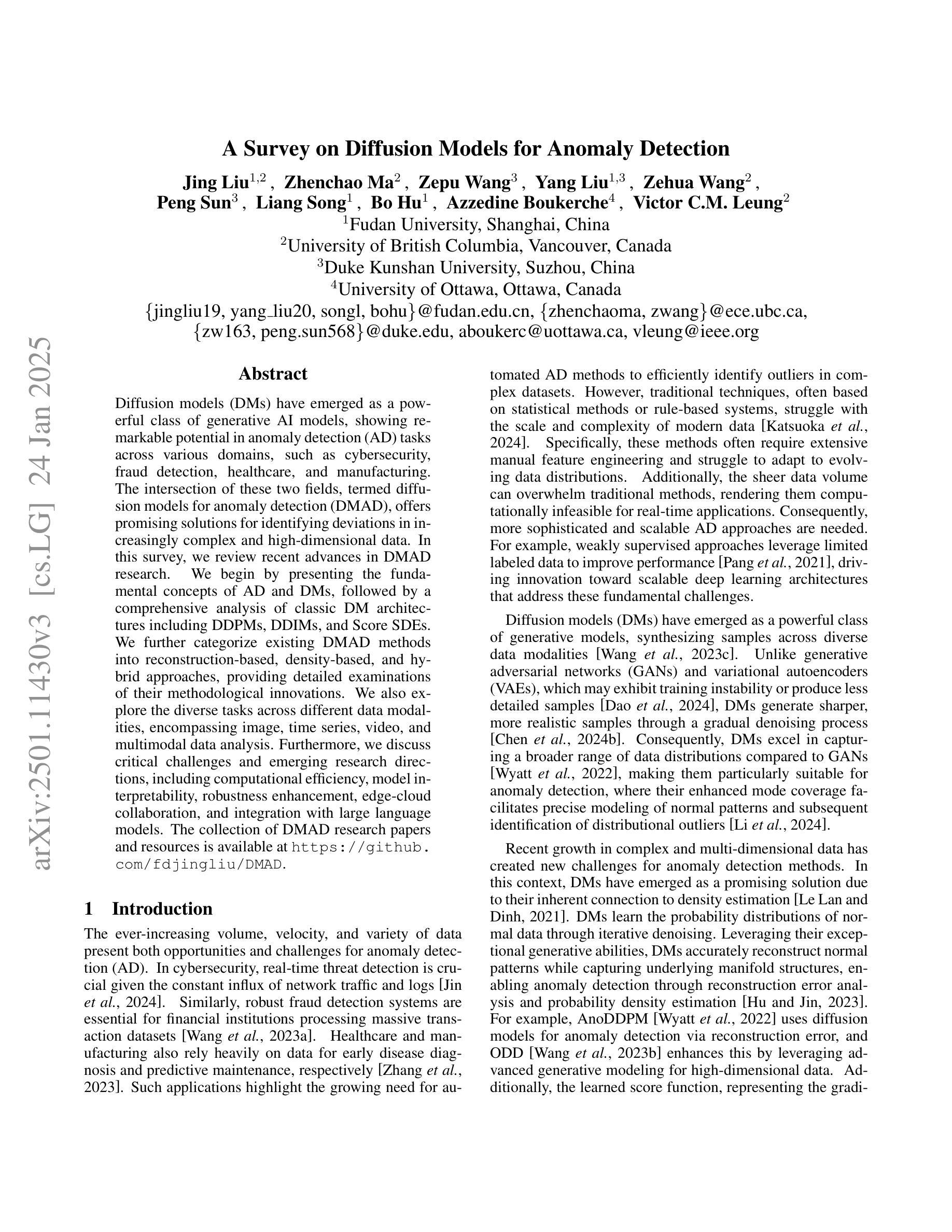
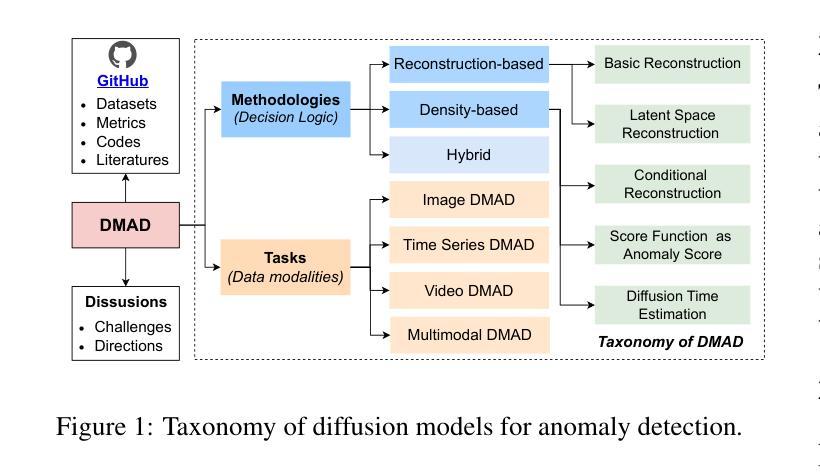
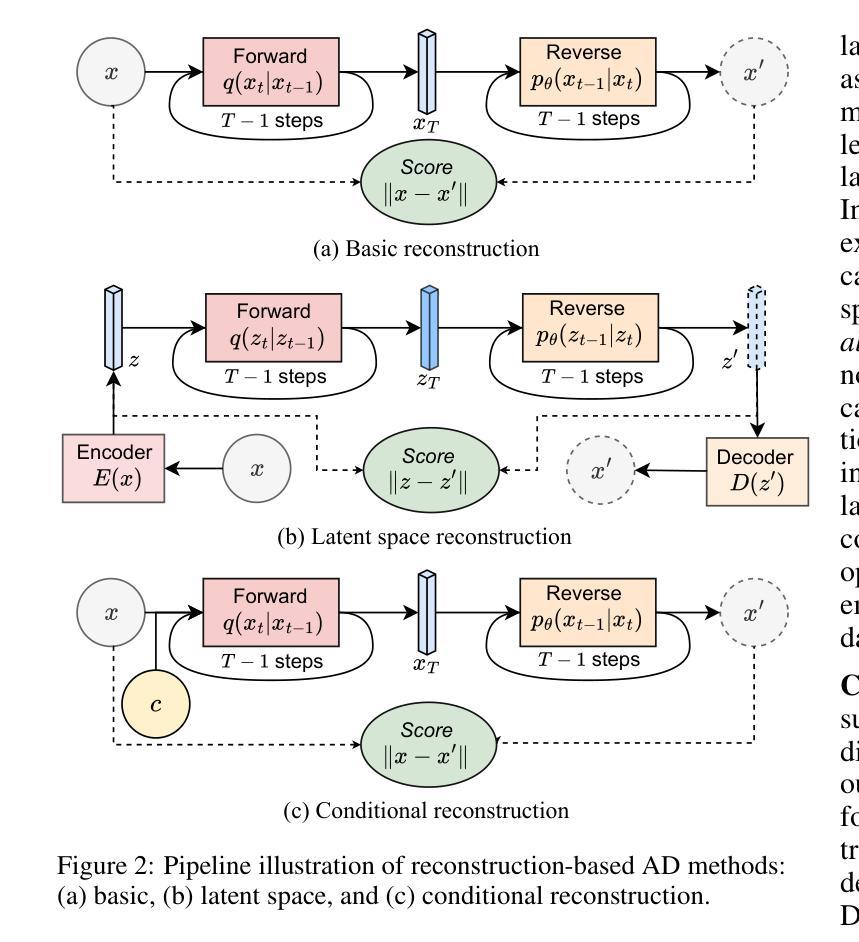
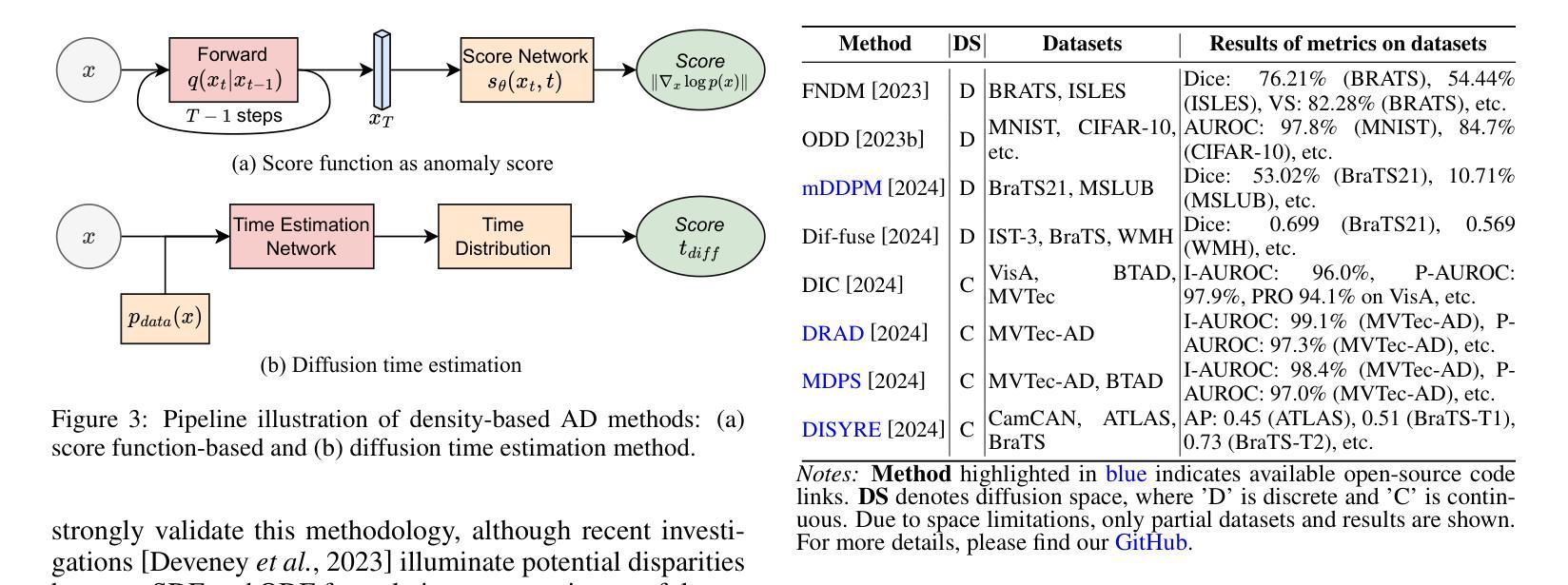
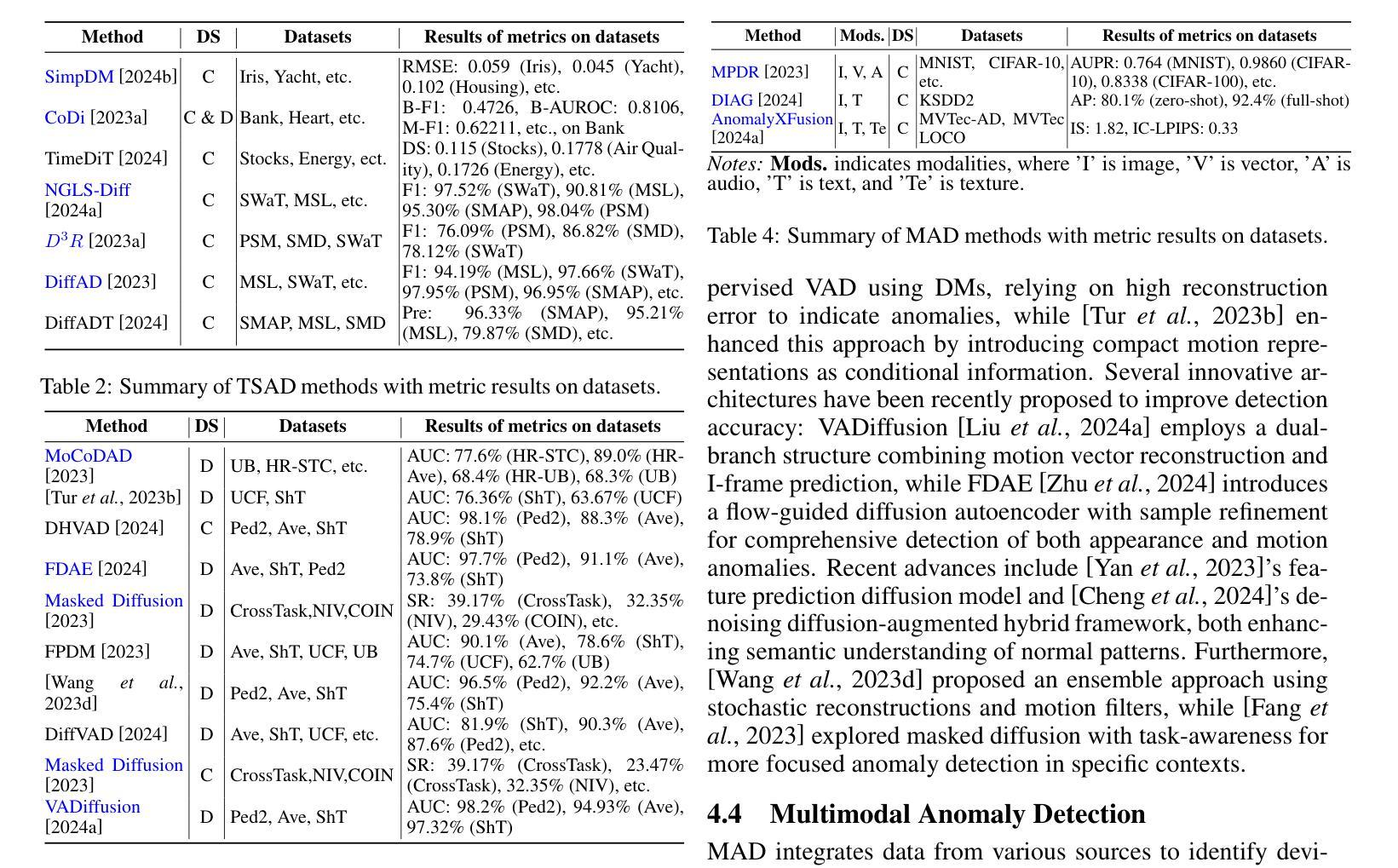
ControlAR: Controllable Image Generation with Autoregressive Models
Authors:Zongming Li, Tianheng Cheng, Shoufa Chen, Peize Sun, Haocheng Shen, Longjin Ran, Xiaoxin Chen, Wenyu Liu, Xinggang Wang
Autoregressive (AR) models have reformulated image generation as next-token prediction, demonstrating remarkable potential and emerging as strong competitors to diffusion