⚠️ 以下所有内容总结都来自于 大语言模型的能力,如有错误,仅供参考,谨慎使用
🔴 请注意:千万不要用于严肃的学术场景,只能用于论文阅读前的初筛!
💗 如果您觉得我们的项目对您有帮助 ChatPaperFree ,还请您给我们一些鼓励!⭐️ HuggingFace免费体验
2025-01-30 更新
EmoFace: Emotion-Content Disentangled Speech-Driven 3D Talking Face Animation
Authors:Yihong Lin, Liang Peng, Xianjia Wu, Jianqiao Hu, Xiandong Li, Wenxiong Kang, Songju Lei, Huang Xu
The creation of increasingly vivid 3D talking face has become a hot topic in recent years. Currently, most speech-driven works focus on lip synchronisation but neglect to effectively capture the correlations between emotions and facial motions. To address this problem, we propose a two-stream network called EmoFace, which consists of an emotion branch and a content branch. EmoFace employs a novel Mesh Attention mechanism to analyse and fuse the emotion features and content features. Particularly, a newly designed spatio-temporal graph-based convolution, SpiralConv3D, is used in Mesh Attention to learn potential temporal and spatial feature dependencies between mesh vertices. In addition, to the best of our knowledge, it is the first time to introduce a new self-growing training scheme with intermediate supervision to dynamically adjust the ratio of groundtruth adopted in the 3D face animation task. Comprehensive quantitative and qualitative evaluations on our high-quality 3D