⚠️ 以下所有内容总结都来自于 大语言模型的能力,如有错误,仅供参考,谨慎使用
🔴 请注意:千万不要用于严肃的学术场景,只能用于论文阅读前的初筛!
💗 如果您觉得我们的项目对您有帮助 ChatPaperFree ,还请您给我们一些鼓励!⭐️ HuggingFace免费体验
2025-01-29 更新
Tumor Detection, Segmentation and Classification Challenge on Automated 3D Breast Ultrasound: The TDSC-ABUS Challenge
Authors:Gongning Luo, Mingwang Xu, Hongyu Chen, Xinjie Liang, Xing Tao, Dong Ni, Hyunsu Jeong, Chulhong Kim, Raphael Stock, Michael Baumgartner, Yannick Kirchhoff, Maximilian Rokuss, Klaus Maier-Hein, Zhikai Yang, Tianyu Fan, Nicolas Boutry, Dmitry Tereshchenko, Arthur Moine, Maximilien Charmetant, Jan Sauer, Hao Du, Xiang-Hui Bai, Vipul Pai Raikar, Ricardo Montoya-del-Angel, Robert Marti, Miguel Luna, Dongmin Lee, Abdul Qayyum, Moona Mazher, Qihui Guo, Changyan Wang, Navchetan Awasthi, Qiaochu Zhao, Wei Wang, Kuanquan Wang, Qiucheng Wang, Suyu Dong
