⚠️ 以下所有内容总结都来自于 大语言模型的能力,如有错误,仅供参考,谨慎使用
🔴 请注意:千万不要用于严肃的学术场景,只能用于论文阅读前的初筛!
💗 如果您觉得我们的项目对您有帮助 ChatPaperFree ,还请您给我们一些鼓励!⭐️ HuggingFace免费体验
2025-01-30 更新
CubeDiff: Repurposing Diffusion-Based Image Models for Panorama Generation
Authors:Nikolai Kalischek, Michael Oechsle, Fabian Manhardt, Philipp Henzler, Konrad Schindler, Federico Tombari
We introduce a novel method for generating 360{\deg} panoramas from text prompts or images. Our approach leverages recent advances in 3D generation by employing multi-view diffusion models to jointly synthesize the six faces of a cubemap. Unlike previous methods that rely on processing equirectangular projections or autoregressive generation, our method treats each face as a standard perspective image, simplifying the generation process and enabling the use of existing multi-view diffusion models. We demonstrate that these models can be adapted to produce high-quality cubemaps without requiring correspondence-aware attention layers. Our model allows for fine-grained text control, generates high resolution panorama images and generalizes well beyond its training set, whilst achieving state-of-the-art results, both qualitatively and quantitatively. Project page: https://cubediff.github.io/
我们介绍了一种从文字提示或图像生成360°全景的新方法。我们的方法利用3D生成的最新进展,采用多视角扩散模型联合合成立方体贴图的六个面。与以往依赖于处理等距投影或自回归生成的方法不同,我们的方法将每面视为标准透视图像,简化了生成过程,并使得现有多视角扩散模型的使用成为可能。我们证明,这些模型可以适应产生高质量的立方体贴图,而无需对应感知注意力层。我们的模型允许精细的文本控制,生成高分辨率的全景图像,并且在训练集之外也能很好地推广,同时在定性和定量上达到最新水平的结果。项目页面:https://cubediff.github.io/
论文及项目相关链接
PDF Accepted at ICLR 2025
Summary
本文介绍了一种从文本提示或图像生成360°全景图的新方法。该方法利用最新的3D生成技术,通过采用多视角扩散模型联合合成立方体贴图的六个面。不同于以往依赖等距投影或自回归生成的方法,本文方法将每个面视为标准透视图像,简化了生成过程,并允许使用现有的多视角扩散模型。实验证明,这些模型可适应产生高质量立方体贴图,无需对应感知注意力层。该方法具有精细的文本控制功能,可生成高分辨率全景图像,并在训练集之外具有良好的泛化能力,同时达到定性和定量的最佳结果。
Key Takeaways
- 提出了一种生成360°全景图的新方法。
- 利用多视角扩散模型联合合成立方体贴图的六个面。
- 将每个面视为标准透视图像,简化了生成过程。
- 方法不需要对应感知注意力层,即可产生高质量立方体贴图。
- 具有精细的文本控制功能,可生成高分辨率全景图像。
- 在训练集之外具有良好的泛化能力。
点此查看论文截图
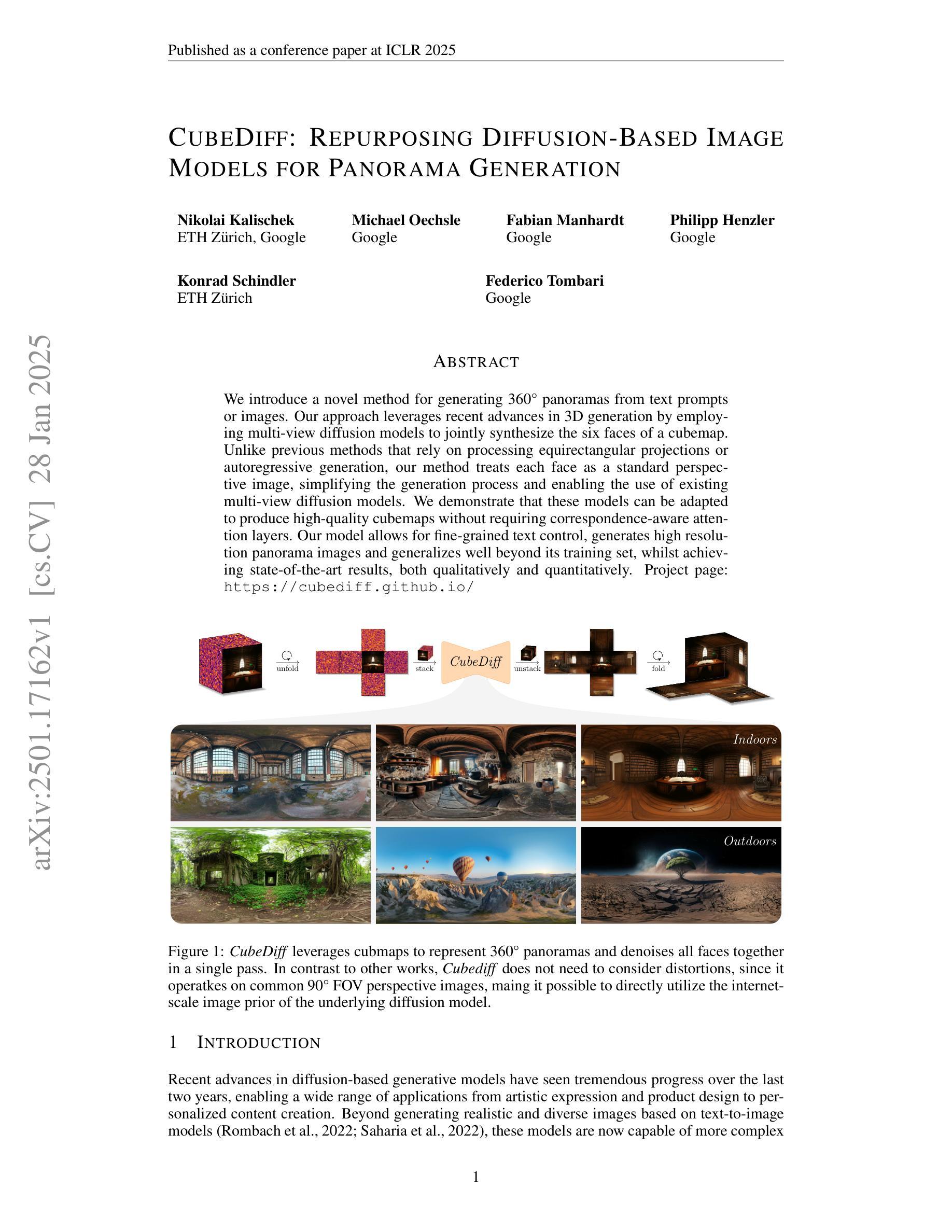
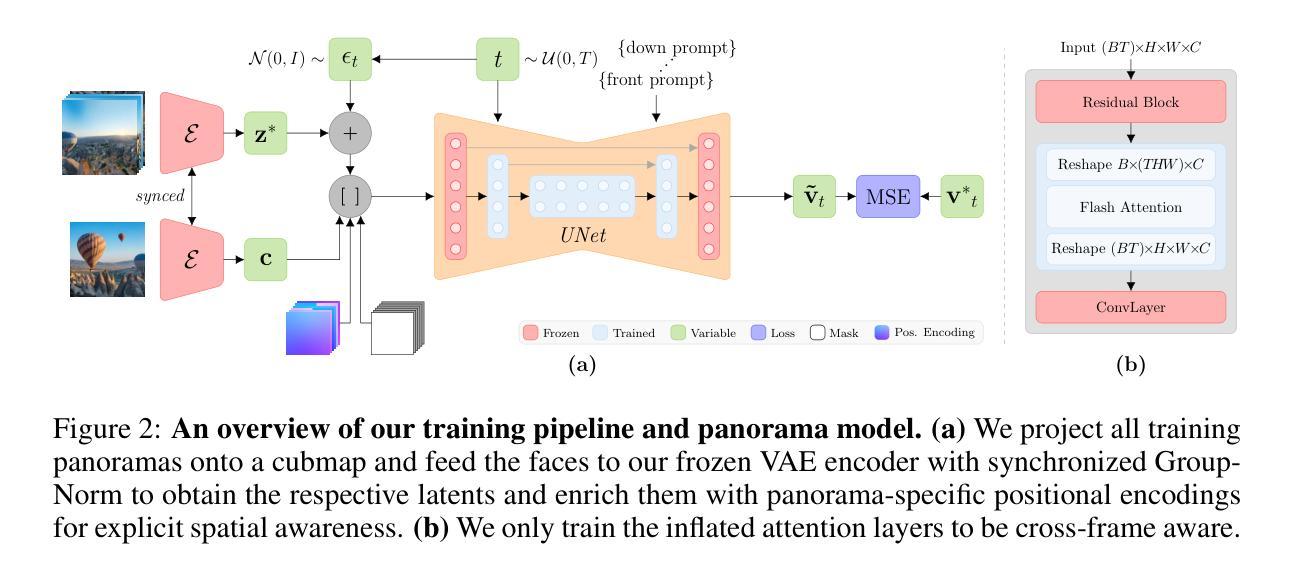
IC-Portrait: In-Context Matching for View-Consistent Personalized Portrait
Authors:Han Yang, Enis Simsar, Sotiris Anagnostidi, Yanlong Zang, Thomas Hofmann, Ziwei Liu
Existing diffusion models show great potential for identity-preserving generation. However, personalized portrait generation remains challenging due to the diversity in user profiles, including variations in appearance and lighting conditions. To address these challenges, we propose IC-Portrait, a novel framework designed to accurately encode individual identities for personalized portrait generation. Our key insight is that pre-trained diffusion models are fast learners (e.g.,100 ~ 200 steps) for in-context dense correspondence matching, which motivates the two major designs of our IC-Portrait framework. Specifically, we reformulate portrait generation into two sub-tasks: 1) Lighting-Aware Stitching: we find that masking a high proportion of the input image, e.g., 80%, yields a highly effective self-supervisory representation learning of reference image lighting. 2) View-Consistent Adaptation: we leverage a synthetic view-consistent profile dataset to learn the in-context correspondence. The reference profile can then be warped into arbitrary poses for strong spatial-aligned view conditioning. Coupling these two designs by simply concatenating latents to form ControlNet-like supervision and modeling, enables us to significantly enhance the identity preservation fidelity and stability. Extensive evaluations demonstrate that IC-Portrait consistently outperforms existing state-of-the-art methods both quantitatively and qualitatively, with particularly notable improvements in visual qualities. Furthermore, IC-Portrait even demonstrates 3D-aware relighting capabilities.
