⚠️ 以下所有内容总结都来自于 大语言模型的能力,如有错误,仅供参考,谨慎使用
🔴 请注意:千万不要用于严肃的学术场景,只能用于论文阅读前的初筛!
💗 如果您觉得我们的项目对您有帮助 ChatPaperFree ,还请您给我们一些鼓励!⭐️ HuggingFace免费体验
2025-01-29 更新
KETA: Kinematic-Phrases-Enhanced Text-to-Motion Generation via Fine-grained Alignment
Authors:Yu Jiang, Yixing Chen, Xingyang Li
Motion synthesis plays a vital role in various fields of artificial intelligence. Among the various conditions of motion generation, text can describe motion details elaborately and is easy to acquire, making text-to-motion(T2M) generation important. State-of-the-art T2M techniques mainly leverage diffusion models to generate motions with text prompts as guidance, tackling the many-to-many nature of T2M tasks. However, existing T2M approaches face challenges, given the gap between the natural language domain and the physical domain, making it difficult to generate motions fully consistent with the texts. We leverage kinematic phrases(KP), an intermediate representation that bridges these two modalities, to solve this. Our proposed method, KETA, decomposes the given text into several decomposed texts via a language model. It trains an aligner to align decomposed texts with the KP segments extracted from the generated motions. Thus, it’s possible to restrict the behaviors for diffusion-based T2M models. During the training stage, we deploy the text-KP alignment loss as an auxiliary goal to supervise the models. During the inference stage, we refine our generated motions for multiple rounds in our decoder structure, where we compute the text-KP distance as the guidance signal in each new round. Experiments demonstrate that KETA achieves up to 1.19x, 2.34x better R precision and FID value on