⚠️ 以下所有内容总结都来自于 大语言模型的能力,如有错误,仅供参考,谨慎使用
🔴 请注意:千万不要用于严肃的学术场景,只能用于论文阅读前的初筛!
💗 如果您觉得我们的项目对您有帮助 ChatPaperFree ,还请您给我们一些鼓励!⭐️ HuggingFace免费体验
2025-01-29 更新
Can Location Embeddings Enhance Super-Resolution of Satellite Imagery?
Authors:Daniel Panangian, Ksenia Bittner
Publicly available satellite imagery, such as Sentinel- 2, often lacks the spatial resolution required for accurate analysis of remote sensing tasks including urban planning and disaster response. Current super-resolution techniques are typically trained on limited datasets, leading to poor generalization across diverse geographic regions. In this work, we propose a novel super-resolution framework that enhances generalization by incorporating geographic context through location embeddings. Our framework employs Generative Adversarial Networks (GANs) and incorporates techniques from diffusion models to enhance image quality. Furthermore, we address tiling artifacts by integrating information from neighboring images, enabling the generation of seamless, high-resolution outputs. We demonstrate the effectiveness of our method on the building segmentation task, showing significant improvements over state-of-the-art methods and highlighting its potential for real-world applications.
公开可用的卫星图像,如Sentinel-2,通常缺乏用于准确分析包括城市规划和灾害应对的遥感任务所需的空间分辨率。当前的超分辨率技术通常是在有限的数据集上进行训练的,导致在不同的地理区域之间的泛化能力较差。在这项工作中,我们提出了一种新型的超分辨率框架,该框架通过融入地理位置嵌入信息来提高模型的泛化能力。我们的框架采用对抗生成网络(GANs),并融入了扩散模型的技巧来提升图像质量。此外,我们通过对相邻图像的信息进行整合来解决拼贴痕迹问题,从而生成无缝、高分辨率的输出。我们在建筑分割任务上展示了该方法的有效性,相较于最前沿的方法有明显的提升,并突出了其在现实世界应用中的潜力。