现有的扩散模型在身份保留生成方面显示出巨大潜力。然而,由于用户资料的多样性,包括外观和光照条件的差异,个性化肖像生成仍然具有挑战性。为了解决这些挑战,我们提出了IC-Portrait,这是一个旨在准确编码个人身份用于个性化肖像生成的新型框架。我们的关键见解是,预训练的扩散模型对于上下文中的密集对应关系匹配是快速学习者(例如,100至200步),这激发了我们IC-Portrait框架的两个主要设计。具体来说,我们将肖像生成重新定义为两个子任务:1)光照感知拼接:我们发现遮挡输入图像的高比例部分(例如80%)可以有效地进行参考图像光照的自监督表示学习。2)视图一致适应:我们利用合成视图一致的轮廓数据集来学习上下文中的对应关系。然后,可以将参考轮廓变形为任意姿势,以实现强大的空间对齐视图条件。通过简单地连接潜在空间以形成类似ControlNet的监督和管理,可以大大增强身份保留的保真度和稳定性。大量评估表明,IC-Portrait在定量和定性方面均始终优于现有最先进的方法,在视觉品质方面尤其取得了显著的改进。此外,IC-Portrait甚至展示了3D感知的重照明能力。
论文及项目相关链接
PDF technical report
摘要
本文提出一种名为IC-Portrait的新型框架,旨在解决个性化肖像生成中的身份保留挑战。该框架利用预训练的扩散模型进行快速学习,通过两个主要设计实现准确编码个体身份:一是光照感知拼接,通过遮挡大部分输入图像实现自我监督学习参考图像光照;二是视角一致性适应,利用合成视角一致性轮廓数据集学习上下文对应关系。IC-Portrait通过结合这两个设计,显著提高了身份保留的保真度和稳定性。评估表明,IC-Portrait在定量和定性上均优于现有先进技术,尤其在视觉品质上有显著改进,甚至展示了3D感知的重照明能力。
关键见解
- IC-Portrait框架被设计为解决个性化肖像生成中的身份保留挑战。
- 利用预训练的扩散模型进行快速学习,实现准确编码个体身份。
- 通过光照感知拼接和视角一致性适应两个主要设计,提高身份保留的保真度和稳定性。
- 光照感知拼接通过遮挡大部分输入图像实现自我监督学习参考图像光照。
- 视角一致性适应利用合成视角一致性轮廓数据集学习上下文对应关系。
- IC-Portrait在定量和定性评估上优于现有技术,尤其在视觉品质上有显著改进。
点此查看论文截图
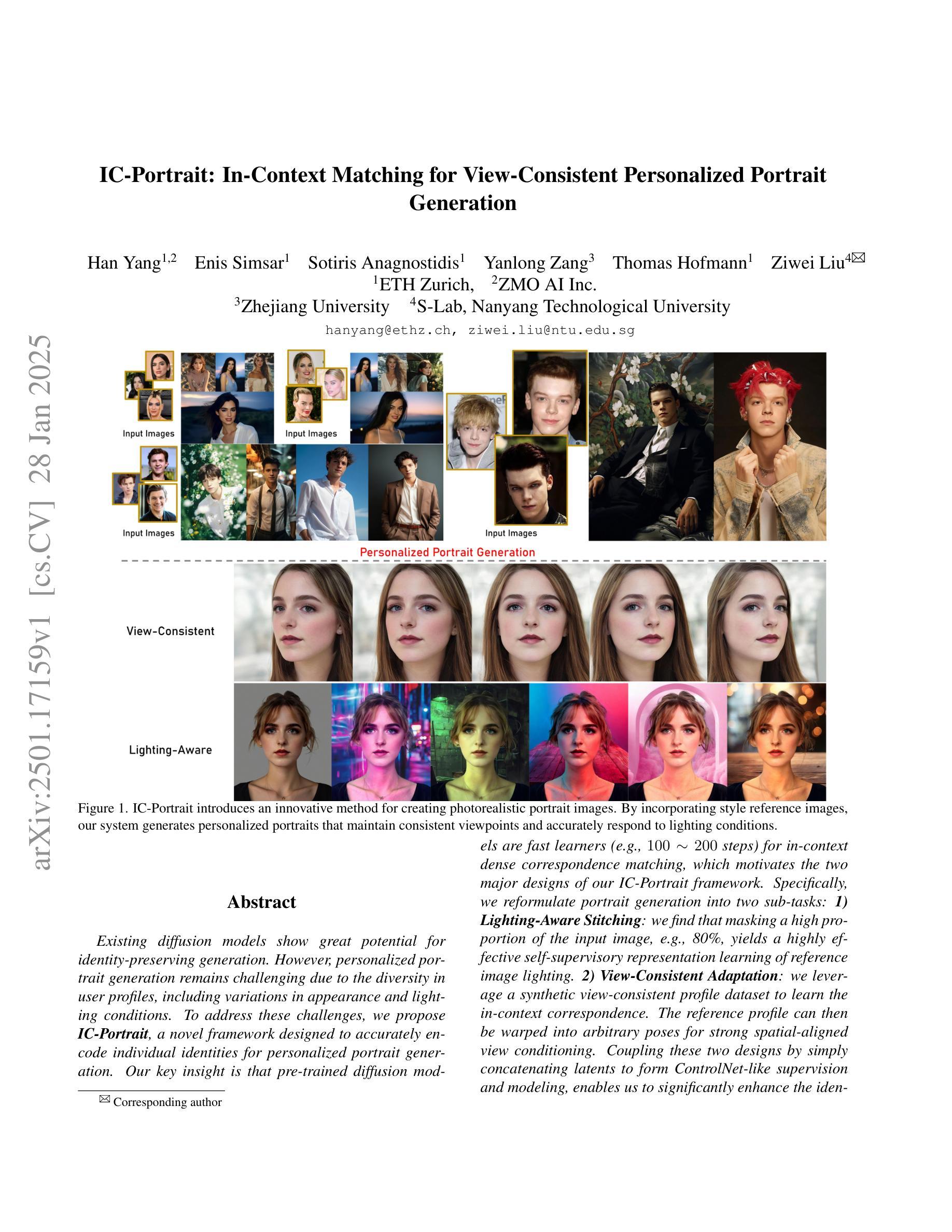
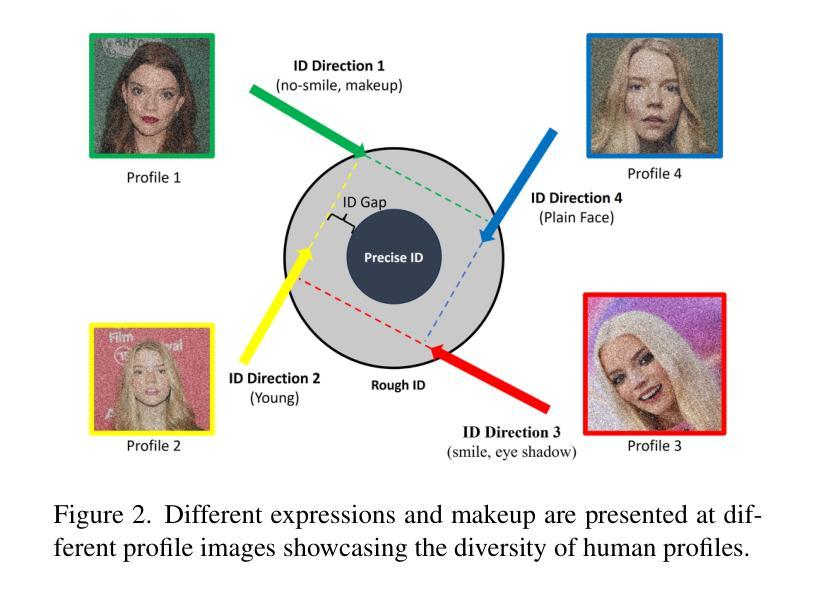
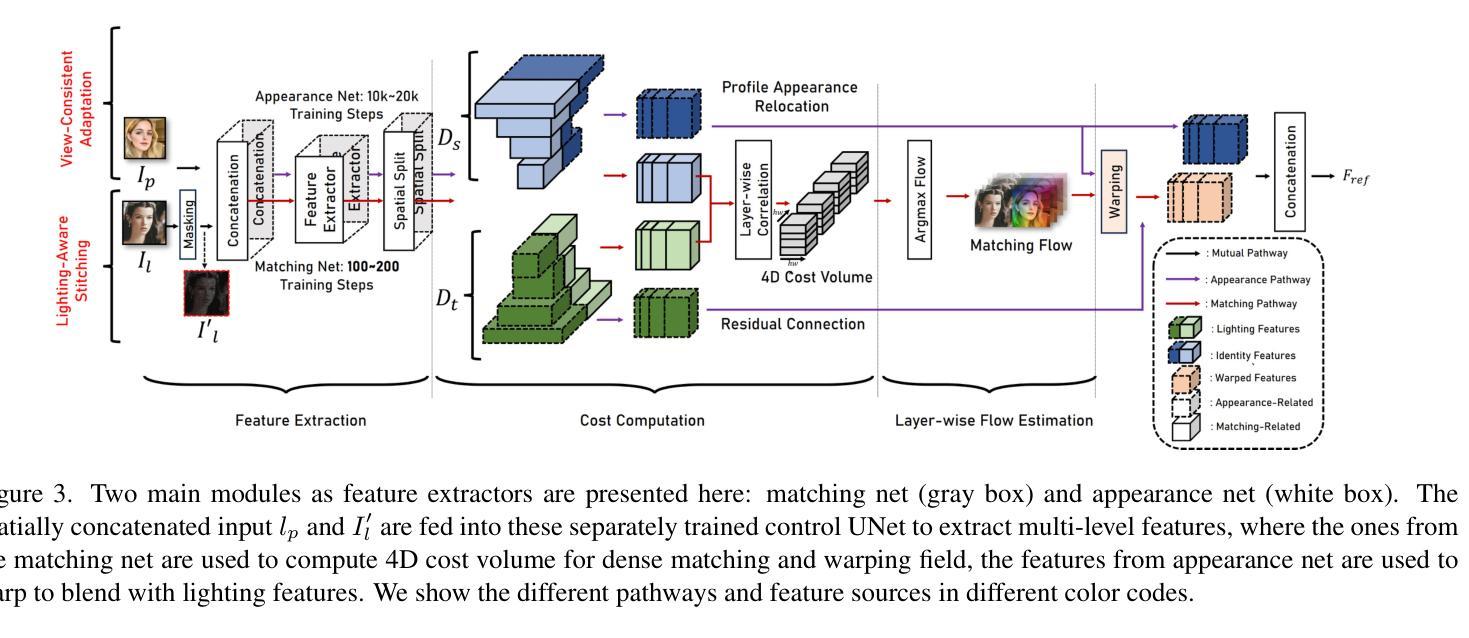
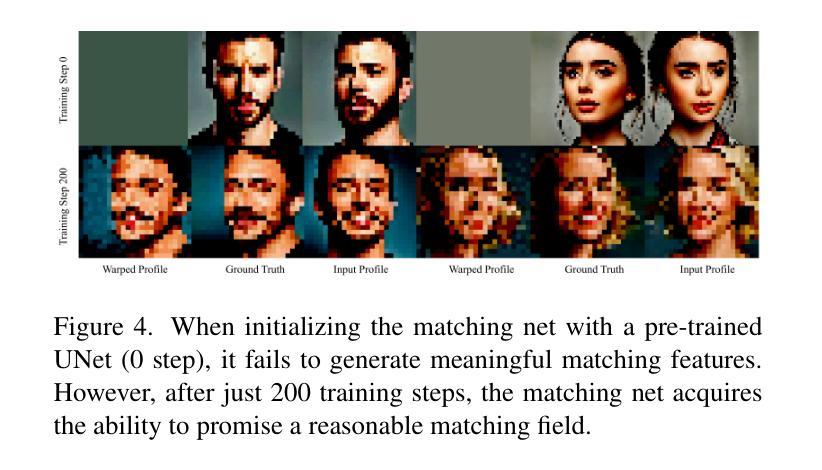
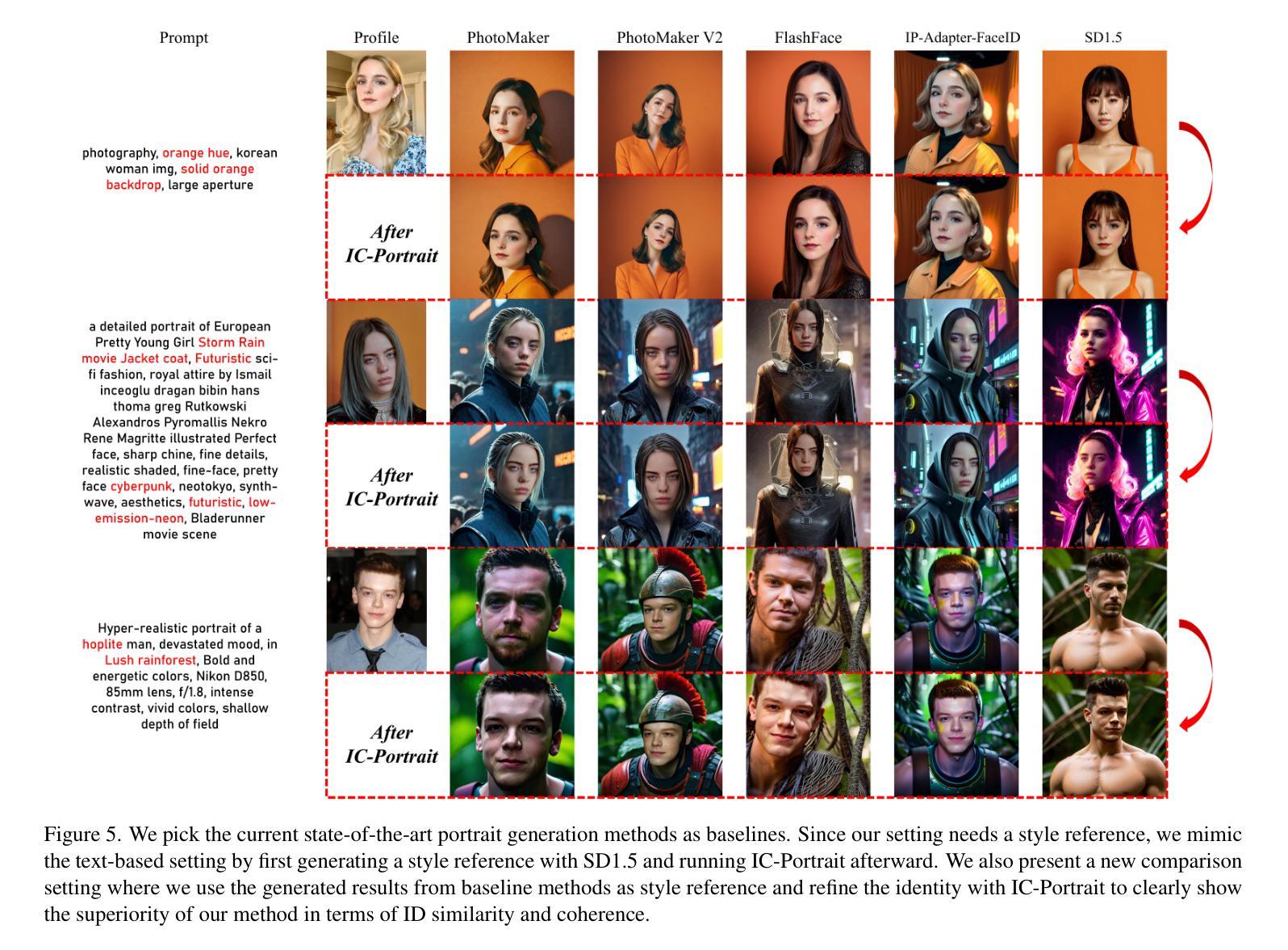
DIRIGENt: End-To-End Robotic Imitation of Human Demonstrations Based on a Diffusion Model
Authors:Josua Spisak, Matthias Kerzel, Stefan Wermter
There has been substantial progress in humanoid robots, with new skills continuously being taught, ranging from navigation to manipulation. While these abilities may seem impressive, the teaching methods often remain inefficient. To enhance the process of teaching robots, we propose leveraging a mechanism effectively used by humans: teaching by demonstrating. In this paper, we introduce DIRIGENt (DIrect Robotic Imitation GENeration model), a novel end-to-end diffusion approach that directly generates joint values from observing human demonstrations, enabling a robot to imitate these actions without any existing mapping between it and humans. We create a dataset in which humans imitate a robot and then use this collected data to train a diffusion model that enables a robot to imitate humans. The following three aspects are the core of our contribution. First is our novel dataset with natural pairs between human and robot poses, allowing our approach to imitate humans accurately despite the gap between their anatomies. Second, the diffusion input to our model alleviates the challenge of redundant joint configurations, limiting the search space. And finally, our end-to-end architecture from perception to action leads to an improved learning capability. Through our experimental analysis, we show that combining these three aspects allows DIRIGENt to outperform existing state-of-the-art approaches in the field of generating joint values from RGB images.
人形机器人在技能学习方面取得了巨大进步,不断习得新技能,从导航到操作都有涉及。尽管这些能力令人印象深刻,但教学方法往往效率低下。为了改进机器人教学过程,我们提出利用人类有效使用的一种机制:通过示范进行教学。在本文中,我们介绍了DIRIGENt(直接机器人模仿生成模型),这是一种新型端到端扩散方法,能够直接从人类示范中生成关节值,使机器人能够模仿这些动作,而无需在机器人和人类之间建立现有映射。我们创建了一个数据集,人类在其中模仿机器人,然后使用收集的数据来训练一个扩散模型,使机器人能够模仿人类。我们贡献的核心在于以下三个方面。首先,我们拥有自然的人类和机器人姿势配对数据集,这使得我们的方法即使在他们解剖结构之间存在差异的情况下,也能准确地模仿人类。其次,我们模型的扩散输入减轻了冗余关节配置的挑战,限制了搜索空间。最后,我们从感知到动作的端到端架构提高了学习能力。通过我们的实验分析,我们证明了结合这三个方面可以使DIRIGENt在从RGB图像生成关节值领域超越现有最先进的方法。
论文及项目相关链接
Summary
人类型机器人取得显著进展,习得新技能,如导航和操控。为提升机器人教学过程的效率,本文提出借鉴人类常用的教学方式——通过示范进行教学,并介绍DIRIGENt(直接机器人模仿生成模型),这是一种全新的端到端扩散方法,它能从观察人类示范动作中直接生成关节值,让机器人在无需与人类建立映射关系的情况下模仿动作。本文创建了人类模仿机器人的数据集,并用其训练扩散模型,使机器人能模仿人类。本文的核心贡献包括三个方面:自然配对的人类和机器人姿态构成的新数据集,让机器人能够准确模仿人类;模型扩散输入解决了关节配置冗余问题并缩减了搜索空间;端到端的感知到行动架构提高了学习能力。实验证明DIRIGENt在由RGB图像生成关节值方面表现超越现有技术。
Key Takeaways
- 人类型机器人在技能和动作上取得显著进步。
- 当前机器人教学方式存在效率问题。
- 借鉴人类通过示范进行教学的方式,提出DIRIGENt模型。
- DIRIGENt模型是一种端到端的扩散方法,能从人类示范中直接生成关节值。
- 创建了人类模仿机器人的数据集用于训练扩散模型。
- 模型的核心贡献包括新数据集、扩散输入解决关节配置冗余问题以及端到端的感知到行动架构。
点此查看论文截图
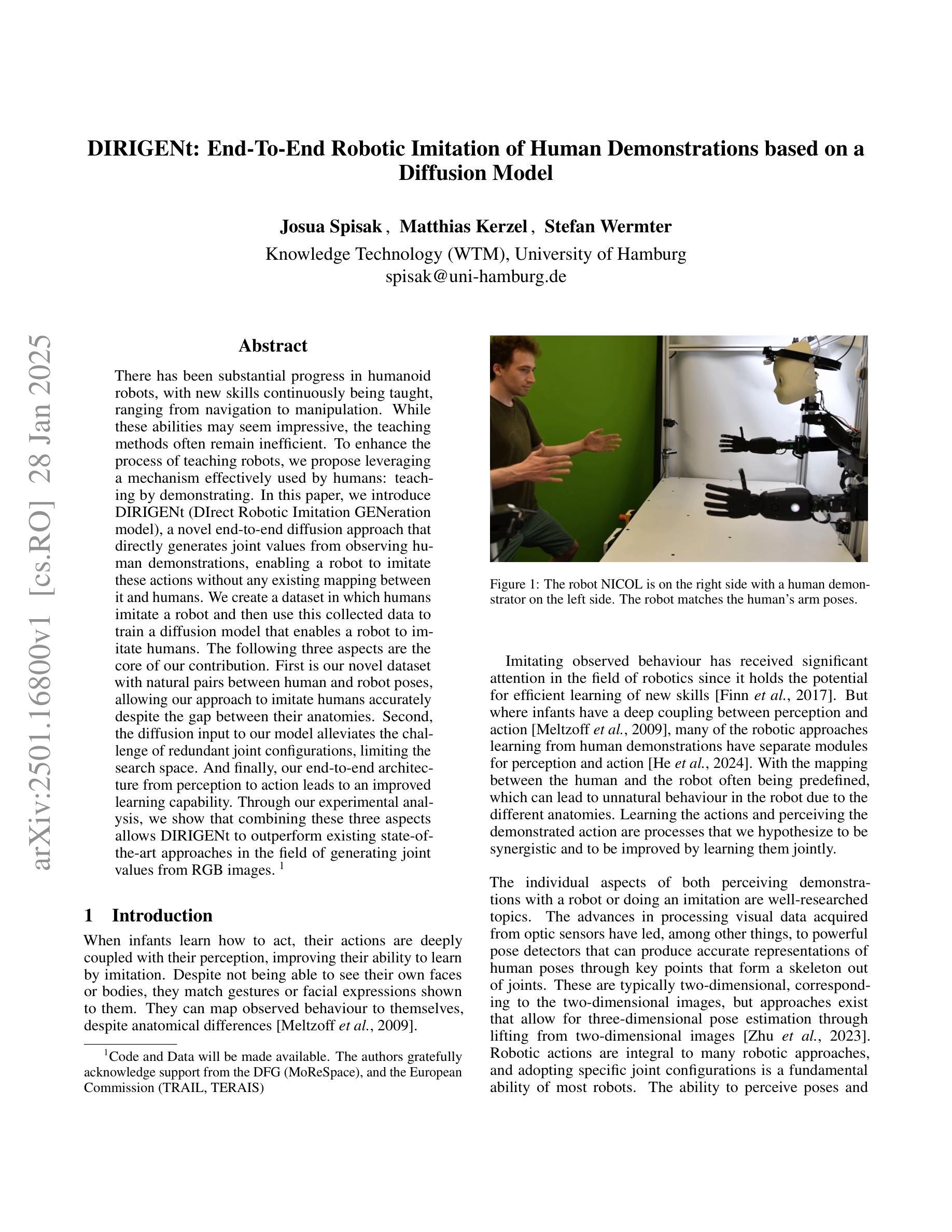
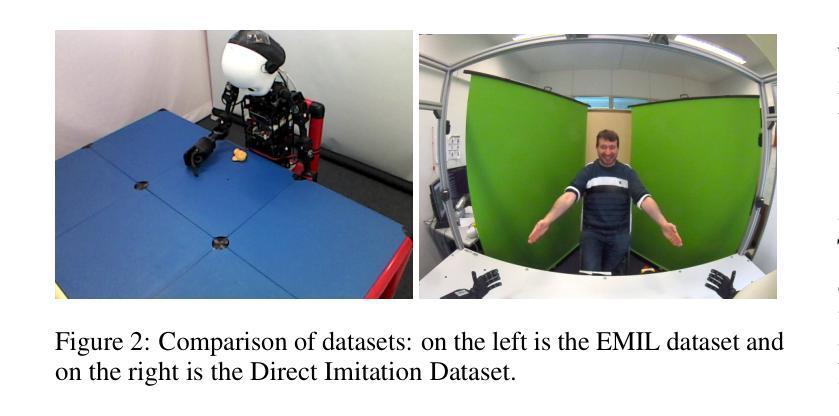
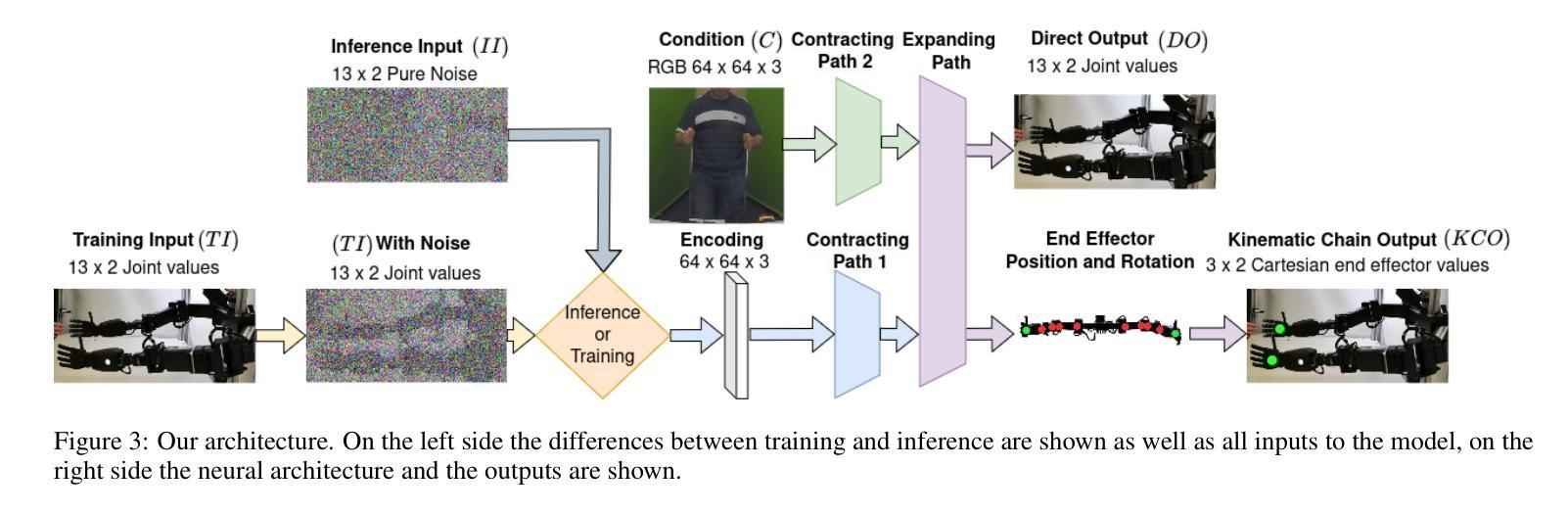
