⚠️ 以下所有内容总结都来自于 大语言模型的能力,如有错误,仅供参考,谨慎使用
🔴 请注意:千万不要用于严肃的学术场景,只能用于论文阅读前的初筛!
💗 如果您觉得我们的项目对您有帮助 ChatPaperFree ,还请您给我们一些鼓励!⭐️ HuggingFace免费体验
2025-01-29 更新
Compensator-based small animal IMRT enables conformal preclinical dose painting: application to tumor hypoxia
Authors:Jordan M. Slagowski, Erik Pearson, Rajit Tummala, Gage Redler, Daniela Olivera Velarde, Boris Epel, Howard J. Halpern, Bulent Aydogan
Techniques for preclinical intensity modulated radiation therapy are being developed to improve translation by replicating the clinical paradigm. This study presents the first treatment planning comparison between small animal IMRT (SA-IMRT) and three-dimensional conformal radiotherapy (CRT) in a model application, oxygen-guided dose painting of tumor hypoxia, using actual mouse data. A novel compensator-based platform was employed to generate SA-IMRT and CRT plans with 2-15 beam angles for seventeen mice with fibrosarcoma tumors. The whole tumor received a dose of 22.5 Gy, with a simultaneous integrated boost of 13 Gy to hypoxic voxels identified via electron paramagnetic resonance imaging. Plan quality was assessed using the Paddick conformity index (CI), uniformity, and dose volume histograms. For 3-angles, SA-IMRT yielded significantly improved dose conformity (median hypoxic CI =0.45 versus 0.17), tumor dose uniformity (11.0% versus 14.3%), and dosimetric spread between boost and non-boost targets (D50% difference = 13.0 Gy [ideal], 13.1 Gy [SA-IMRT], 7. 3 Gy [CRT]). No significant improvement in CI was associated with >3 beam angles (Wilcoxon signed-rank test, p < 0.05). This study demonstrates that SA-IMRT provides significant improvements in radiation plan quality and yields dose distributions that more closely mimic the clinical setting relative to current CRT approaches.
预临床强度调制辐射治疗技术正在开发,以复制临床范例来改善翻译。本研究以小鼠体内真实数据为例,提出一种在模型应用中小动物IMRT(SA-IMRT)与三维适形放疗(CRT)的首次治疗计划比较,即在肿瘤缺氧条件下采用氧导向剂量染色。本研究采用基于补偿器平台生成SA-IMRT和CRT计划,在面向17只患有纤维肉瘤肿瘤的鼠的照射中使用了从2到15个束角。整个肿瘤接受的剂量为22.5 Gy,同时给予缺氧体积瞬时提升剂量为13 Gy(基于电子顺磁共振成像法确定)。计划质量通过Paddick一致性指数(CI)、均匀性和剂量体积直方图进行评估。对于3个角度而言,SA-IMRT在剂量一致性方面显著改善(缺氧中位数CI为0.45对比CRT为0.17),肿瘤剂量均匀性也显著改善(对比差异值为十一一点零%对比为十四点三个点),且在加强照射和非加强目标之间的剂量散布更好(D五〇%的差异值为理想的十三点零Gy,SA-IMRT为十三点一Gy,CRT为七点三Gy)。对于超过三个束角的情况,一致性指数没有显著改善(Wilcoxon符号秩检验,p < 0.05)。本研究表明,相对于当前的CRT方法,SA-IMRT在辐射计划质量方面提供了显著改善,产生的剂量分布更接近模拟临床环境。