models. However, control-to-image generation, akin to ControlNet, remains largely unexplored within AR models. Although a natural approach, inspired by advancements in Large Language Models, is to tokenize control images into tokens and prefill them into the autoregressive model before decoding image tokens, it still falls short in generation quality compared to ControlNet and suffers from inefficiency. To this end, we introduce ControlAR, an efficient and effective framework for integrating spatial controls into autoregressive image generation models. Firstly, we explore control encoding for AR models and propose a lightweight control encoder to transform spatial inputs (e.g., canny edges or depth maps) into control tokens. Then ControlAR exploits the conditional decoding method to generate the next image token conditioned on the per-token fusion between control and image tokens, similar to positional encodings. Compared to prefilling tokens, using conditional decoding significantly strengthens the control capability of AR models but also maintains the model’s efficiency. Furthermore, the proposed ControlAR surprisingly empowers AR models with arbitrary-resolution image generation via conditional decoding and specific controls. Extensive experiments can demonstrate the controllability of the proposed ControlAR for the autoregressive control-to-image generation across diverse inputs, including edges, depths, and segmentation masks. Furthermore, both quantitative and qualitative results indicate that ControlAR surpasses previous state-of-the-art controllable diffusion models, e.g., ControlNet++. Code, models, and demo will soon be available at https://github.com/hustvl/ControlAR.