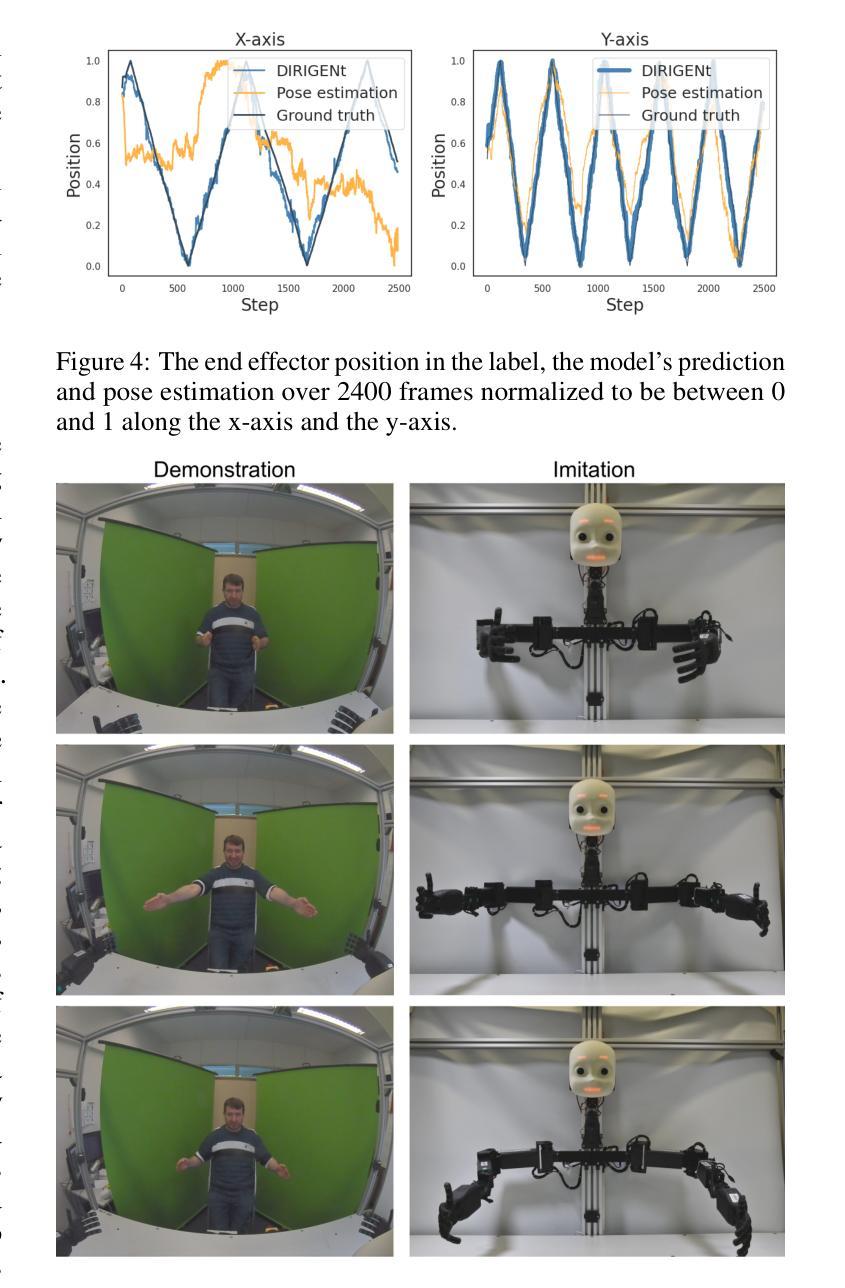
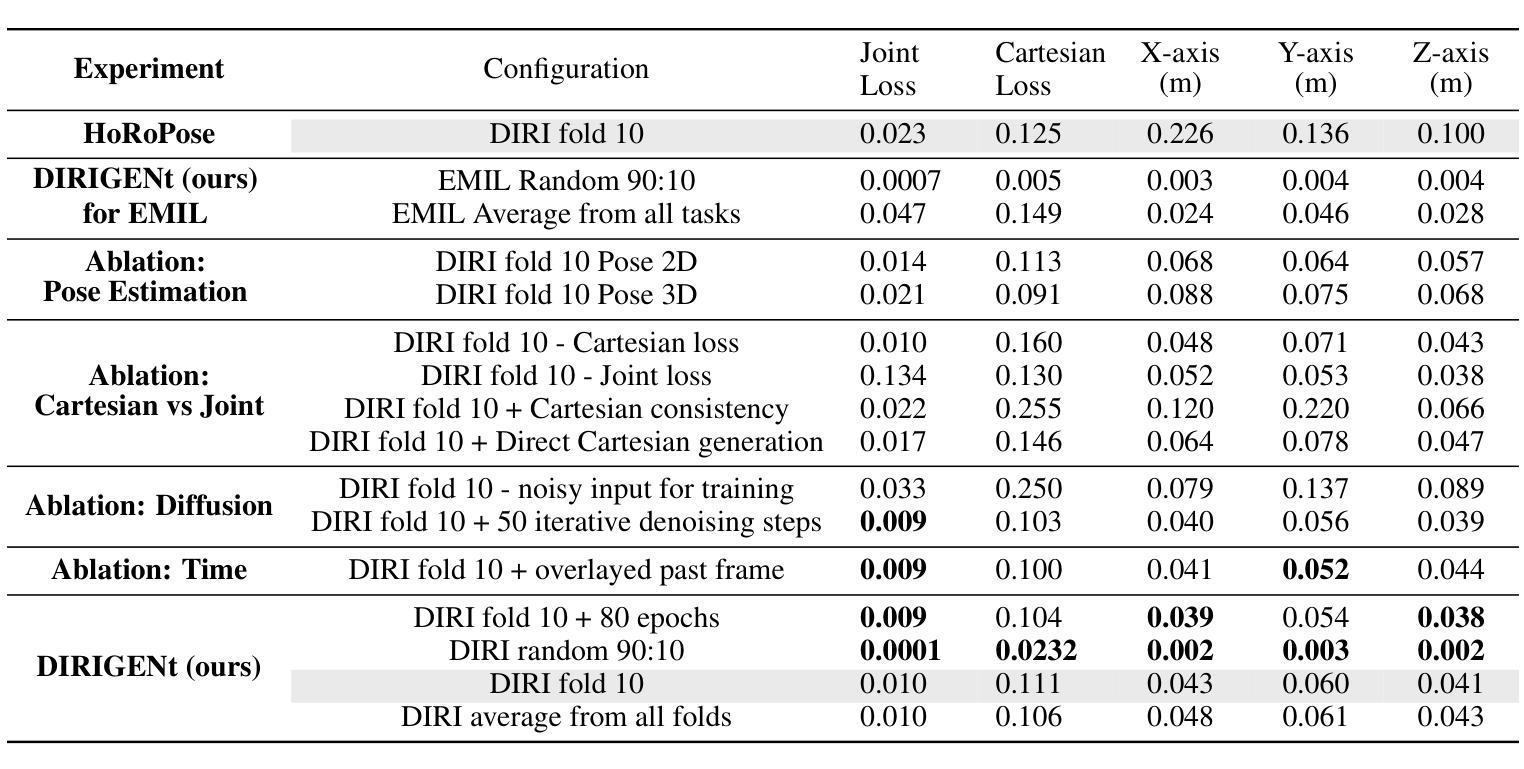
DiffSplat: Repurposing Image Diffusion Models for Scalable Gaussian Splat Generation
Authors:Chenguo Lin, Panwang Pan, Bangbang Yang, Zeming Li, Yadong Mu
Recent advancements in 3D content generation from text or a single image struggle with limited high-quality 3D datasets and inconsistency from 2D multi-view generation. We introduce DiffSplat, a novel 3D generative framework that natively generates 3D Gaussian splats by taming large-scale text-to-image diffusion models. It differs from previous 3D generative models by effectively utilizing web-scale 2D priors while maintaining 3D consistency in a unified model. To bootstrap the training, a lightweight reconstruction model is proposed to instantly produce multi-view Gaussian splat grids for scalable dataset curation. In conjunction with the regular diffusion loss on these grids, a 3D rendering loss is introduced to facilitate 3D coherence across arbitrary views. The compatibility with image diffusion models enables seamless adaptions of numerous techniques for image generation to the 3D realm. Extensive experiments reveal the superiority of DiffSplat in text- and image-conditioned generation tasks and downstream applications. Thorough ablation studies validate the efficacy of each critical design choice and provide insights into the underlying mechanism.
关于文本或单幅图像生成三维内容的最新进展受限于高质量的三维数据集以及二维多视角生成的不一致性。我们引入了DiffSplat,这是一种新型的三维生成框架,它通过驯服大规模文本到图像扩散模型来原生生成三维高斯光斑。它与之前的三维生成模型不同,能够在统一模型中有效利用网络规模的二维先验知识,同时保持三维一致性。为了启动训练,我们提出了一种轻量级的重建模型,用于即时生成多视角高斯光斑网格,以实现可扩展的数据集整理。结合这些网格上的常规扩散损失,引入了一种三维渲染损失,以促进任意视角下的三维连贯性。其与图像扩散模型的兼容性使得众多图像生成技术能够无缝适应三维领域。大量实验表明,DiffSplat在文本和图像条件生成任务以及下游应用中表现出卓越性能。详尽的消融研究验证了每个关键设计选择的有效性,并深入了解了其内在机制。
论文及项目相关链接
PDF Accepted to ICLR 2025; Project page: https://chenguolin.github.io/projects/DiffSplat
Summary
新一代三维内容生成技术面临数据集质量不高和多视角生成不一致的问题。我们推出DiffSplat,一种新型三维生成框架,通过驾驭大规模文本到图像扩散模型,直接生成三维高斯点云。它不同于以往的三维生成模型,能有效利用互联网规模的二维先验知识,同时在统一模型中保持三维一致性。为启动训练,我们提出了一种轻量级重建模型,可立即生成多视角高斯点云网格,便于扩展数据集整理。除了在这些网格上的常规扩散损失,我们还引入了三维渲染损失,以促进任意视角下的三维连贯性。其与图像扩散模型的兼容性使得众多图像生成技术能够无缝适应三维领域。大量实验表明,DiffSplat在文本和图像条件生成任务以及下游应用中表现出卓越性能。彻底的消融研究验证了关键设计选择的有效性,并揭示了其内在机制。
Key Takeaways
- DiffSplat是一种新型三维生成框架,能够直接生成三维高斯点云。
- 与其他三维生成模型不同,DiffSplat有效利用互联网规模的二维先验知识,并维持统一模型中的三维一致性。
- 通过轻量级重建模型快速生成多视角高斯点云网格,促进数据集整理。
- 引入三维渲染损失,增强任意视角下的三维连贯性。
- DiffSplat与图像扩散模型的兼容性使得图像生成技术能无缝适应三维领域。
- 大量实验证明DiffSplat在文本和图像条件生成任务以及下游应用中的卓越性能。
- 消融研究验证了关键设计选择的有效性,揭示了其内在机制和工作原理。
点此查看论文截图
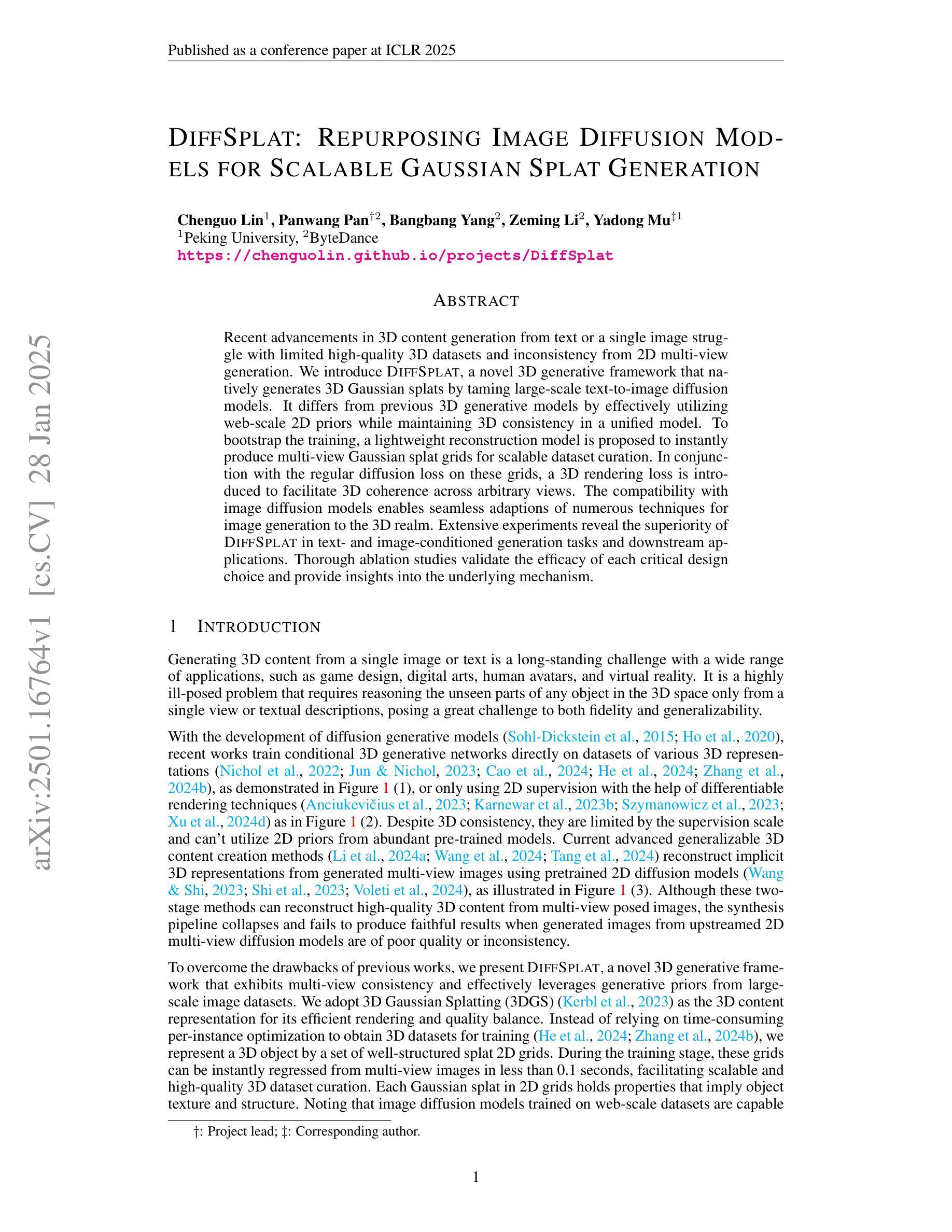
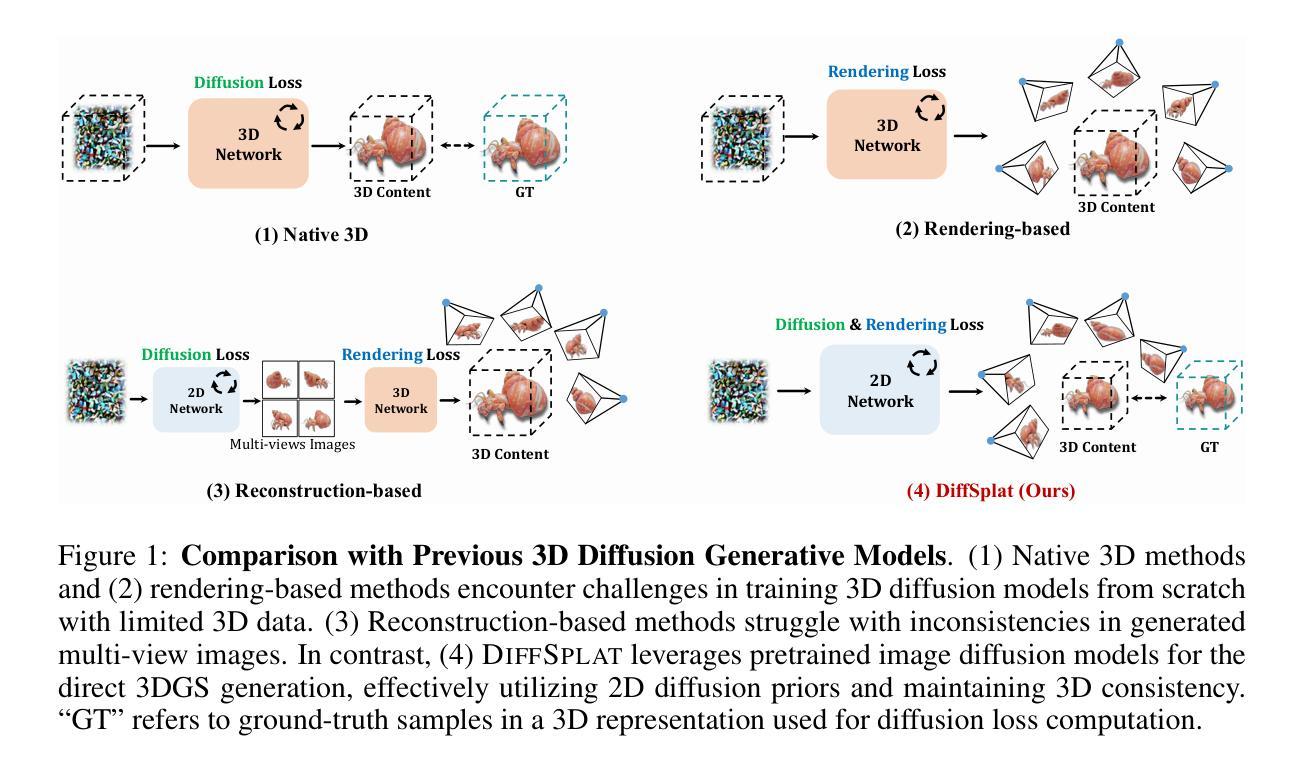
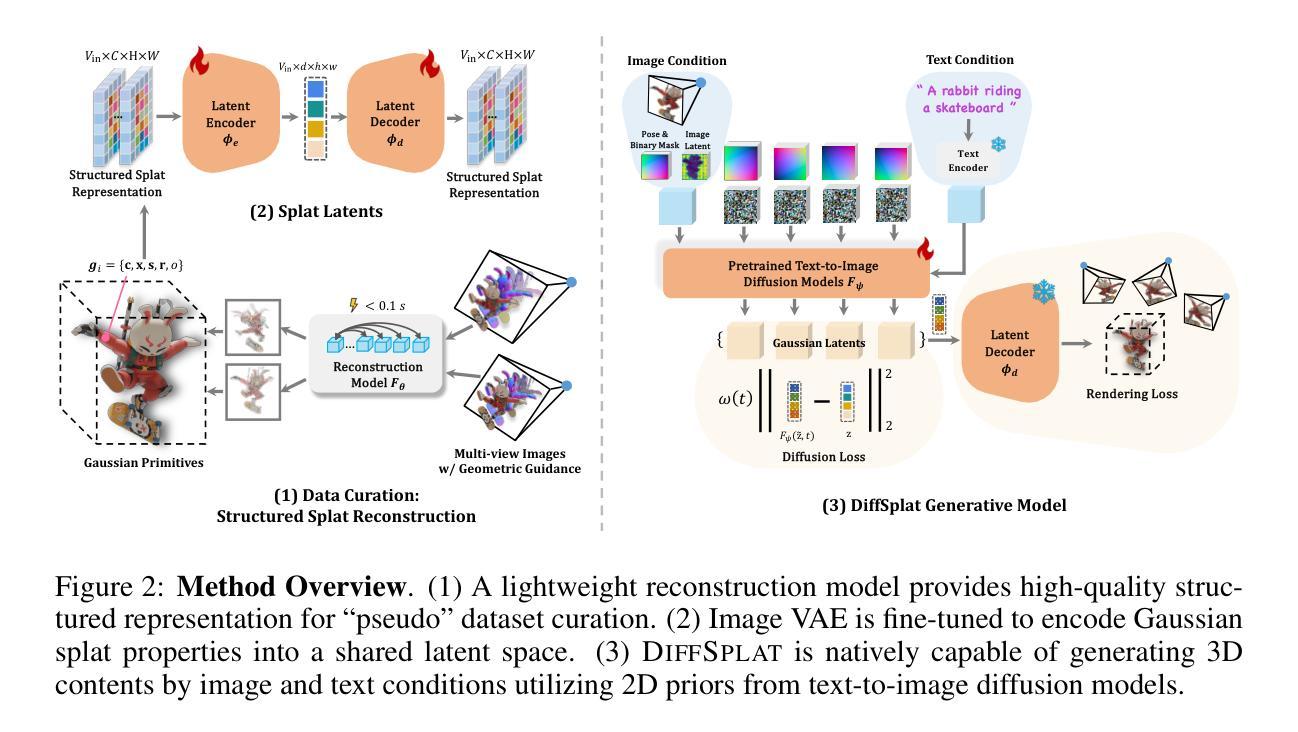
ITVTON:Virtual Try-On Diffusion Transformer Model Based on Integrated Image and Text
Authors:Haifeng Ni
Recent advancements in virtual fitting for characters and clothing have leveraged diffusion models to improve the realism of garment fitting. However, challenges remain in handling complex scenes and poses, which can result in unnatural garment fitting and poorly rendered intricate patterns. In this work, we introduce ITVTON, a novel method that enhances clothing-character interactions by combining clothing and character images along spatial channels as inputs, thereby improving fitting accuracy for the inpainting model. Additionally, we incorporate integrated textual descriptions from multiple images to boost the realism of the generated visual effects. To optimize computational efficiency, we limit training to the attention parameters within a single diffusion transformer (Single-DiT) block. To more rigorously address the complexities of real-world scenarios, we curated training samples from the IGPair dataset, thereby enhancing ITVTON’s performance across diverse environments. Extensive experiments demonstrate that ITVTON outperforms baseline methods both qualitatively and quantitatively, setting a new standard for virtual fitting tasks.