论文及项目相关链接
PDF Accepted to IEEE/CVF Winter Conference on Applications of Computer Vision (WACV)
Summary
本论文针对公开卫星图像分辨率不足的问题,提出了一种结合地理上下文信息的新型超分辨率框架。该框架利用生成对抗网络(GANs)和扩散模型技术,提升图像质量,并解决了图像拼接导致的接缝问题。在建筑物分割任务上,该方法效果显著,相较于现有技术有着明显的提升,为实际应用提供了潜力。
Key Takeaways
- 公开卫星图像如Sentinel-2分辨率不足,影响遥感分析任务的准确性。
- 当前超分辨率技术因训练数据集有限,地理区域间的泛化能力较差。
- 新型超分辨率框架结合地理上下文信息,通过位置嵌入增强泛化能力。
- 框架采用生成对抗网络(GANs)和扩散模型技术,提升图像质量。
- 框架解决了图像拼接导致的接缝问题,实现无缝高清晰度输出。
- 该方法在建筑物分割任务上效果突出,显著优于现有技术。
点此查看论文截图
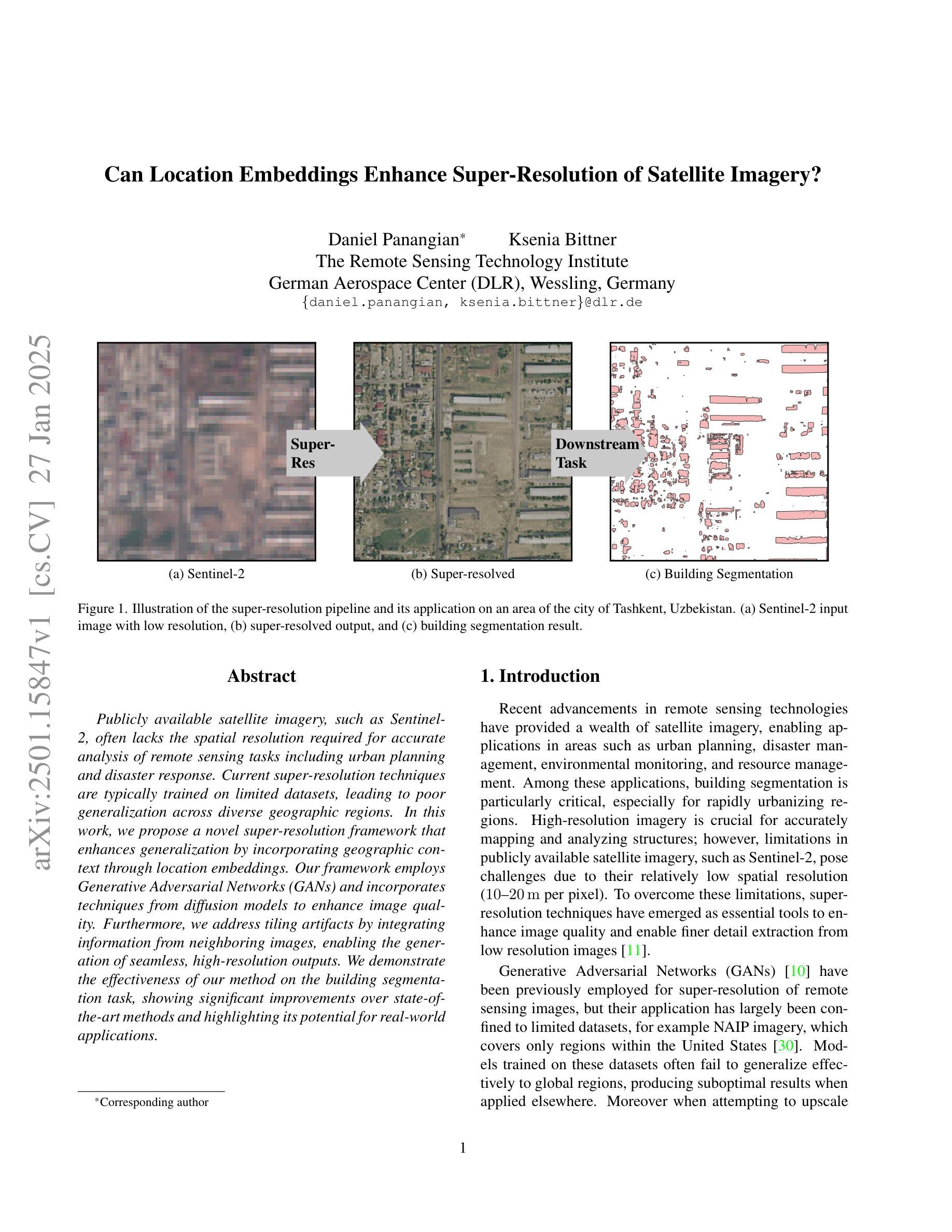
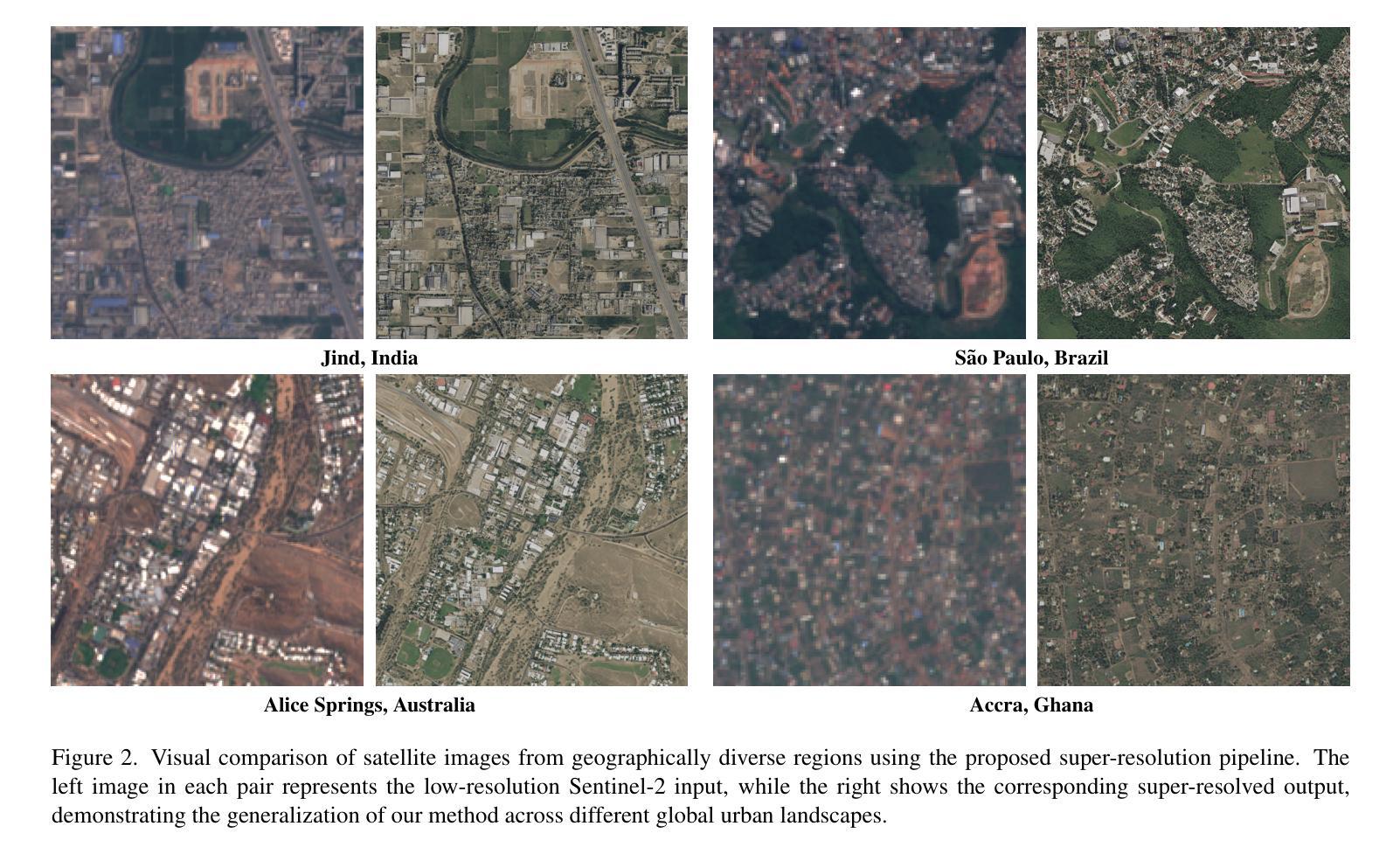
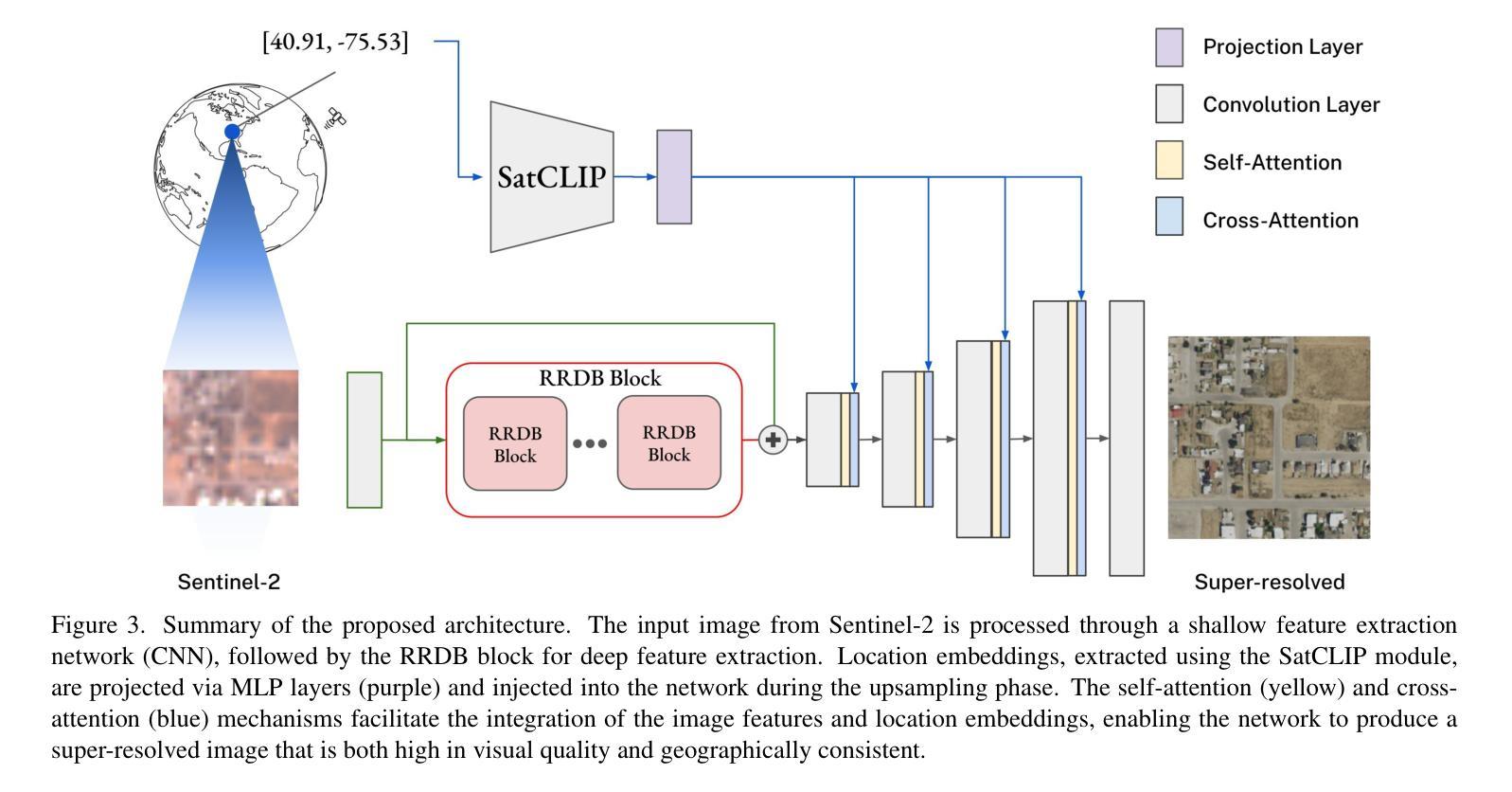
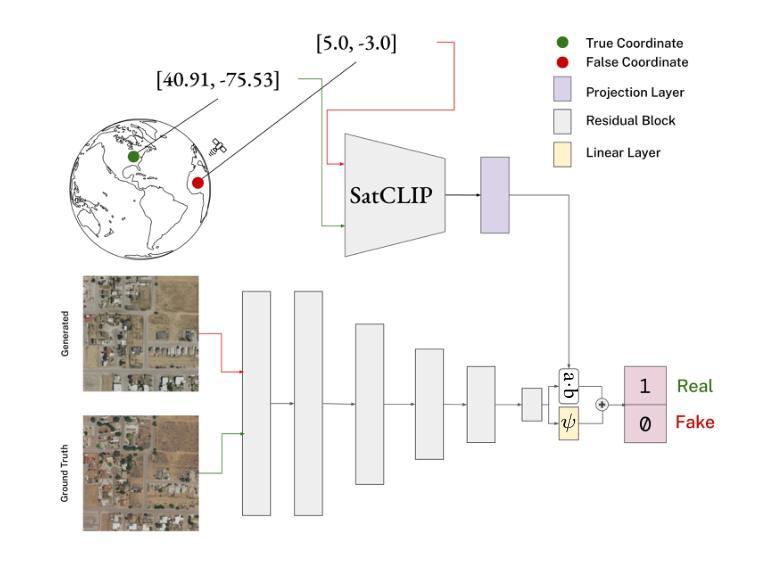
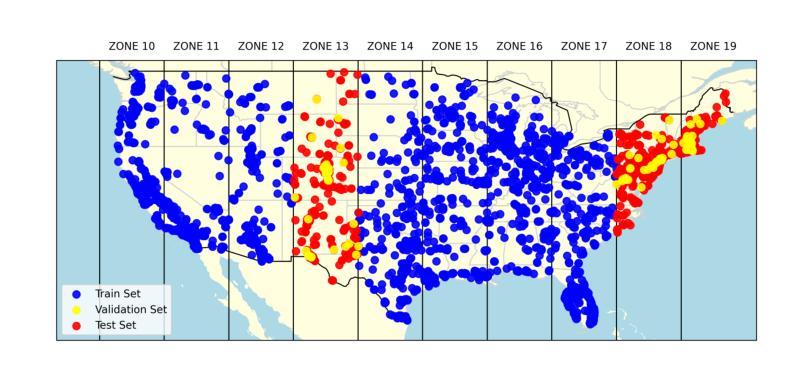
Comparative clinical evaluation of “memory-efficient” synthetic 3d generative adversarial networks (gan) head-to-head to state of art: results on computed tomography of the chest
Authors:Mahshid shiri, Chandra Bortolotto, Alessandro Bruno, Alessio Consonni, Daniela Maria Grasso, Leonardo Brizzi, Daniele Loiacono, Lorenzo Preda
Introduction: Generative Adversarial Networks (GANs) are increasingly used to generate synthetic medical images, addressing the critical shortage of annotated data for training Artificial Intelligence (AI) systems. This study introduces a novel memory-efficient GAN architecture, incorporating Conditional