both backbones of the base model, motion diffusion model. Compared to a wide range of T2M generation models. KETA achieves either the best or the second-best performance.
运动合成在人工智能的各个领域都扮演着至关重要的角色。在运动生成的多种条件中,文本能够详细地描述运动细节并且易于获取,这使得文本到运动(T2M)的生成至关重要。最先进的T2M技术主要利用扩散模型来生成受文本提示引导的运动,解决T2M任务的多对多性质。然而,现有的T2M方法面临着挑战,因为自然语言域和物理域之间的差距使得生成与文本完全一致的运动变得困难。我们利用运动学短语(KP),一种连接这两种模态的中间表示,来解决这个问题。我们提出的方法KETA通过语言模型将给定文本分解成多个分解文本。它训练一个对齐器,将分解文本与从生成的运动中提取的KP片段对齐。因此,可以限制基于扩散的T2M模型的行为。在训练阶段,我们部署文本-KP对齐损失作为辅助目标来监督模型。在推理阶段,我们在解码器结构中进行了多轮运动生成优化,其中我们将文本-KP距离作为每轮的新指导信号。实验表明,KETA在基础模型的两种主干,即运动扩散模型上,实现了高达1.19倍和2.34倍的R精度和FID值提升。与多种T2M生成模型相比,KETA取得了最佳或第二的最佳性能。