在字符和服装虚拟适配方面的最新进展已经利用扩散模型提高了服装适配的真实性。然而,在处理复杂场景和姿势时仍存在挑战,这可能导致服装适配不自然,精细图案渲染不佳。在这项工作中,我们引入了ITVTON,这是一种通过结合服装和角色图像作为空间通道输入来增强服装与角色交互的新型方法,从而提高补全模型的适配准确性。此外,我们从多张图像中融入集成的文本描述,以提高生成视觉效果的真实性。为了优化计算效率,我们将训练限制在单个扩散变压器(Single-DiT)块内的注意力参数。为了更严格地解决现实场景的复杂性,我们从IGPair数据集中精选训练样本,从而提高ITVTON在不同环境中的性能。大量实验表明,ITVTON在定性和定量方面都优于基准方法,为虚拟适配任务树立了新的标准。
论文及项目相关链接
Summary
扩散模型在虚拟角色服装拟合中的应用,近期取得了显著进展,提升了服装真实感。然而,处理复杂场景和姿势的挑战仍然存在,可能导致服装拟合不自然和精细图案渲染不良。本研究引入ITVTON方法,通过结合服装和角色图像作为空间通道输入,提高衣物与角色的互动,改善填充模型的拟合精度。此外,还融入多张图像的文本描述,增强生成视觉效果的真实感。为优化计算效率,仅在单个扩散变压器(Single-DiT)块内训练注意力参数。通过采集IGPair数据集的训练样本,应对现实场景的复杂性,ITVTON在多种环境下表现出卓越性能。实验证明,ITVTON在定性和定量上均优于基准方法,为虚拟拟合任务树立了新标准。
Key Takeaways
- 扩散模型用于改进虚拟角色服装拟合的逼真度。
- 处理复杂场景和姿势的挑战仍然突出。
- ITVTON方法结合服装和角色图像作为输入,提高衣物与角色的互动。
- 通过结合多张图像的文本描述,增强生成的视觉效果的真实感。
- 为优化计算效率,仅在单个扩散变压器块内训练注意力参数。
- 使用IGPair数据集的训练样本应对现实场景的复杂性。
点此查看论文截图
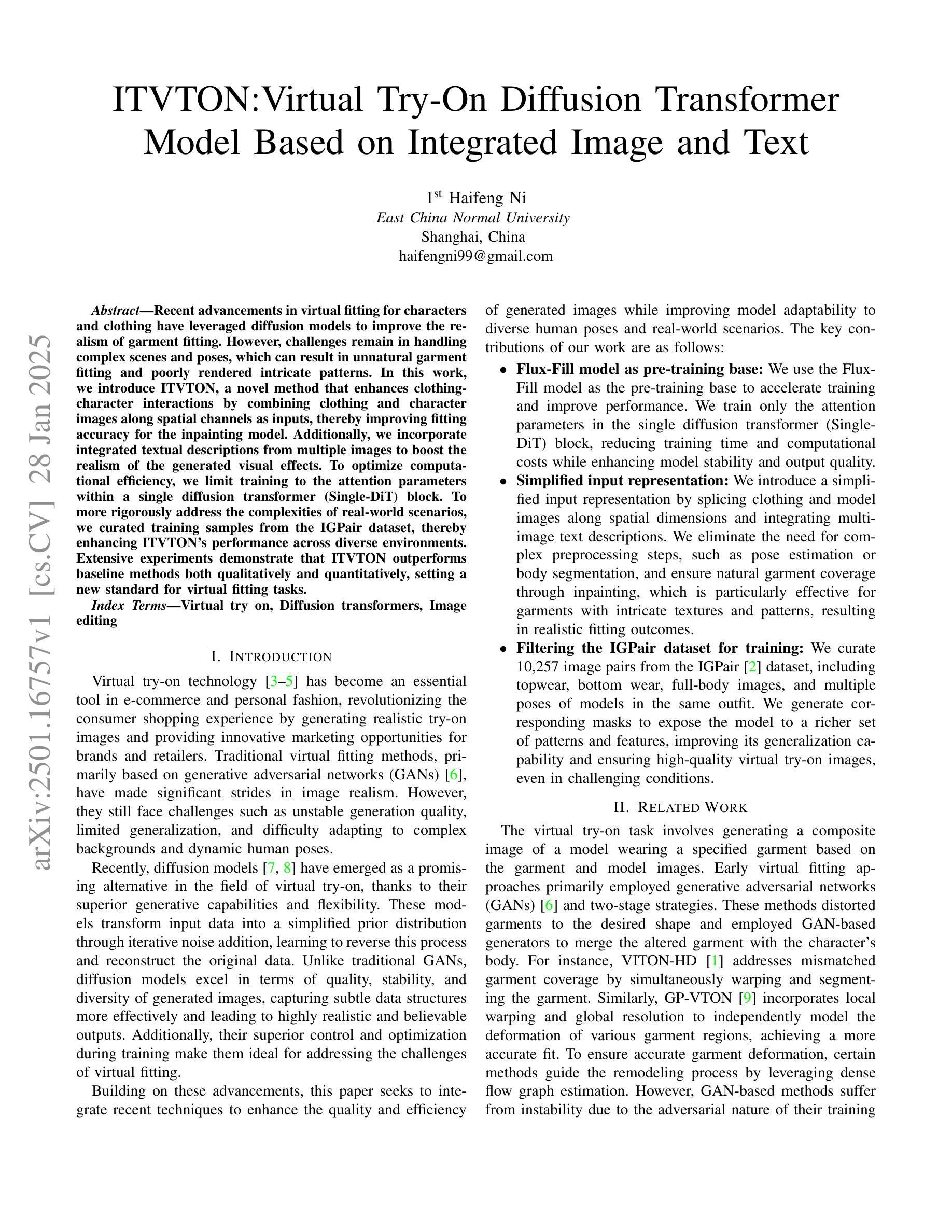
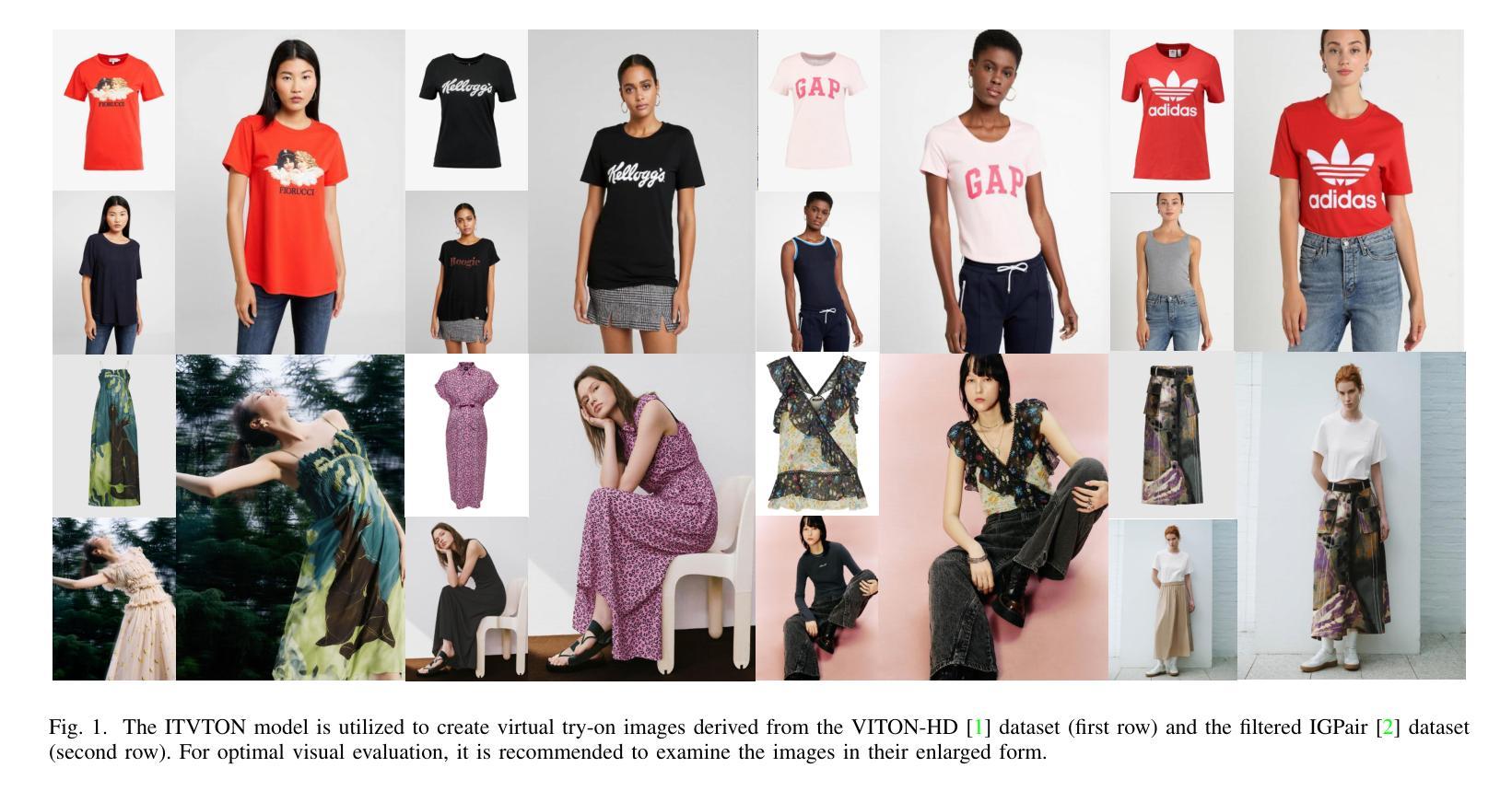
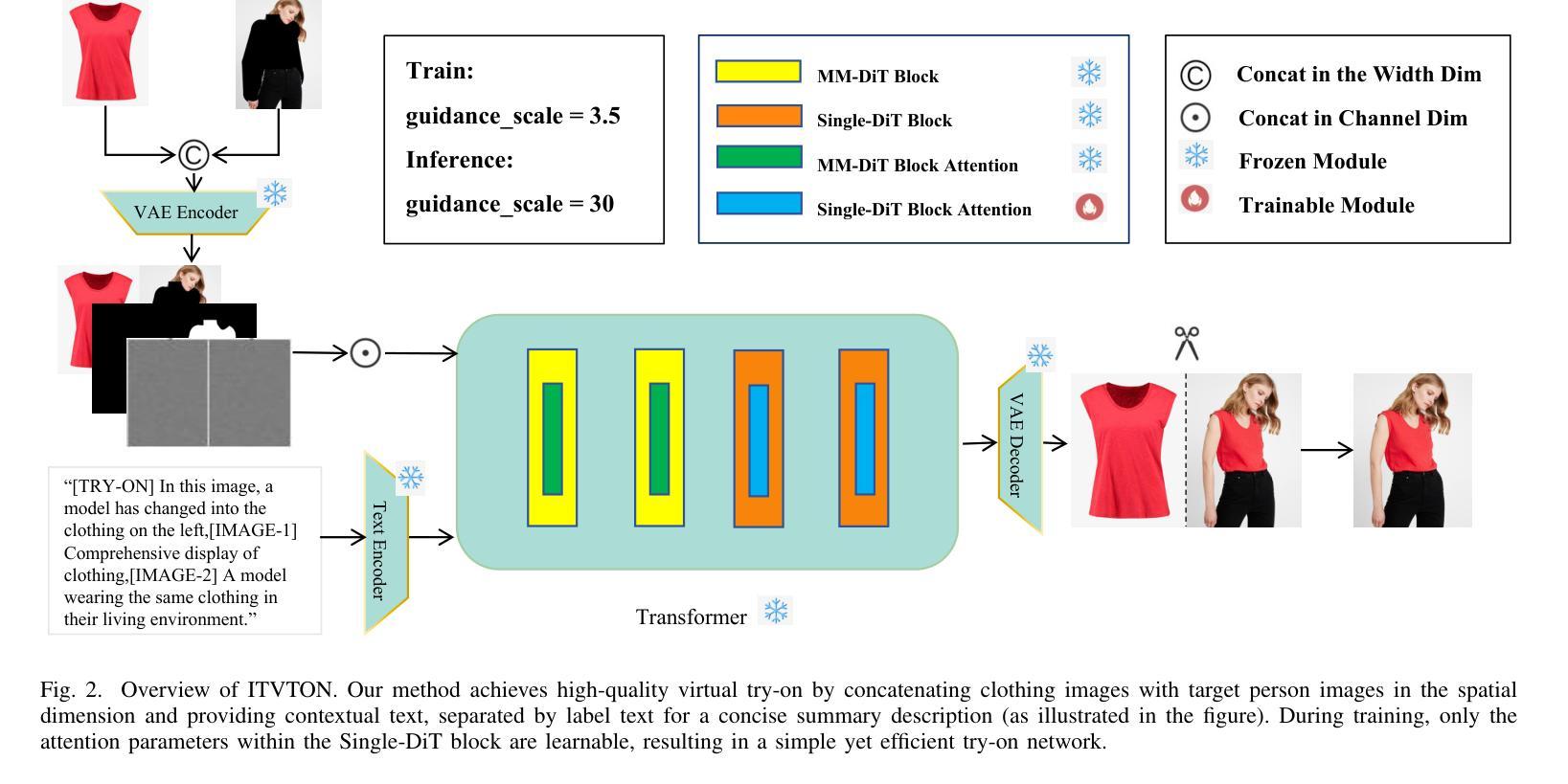
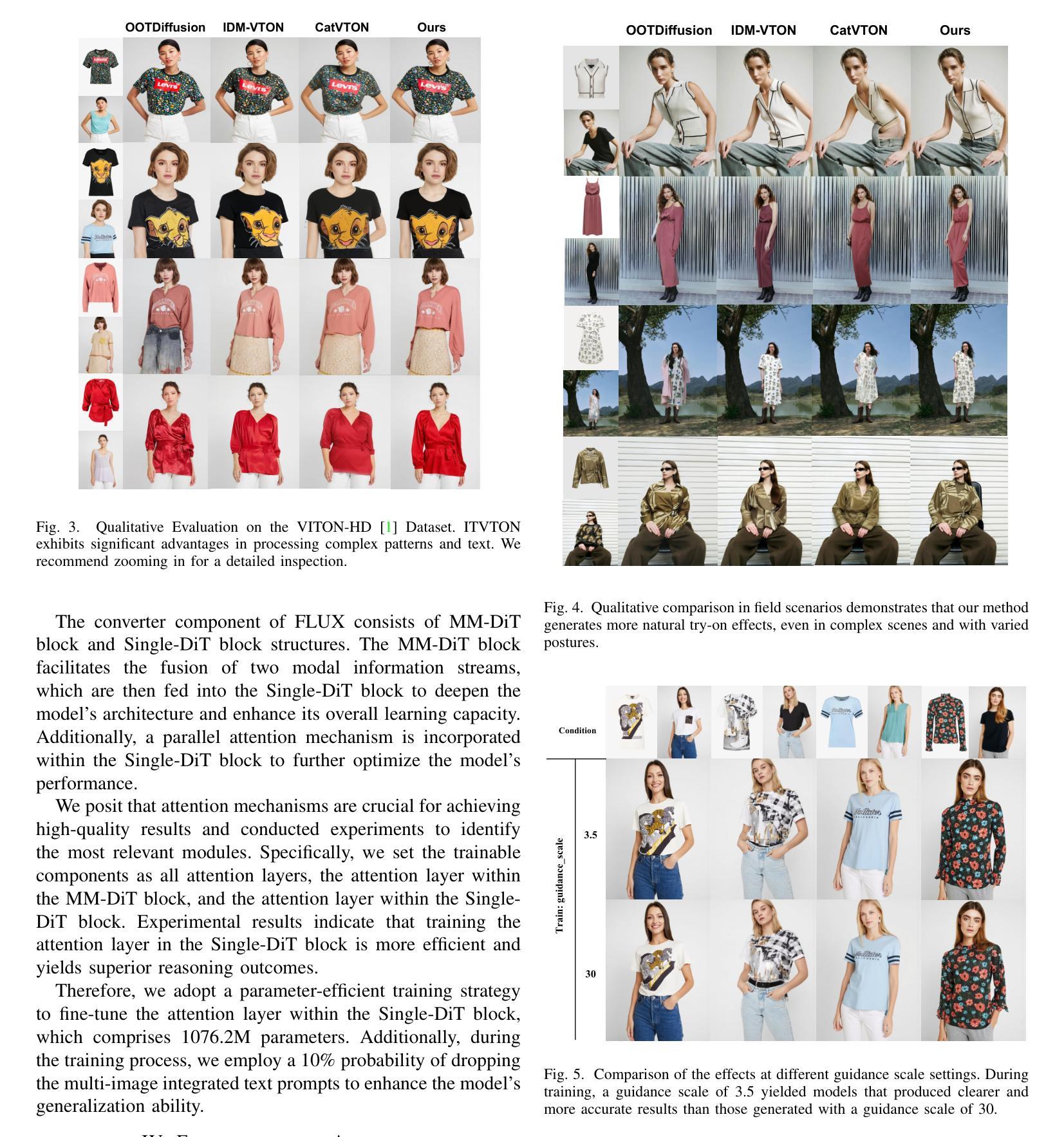
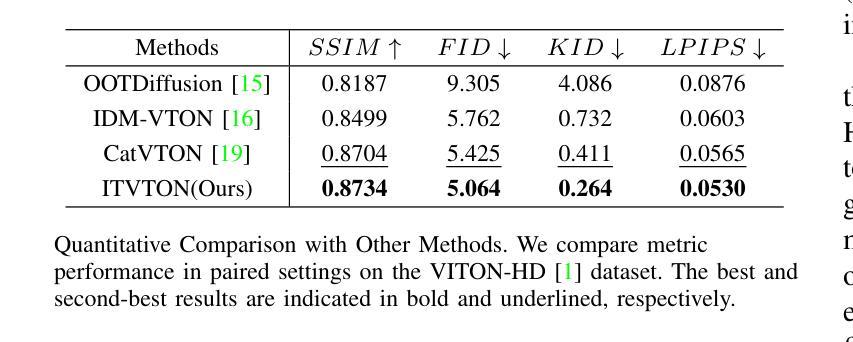

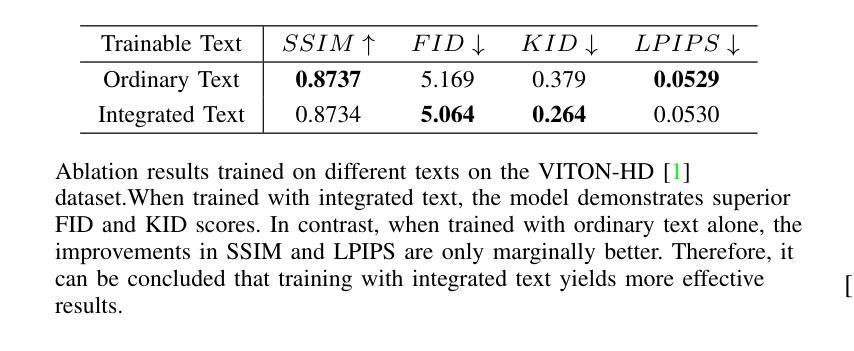
CascadeV: An Implementation of Wurstchen Architecture for Video Generation
Authors:Wenfeng Lin, Jiangchuan Wei, Boyuan Liu, Yichen Zhang, Shiyue Yan, Mingyu Guo
Recently, with the tremendous success of diffusion models in the field of text-to-image (T2I) generation, increasing attention has been directed toward their potential in text-to-video (T2V) applications. However, the computational demands of diffusion models pose significant challenges, particularly in generating high-resolution videos with high frame rates. In this paper, we propose CascadeV, a cascaded latent diffusion model (LDM), that is capable of producing state-of-the-art 2K resolution videos. Experiments demonstrate that our cascaded model achieves a higher compression ratio, substantially reducing the computational challenges associated with high-quality video generation. We also implement a spatiotemporal alternating grid 3D attention mechanism, which effectively integrates spatial and temporal information, ensuring superior consistency across the generated video frames. Furthermore, our model can be cascaded with existing T2V models, theoretically enabling a 4$\times$ increase in resolution or frames per second without any fine-tuning. Our code is available at https://github.com/bytedance/CascadeV.