论文及项目相关链接
Summary
该研究对比了小动物IMRT(SA-IMRT)与三维适形放疗(CRT)在治疗计划方面的差异,以模拟临床环境中的肿瘤缺氧放疗计划为例。研究使用基于补偿器平台的技术,对带有纤维肉瘤肿瘤的17只小鼠进行SA-IMRT和CRT计划。结果显示,SA-IMRT在剂量一致性、肿瘤剂量均匀性和剂量分布方面显著优于CRT。特别是在使用较少的三个角度时,SA-IMRT表现出明显的优势。总的来说,该研究证实了SA-IMRT在提升放疗计划质量方面具有重要意义,并且其在剂量分布上更接近临床环境相对的传统CRT方法。
Key Takeaways
- SA-IMRT与CRT在小动物模型上的治疗计划比较。
- 使用基于补偿器平台的技术进行放疗计划。
- SA-IMRT在剂量一致性、肿瘤剂量均匀性和剂量分布方面优于CRT。
- 在使用较少的三个角度时,SA-IMRT表现出明显的优势。
- SA-IMRT计划更贴近临床环境。
- 研究证实了SA-IMRT在提升放疗计划质量上的重要性。
点此查看论文截图
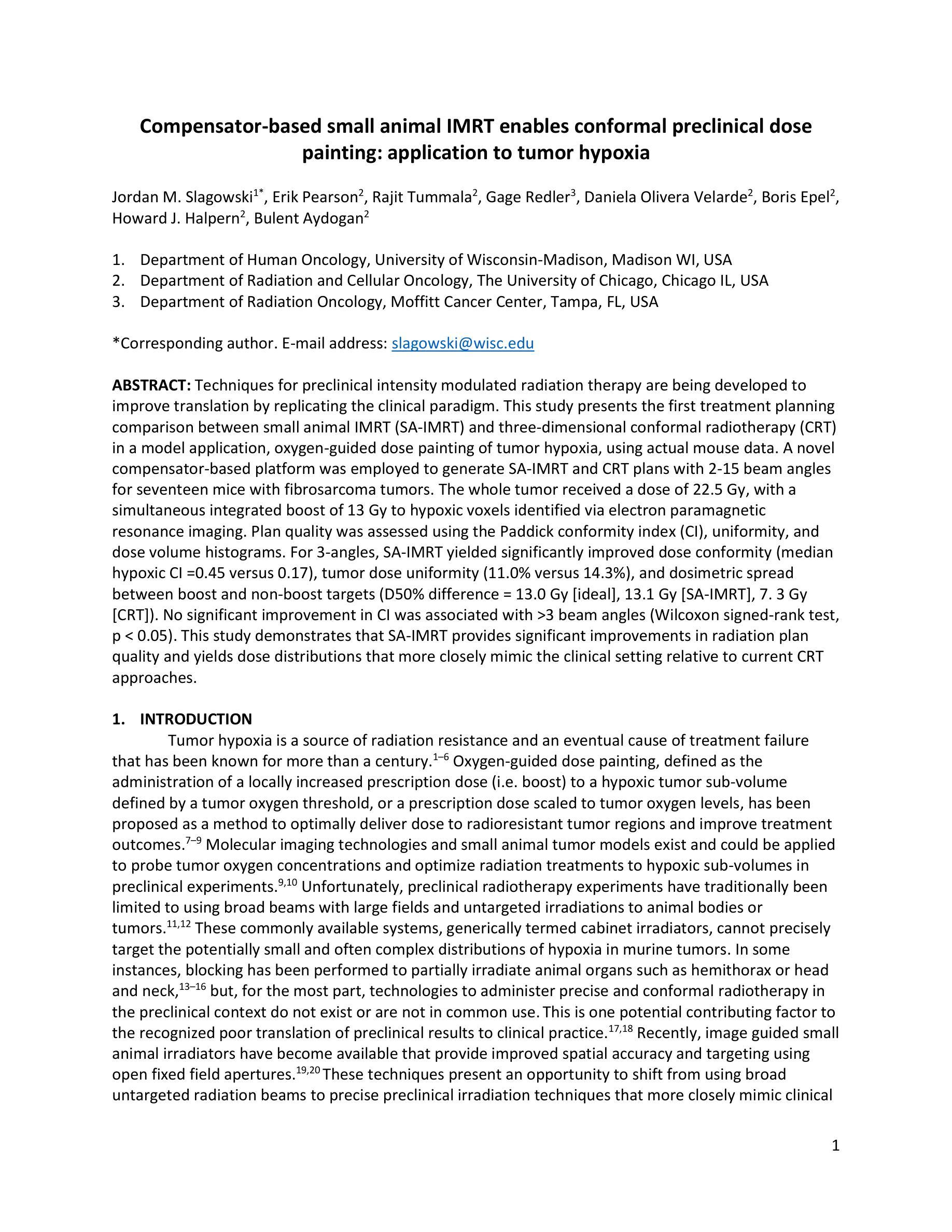
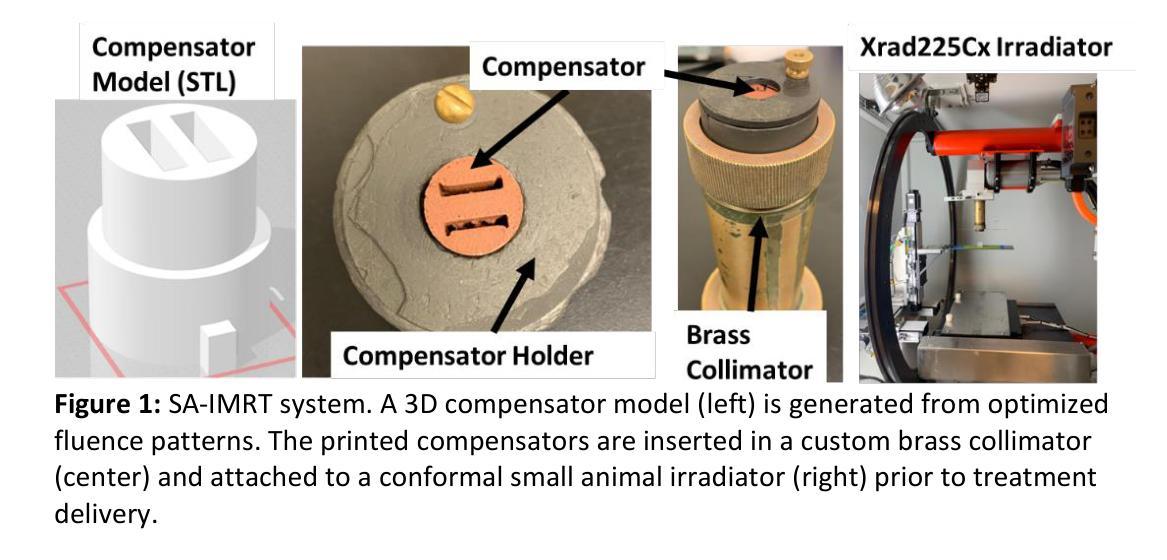
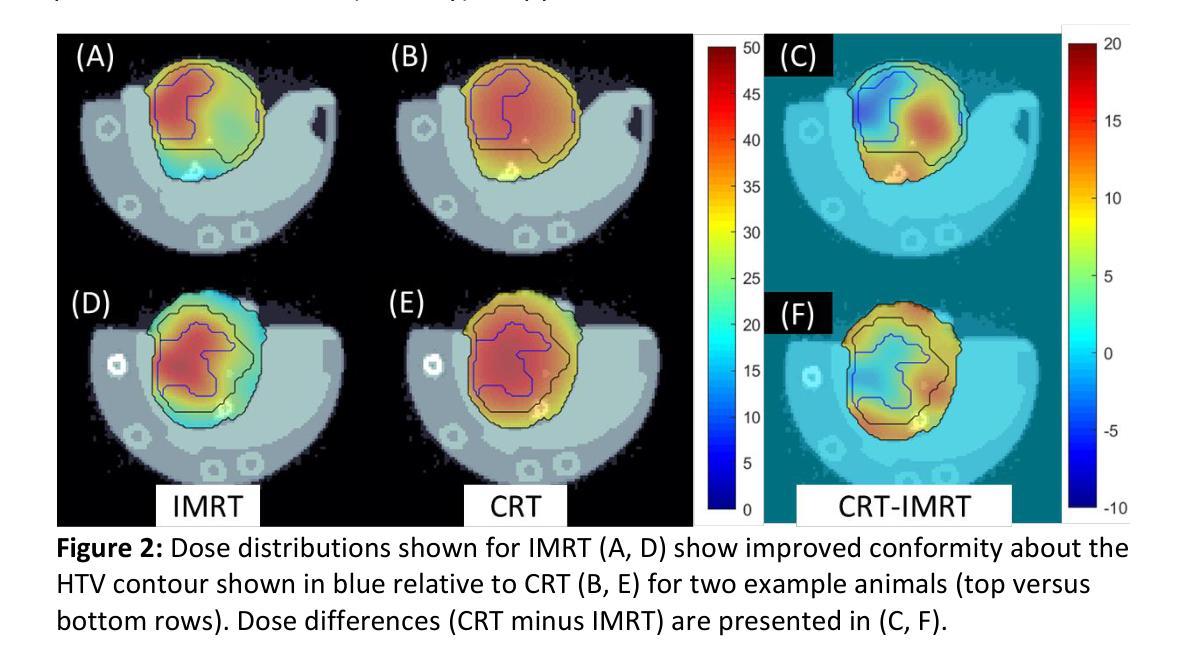
OCSU: Optical Chemical Structure Understanding for Molecule-centric Scientific Discovery
Authors:Siqi Fan, Yuguang Xie, Bowen Cai, Ailin Xie, Gaochao Liu, Mu Qiao, Jie Xing, Zaiqing Nie
Understanding the chemical structure from a graphical representation of a molecule is a challenging image caption task that would greatly benefit molecule-centric scientific discovery. Variations in molecular images and caption subtasks pose a significant challenge in both image representation learning and task modeling. Yet, existing methods only focus on a specific caption task that translates a molecular image into its graph structure, i.e., OCSR. In this paper, we propose the Optical Chemical Structure Understanding (OCSU) task, which extends OCSR to molecular image caption from motif level to molecule level and abstract level. We present two approaches for that, including an OCSR-based method and an end-to-end OCSR-free method. The proposed Double-Check achieves SOTA OCSR performance on real-world patent and journal article scenarios via attentive feature enhancement for local ambiguous atoms. Cascading with SMILES-based molecule understanding methods, it can leverage the power of existing task-specific models for OCSU. While Mol-VL is an end-to-end optimized VLM-based model. An OCSU