Breast cancer is one of the most common causes of death among women worldwide. Early detection helps in reducing the number of deaths. Automated 3D Breast Ultrasound (ABUS) is a newer approach for breast screening, which has many advantages over handheld mammography such as safety, speed, and higher detection rate of breast cancer. Tumor detection, segmentation, and classification are key components in the analysis of medical images, especially challenging in the context of 3D ABUS due to the significant variability in tumor size and shape, unclear tumor boundaries, and a low signal-to-noise ratio. The lack of publicly accessible, well-labeled ABUS datasets further hinders the advancement of systems for breast tumor analysis. Addressing this gap, we have organized the inaugural Tumor Detection, Segmentation, and Classification Challenge on Automated 3D Breast Ultrasound 2023 (TDSC-ABUS2023). This initiative aims to spearhead research in this field and create a definitive benchmark for tasks associated with 3D ABUS image analysis. In this paper, we summarize the top-performing algorithms from the challenge and provide critical analysis for ABUS image examination. We offer the TDSC-ABUS challenge as an open-access platform at https://tdsc-abus2023.grand-challenge.org/ to benchmark and inspire future developments in algorithmic research.
乳腺癌是全球女性最常见的致死原因之一。早期发现有助于减少死亡人数。自动三维乳腺超声(ABUS)是一种新型的乳腺筛查方法,相对于手持式乳腺X线摄影,它具有安全、快速和更高的乳腺癌检测率等诸多优势。肿瘤检测、分割和分类是医学图像分析的关键组成部分,在3D ABUS的上下文中尤其具有挑战性,这是由于肿瘤大小、形状存在重大差异,肿瘤边界不清以及信噪比低。缺乏可公开访问、标注良好的ABUS数据集进一步阻碍了乳腺肿瘤分析系统的进步。为了弥补这一空白,我们组织了首届自动三维乳腺超声肿瘤检测、分割和分类挑战赛(TDSC-ABUS2023)。这一倡议旨在推动该领域的研究,并为与3D ABUS图像分析相关的任务建立明确的基准。本文总结了挑战赛中最优秀的算法,并对ABUS图像检查进行了关键性分析。我们已在https://tdsc-abus2023.grand-challenge.org/上提供TDSC-ABUS挑战赛作为开放访问平台,以评估和激励未来算法研究的发展。