最近,随着扩散模型在文本到图像(T2I)生成领域的巨大成功,人们越来越关注其在文本到视频(T2V)应用中的潜力。然而,扩散模型的计算需求构成了重大挑战,特别是在生成高帧率的高分辨率视频时。在本文中,我们提出了CascadeV,这是一种级联的潜在扩散模型(LDM),能够产生最先进的2K分辨率视频。实验表明,我们的级联模型实现了更高的压缩比,大大降低了高质量视频生成的计算挑战。我们还实现了一种时空交替网格3D注意力机制,有效地结合了空间和时间信息,确保了生成视频帧之间的一致性。此外,我们的模型可以与现有的T2V模型级联,理论上可以在不进行微调的情况下实现每秒帧数或分辨率的四倍提升。我们的代码可在https://github.com/bytedance/CascadeV找到。
论文及项目相关链接
Summary
扩散模型在文本到图像生成领域取得了巨大成功,其在文本到视频(T2V)应用中的潜力正受到越来越多的关注。然而,扩散模型在计算需求方面存在挑战,特别是在生成高分辨率和高帧率视频时。本文提出了CascadeV,一种级联潜在扩散模型(LDM),能够生成最先进的2K分辨率视频。实验表明,级联模型实现了更高的压缩比,减少了高质量视频生成的计算挑战。此外,还实现了时空交替网格3D注意力机制,有效整合空间和时间信息,确保生成视频帧之间的一致性。该模型还可以与现有T2V模型级联,理论上可以提高分辨率或帧率而不需微调。
Key Takeaways
- 扩散模型在文本到视频生成领域的应用潜力正在受到关注。
- 级联潜在扩散模型(CascadeV)能够生成高质量的2K分辨率视频。
- 级联模型实现了高压缩比,降低计算需求。
- 实现了时空交替网格3D注意力机制,增强视频帧间一致性。
- CascadeV模型可以与现有T2V模型级联,提高分辨率或帧率。
- 该模型理论上的优点包括在不进行微调的情况下提高分辨率或帧率。
点此查看论文截图
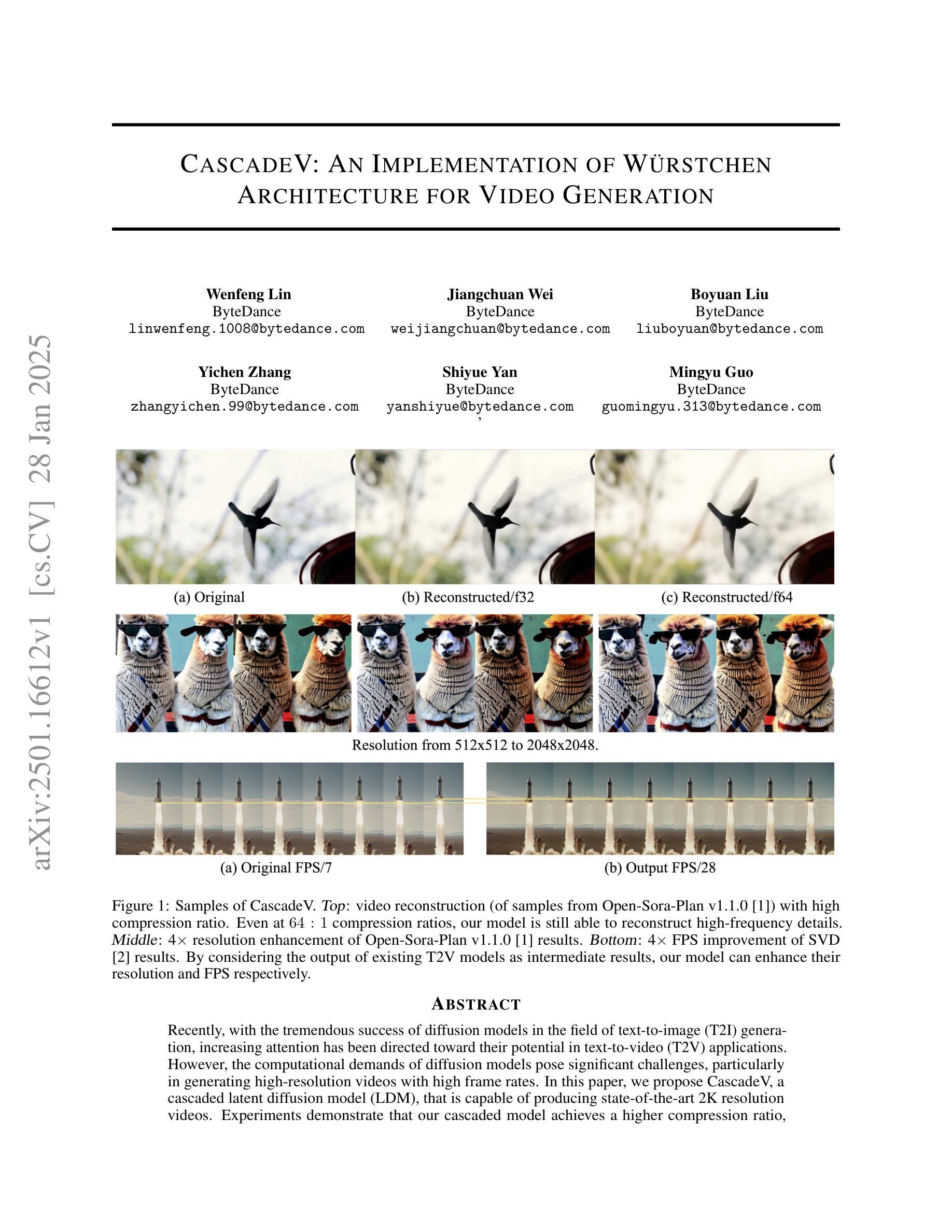
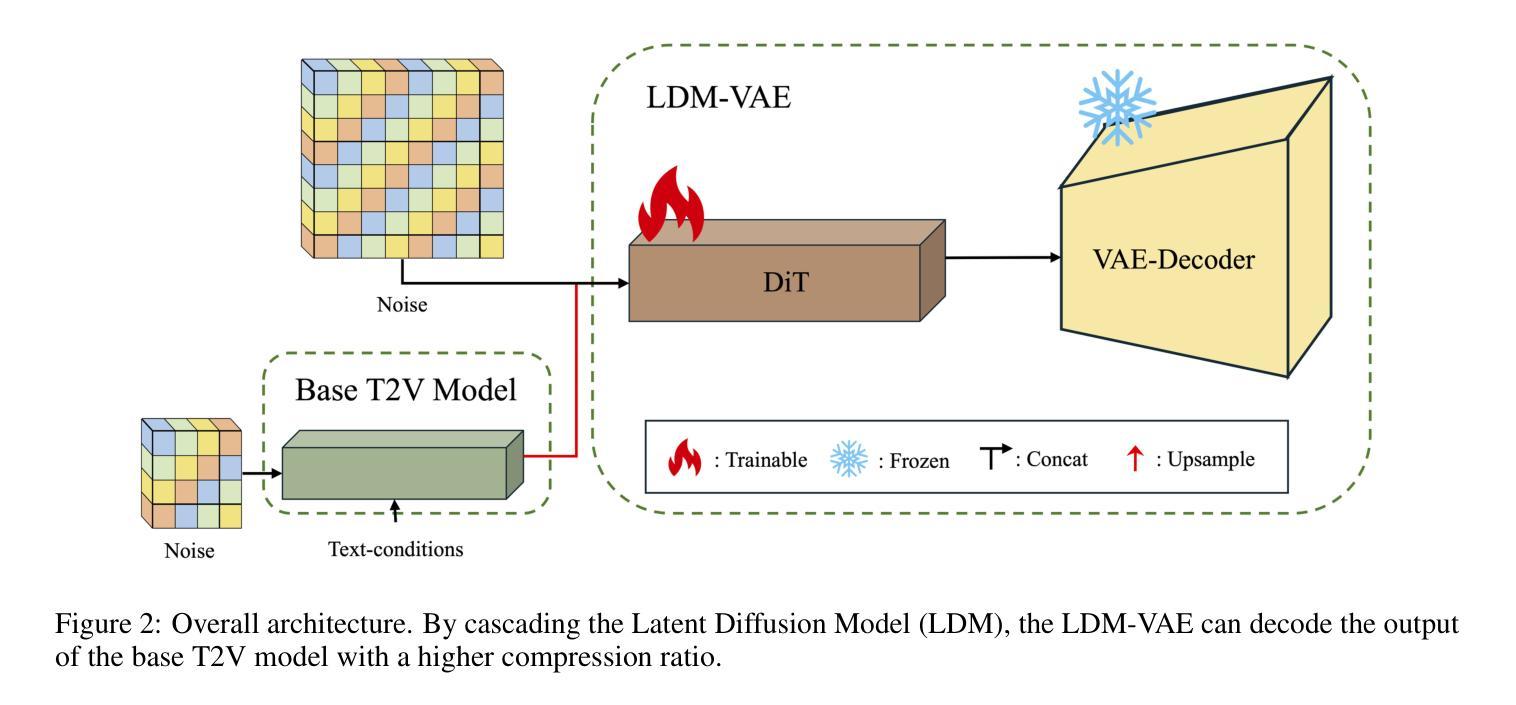
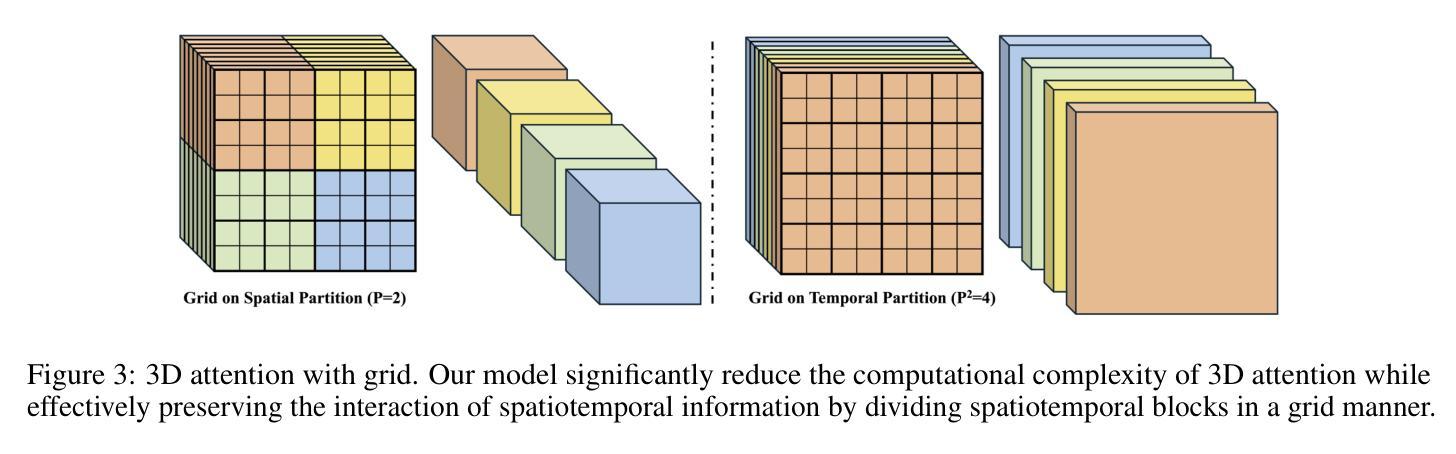
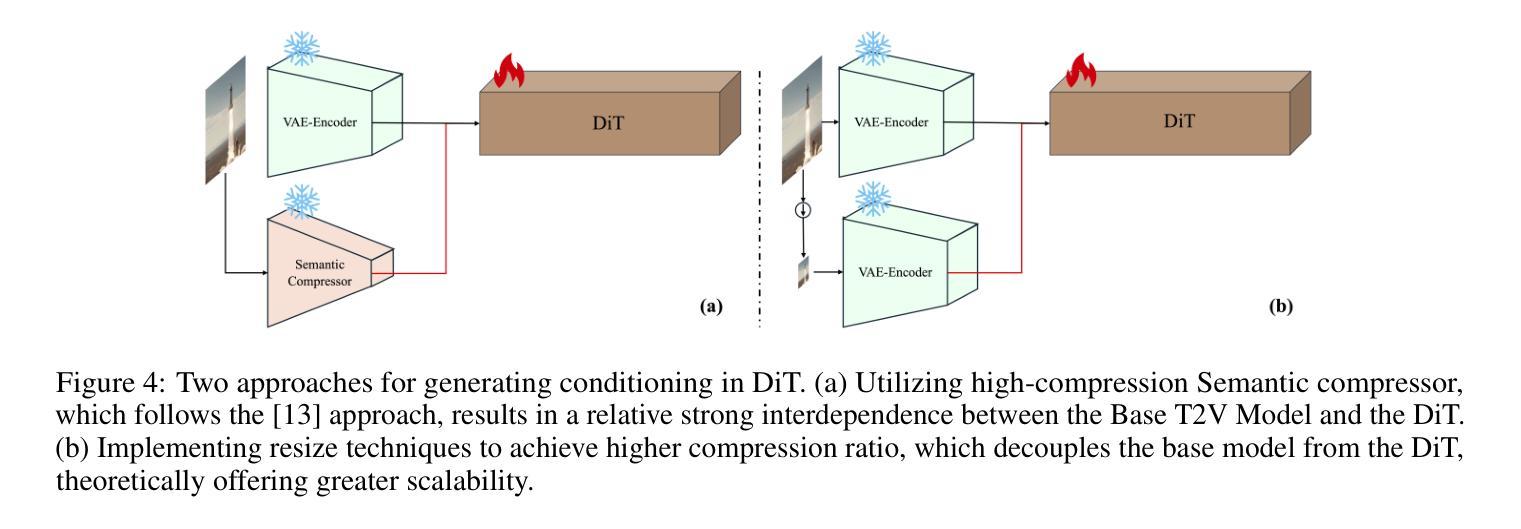
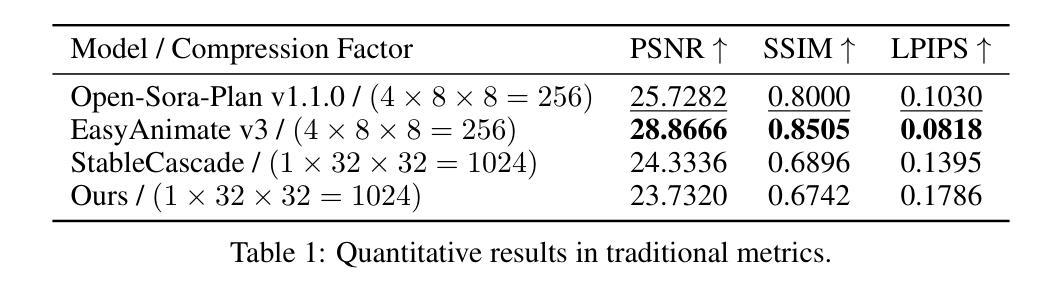
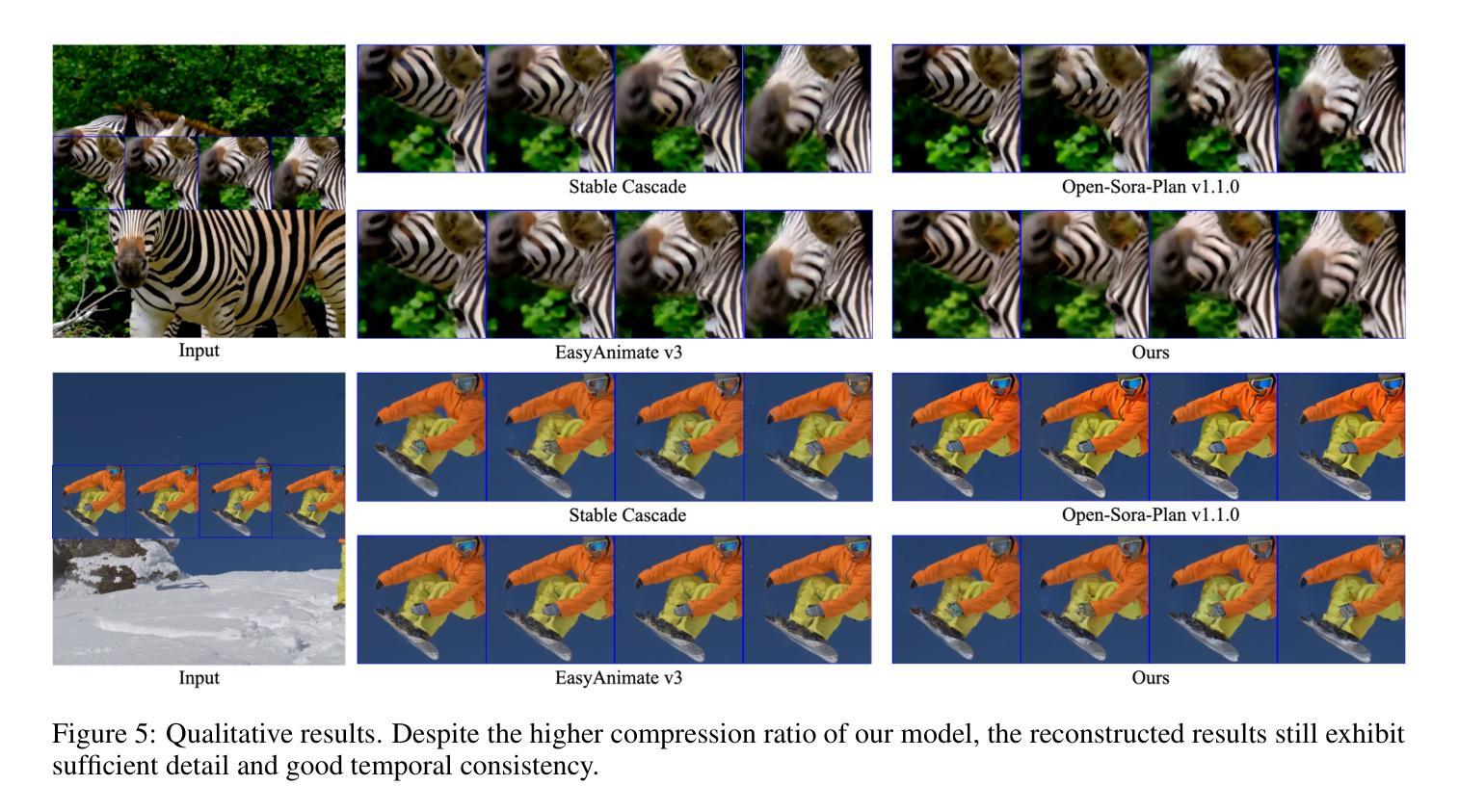
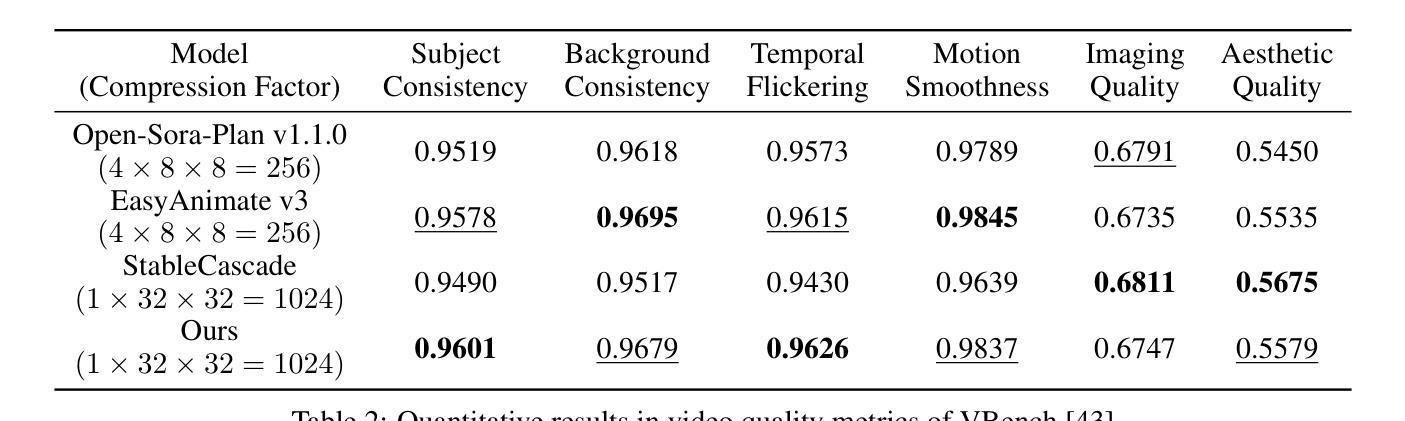
Slot-Guided Adaptation of Pre-trained Diffusion Models for Object-Centric Learning and Compositional Generation
Authors:Adil Kaan Akan, Yucel Yemez
We present SlotAdapt, an object-centric learning method that combines slot attention with pretrained diffusion models by introducing adapters for slot-based conditioning. Our method preserves the generative power of pretrained diffusion models, while avoiding their text-centric conditioning bias. We also incorporate an additional guidance loss into our architecture to align cross-attention from adapter layers with slot attention. This enhances the alignment of our model with the objects in the input image without using external supervision. Experimental results show that our method outperforms state-of-the-art techniques in object discovery and image generation tasks across multiple datasets, including those with real images. Furthermore, we demonstrate through experiments that our method performs remarkably well on complex real-world images for compositional generation, in contrast to other slot-based generative methods in the literature. The project page can be found at https://kaanakan.github.io/SlotAdapt/.