自回归(AR)模型通过将图像生成重新定义为下一个令牌预测,展现了显著潜力,并成为扩散模型的强劲竞争对手。然而,类似于ControlNet的控制到图像生成在AR模型中仍然未得到广泛探索。尽管受大型语言模型进展的启发,将控制图像令牌化并在解码图像令牌之前将其预先填充到自回归模型中是一种自然的方法,但在生成质量方面与控制网络和效率方面仍存在不足。为此,我们引入了ControlAR,一个高效且有效的框架,用于将空间控制集成到自回归图像生成模型中。首先,我们探索了AR模型的控制编码,并提出了一种轻量级控制编码器,将空间输入(如Canny边缘或深度图)转换为控制令牌。然后,ControlAR利用条件解码方法,根据控制令牌和图像令牌之间的每个令牌的融合来生成下一个图像令牌,类似于位置编码。与使用预先填充令牌相比,使用条件解码显著增强了AR模型的控制能力同时保持了模型的效率。此外,所提出的ControlAR令人惊讶的是通过条件解码和特定控制实现了AR模型的任意分辨率图像生成。大量实验表明,ControlAR在边缘、深度和分割掩码等多种输入下具有可控的自回归控制到图像生成能力。此外,定量和定性结果均表明ControlAR超越了之前的先进可控扩散模型(例如ControlNet++)。代码、模型和演示将很快在https://github.com/hustvl/ControlAR上提供。
论文及项目相关链接
PDF To appear in ICLR 2025. Work in progress
Summary
本文介绍了ControlAR框架,该框架将空间控制集成到自回归图像生成模型中,提高了控制能力和效率。ControlAR通过控制编码将空间输入转换为控制令牌,并采用条件解码方法生成下一个图像令牌,该方法在控制和能力方面优于预填充令牌。此外,ControlAR支持任意分辨率的图像生成,并在边缘、深度、分割蒙版等多样输入上展示了可控的自回归控制到图像生成的效果。