dataset, Vis-CheBI20, is built based on the widely used CheBI20 dataset for training and evaluation. Extensive experimental results on Vis-CheBI20 demonstrate the effectiveness of the proposed approaches. Improving OCSR capability can lead to a better OCSU performance for OCSR-based approach, and the SOTA performance of Mol-VL demonstrates the great potential of end-to-end approach.
从分子的图形表示理解其化学结构是一项具有挑战性的图像描述任务,对于以分子为中心的科学发现将大有裨益。分子图像和描述子任务的差异对图像表示学习和任务建模都构成了重大挑战。然而,现有方法只关注特定的描述任务,即将分子图像转化为其图形结构,即OCSR(光学化学结构识别)。在本文中,我们提出了光学化学结构理解(OCSU)任务,它扩展了OCSR,从动机水平到分子水平和抽象水平进行分子图像描述。我们为此提出了两种方法,包括基于OCSR的方法和端到端的无OCSR方法。所提出的一种名为“双重检查”的方法通过局部模糊原子的特征增强达到了现实世界专利和期刊文章场景中的SOTA OCSR性能。与SMILES基于分子的理解方法相结合,它可以利用现有特定任务的模型进行OCSU。而Mol-VL则是一个基于VLM的端到端优化模型。基于广泛使用的CheBI20数据集,构建了用于训练和评估的OCSU数据集Vis-CheBI20。在Vis-CheBI20上的大量实验结果表明了所提出方法的有效性。提高OCSR能力可以提高基于OCSR的方法的OCSU性能,而Mol-VL的SOTA性能表明了端到端方法的巨大潜力。
论文及项目相关链接
Summary
本文提出了光学化学结构理解(OCSU)任务,扩展了分子图像字幕任务(OCSR)从动机水平到分子水平和抽象水平。为此,提出了两种新方法:基于OCSR的方法和端到端的OCSR自由方法。通过关注局部模糊原子的注意力特征增强,Double-Check在现实世界专利和期刊文章场景中实现了先进的OCSR性能。与SMILES基础上的分子理解方法相结合,它可以利用现有特定任务的模型进行OCSU。同时,基于广泛使用的CheBI20数据集构建了用于训练和评估的OCSU数据集Vis-CheBI20。在Vis-CheBI20上的大量实验证明了所提出方法的有效性。
Key Takeaways
- 光学化学结构理解(OCSU)任务被提出,旨在从分子图像中理解化学结构,扩展了现有的分子图像字幕任务(OCSR)。
- OCSU任务分为三个层次:动机水平、分子水平和抽象水平。
- 提出了两种完成OCSU任务的新方法:基于OCSR的方法和端到端的OCSR自由方法。
- Double-Check方法通过关注局部模糊原子,实现了在现实世界场景中的先进OCSR性能。
- Double-Check可以与SMILES基础上的分子理解方法结合,利用现有特定任务的模型进行OCSU。
- 构建了用于训练和评估的OCSU数据集Vis-CheBI20,基于广泛使用的CheBI20数据集。
点此查看论文截图
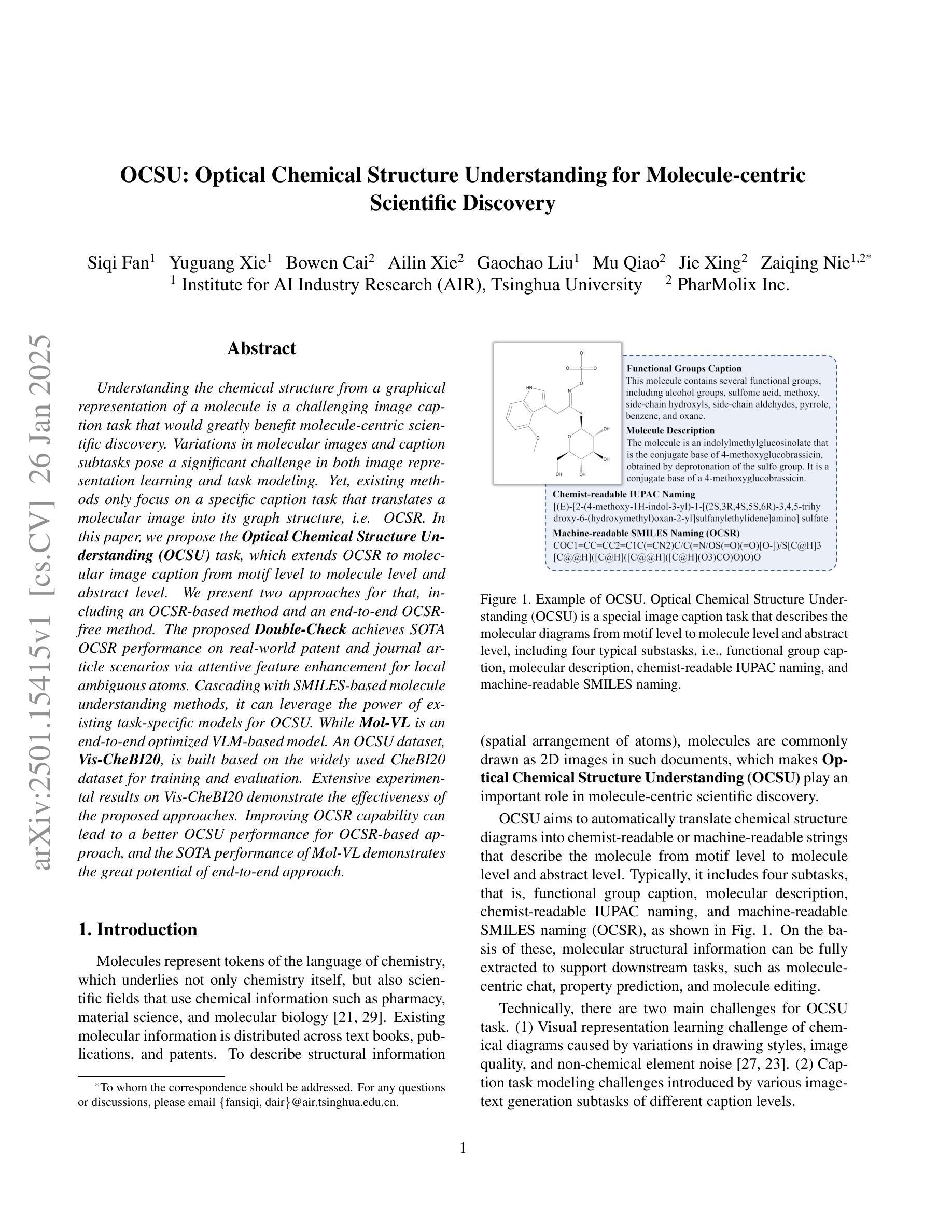
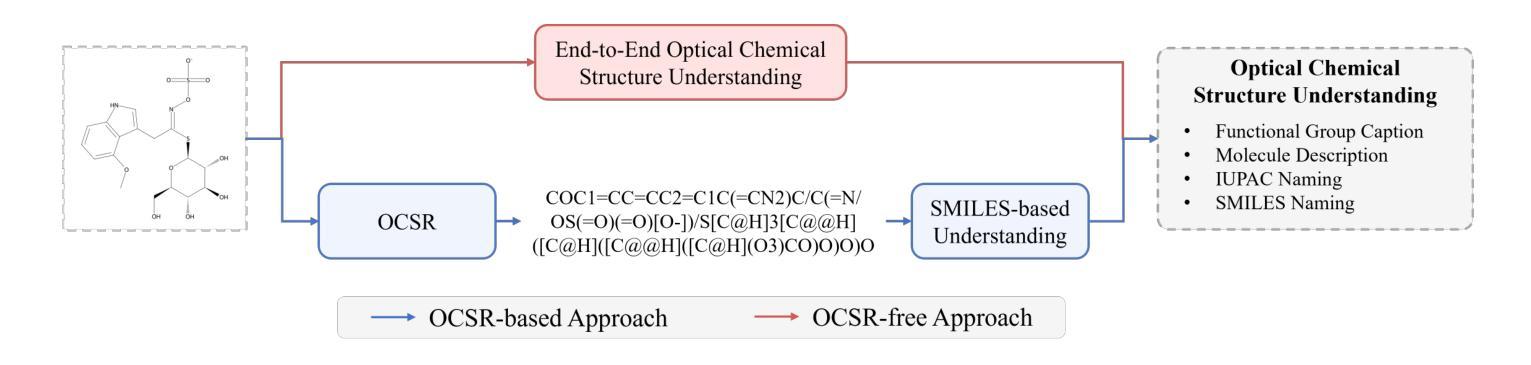
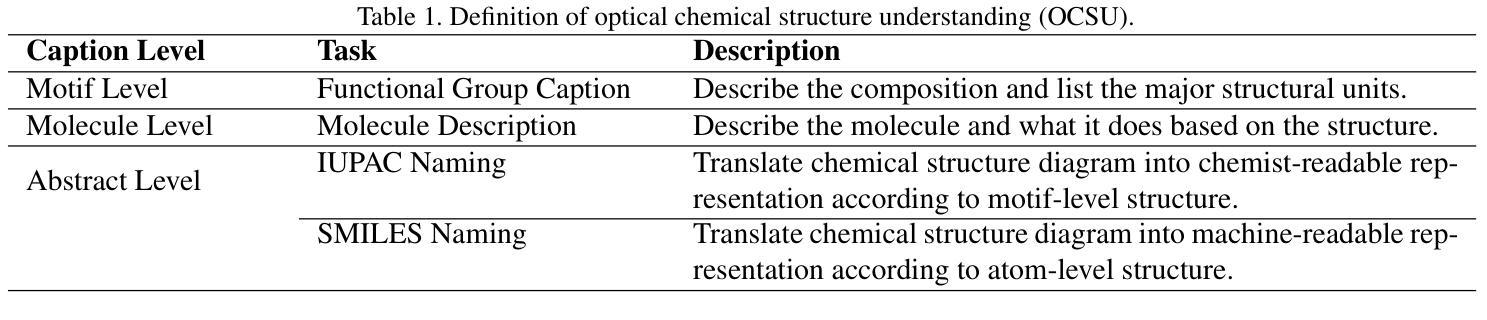
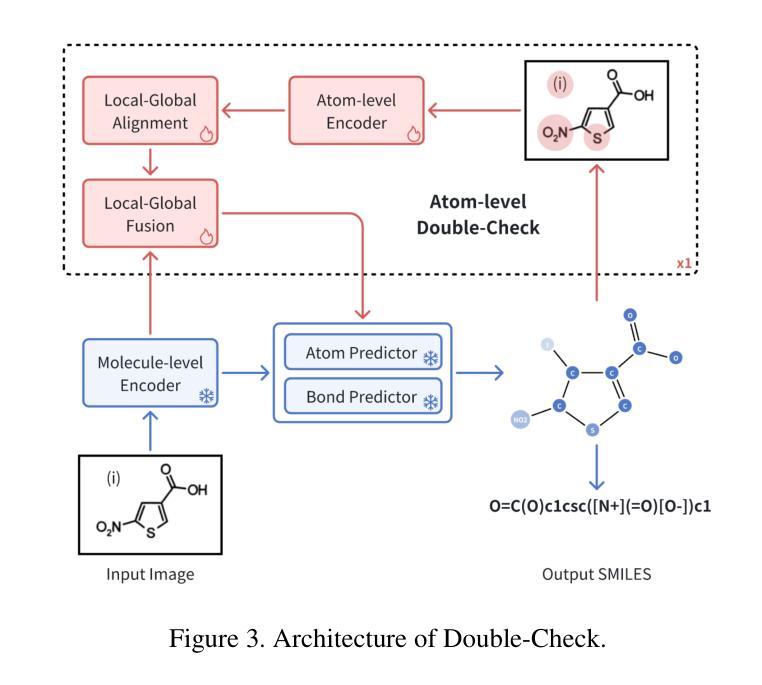
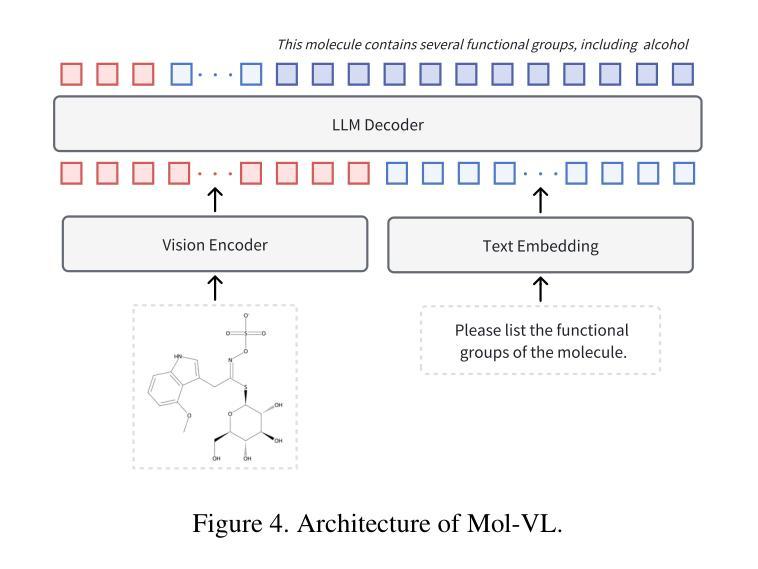
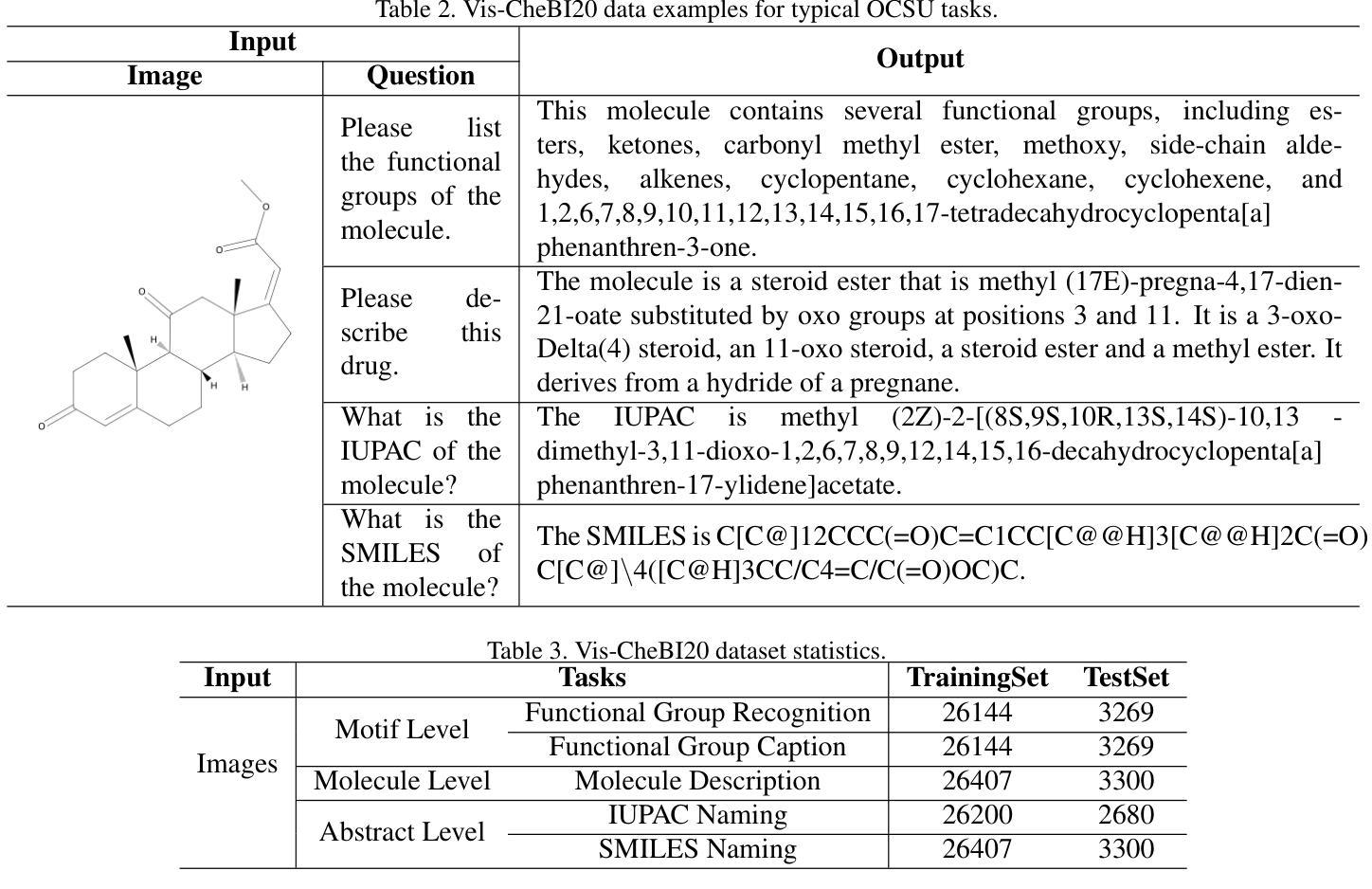
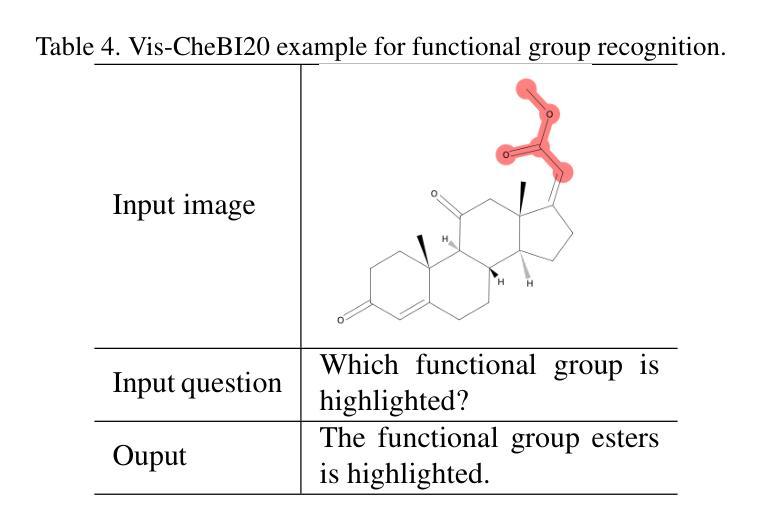
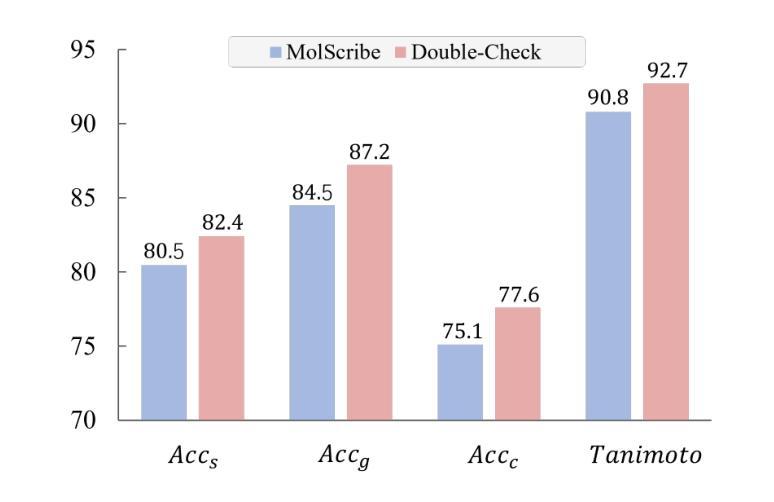
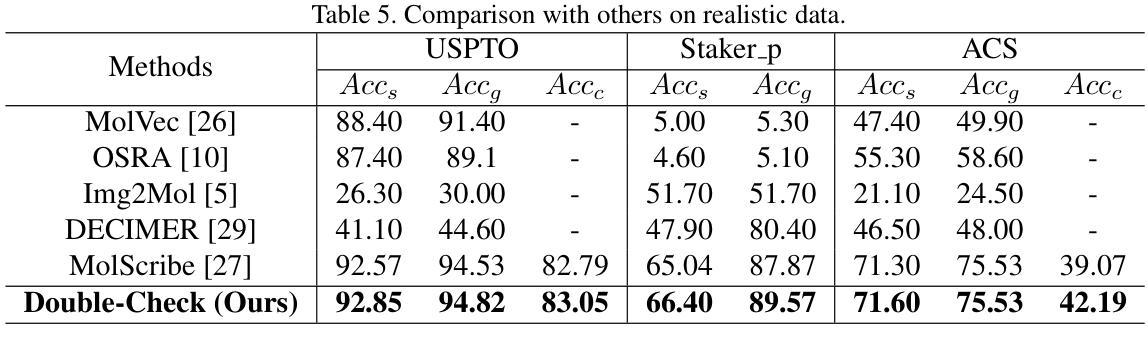
HECLIP: Histology-Enhanced Contrastive Learning for Imputation of Transcriptomics Profiles
Authors:Qing Wang, Wen-jie Chen, Bo Li, Jing Su, Guangyu Wang, Qianqian Song