我们提出了SlotAdapt,这是一种结合插槽注意力和预训练扩散模型的面向对象的学习方法,它通过引入适配器来实现基于插槽的条件。我们的方法保留了预训练扩散模型的生成能力,同时避免了其面向文本的条件偏差。我们还将额外的指导损失纳入我们的架构,以调整适配器层的交叉注意力与插槽注意力。这增强了我们的模型与输入图像中的对象的对齐性,而无需使用外部监督。实验结果表明,我们的方法在多个数据集上的对象发现和图像生成任务中优于最先进的技术,包括那些带有真实图像的数据集。此外,通过试验,我们证明我们的方法在复杂真实图像的合成生成方面表现出色,与文献中的其他基于插槽的生成方法相比具有显著优势。项目页面位于https://kaanakan.github.io/SlotAdapt/。
论文及项目相关链接
PDF Accepted to ICLR2025. Project page: https://kaanakan.github.io/SlotAdapt/
Summary
SlotAdapt结合槽位注意力和预训练扩散模型,通过引入适配器进行槽位条件化,实现对象级学习。该方法在保留预训练扩散模型的生成能力的同时,避免了其文本中心化的条件偏差。通过引入额外的指导损失,增强模型与输入图像中对象的对齐度,无需外部监督。实验表明,该方法在多个数据集上的物体发现和图像生成任务上表现优异,尤其擅长处理复杂真实图像的合成生成。
Key Takeaways
- SlotAdapt结合了槽位注意力和预训练的扩散模型。
- 通过引入适配器实现槽位条件化,保留扩散模型的生成能力。
- 方法避免了文本中心化的条件偏差。
- 通过引入额外的指导损失增强模型与输入图像中对象的对齐。
- 该方法在不同数据集上的物体发现和图像生成任务上表现优异。
- 特别擅长处理复杂真实图像的合成生成。
点此查看论文截图
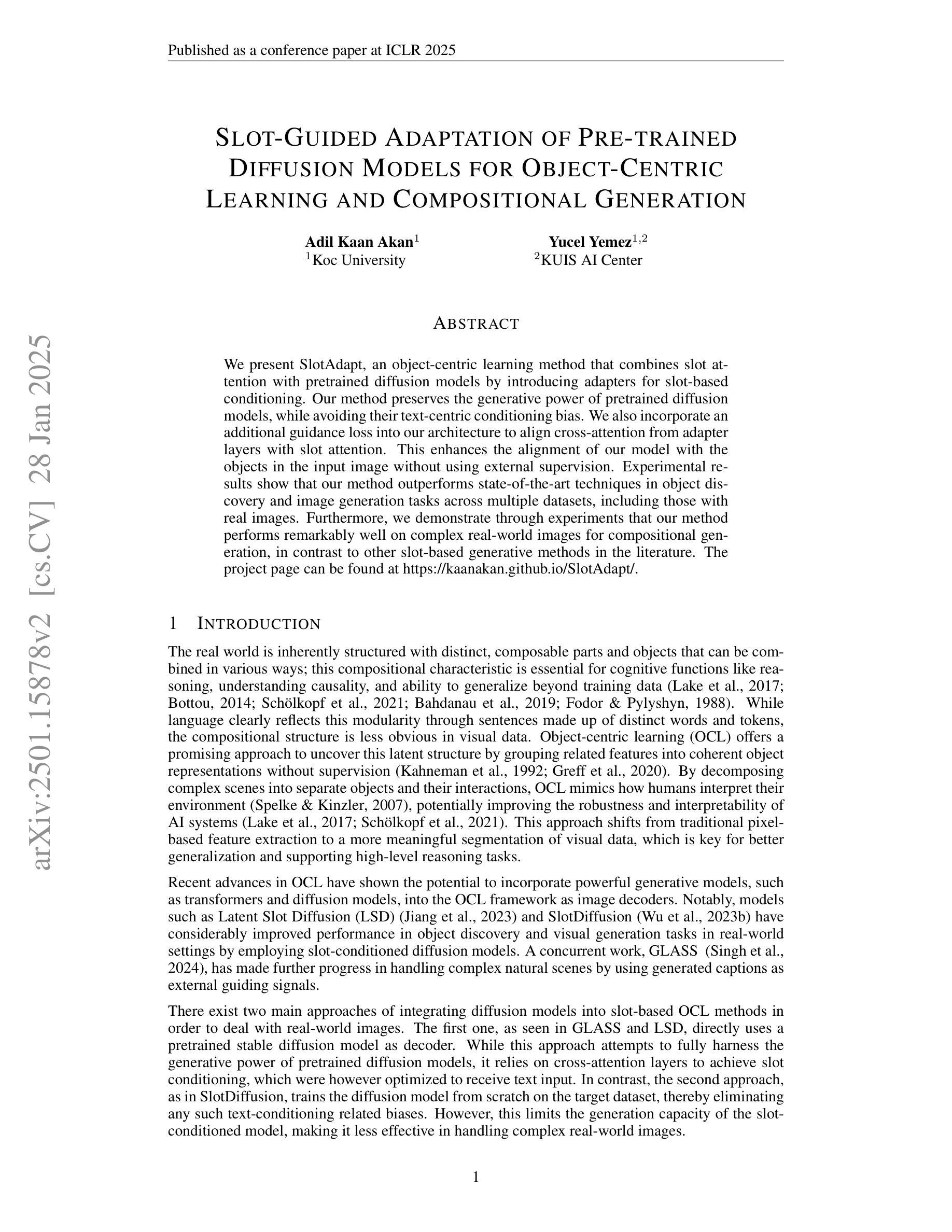
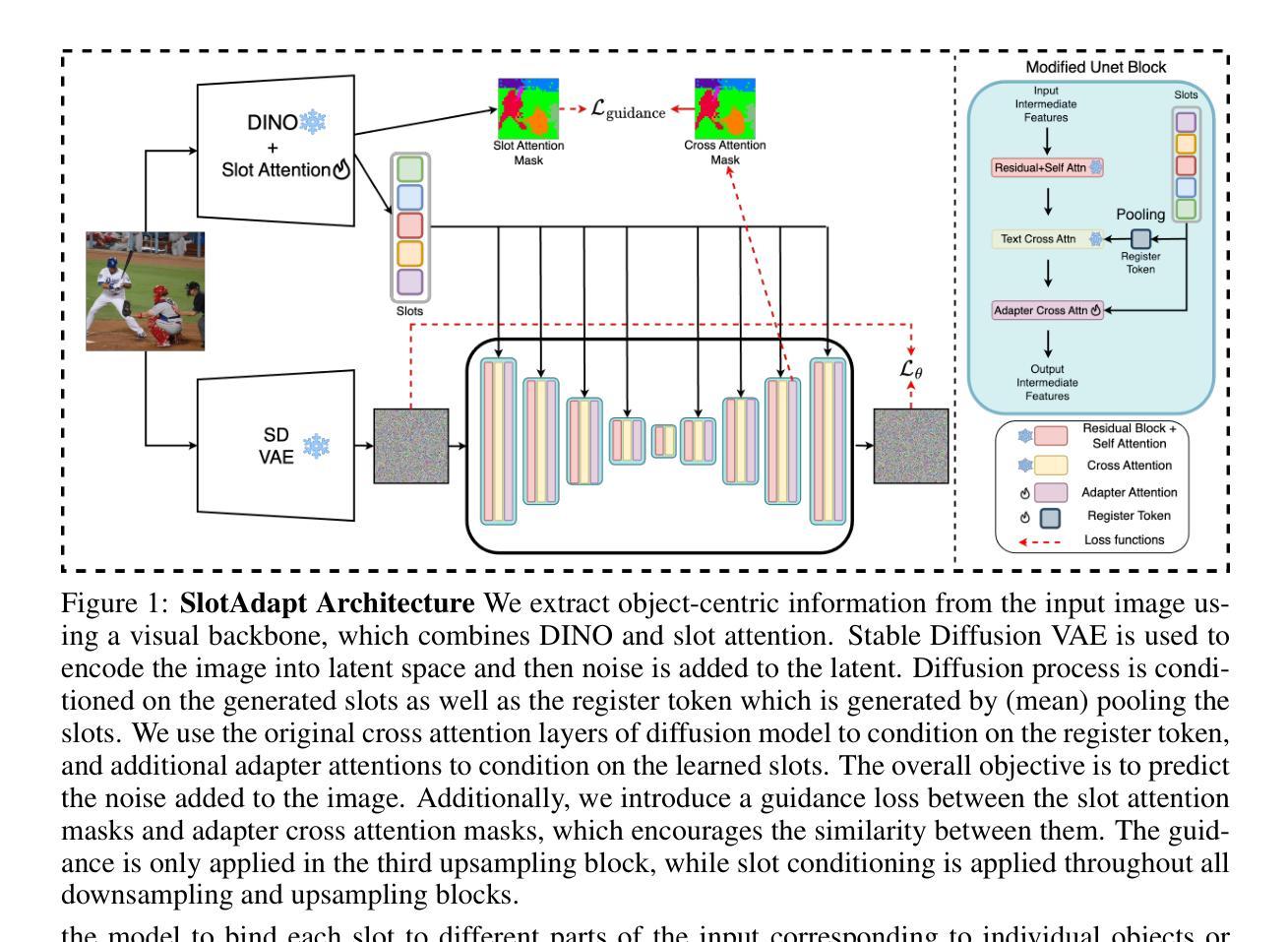
Label-Efficient Data Augmentation with Video Diffusion Models for Guidewire Segmentation in Cardiac Fluoroscopy
Authors:Shaoyan Pan, Yikang Liu, Lin Zhao, Eric Z. Chen, Xiao Chen, Terrence Chen, Shanhui Sun
The accurate segmentation of guidewires in interventional cardiac fluoroscopy videos is crucial for computer-aided navigation tasks. Although deep learning methods have demonstrated high accuracy and robustness in wire segmentation, they require substantial annotated datasets for generalizability, underscoring the need for extensive labeled data to enhance model performance. To address this challenge, we propose the Segmentation-guided Frame-consistency Video Diffusion Model (SF-VD) to generate large collections of labeled fluoroscopy videos, augmenting the training data for wire segmentation networks. SF-VD leverages videos with limited annotations by independently modeling scene distribution and motion distribution. It first samples the scene distribution by generating 2D fluoroscopy images with wires positioned according to a specified input mask, and then samples the motion distribution by progressively generating subsequent frames, ensuring frame-to-frame coherence through a frame-consistency strategy. A segmentation-guided mechanism further refines the process by adjusting wire contrast, ensuring a diverse range of visibility in the synthesized image. Evaluation on a fluoroscopy dataset confirms the superior quality of the generated videos and shows significant improvements in guidewire segmentation.