Random Fields (CRFs) to generate high-resolution 3D medical images and evaluates its performance against the state-of-the-art hierarchical (HA)-GAN model. Materials and Methods: The CRF-GAN was trained using the open-source lung CT LUNA16 dataset. The architecture was compared to HA-GAN through a quantitative evaluation, using Frechet Inception Distance (FID) and Maximum Mean Discrepancy (MMD) metrics, and a qualitative evaluation, through a two-alternative forced choice (2AFC) test completed by a pool of 12 resident radiologists, in order to assess the realism of the generated images. Results: CRF-GAN outperformed HA-GAN with lower FID (0.047 vs. 0.061) and MMD (0.084 vs. 0.086) scores, indicating better image fidelity. The 2AFC test showed a significant preference for images generated by CRF-Gan over those generated by HA-GAN with a p-value of 1.93e-05. Additionally, CRF-GAN demonstrated 9.34% lower memory usage at 256 resolution and achieved up to 14.6% faster training speeds, offering substantial computational savings. Discussion: CRF-GAN model successfully generates high-resolution 3D medical images with non-inferior quality to conventional models, while being more memory-efficient and faster. Computational power and time saved can be used to improve the spatial resolution and anatomical accuracy of generated images, which is still a critical factor limiting their direct clinical applicability.