Histopathology, particularly hematoxylin and eosin (H&E) staining, plays a critical role in diagnosing and characterizing pathological conditions by highlighting tissue morphology. However, H&E-stained images inherently lack molecular information, requiring costly and resource-intensive methods like spatial transcriptomics to map gene expression with spatial resolution. To address these challenges, we introduce HECLIP (Histology-Enhanced Contrastive Learning for Imputation of Profiles), an innovative deep learning framework that bridges the gap between histological imaging and molecular profiling. HECLIP is specifically designed to infer gene expression profiles directly from H&E-stained images, eliminating the need for expensive spatial transcriptomics assays. HECLIP leverages an advanced image-centric contrastive loss function to optimize image representation learning, ensuring that critical morphological patterns in histology images are effectively captured and translated into accurate gene expression profiles. This design enhances the predictive power of the image modality while minimizing reliance on gene expression data. Through extensive benchmarking on publicly available datasets, HECLIP demonstrates superior performance compared to existing approaches, delivering robust and biologically meaningful predictions. Detailed ablation studies further underscore its effectiveness in extracting molecular insights from histology images. Additionally, HECLIP’s scalable and cost-efficient approach positions it as a transformative tool for both research and clinical applications, driving advancements in precision medicine. The source code for HECLIP is openly available at https://github.com/QSong-github/HECLIP.
组织病理学,特别是苏木精和伊红(H&E)染色,在通过突出组织形态来诊断和表征病理状况方面发挥着关键作用。然而,H&E染色图像本身缺乏分子信息,需要昂贵的空间转录组学等方法以空间分辨率绘制基因表达图谱。为了解决这些挑战,我们引入了HECLIP(用于推断轮廓的组织学增强对比学习,Histology-Enhanced Contrastive Learning for Imputation of Profiles),这是一个创新的深度学习框架,它填补了组织成像和分子轮廓之间的空白。HECLIP专门设计用于直接从H&E染色图像推断基因表达轮廓,从而无需昂贵的空间转录学测定。HECLIP利用先进的以图像为中心的对比损失函数来优化图像表示学习,确保有效地捕获组织图像中的关键形态模式并将其转化为准确的基因表达轮廓。这一设计提高了图像模态的预测能力,同时最大限度地减少对基因表达数据的依赖。在公开数据集上进行广泛的标准测试表明,与现有方法相比,HECLIP具有卓越的性能,能够进行稳健且具有生物学意义的预测。详细的消融研究进一步强调了它从组织图像中提取分子见解的有效性。此外,HECLIP的可扩展性和成本效益使其成为研究和临床应用中的变革性工具,推动精准医学的进步。HECLIP的源代码可公开访问:https://github.com/QSong-github/HECLIP。