在介入心脏荧光透视视频中,对导线进行准确的分割对于计算机辅助导航任务至关重要。虽然深度学习的方法在导线分割方面已经显示出高准确度和稳健性,但它们需要大规模的标注数据集来实现普遍适用性,这凸显了对大量标注数据的需要,以提高模型性能。为了解决这一挑战,我们提出了“基于分割引导的帧一致性视频扩散模型(SF-VD)”,用于生成大量标记的荧光透视视频,增强导线分割网络的训练数据。SF-VD通过独立建模场景分布和运动分布来利用有限的标注视频。它通过根据指定的输入掩码生成带有导线的二维荧光透视图像来采样场景分布,然后通过逐步生成后续帧来采样运动分布,并通过帧一致性策略确保帧与帧之间的连贯性。分割引导机制进一步调整了导线对比度,确保合成图像的可见性范围多样化。在荧光数据集上的评估证实了所生成视频的卓越质量,并显示出导线分割的显著改善。
论文及项目相关链接
PDF AAAI 2025
Summary
在心脏介入手术荧光透视视频中准确分割导丝对于计算机辅助导航任务至关重要。针对深度学习方法需要大量标注数据集以提高导丝分割的普遍性问题,我们提出了基于分割引导的帧一致性视频扩散模型(SF-VD)。该模型能够生成大量标注的荧光透视视频,增强导丝分割网络的训练数据。SF-VD通过独立建模场景分布和运动分布,利用有限标注的视频进行工作。它首先根据指定的输入掩膜生成二维荧光透视图像来采样场景分布,然后通过逐步生成后续帧来采样运动分布,确保帧间一致性。分割引导机制进一步调整导丝对比度,确保合成图像的可见性多样性。在荧光数据集上的评估证明了生成视频的高质量,并显示出在导丝分割方面的显著改善。
Key Takeaways
- 导丝在心脏介入手术荧光透视视频中的准确分割对计算机辅助导航至关重要。
- 深度学习方法虽能高精度、稳健地进行导丝分割,但需大量标注数据以改善模型性能。
- 提出基于分割引导的帧一致性视频扩散模型(SF-VD)以生成大量标注的荧光透视视频,增强训练数据。
- SF-VD通过独立建模场景分布和运动分布来工作。
- 模型通过生成二维荧光透视图像采样场景分布,并通过逐步生成后续帧来确保帧间一致性。
- 分割引导机制调整导丝对比度,确保合成图像的可见性多样性。
点此查看论文截图
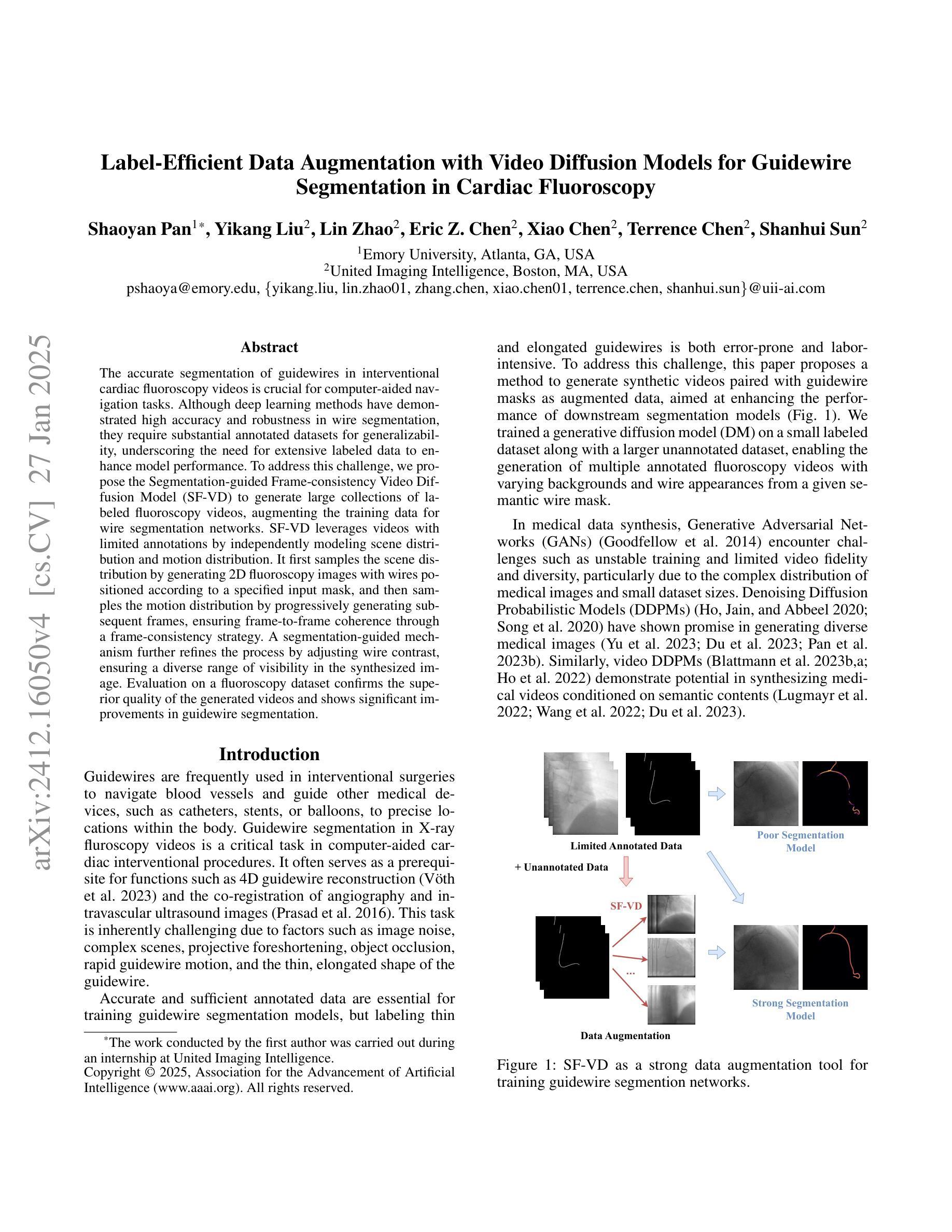
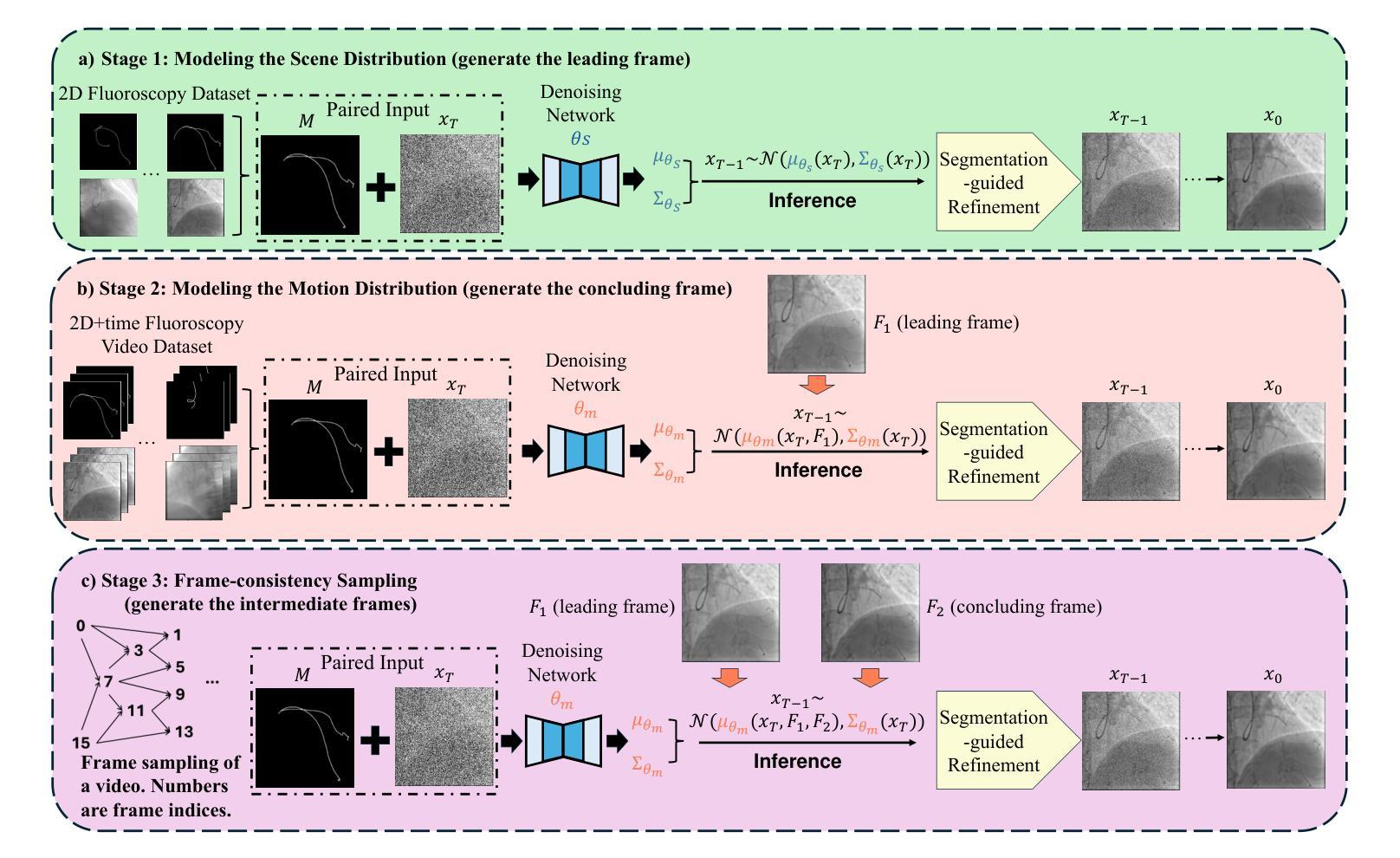
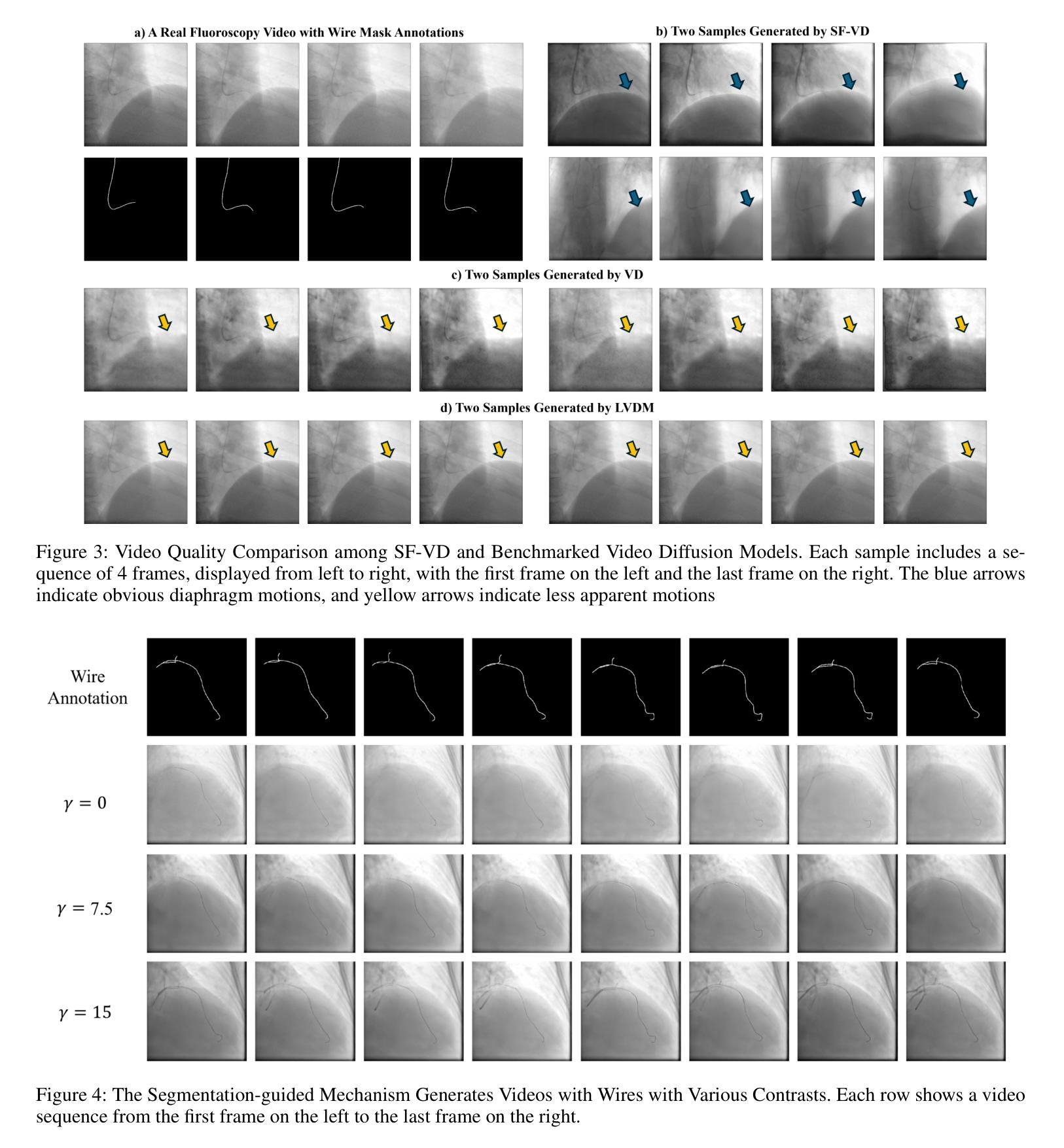
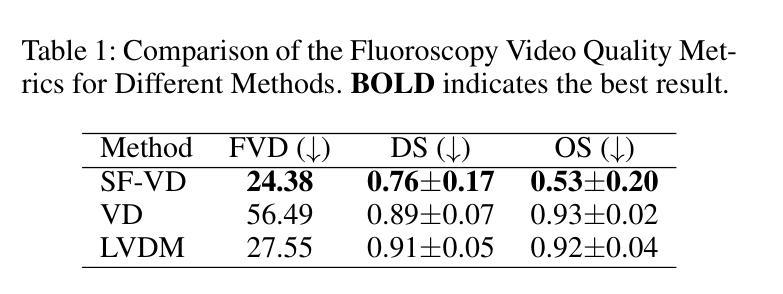
StableMaterials: Enhancing Diversity in Material Generation via Semi-Supervised Learning
Authors:Giuseppe Vecchio
We introduce StableMaterials, a novel approach for generating photorealistic physical-based rendering (PBR) materials that integrate semi-supervised learning with Latent Diffusion Models (LDMs). Our method employs adversarial training to distill knowledge from existing large-scale image generation models, minimizing the reliance on annotated data and enhancing the diversity in generation. This distillation approach aligns the distribution of the generated materials with that of image textures from an SDXL model, enabling the generation of novel materials that are not present in the initial training dataset. Furthermore, we employ a diffusion-based refiner model to improve the visual quality of the samples and achieve high-resolution generation. Finally, we distill a latent consistency model for fast generation in just four steps and propose a new tileability technique that removes visual artifacts typically associated with fewer diffusion steps. We detail the architecture and training process of StableMaterials, the integration of semi-supervised training within existing LDM frameworks and show the advantages of our approach. Comparative evaluations with state-of-the-art methods show the effectiveness of StableMaterials, highlighting its potential applications in computer graphics and beyond. StableMaterials is publicly available at https://gvecchio.com/stablematerials.