简介:生成对抗网络(GANs)越来越多地被用于生成合成医学图像,以解决训练人工智能(AI)系统时标注数据严重短缺的问题。本研究介绍了一种新的内存高效的GAN架构,该架构结合了条件随机场(CRFs)来生成高分辨率的3D医学图像,并与当前先进的分层(HA)-GAN模型进行了性能评估。材料与方法:CRF-GAN使用开源的肺部CT LUNA16数据集进行训练。通过与HA-GAN进行定量评估,使用Frechet Inception Distance(FID)和Maximum Mean Discrepancy(MMD)指标,以及定性评估,通过由12名驻地放射科医生完成的两种替代强制选择(2AFC)测试,以评估生成图像的真实性。结果:CRF-GAN在FID(0.047 vs. 0.061)和MMD(0.084 vs. 0.086)得分上优于HA-GAN,表明图像保真度更高。2AFC测试显示,CRF-Gan生成的图像比HA-GAN生成的图像更受欢迎,p值为1.93e-05。此外,CRF-GAN在256分辨率下内存使用率低9.34%,训练速度提高了高达14.6%,从而实现了大量的计算节约。讨论:CRF-GAN模型成功地生成了高分辨率的3D医学图像,其质量与传统模型相当或更好,同时更节省内存、速度更快。所节省的计算能力和时间可用于提高生成图像的空间分辨率和解剖准确性,这仍然是限制其直接临床应用的关键因素。
论文及项目相关链接
Summary
本研究介绍了一种结合条件随机场(CRF)的新型内存高效生成对抗网络(CRF-GAN),用于生成高质量的三维医学图像,解决了训练人工智能系统所需的标注数据短缺的问题。与现有的层次化对抗生成网络(HA-GAN)相比,CRF-GAN在图像保真度、训练速度和内存使用效率方面表现出优势。通过定量评估和定性评估验证了其性能。
Key Takeaways
- CRF-GAN被引入用于生成高分辨率的三维医学图像,以解决标注数据短缺的问题。
- CRF-GAN结合了条件随机场(CRF)以提高图像生成的质量。
- 与现有的HA-GAN相比,CRF-GAN在图像保真度方面表现更优,具有更低的Frechet Inception Distance (FID)和Maximum Mean Discrepancy (MMD)得分。
- CRF-GAN生成的图像获得了居民放射科医师的显著偏好。
- CRF-GAN具有更高的内存效率和更快的训练速度。
- CRF-GAN的优异性能使其在医学图像生成方面具有潜在的临床应用价值。
点此查看论文截图
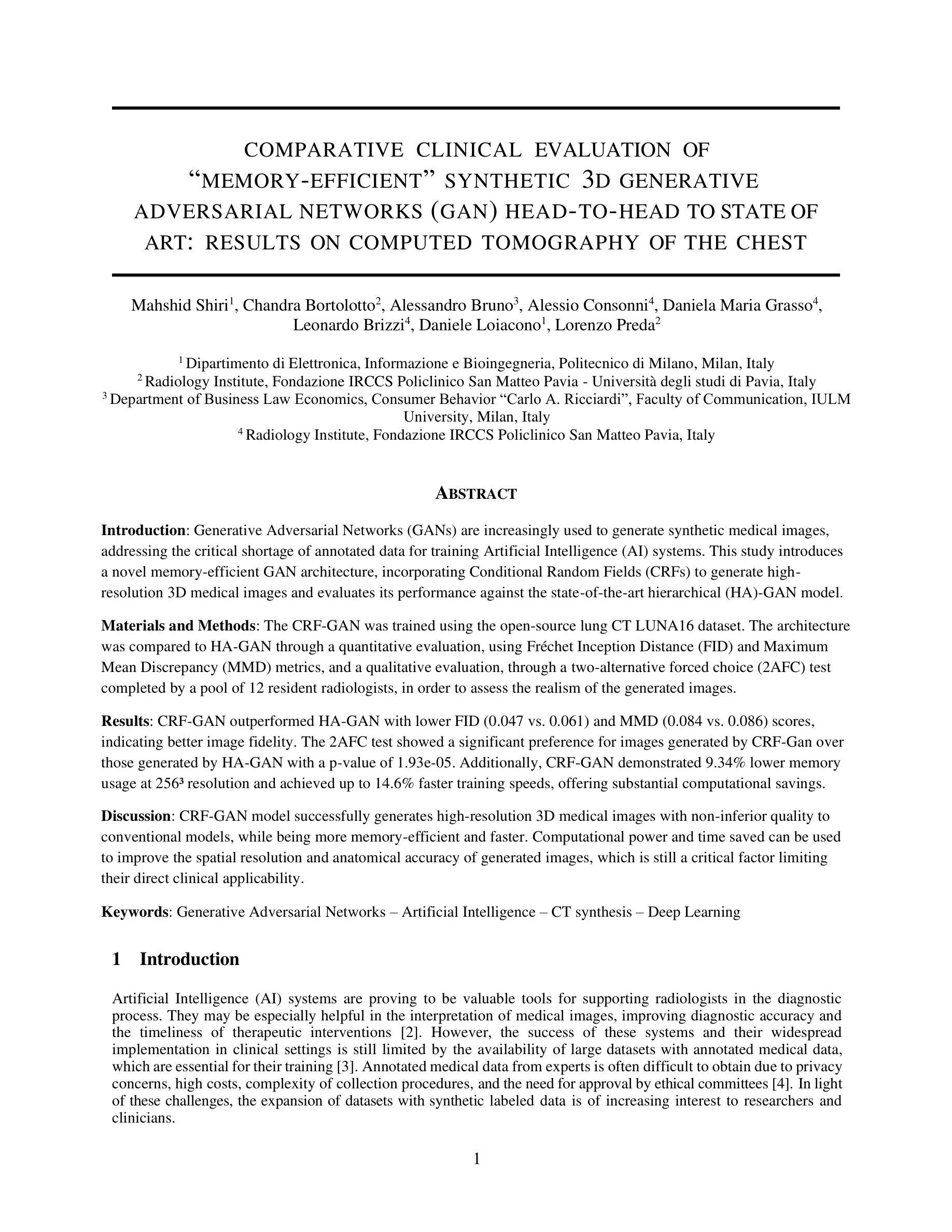
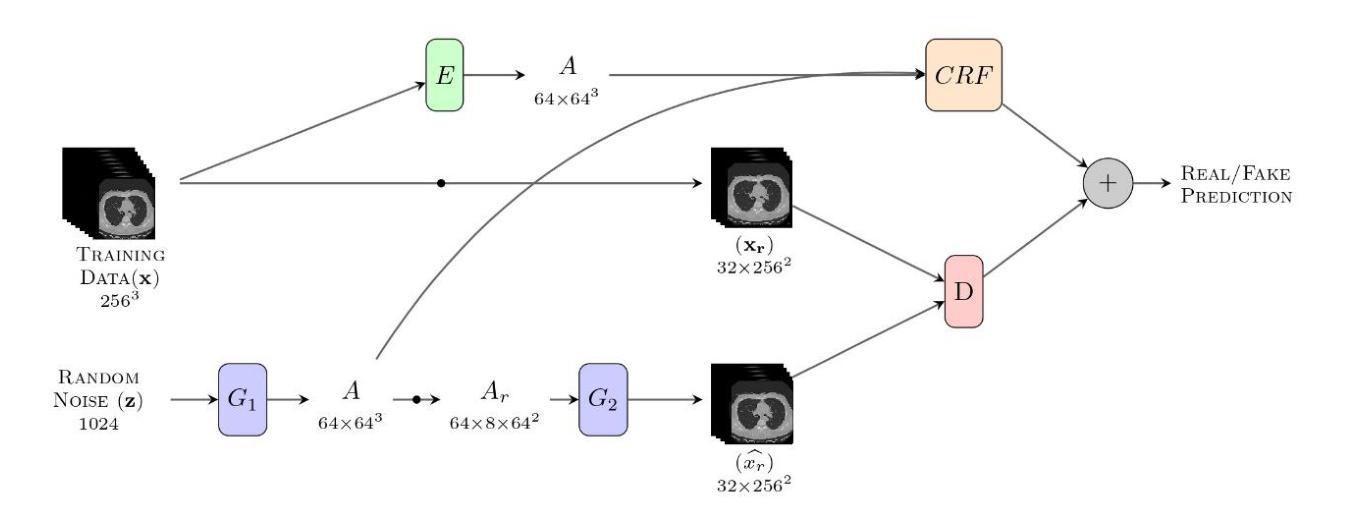
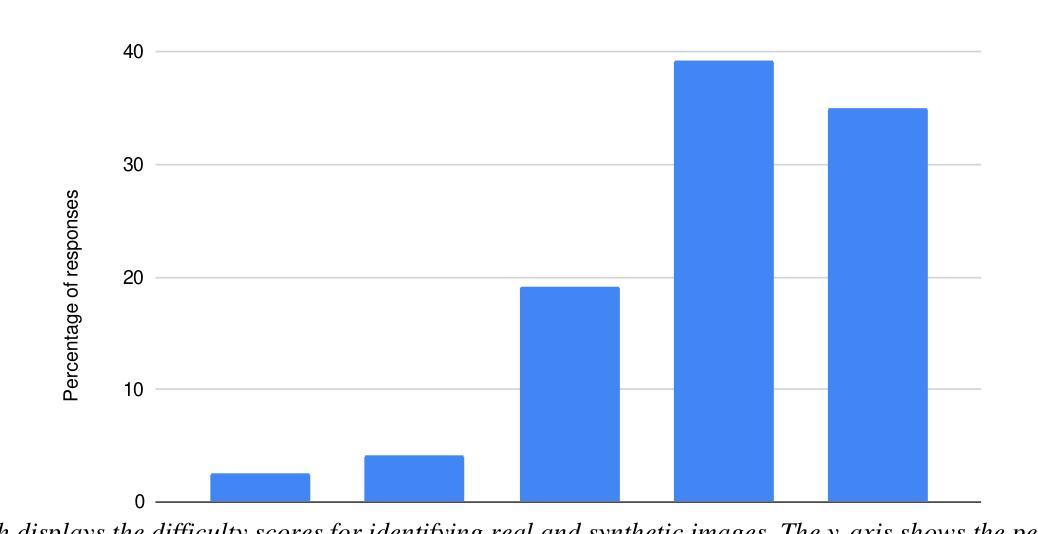
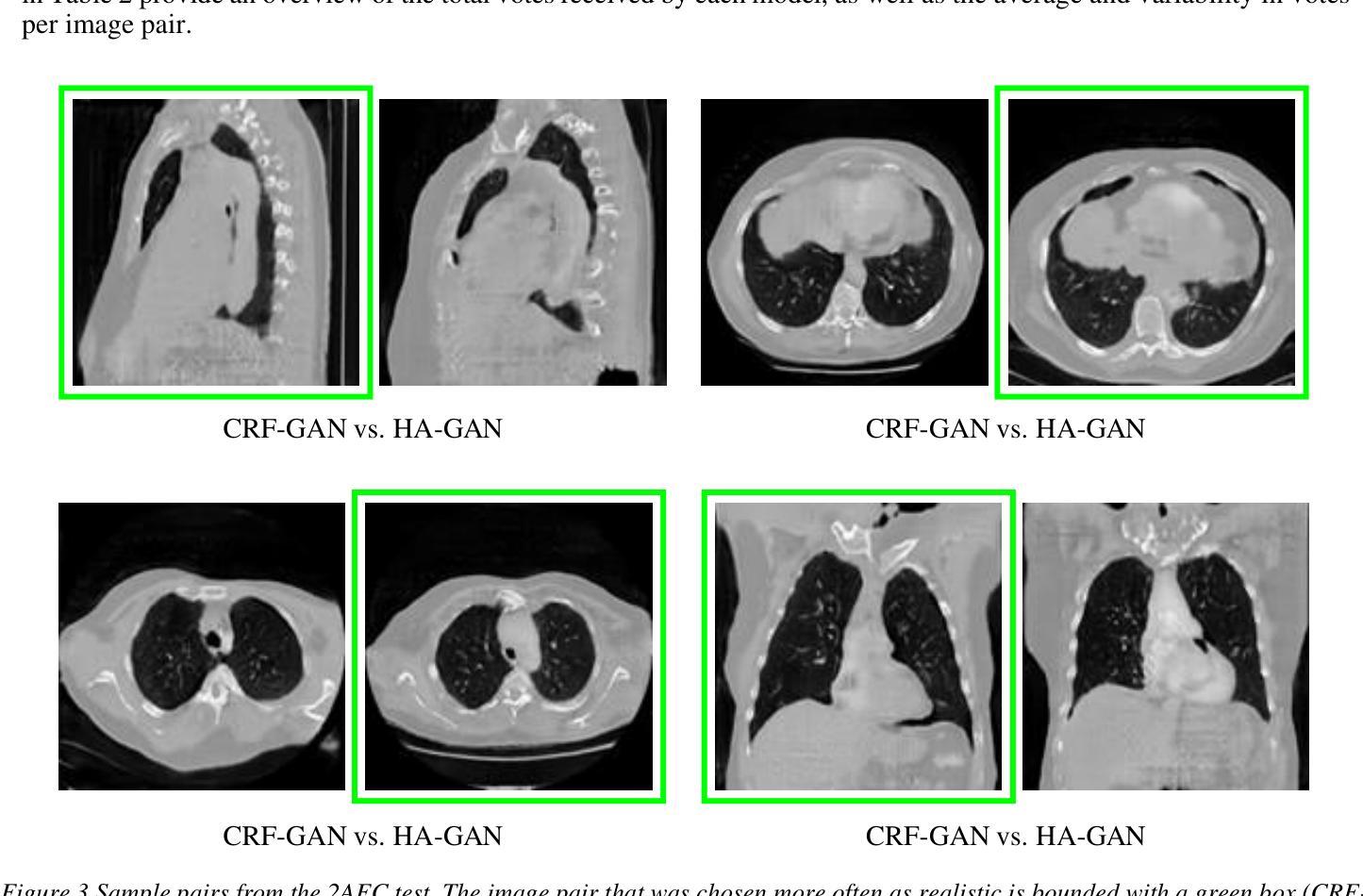
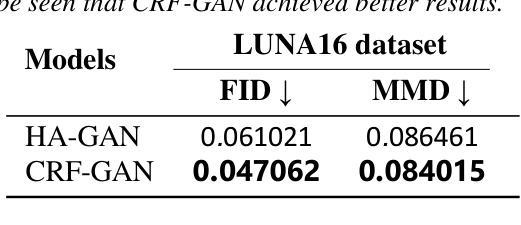
Generalizable Deepfake Detection via Effective Local-Global Feature Extraction
Authors:Jiazhen Yan, Ziqiang Li, Ziwen He, Zhangjie Fu
The rapid advancement of GANs and diffusion models has led to the generation of increasingly realistic fake images, posing significant hidden dangers and threats to society. Consequently, deepfake detection has become a pressing issue in today’s world. While some existing methods focus on forgery features from either a local or global perspective, they often overlook the complementary nature of these features. Other approaches attempt to incorporate both local and global features but rely on simplistic strategies, such as cropping, which fail to capture the intricate relationships between local features. To address these limitations, we propose a novel method that effectively combines local spatial-frequency domain features with global frequency domain information, capturing detailed and holistic forgery traces. Specifically, our method uses Discrete Wavelet Transform (DWT) and sliding windows to tile forged features and leverages attention mechanisms to extract local spatial-frequency domain information. Simultaneously, the phase component of the Fast Fourier Transform (FFT) is integrated with attention mechanisms to extract global frequency domain information, complementing the local features and ensuring the integrity of forgery detection. Comprehensive evaluations on open-world datasets generated by 34 distinct generative models demonstrate a significant improvement of 2.9% over existing state-of-the-art methods.