论文及项目相关链接
Summary:HECLIP是一个基于深度学习的框架,旨在从H&E染色图像直接推断基因表达谱,无需昂贵的空间转录组学检测。它通过图像对比损失函数优化图像表示学习,能够从组织学图像中提取关键形态模式并准确预测基因表达谱。HECLIP具有出色的性能表现,展示了其在研究和临床应用中作为精准医疗领域突破性工具的潜力。
Key Takeaways:
- HECLIP框架成功实现从H&E染色图像推断基因表达谱,避免了昂贵的空间转录组学检测需求。
- HECLIP利用先进的图像对比损失函数优化图像表示学习,确保准确捕捉和翻译组织学图像中的关键形态模式。
- 该框架在公开数据集上的表现优于现有方法,提供了稳健且具有生物学意义的预测。
- 消融研究进一步证明了HECLIP从组织学图像中提取分子见解的有效性。
- HECLIP的可扩展性和成本效益使其成为研究和临床应用的变革性工具。
- HECLIP的源代码已公开可用,便于进一步研究和应用。
点此查看论文截图
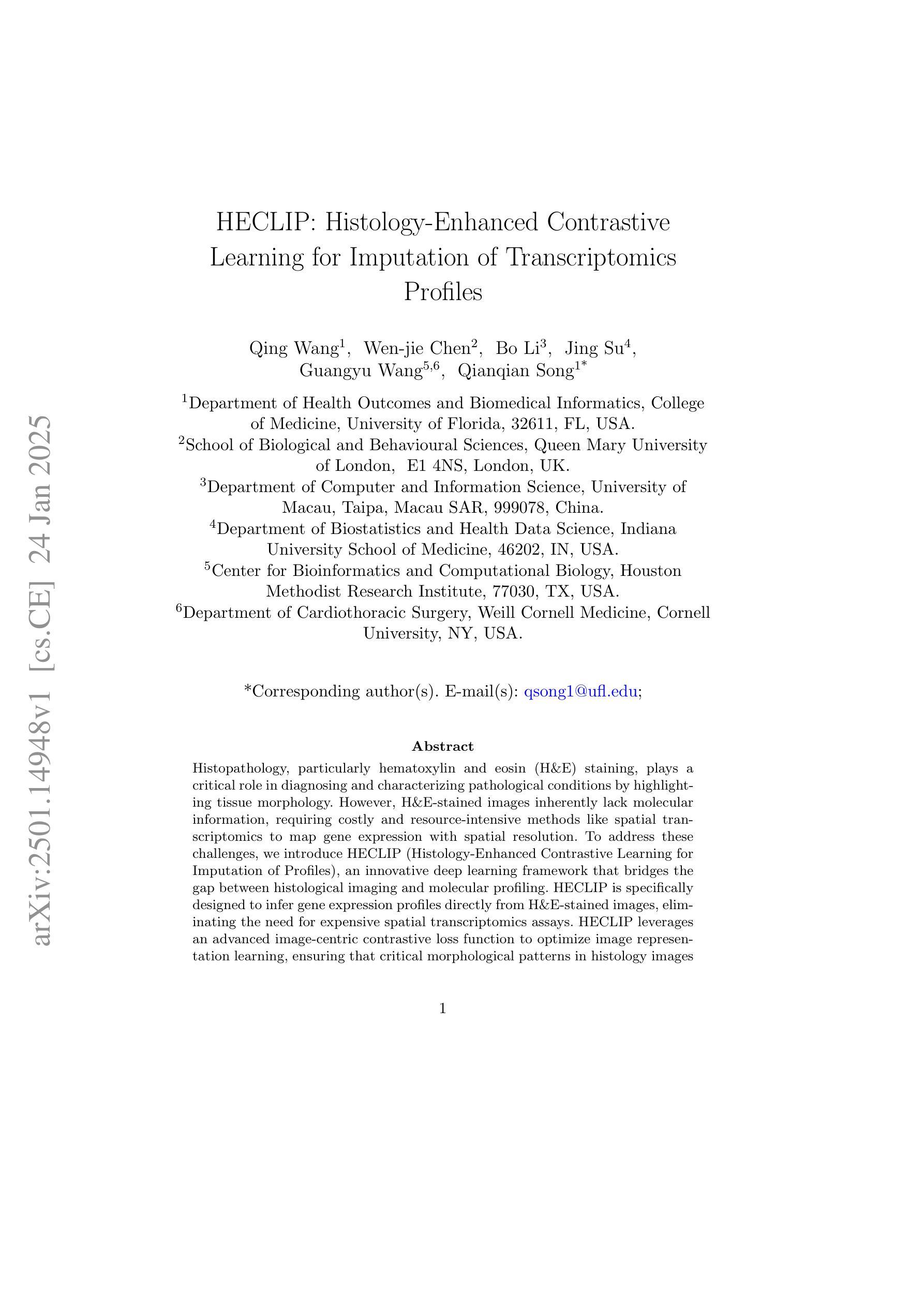
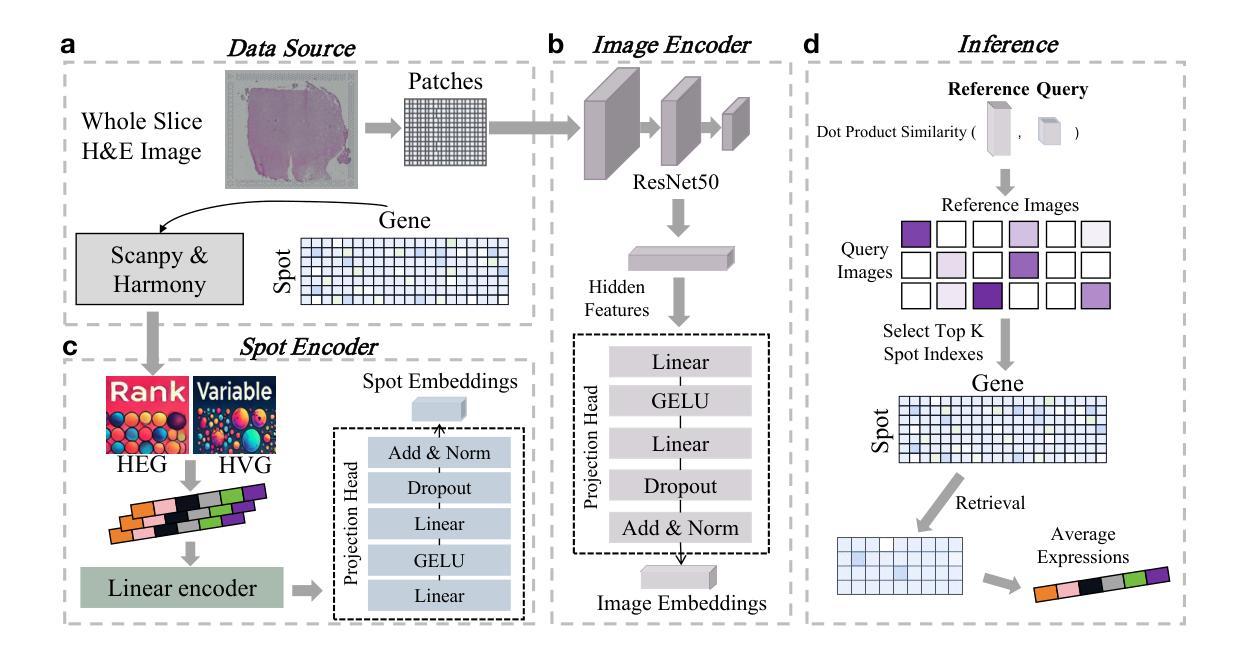
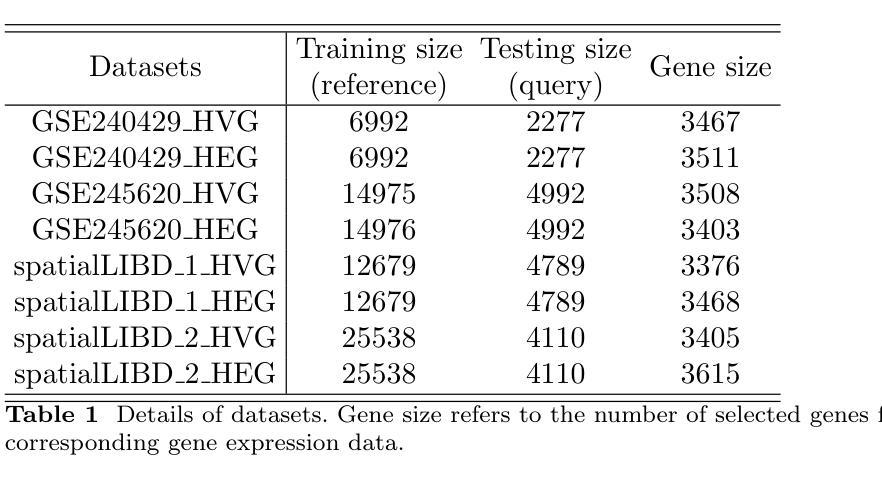
Plasma-CycleGAN: Plasma Biomarker-Guided MRI to PET Cross-modality Translation Using Conditional CycleGAN
Authors:Yanxi Chen, Yi Su, Celine Dumitrascu, Kewei Chen, David Weidman, Richard J Caselli, Nicholas Ashton, Eric M Reiman, Yalin Wang
Cross-modality translation between MRI and PET imaging is challenging due to the distinct mechanisms underlying these modalities. Blood-based biomarkers (BBBMs) are revolutionizing Alzheimer’s disease (AD) detection by identifying patients and quantifying brain amyloid levels. However, the potential of BBBMs to enhance PET image synthesis remains unexplored. In this paper, we performed a thorough study on the effect of incorporating BBBM into deep generative models. By evaluating three widely used cross-modality translation models, we found that BBBMs integration consistently enhances the generative quality across all models. By visual inspection of the generated results, we observed that PET images generated by CycleGAN exhibit the best visual fidelity. Based on these findings, we propose Plasma-CycleGAN, a novel generative model based on CycleGAN, to synthesize PET images from MRI using BBBMs as conditions. This is the first approach to integrate BBBMs in conditional cross-modality translation between MRI and PET.