我们介绍了StableMaterials,这是一种基于物理渲染(PBR)材料生成的新型方法,它将半监督学习与潜在扩散模型(LDM)相结合。我们的方法采用对抗训练从现有的大规模图像生成模型中提炼知识,减少对标注数据的依赖,并增强生成的多样性。这种提炼方法使生成材料的分布与SDXL模型中的图像纹理分布相一致,能够生成初始训练数据集中不存在的新材料。此外,我们采用基于扩散的细化模型来提高样本的视觉质量,实现高分辨率生成。最后,我们提炼了一个潜在一致性模型,只需四个步骤即可快速生成,并提出了一种新的可平铺技术,消除了由于较少的扩散步骤而通常出现的视觉伪影。我们详细介绍了StableMaterials的架构和训练过程,以及在现有LDM框架内半监督训练的集成,展示了我们的优势。与最新方法的比较评估表明,StableMaterials是有效的,突出了其在计算机图形等领域的应用潜力。StableMaterials可在https://gvecchio.com/stablematerials公开访问。
论文及项目相关链接
Summary
StableMaterials是一种结合半监督学习与潜在扩散模型(Latent Diffusion Models,LDM)生成真实物理渲染(PBR)材料的新方法。该方法采用对抗训练从现有大规模图像生成模型中提炼知识,减少了对标注数据的依赖,提高了生成的多样性。通过蒸馏方法与SDXL模型图像纹理的分布对齐,生成不存在于初始训练集中的新材料。此外,使用基于扩散的细化模型提高样本的视觉质量,实现高分辨率生成。最后,我们提炼了一个具有快速四步生成能力的潜在一致性模型,并提出一种新的去瓦技术,消除了因减少扩散步骤而产生的典型视觉伪影。StableMaterials的优势在于其架构和训练过程的细节,以及其在计算机图形等领域的应用潜力。
Key Takeaways
- StableMaterials结合了半监督学习与潜在扩散模型(LDM)生成真实物理渲染(PBR)材料。
- 通过对抗训练从大规模图像生成模型中提炼知识,减少标注数据依赖并提高生成多样性。
- 生成的材料与SDXL模型图像纹理分布对齐,能生成初始训练集中不存在的新材料。
- 使用基于扩散的细化模型提高样本的视觉质量,实现高分辨率生成。
- 提炼了一个快速四步生成的潜在一致性模型。
- 提出新的去瓦技术,消除因减少扩散步骤而产生的视觉伪影。
点此查看论文截图
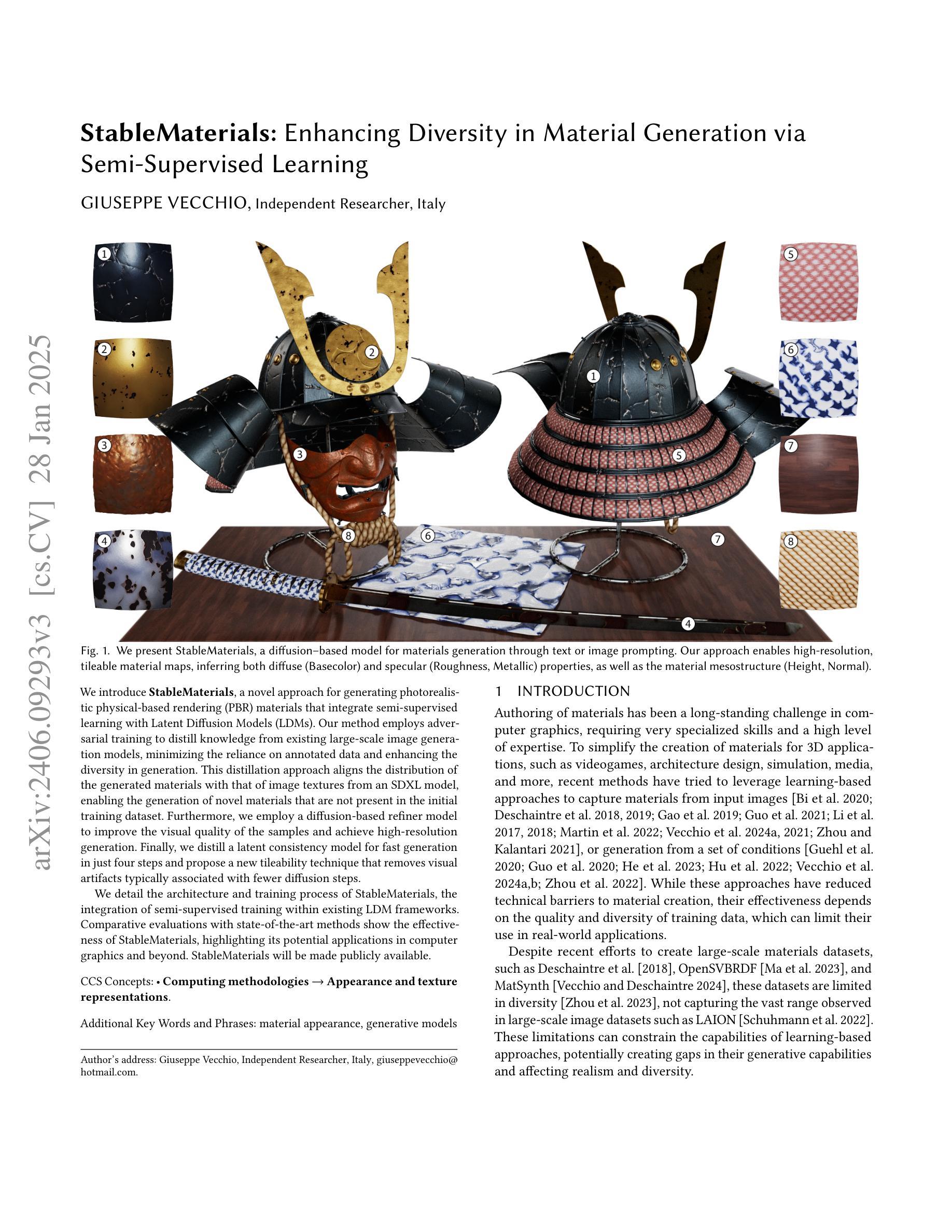
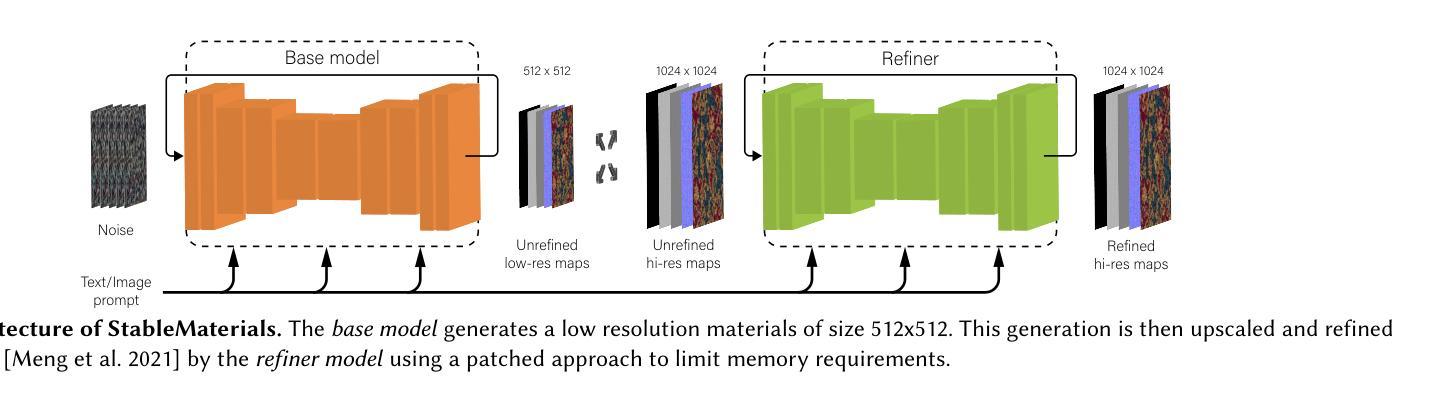
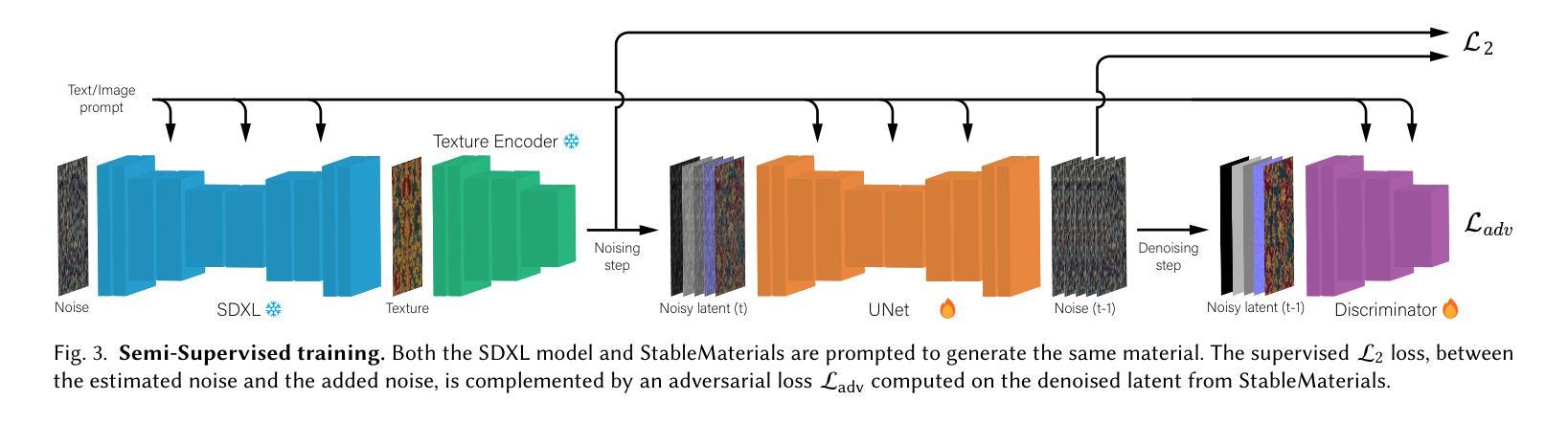
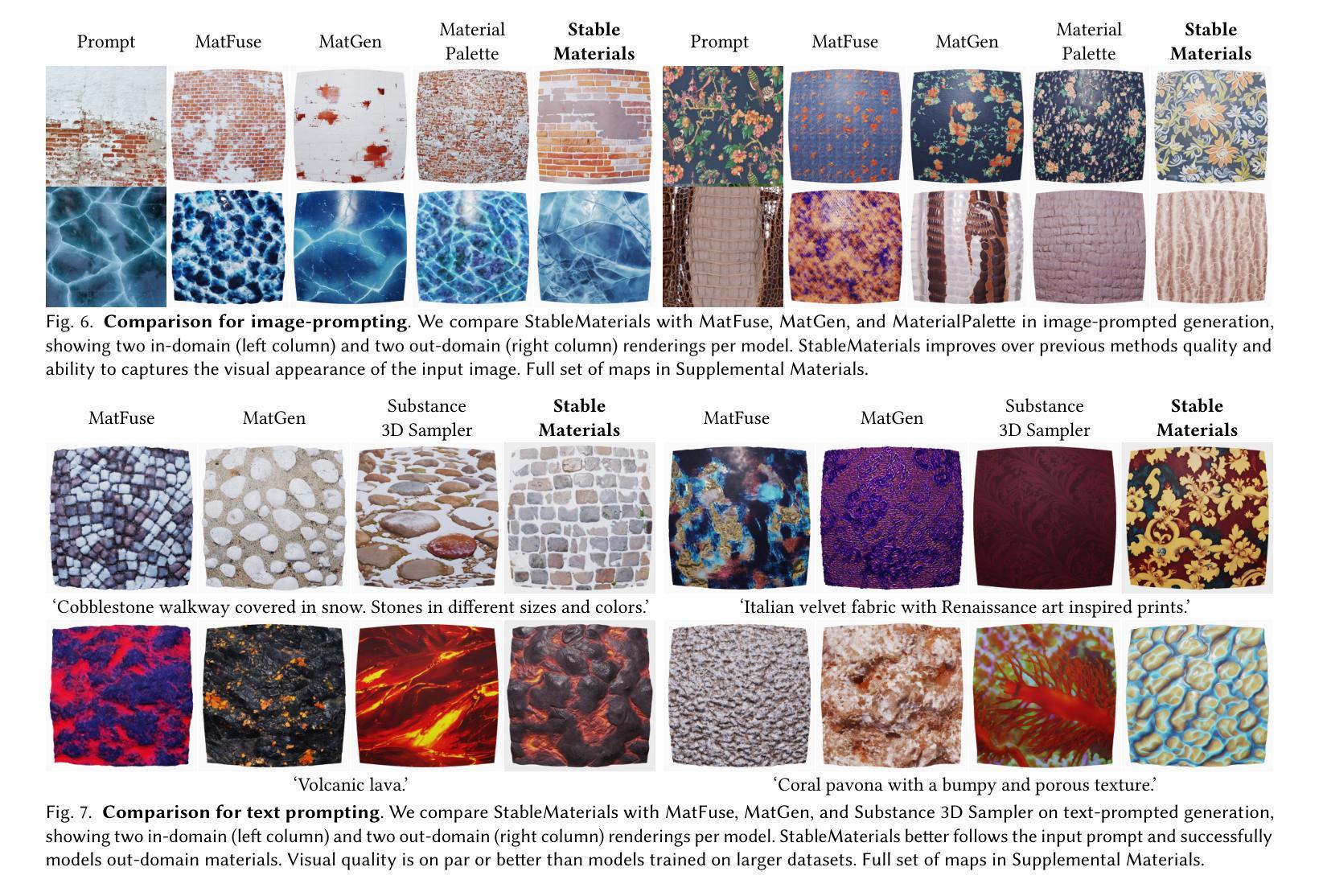
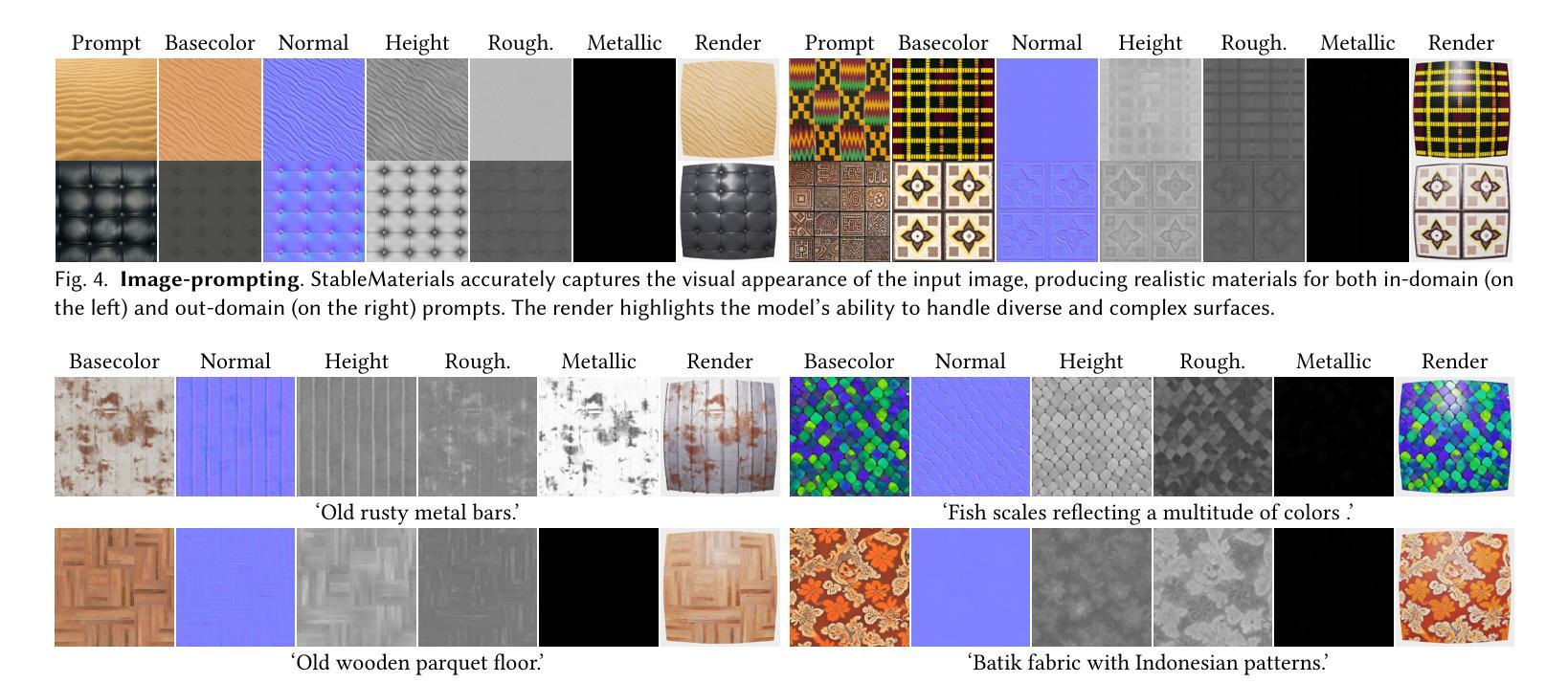