生成对抗网络(GANs)和扩散模型的快速发展导致了越来越逼真的虚假图像生成,给社会带来了重大的潜在危险和威胁。因此,深度伪造检测已成为当今世界的紧迫问题。虽然现有的某些方法侧重于从局部或全局角度提取伪造特征,但它们往往忽略了这些特征的互补性。其他方法试图结合局部和全局特征,但依赖的是裁剪等简单策略,无法捕捉到局部特征之间复杂的关系。为了解决这些局限性,我们提出了一种新的方法,该方法有效地结合了局部空间频域特征和全局频域信息,捕捉详细且整体的伪造痕迹。具体来说,我们的方法使用离散小波变换(DWT)和滑动窗口来分割伪造特征,并利用注意力机制来提取局部空间频域信息。同时,结合快速傅里叶变换(FFT)的相位成分和注意力机制来提取全局频域信息,以补充局部特征并确保伪造检测的完整性。在由34种不同的生成模型生成的公开数据集上的综合评估表明,与现有的最先进方法相比,其性能提高了2.9%。
论文及项目相关链接
PDF under review
Summary
GAN和扩散模型的快速发展导致生成越来越逼真的虚假图像,给社会带来重大潜在危险和威胁。因此,深度伪造检测已成为当今世界的紧迫问题。现有方法多侧重于局部或全局的伪造特征,忽略了特征的互补性。为解决这一问题,我们提出了一种新方法,有效结合局部空间频域特征和全局频域信息,捕捉详细而全面的伪造痕迹。通过离散小波变换(DWT)和滑动窗口来提取伪造特征,并利用注意力机制提取局部空间频域信息。同时,结合快速傅里叶变换(FFT)的相位成分和注意力机制提取全局频域信息,与局部特征互补,确保伪造检测的完整性。在由34种不同生成模型生成的公开数据集上进行全面评估,较现有最先进的检测算法提高了2.9%。
Key Takeaways
- GAN和扩散模型的快速发展导致虚假图像的生成越来越逼真,带来社会威胁,深度伪造检测成为重要议题。