Key Takeaways
- ControlAR框架集成了空间控制到自回归图像生成模型中,提高了图像生成的控制能力和效率。
- 通过控制编码将空间输入转换为控制令牌,为自回归模型提供更准确的指导。
- 采用条件解码方法,根据控制和图像令牌之间的每令牌融合生成下一个图像令牌。
- 与预填充令牌相比,条件解码显著增强了自回归模型的控制能力,同时保持了模型的效率。
- ControlAR支持任意分辨率的图像生成,为用户提供了更大的创作空间。
- 在多种输入(如边缘、深度、分割蒙版等)上展示了ControlAR的可控性和有效性。
点此查看论文截图
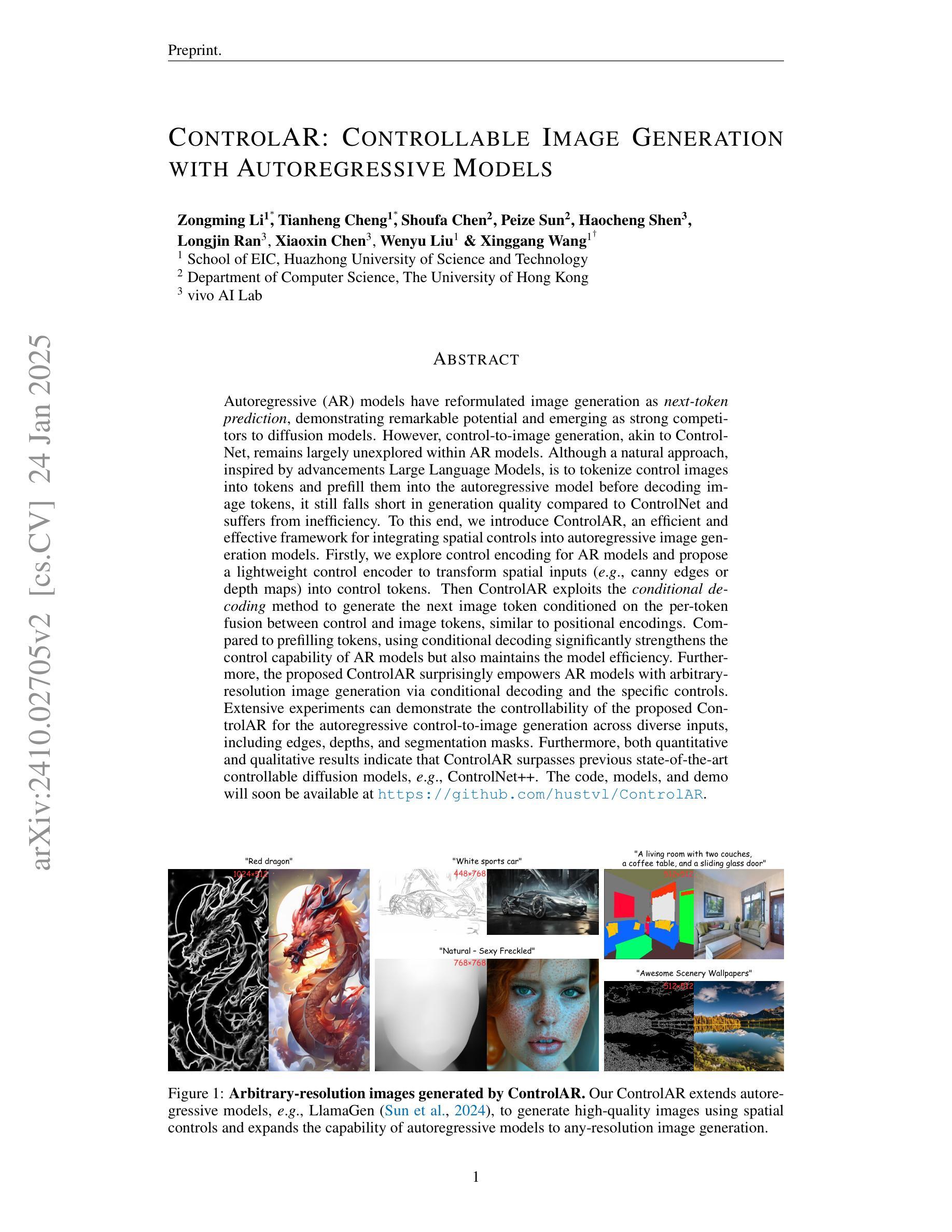
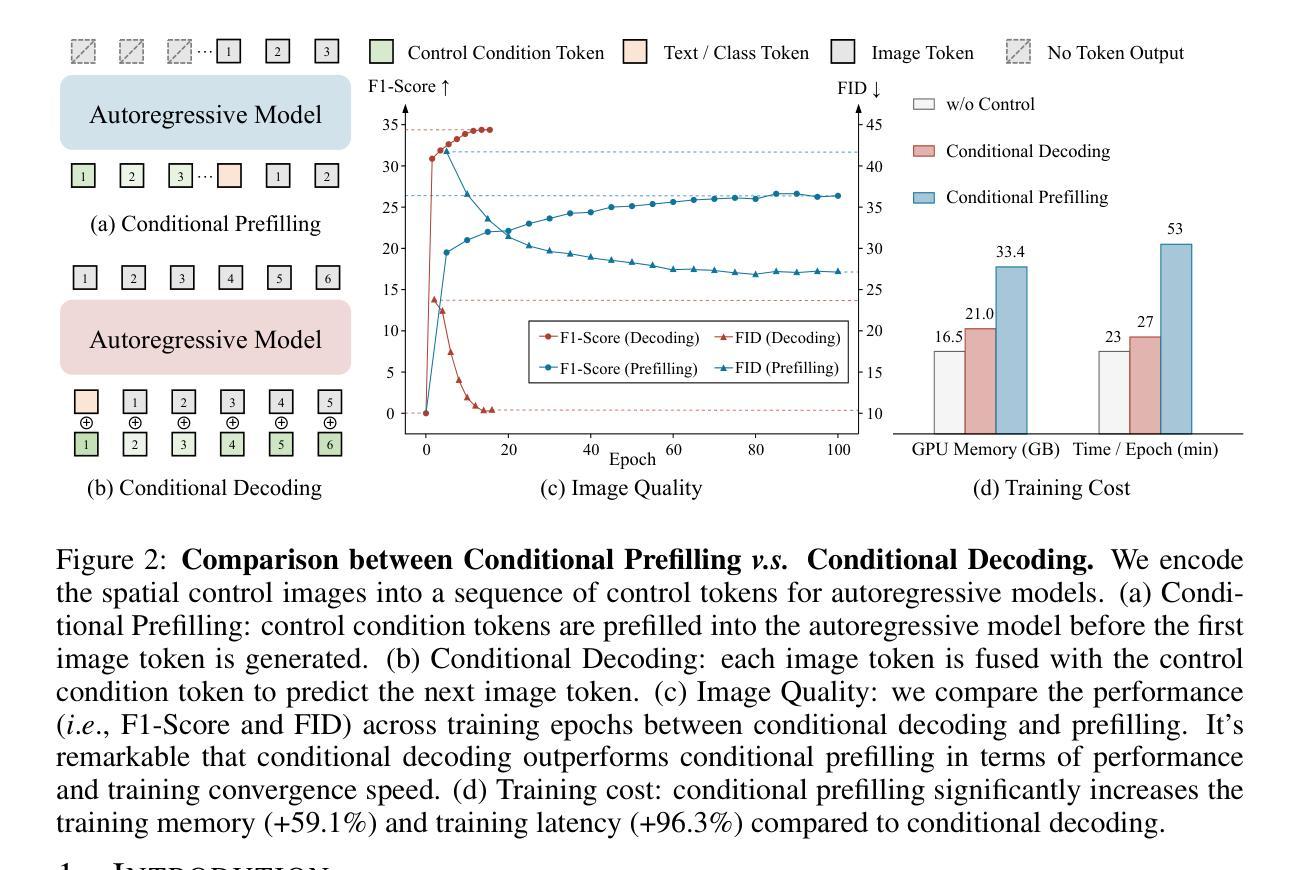
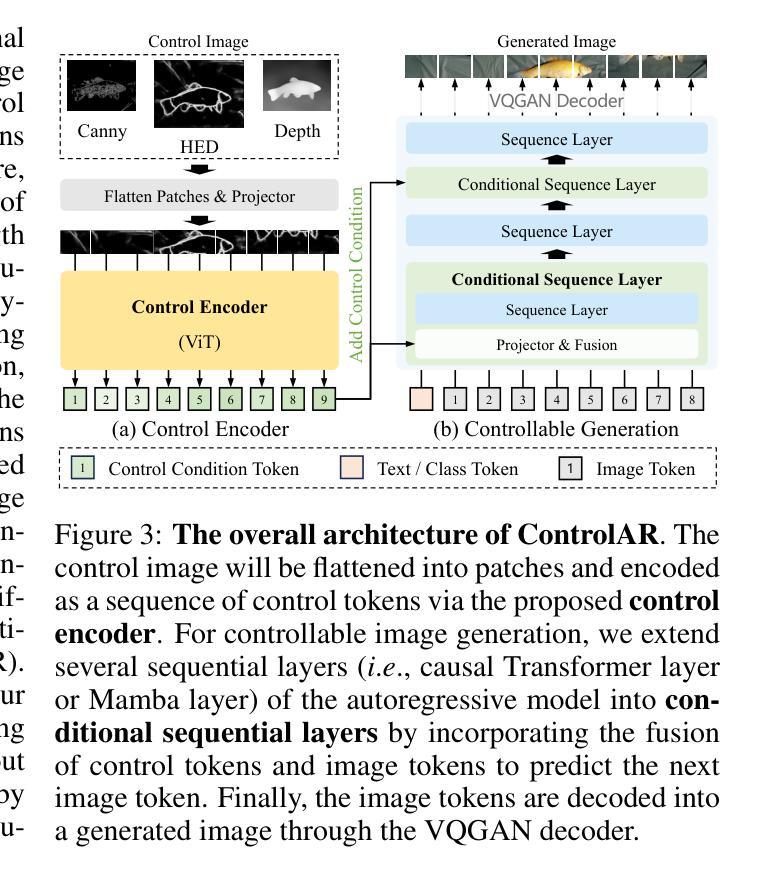