论文及项目相关链接
PDF 7 pages, 5 figures
Summary
文本描述了运动合成在人工智能领域的重要性。现有文本到运动(T2M)生成技术主要利用扩散模型来根据文本提示生成运动,但面临文本与物理领域之间的鸿沟挑战。研究提出了一种新方法KETA,通过利用运动文本中的运动学短语(KP)作为中间表示来连接这两个领域。KETA通过语言模型将给定文本分解为多个分解文本,并通过对齐器与从生成的运动中提取的KP段进行对齐。在训练阶段,部署文本-KP对齐损失作为辅助目标来监督模型。在推理阶段,对生成的动作进行多轮优化,计算文本-KP距离作为每轮的指导信号。实验表明,KETA在基础模型和动作扩散模型的两种骨干网络上实现了高达1.19倍和2.34倍的R精度和FID值提升,相较于一系列T2M生成模型表现出最佳或第二佳性能。
Key Takeaways
- 运动合成在人工智能领域的重要性:文本可以详细描述运动细节,且易于获取,使得文本到运动的生成变得重要。
- 现有T2M技术面临的挑战:自然语言和物理领域的鸿沟导致难以完全根据文本生成一致的运动。
- KETA方法介绍:利用运动学短语(KP)作为中间表示来连接文本和运动的两个领域。
- KETA的工作流程:通过语言模型将文本分解为分解文本,并通过对齐器与KP段对齐;在训练阶段使用文本-KP对齐损失作为辅助目标;在推理阶段进行多轮动作优化,使用文本-KP距离作为指导信号。
- KETA的性能提升:相较于其他T2M生成模型,KETA在R精度和FID值上实现了显著提升。
- 方法创新点:KETA利用文本分解和与KP的对齐来解决T2M任务中的多对多问题,缩小了文本与物理领域的差距。
点此查看论文截图
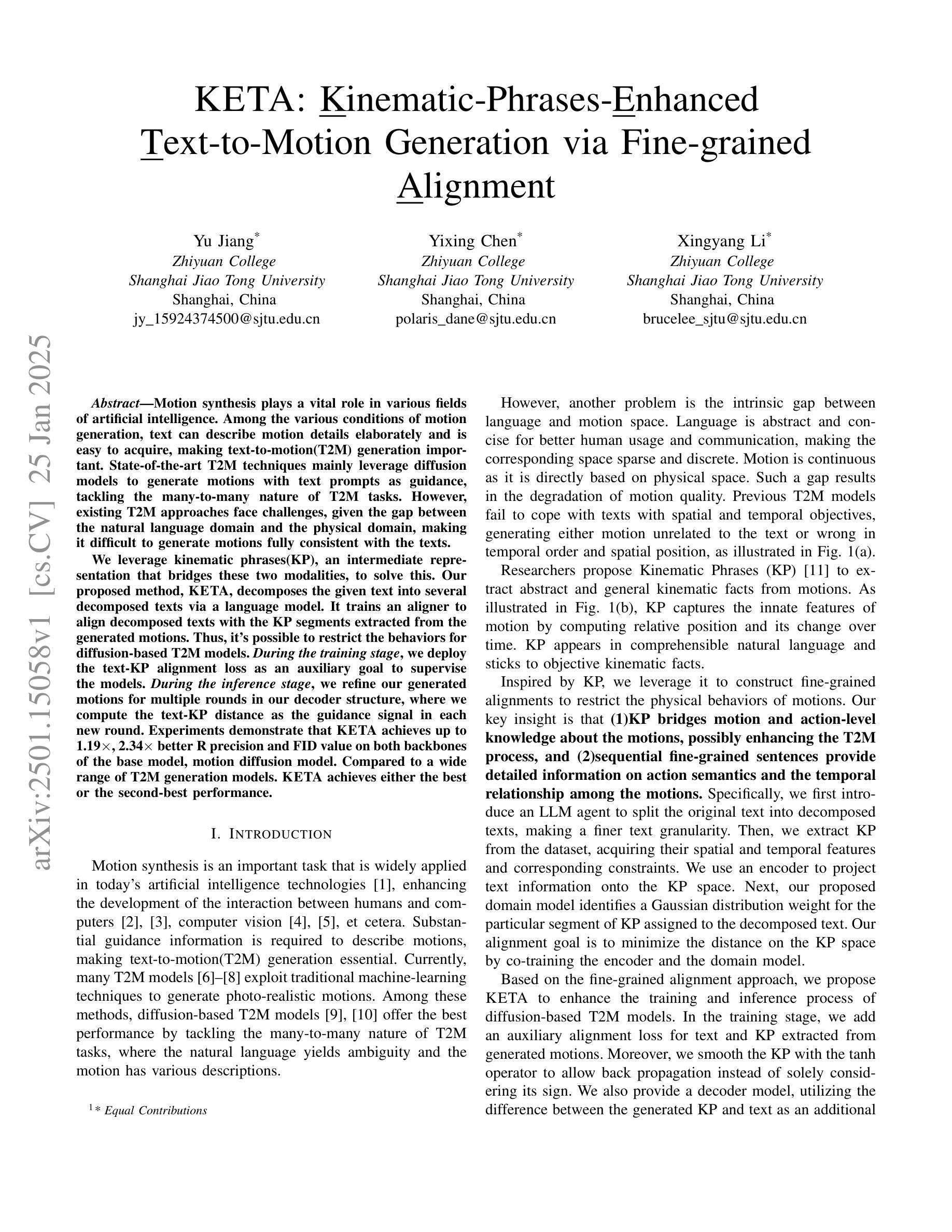
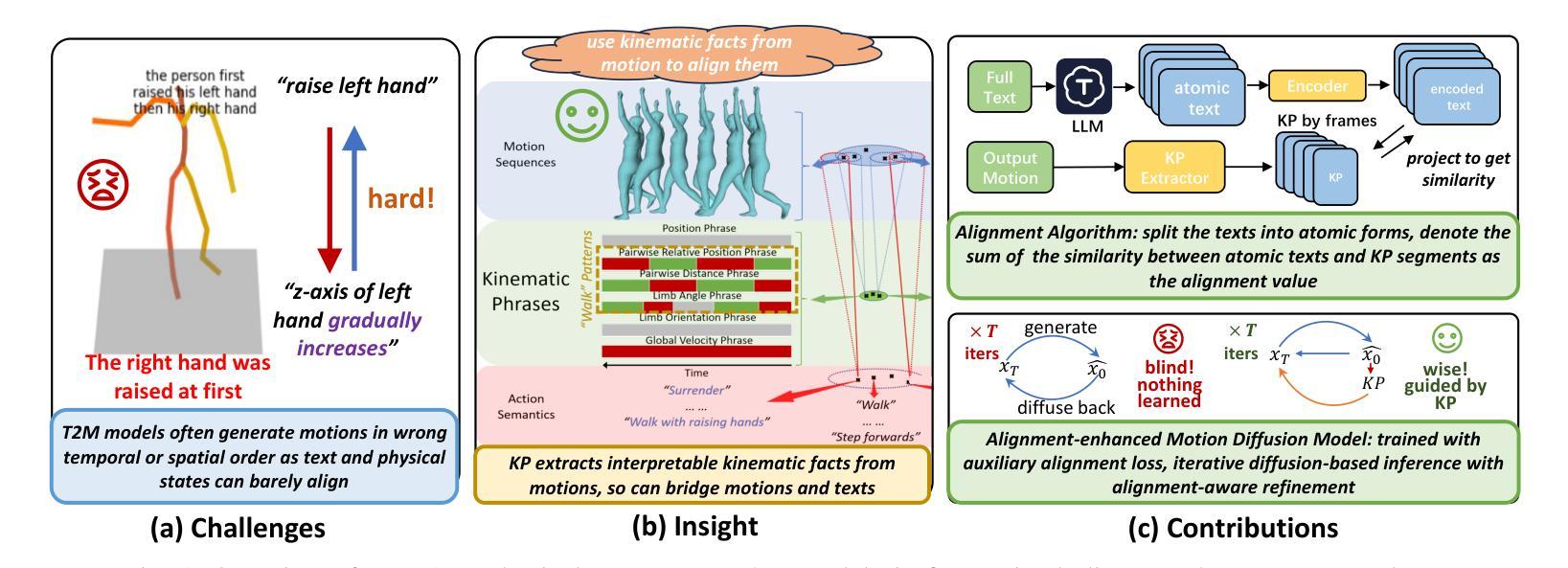
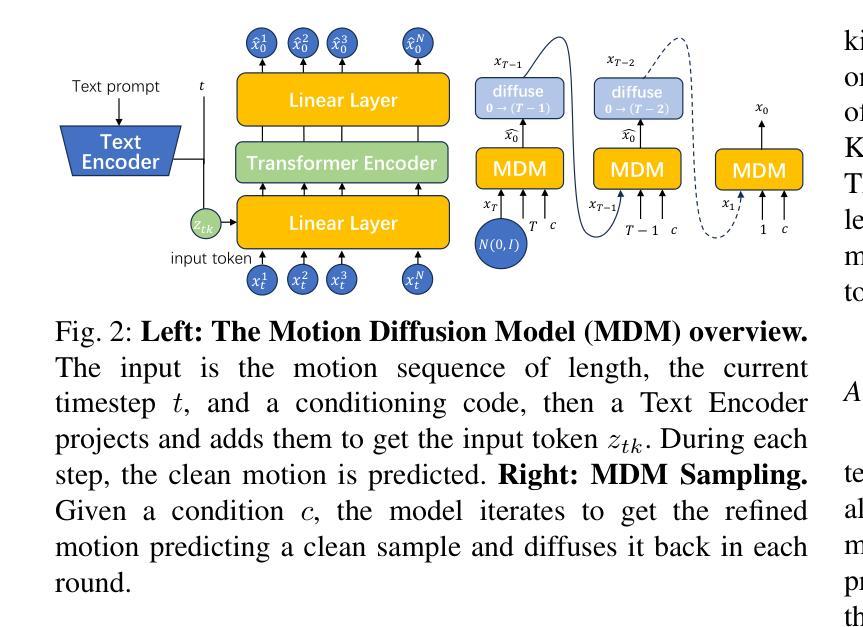
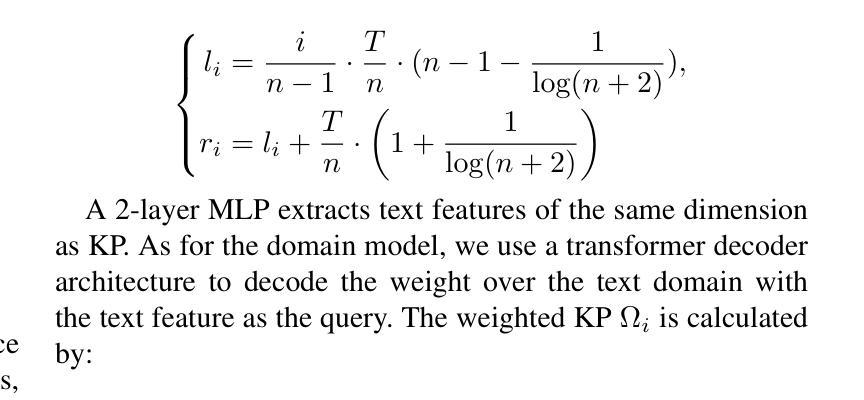
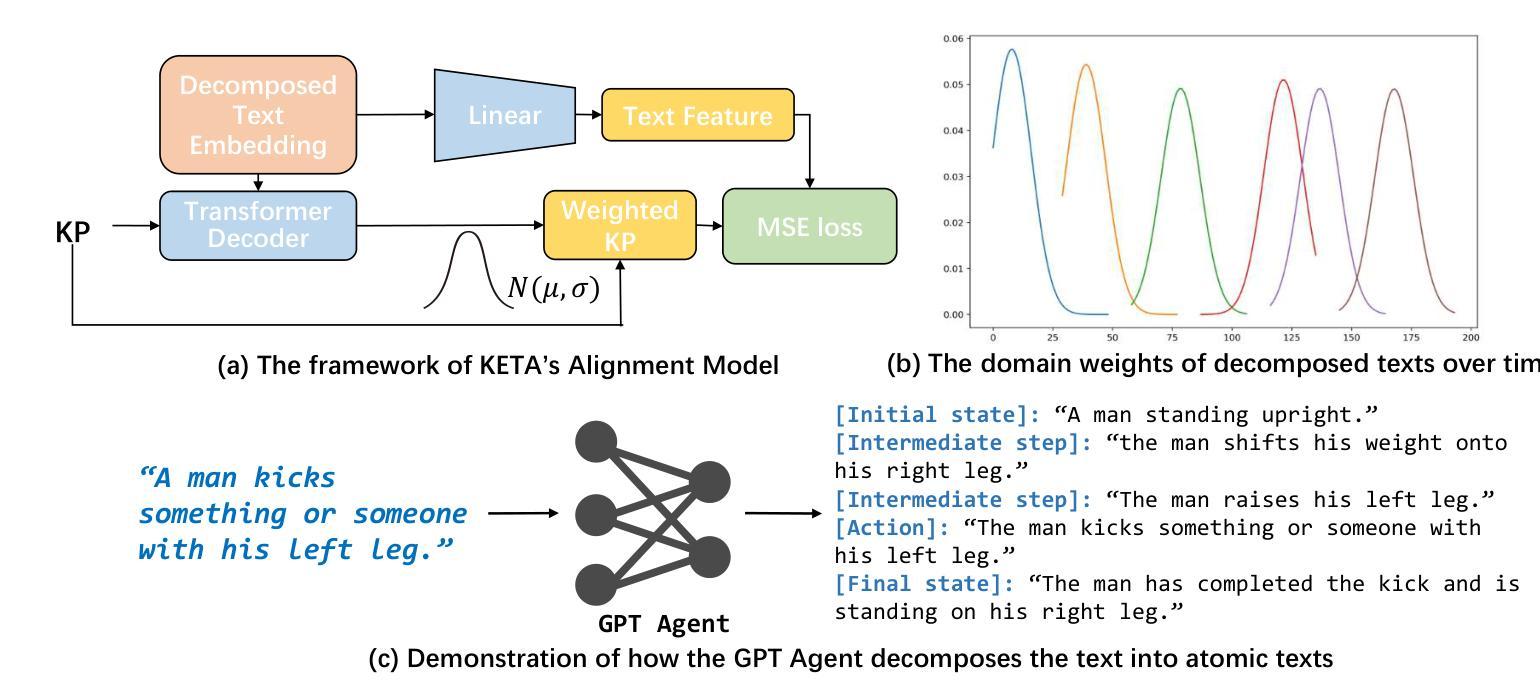
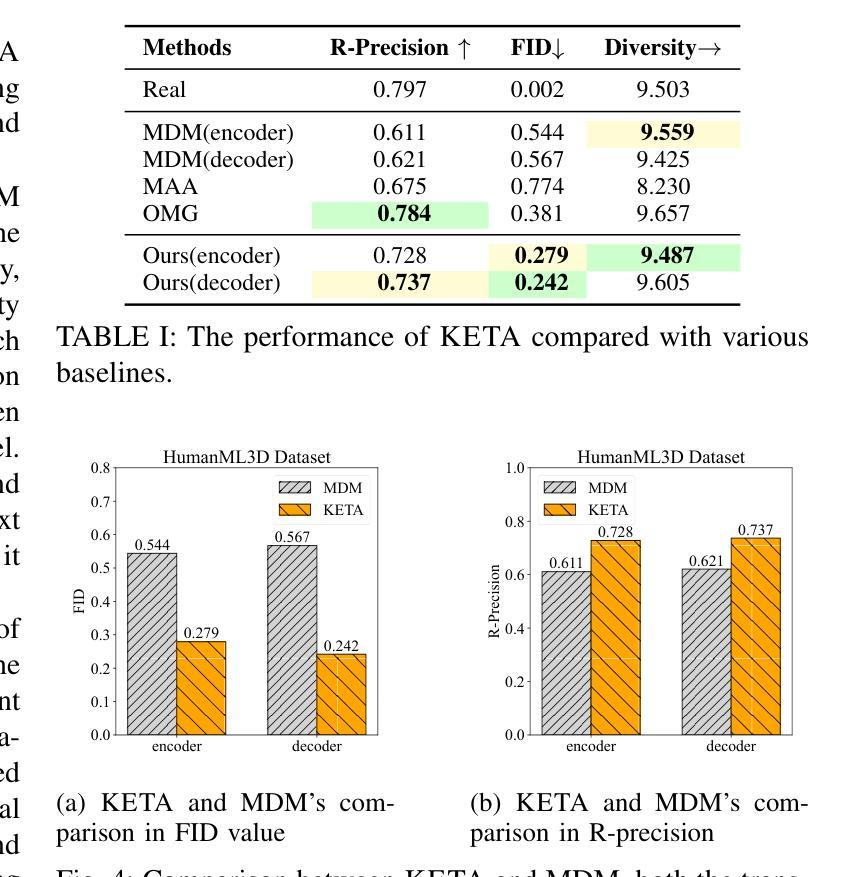
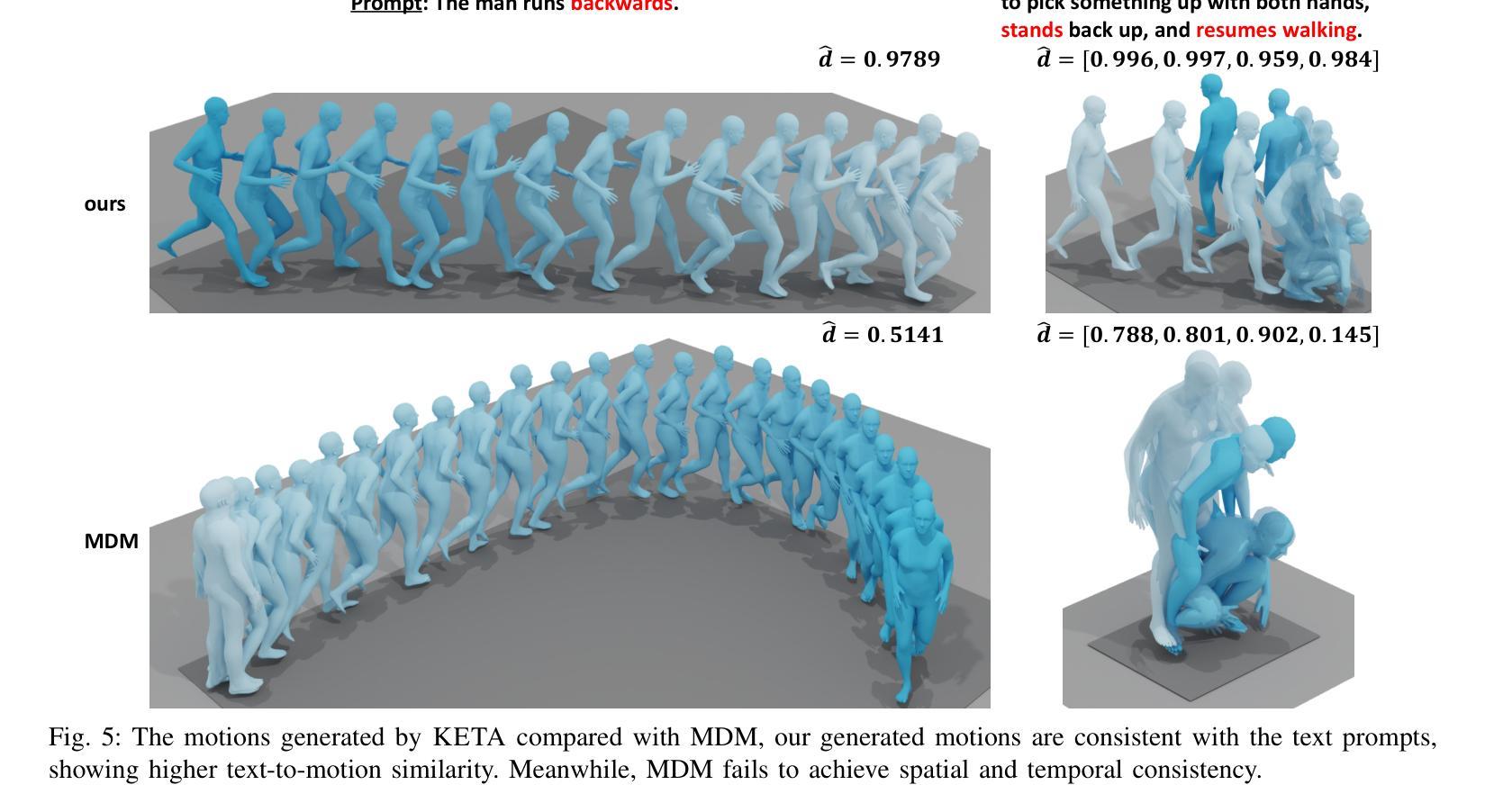
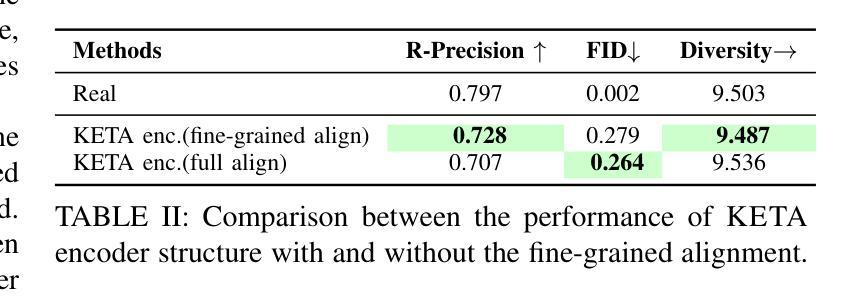