- 现有检测方法的局限性在于忽略局部和全局伪造特征的互补性。
- 提出了一种结合局部空间频域特征和全局频域信息的新方法,以捕捉详细而全面的伪造痕迹。
- 使用离散小波变换(DWT)和滑动窗口提取伪造特征。
- 利用注意力机制提取局部空间频域信息。
- 结合快速傅里叶变换(FFT)的相位成分和注意力机制提取全局频域信息,与局部特征互补。
点此查看论文截图
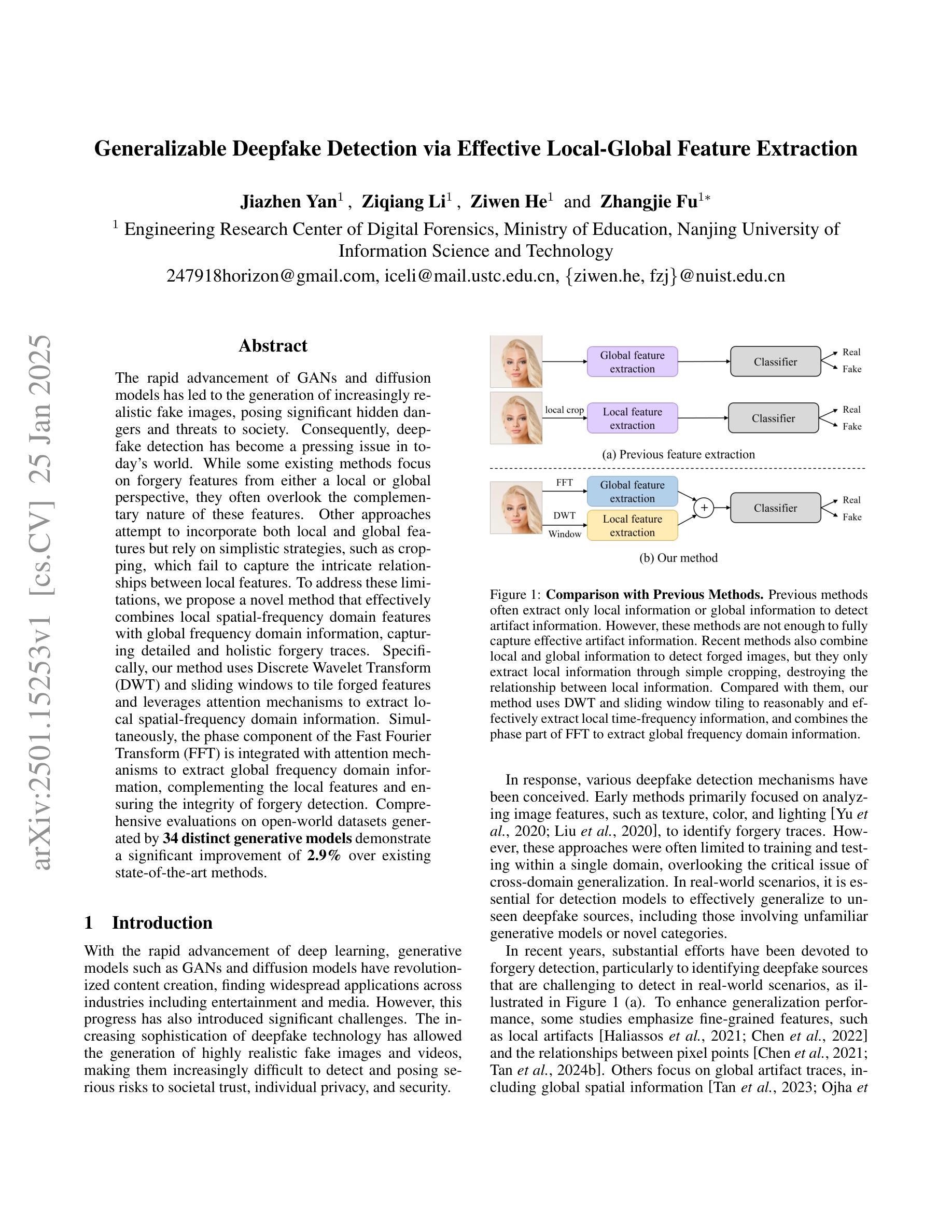
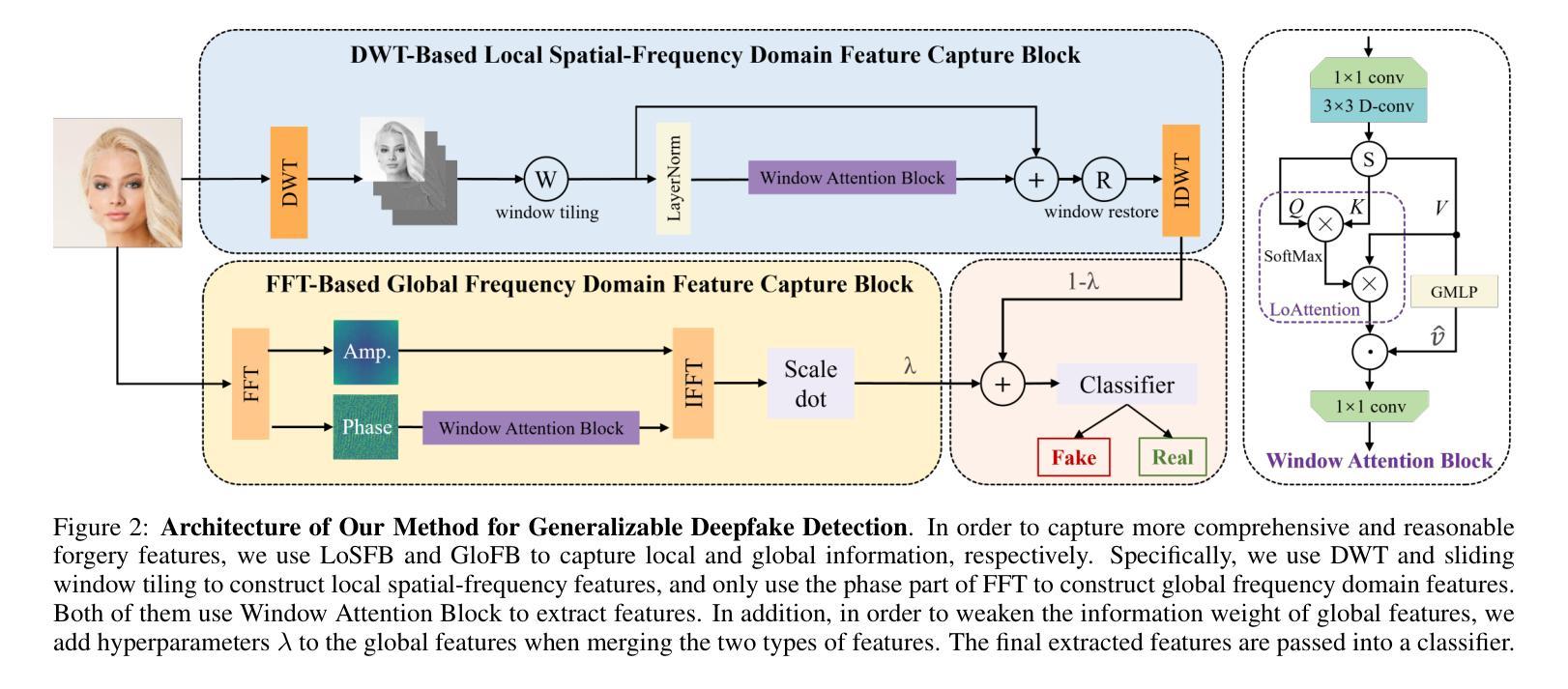
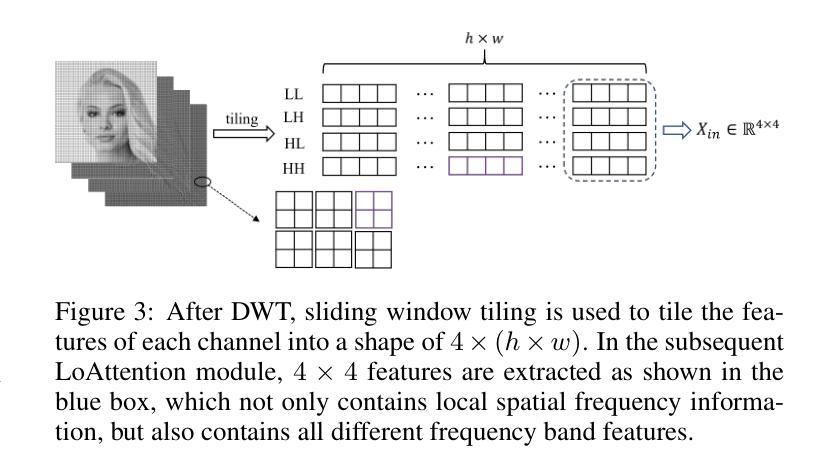
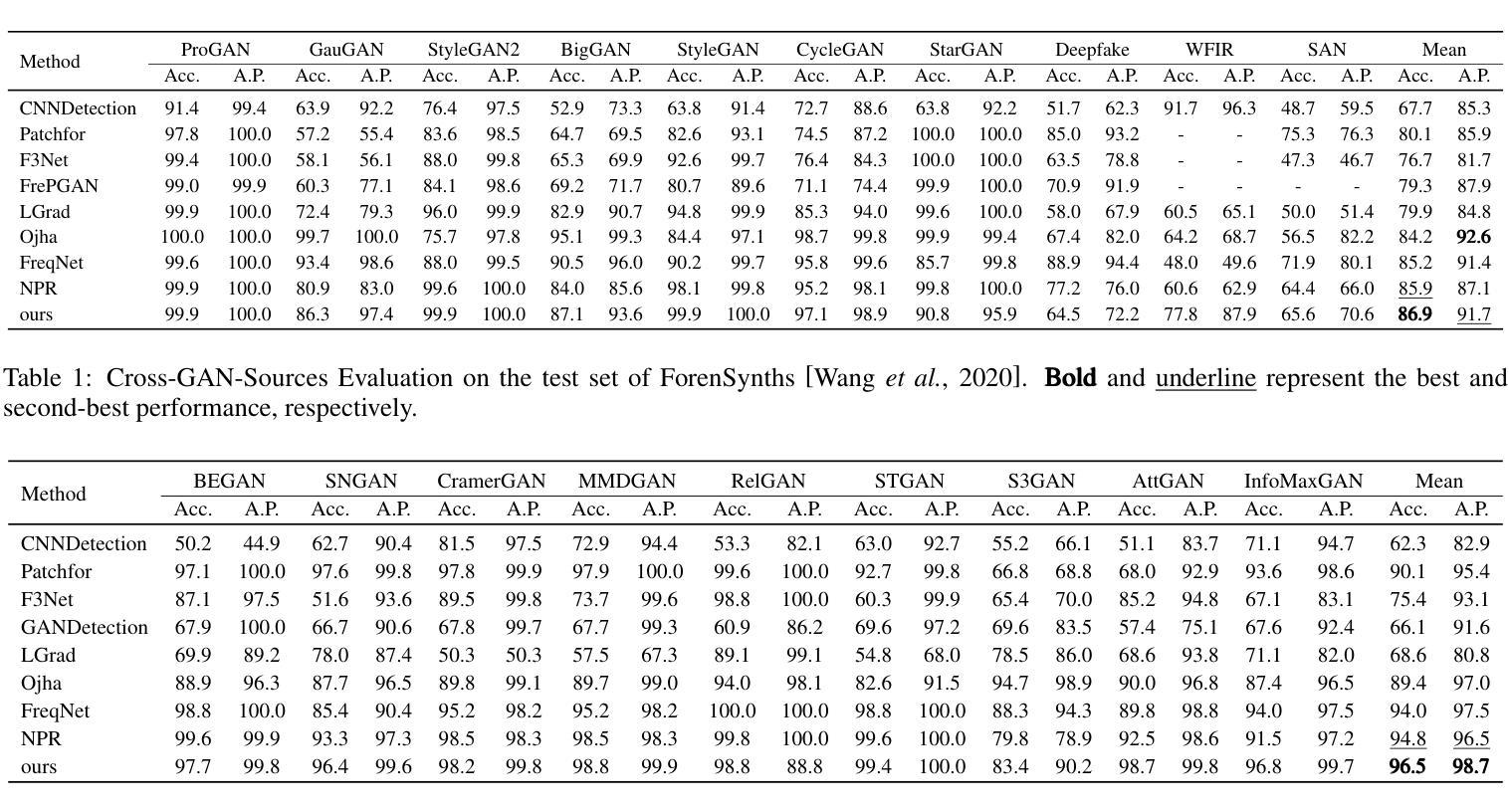
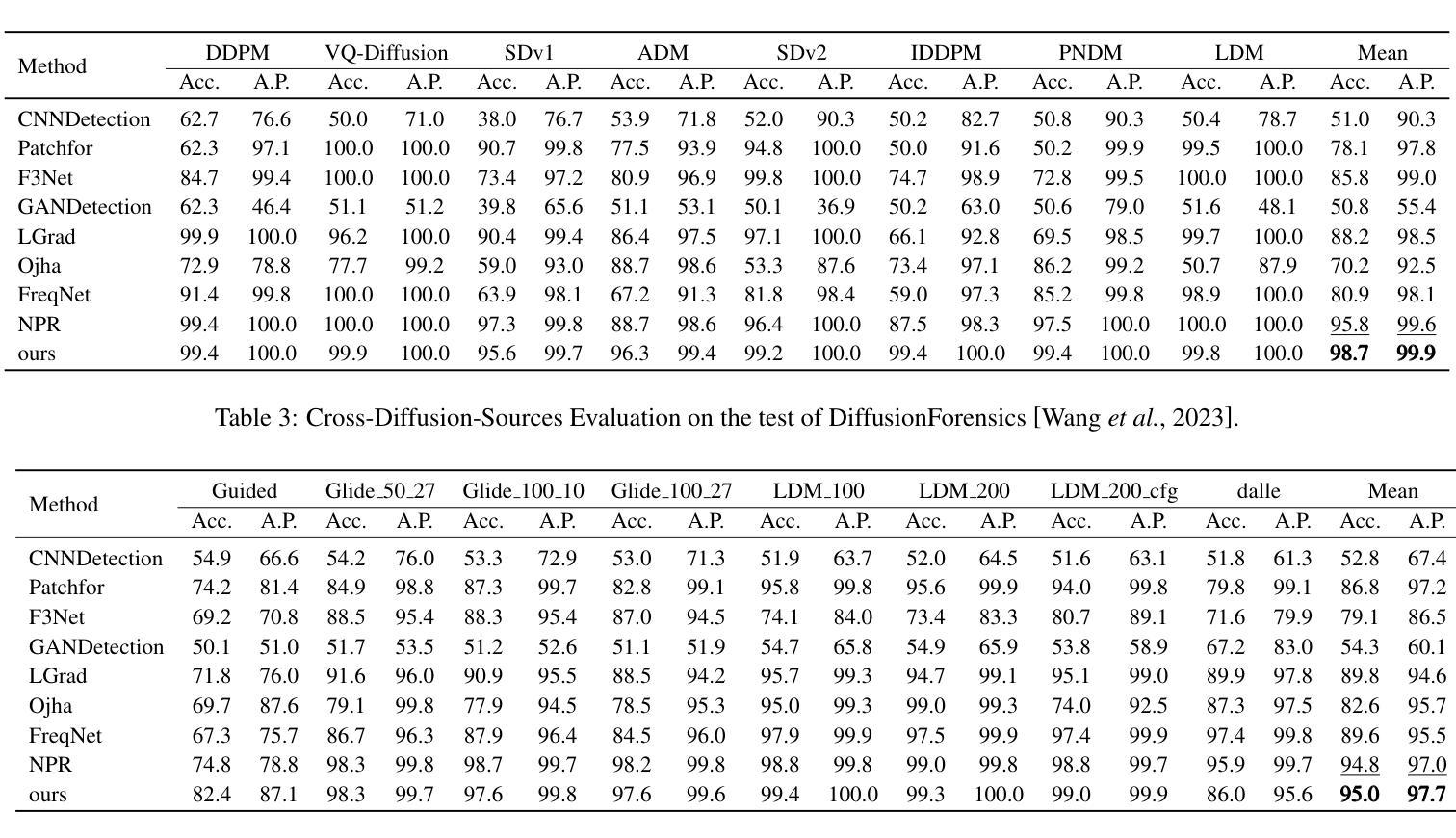