论文及项目相关链接
Summary
乳腺癌是全球女性常见的致死原因,早期发现有助于减少死亡。自动化三维乳腺超声(ABUS)是乳腺筛查的新方法,具有安全性、速度和更高的乳腺癌检测率等优点。肿瘤检测、分割和分类是医学影像分析的关键部分,尤其在ABUS中面临诸多挑战。缺乏公开、标注良好的ABUS数据集阻碍了乳腺肿瘤分析系统的发展。为解决此问题,我们组织了首届自动化三维乳腺超声肿瘤检测、分割和分类挑战赛(TDSC-ABUS2023)。此项目旨在推动相关领域研究,并为ABUS图像分析任务建立基准标准。本文总结了挑战赛表现最佳的算法,并对ABUS图像检查进行了关键性分析。我们提供TDSC-ABUS挑战赛开放平台,以评估并激发未来算法研究的发展。
Key Takeaways
- 乳腺癌是女性常见的致死原因之一,早期发现对降低死亡率至关重要。
- 自动化三维乳腺超声(ABUS)是乳腺筛查的新方法,相比手持式乳腺X光摄影具有诸多优势。
- 肿瘤检测、分割和分类在医学影像分析中至关重要,但在ABUS中面临挑战,如肿瘤大小、形状差异大,边界不清,信噪比低等。
- 缺乏公开、标注良好的ABUS数据集阻碍了乳腺肿瘤分析系统的发展。
- TDSC-ABUS2023挑战赛旨在推动乳腺肿瘤在ABUS中的检测、分割和分类研究,并建立基准标准。
- 挑战赛提供了开放平台供评估和未来算法发展的灵感。
点此查看论文截图
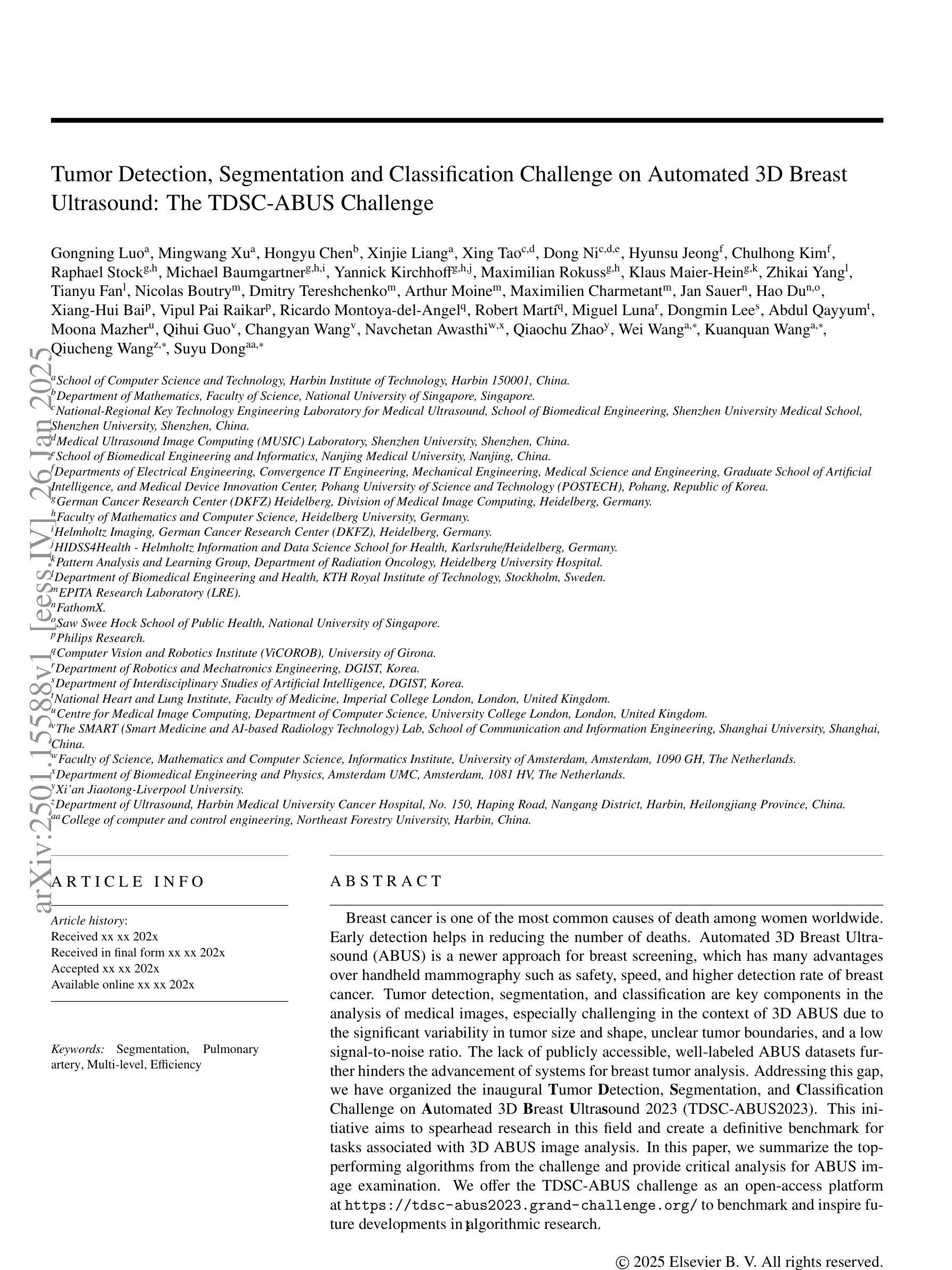
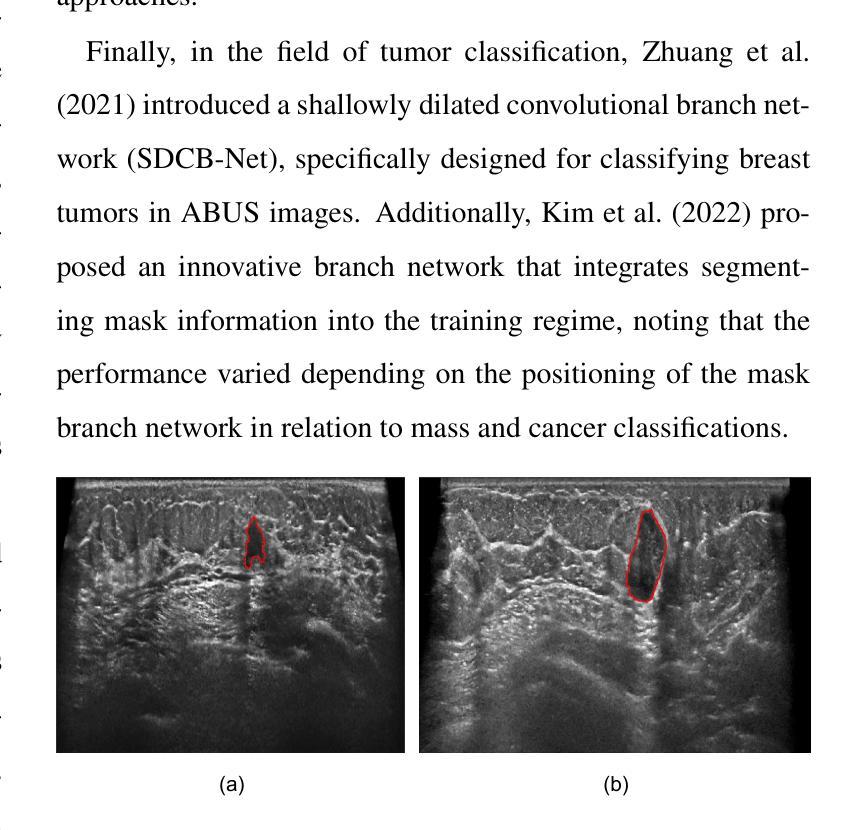
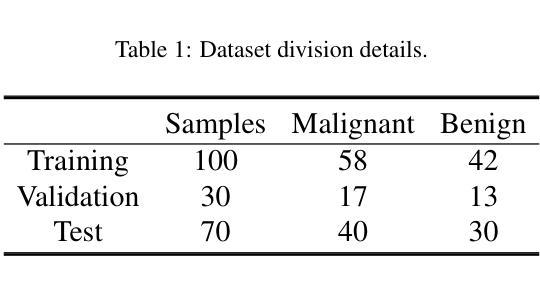
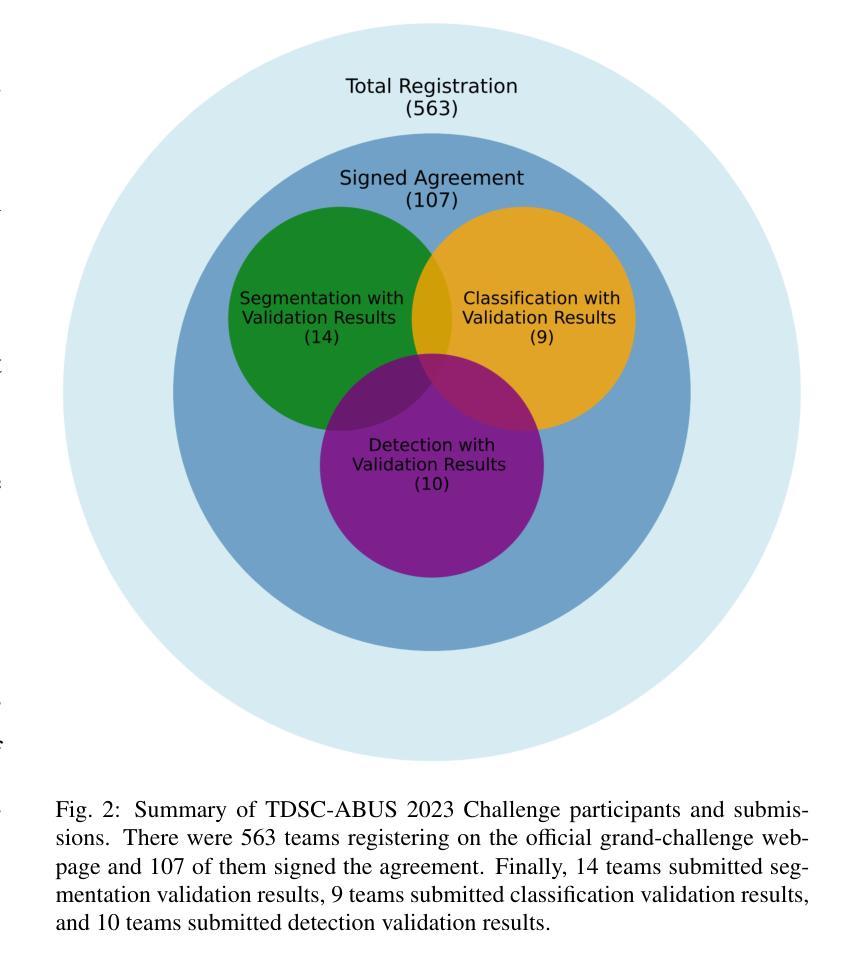
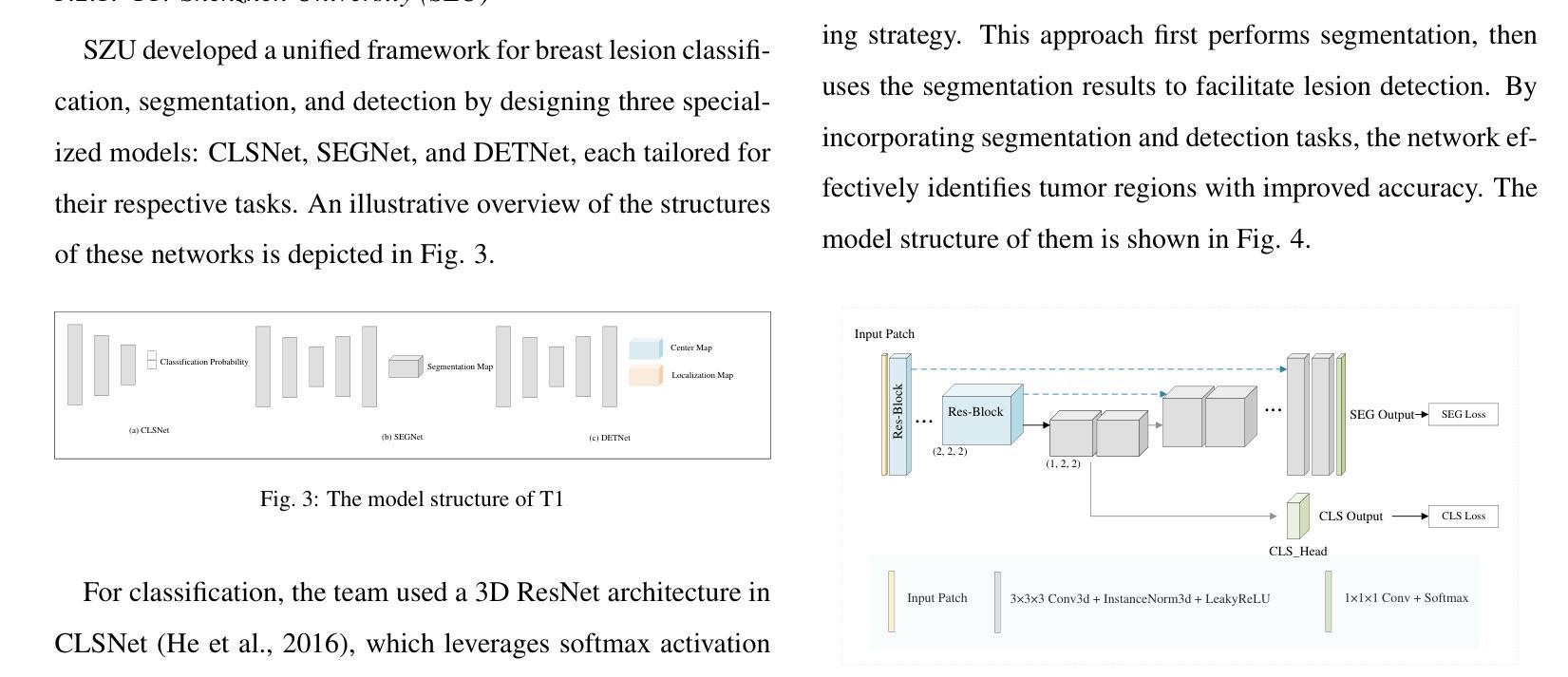