跨模态MRI和PET成像之间的转换是一个挑战,因为它们基于的机制不同。血液生物标志物(BBBMs)正在通过识别患者并量化脑淀粉样蛋白水平来革新阿尔茨海默病(AD)的检测。然而,BBBM增强PET图像合成的潜力尚未被探索。在本文中,我们对将BBBM纳入深度生成模型进行了彻底的研究。通过对三种常用的跨模态转换模型进行评估,我们发现BBBM的集成在所有模型中均提高了生成质量。通过对生成结果的视觉检查,我们观察到CycleGAN生成的PET图像具有最佳的视觉保真度。基于这些发现,我们提出了基于CycleGAN的血浆CycleGAN模型(Plasma-CycleGAN),使用BBBM作为条件合成PET图像从MRI转化。这是MRI和PET之间的条件跨模态转换中首次整合BBBMs的方法。
论文及项目相关链接
PDF Accepted by ISBI 2025
Summary
本文探索了将血液生物标志物(BBBMs)融入深度生成模型以增强PET图像合成的效果。研究发现在三种常用的跨模态转换模型中,融入BBBMs能持续提升生成质量。通过对比,使用CycleGAN生成的PET图像具有最佳视觉保真度。基于此,提出将BBBMs融入条件跨模态转换的Plasma-CycleGAN模型,用于从MRI合成PET图像。
Key Takeaways
- 跨模态翻译在MRI和PET成像之间存在挑战,因这两种方式背后的机制截然不同。
- 血液生物标志物(BBBMs)在识别阿尔茨海默症(AD)患者和量化脑淀粉样蛋白水平方面实现了革命性的突破。
- BBBMs在增强PET图像合成方面的潜力尚未被探索。
- 研究发现,将BBBMs融入深度生成模型能持续提升生成质量。
- CycleGAN生成的PET图像具有最佳视觉保真度。
- 提出了结合BBBMs的Plasma-CycleGAN模型,这是一种基于CycleGAN的新型生成模型,可从MRI合成PET图像。
点此查看论文截图
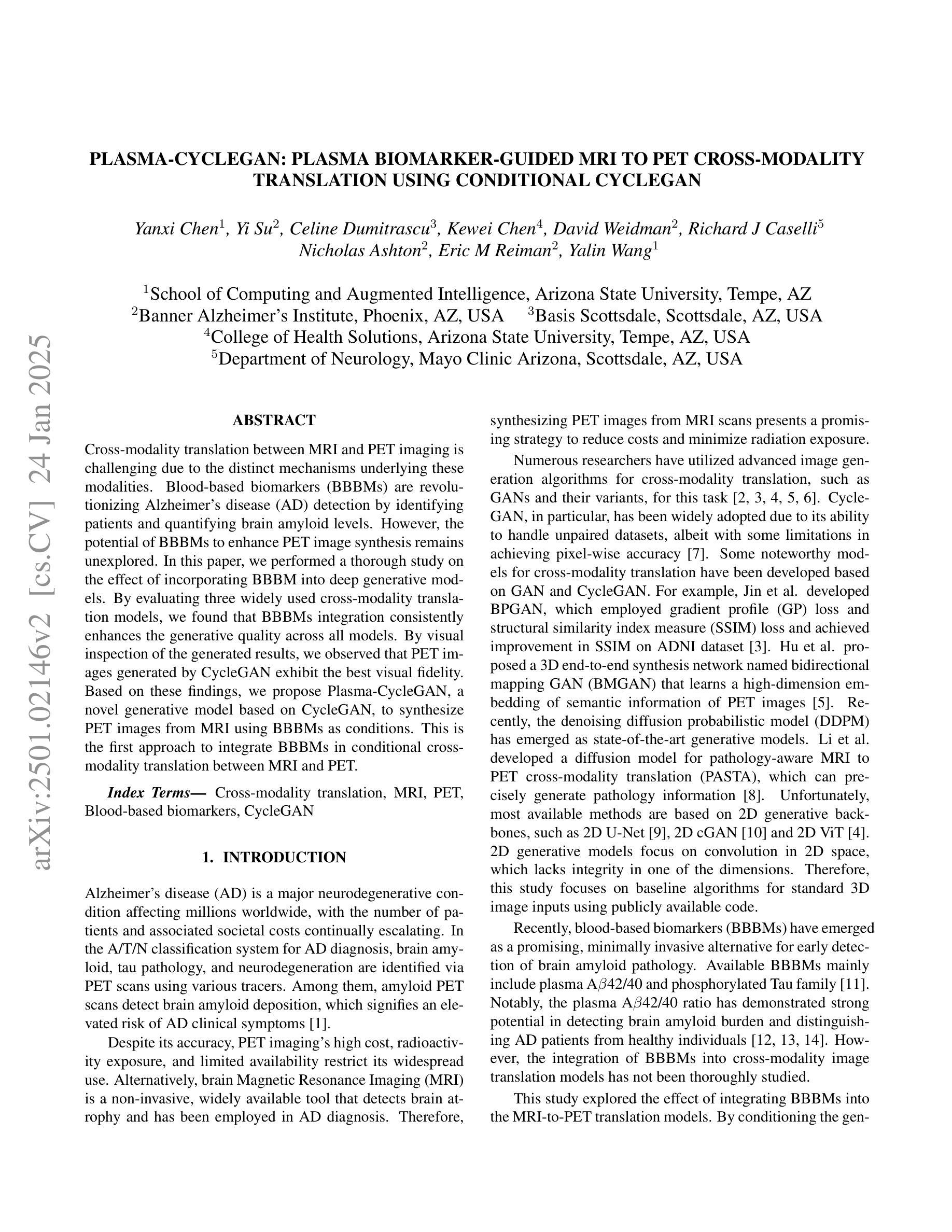
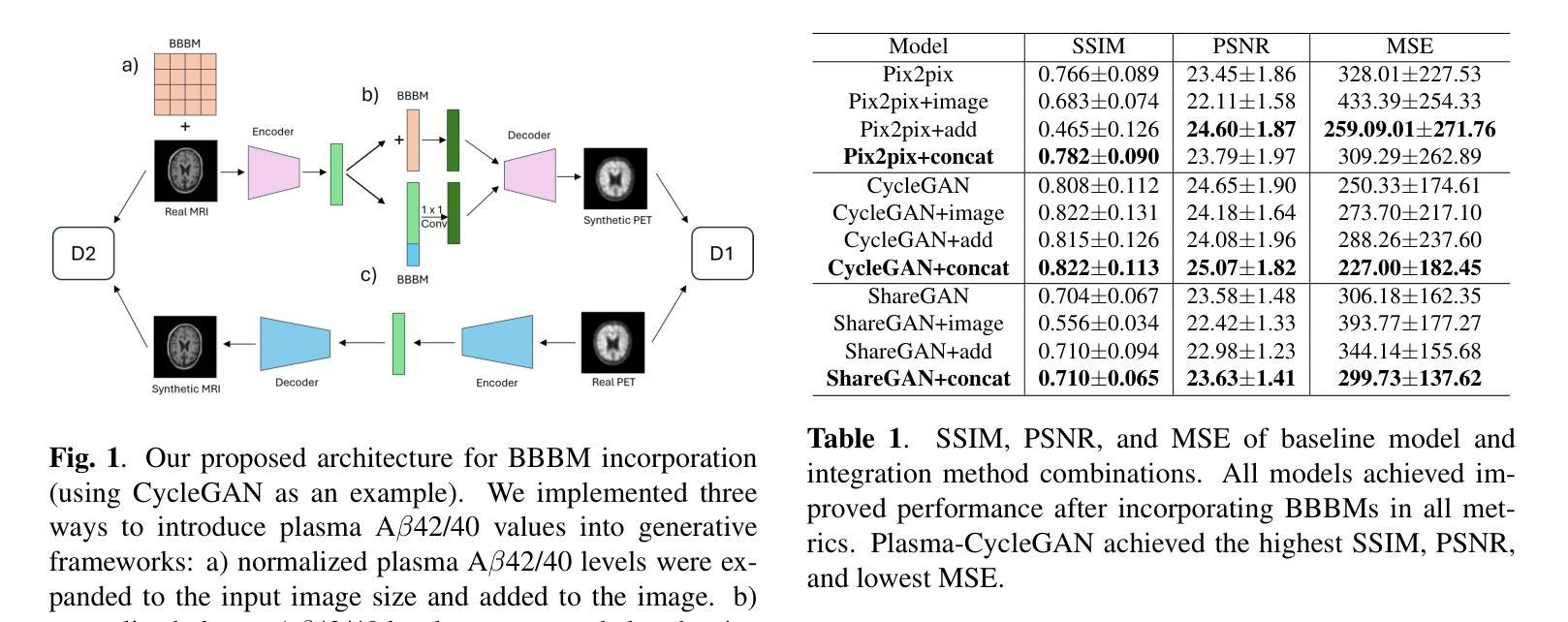
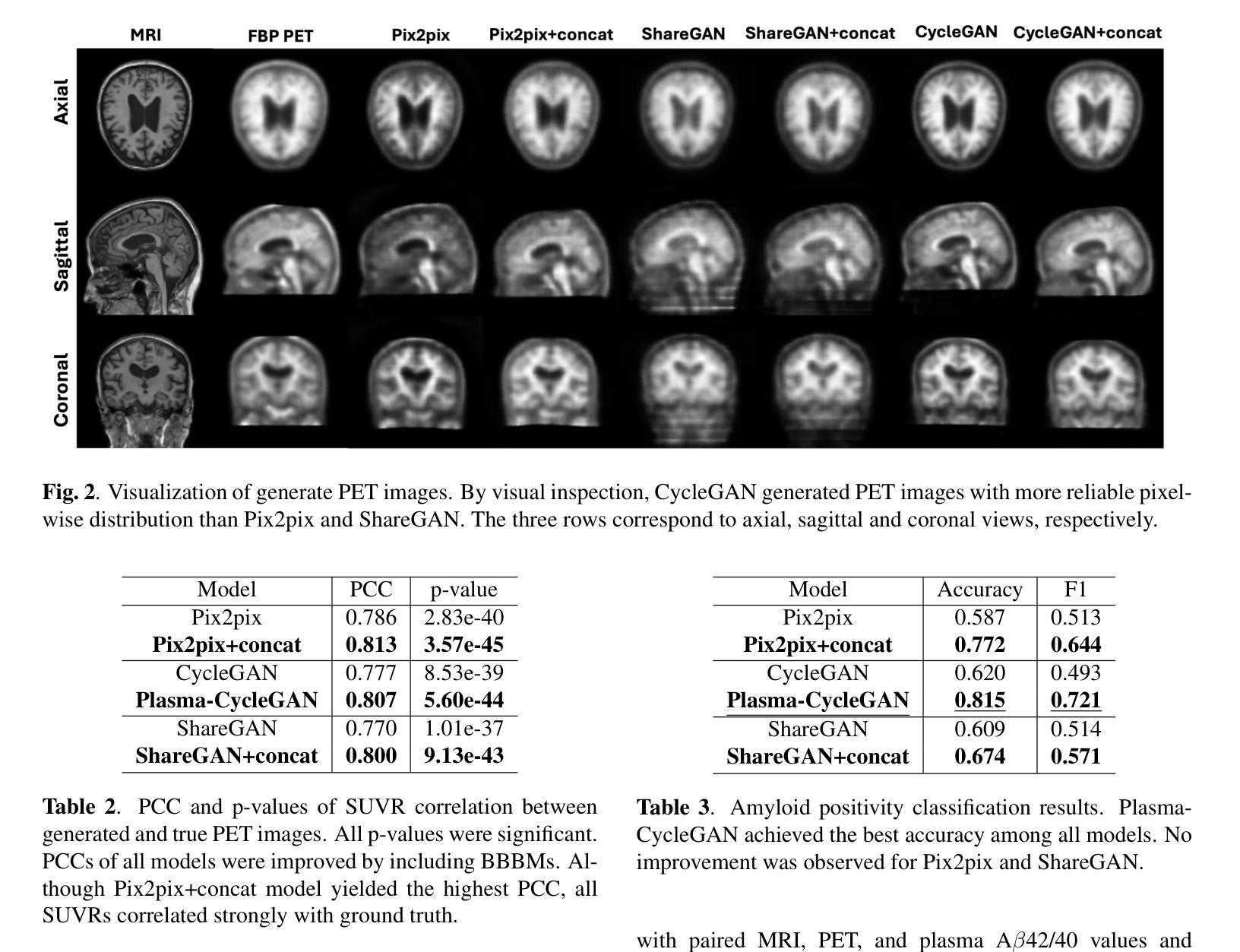
SAMScore: A Content Structural Similarity Metric for Image Translation Evaluation
Authors:Yunxiang Li, Meixu Chen, Kai Wang, Jun Ma, Alan C. Bovik, You Zhang
Image translation has wide applications, such as style transfer and modality conversion, usually aiming to generate images having both high degrees of realism and faithfulness. These problems remain difficult, especially when it is important to preserve content structures. Traditional image-level similarity metrics are of limited use, since the content structures of an image are high-level, and not strongly governed by pixel-wise faithfulness to an original image. To fill this gap, we introduce SAMScore, a generic content structural similarity metric for evaluating the faithfulness of image translation models. SAMScore is based on the recent high-performance Segment Anything Model (SAM), which allows content similarity comparisons with standout accuracy. We applied SAMScore on 19 image translation tasks, and found that it is able to outperform all other competitive metrics on all tasks. We envision that SAMScore will prove to be a valuable tool that will help to drive the vibrant field of image translation, by allowing for more precise evaluations of new and evolving translation models. The code is available at https://github.com/Kent0n-Li/SAMScore.
图像翻译有着广泛的应用,例如风格转换和模态转换,其通常旨在生成具有高度真实感和保真度的图像。这些问题仍然很难解决,尤其是在保留内容结构方面。传统的图像级相似性度量效果有限,因为图像的内容结构是高层次的,并不强烈依赖于对原始图像的像素级忠实度。为了填补这一空白,我们引入了SAMScore,这是一个通用的内容结构相似性度量标准,用于评估图像翻译模型的忠实度。SAMScore基于最近的高性能Segment Anything Model(SAM),该模型允许进行准确的内容相似性比较。我们在19个图像翻译任务上应用了SAMScore,发现它在所有任务上的表现都优于其他所有竞争度量标准。我们预期,SAMScore将成为一个有价值的工具,帮助推动图像翻译这一充满活力的领域的发展,通过更精确地评估新旧翻译模型,推动技术进步。代码可在https://github.com/Kent0n-Li/SAMScore找到。
论文及项目相关链接
Summary
本文介绍了图像翻译应用广泛,旨在生成高度逼真且忠实度高的图像。针对内容结构保留的重要性,提出SAMScore这一通用内容结构相似性度量标准,用于评估图像翻译模型的忠实度。SAMScore基于高性能的Segment Anything Model(SAM),能够进行准确的内容相似性比较。在19个图像翻译任务上应用SAMScore,表现出超越其他竞争指标的性能。SAMScore有望成为图像翻译领域的宝贵工具,为新的和不断发展的翻译模型提供更精确的评价。
Key Takeaways
- 图像翻译广泛应用于风格转换和模态转换等领域,旨在生成高度逼真且忠实度高的图像。
- 传统图像级别的相似性度量在评估图像翻译模型时效果有限,特别是在保留内容结构方面。
- 引入SAMScore,一个基于Segment Anything Model(SAM)的通用内容结构相似性度量标准,用于评估图像翻译模型的忠实度。
- SAMScore在19个图像翻译任务上的表现优于其他竞争指标。
- SAMScore的推出将有助于推动图像翻译领域的发展,为新的和不断发展的翻译模型提供更精确的评价。
- SAMScore的开源代码可在指定网站获取。
点此查看论文截图
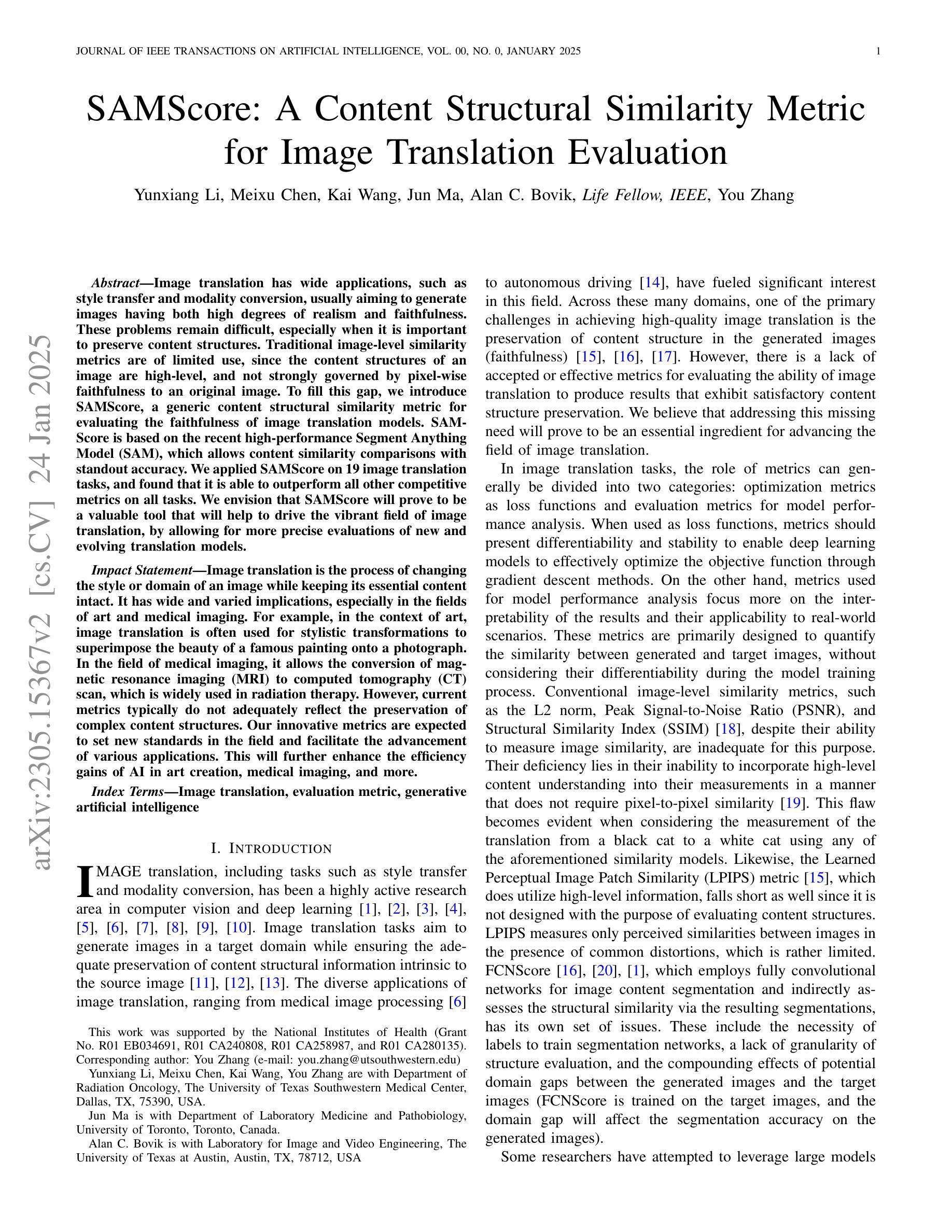
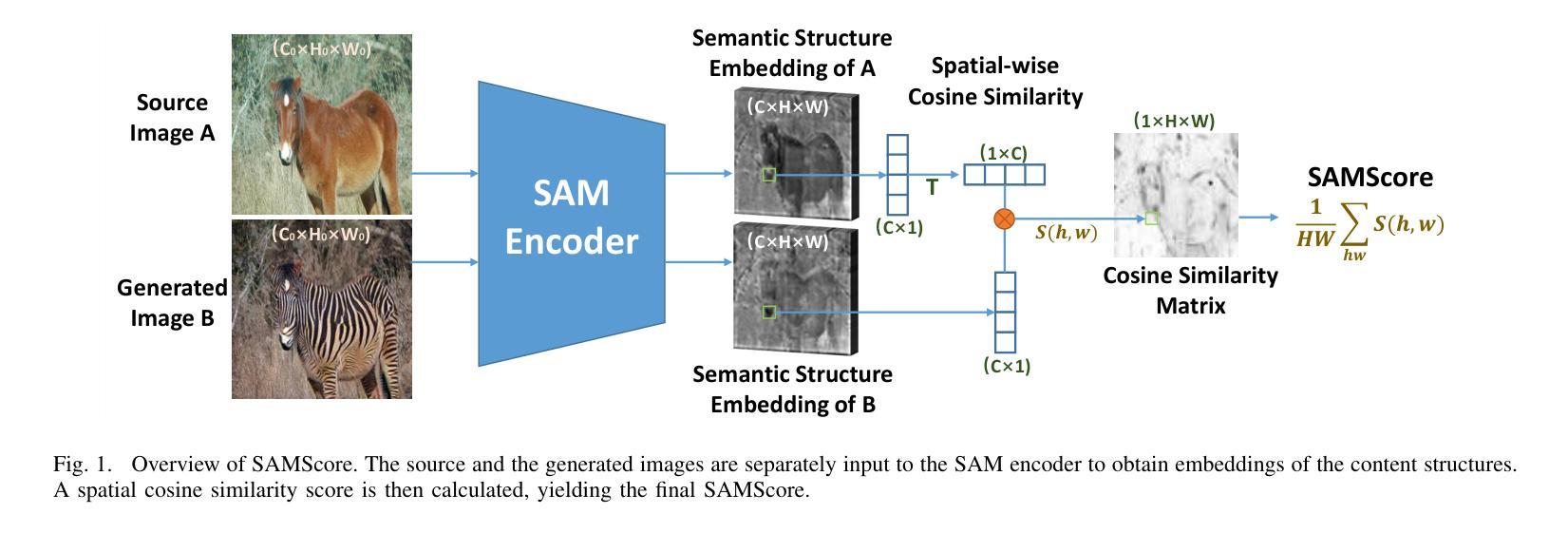
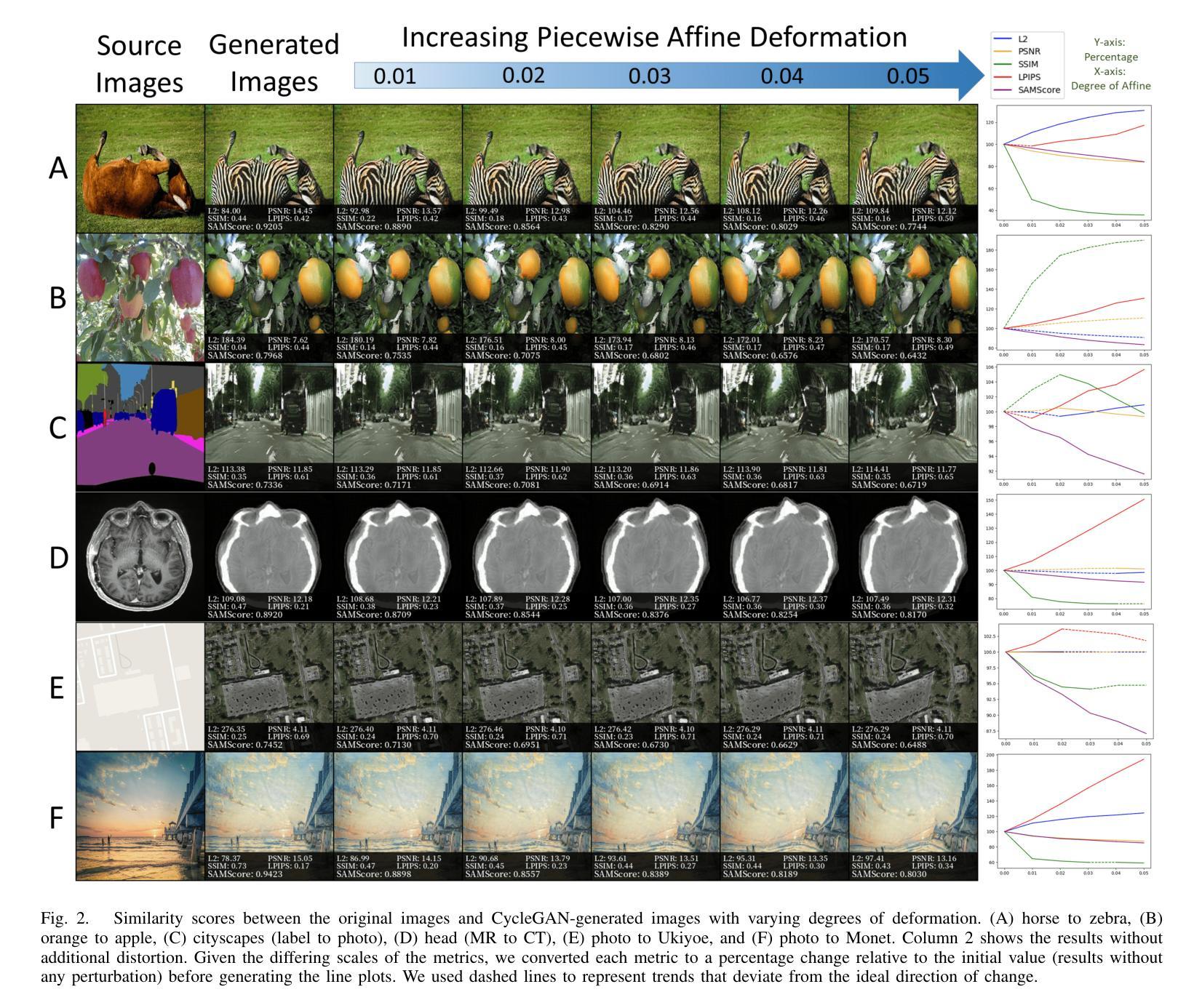
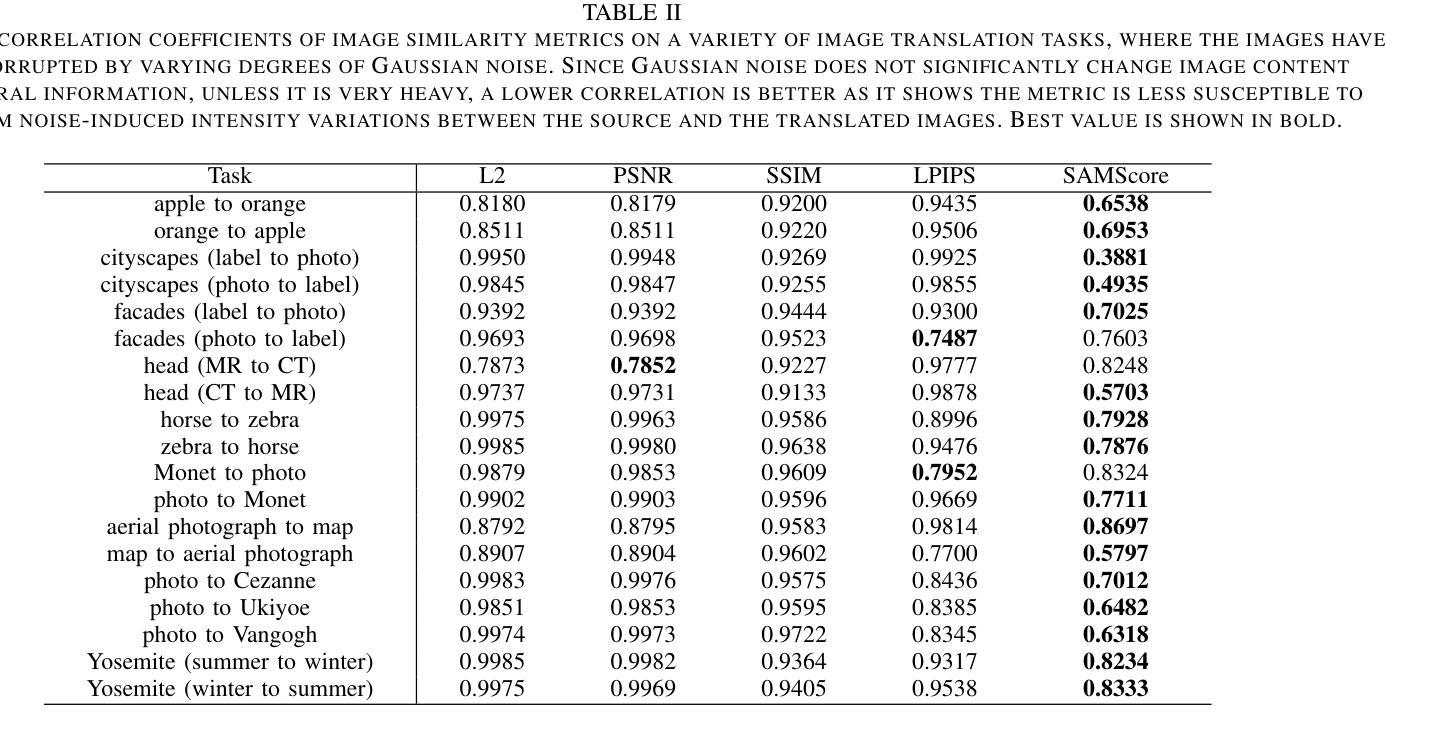
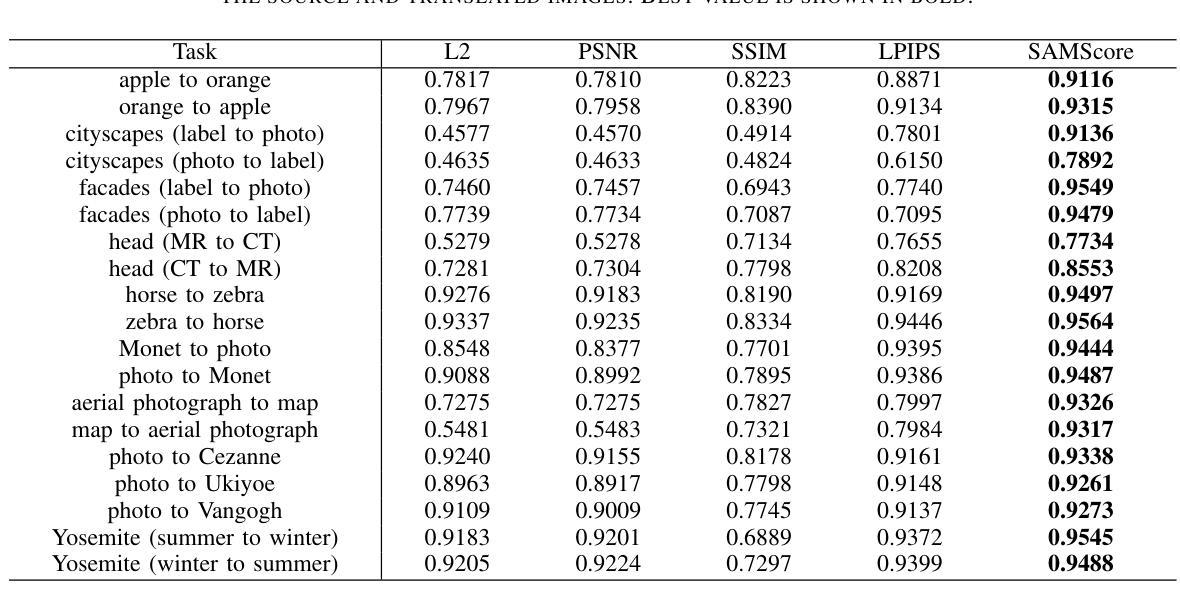
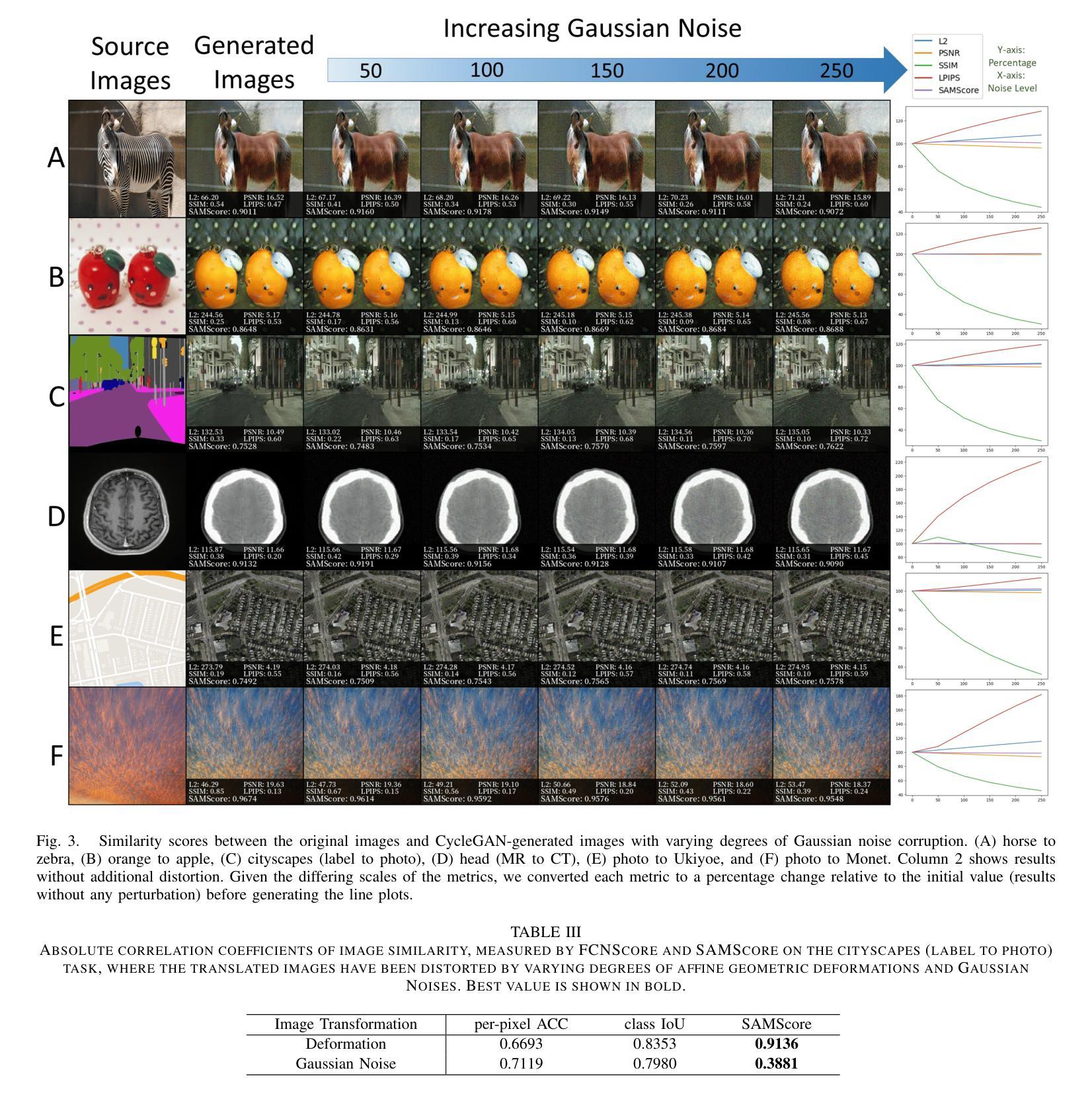