emotional facial animation dataset, 3D-RAVDESS ($4.8863\times 10^{-5}$mm for LVE and $0.9509\times 10^{-5}$mm for EVE), together with the public dataset VOCASET ($2.8669\times 10^{-5}$mm for LVE and $0.4664\times 10^{-5}$mm for EVE), demonstrate that our approach achieves state-of-the-art performance.
近年来,创建越来越逼真的3D对话人脸已成为热门话题。目前,大多数语音驱动的研究工作主要集中在唇同步上,而忽视了情绪与面部动作之间的关联的有效捕捉。为了解决这个问题,我们提出了一种名为EmoFace的两流网络,它包括情感分支和内容分支。EmoFace采用了一种新型的Mesh Attention机制来分析并融合情感特征和内容特征。特别是,新设计的基于时空图的卷积操作SpiralConv3D,在Mesh Attention中用于学习网格顶点之间潜在的临时和空间特征依赖性。此外,据我们所知,首次引入了一种新的自我成长训练方案,带有中间监督,以动态调整用于3D人脸动画任务的真实比例。在我们高质量的三维情感面部动画数据集3D-RAVDESS(LVE为$4.8863\times 10^{-5}$mm,EVE为$0.9509\times 10^{-5}$mm)以及公共数据集VOCASET(LVE为$2.8669\times 10^{-5}$mm,EVE为$0.4664\times 10^{-5}$mm)上的定量和定性评估综合表明,我们的方法达到了最先进的性能。
论文及项目相关链接
Summary
本文提出了一个名为EmoFace的两流网络,包含情感分支和内容分支,以解决当前语音驱动模型忽视情感与面部动作关联的问题。该网络采用新颖的Mesh Attention机制和螺旋卷积技术,有效学习和融合情感与内容的特征。此外,本文首次引入自我成长训练方案,通过中间监督动态调整地面真实数据在3D面部动画任务中的比例。在高质量的情感面部动画数据集上的表现达到了行业前沿水平。
Key Takeaways
- EmoFace网络包含情感分支和内容分支,旨在解决语音驱动模型中忽视情感与面部动作关联的问题。
- Mesh Attention机制用于分析和融合情感特征和内容特征。
- SpiralConv3D技术用于学习潜在的时间空间特征依赖性。
- 自我成长训练方案首次引入,通过中间监督动态调整地面真实数据的使用比例。
点此查看论文截图
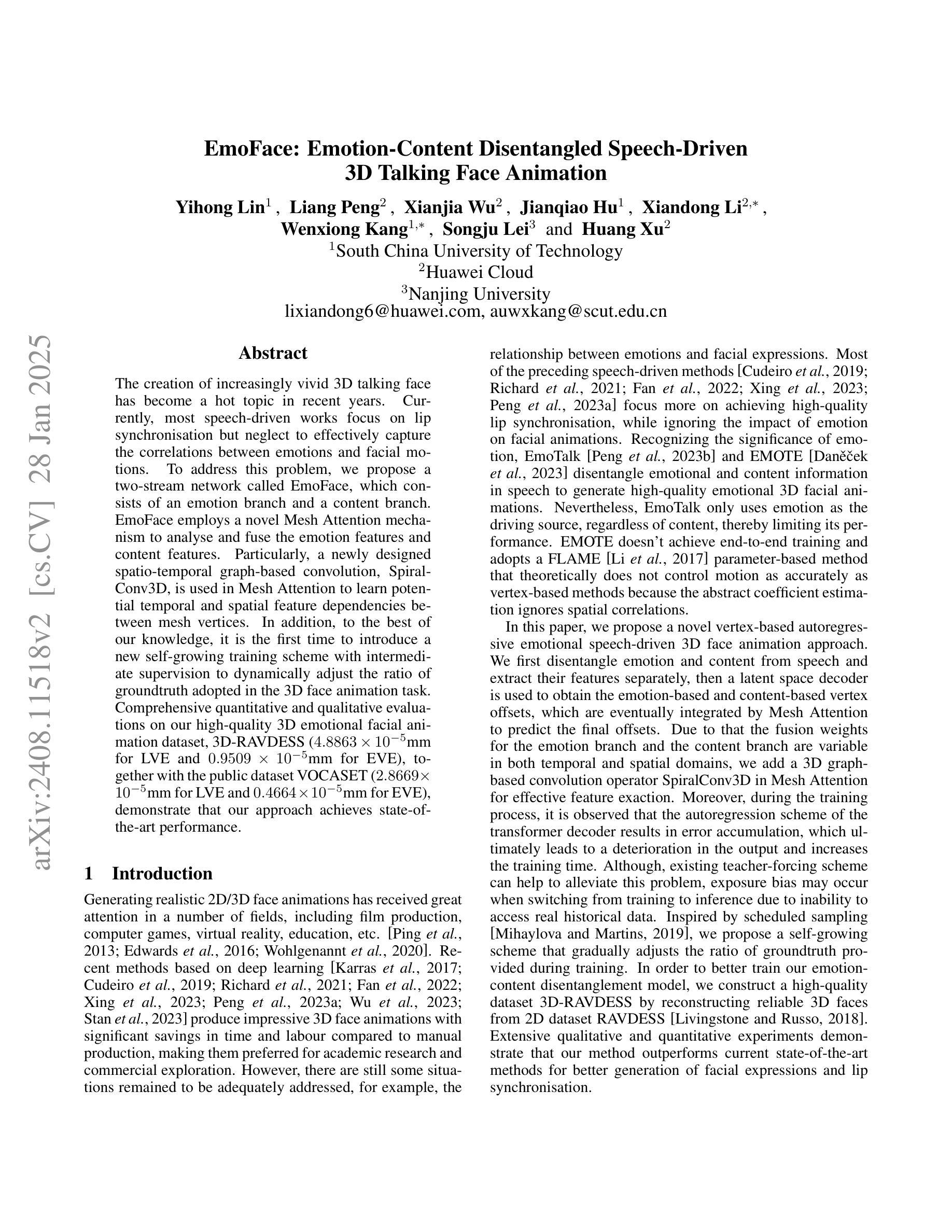
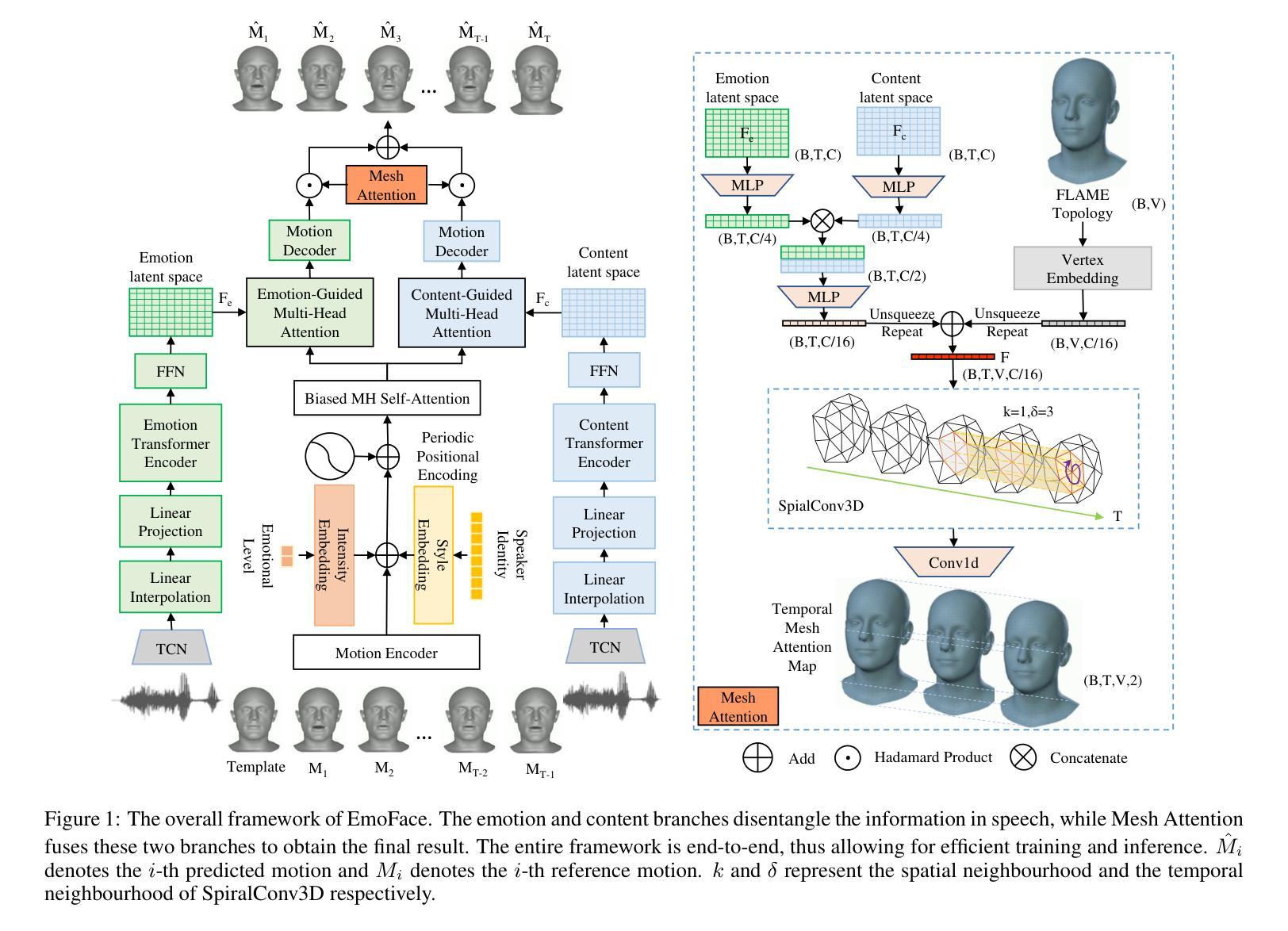
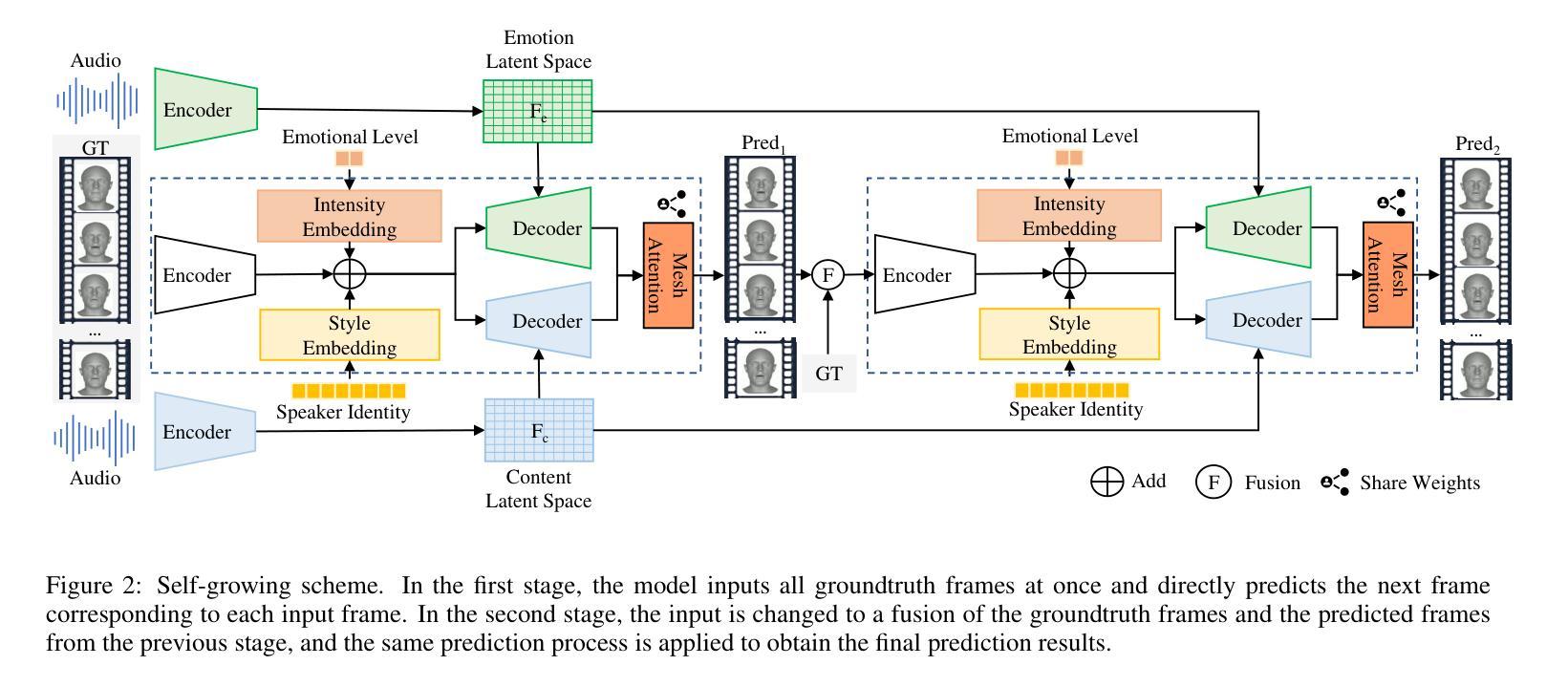
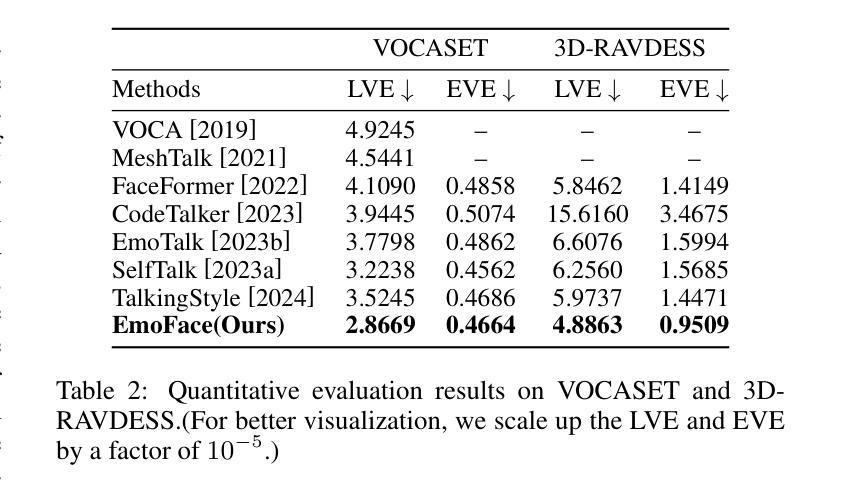
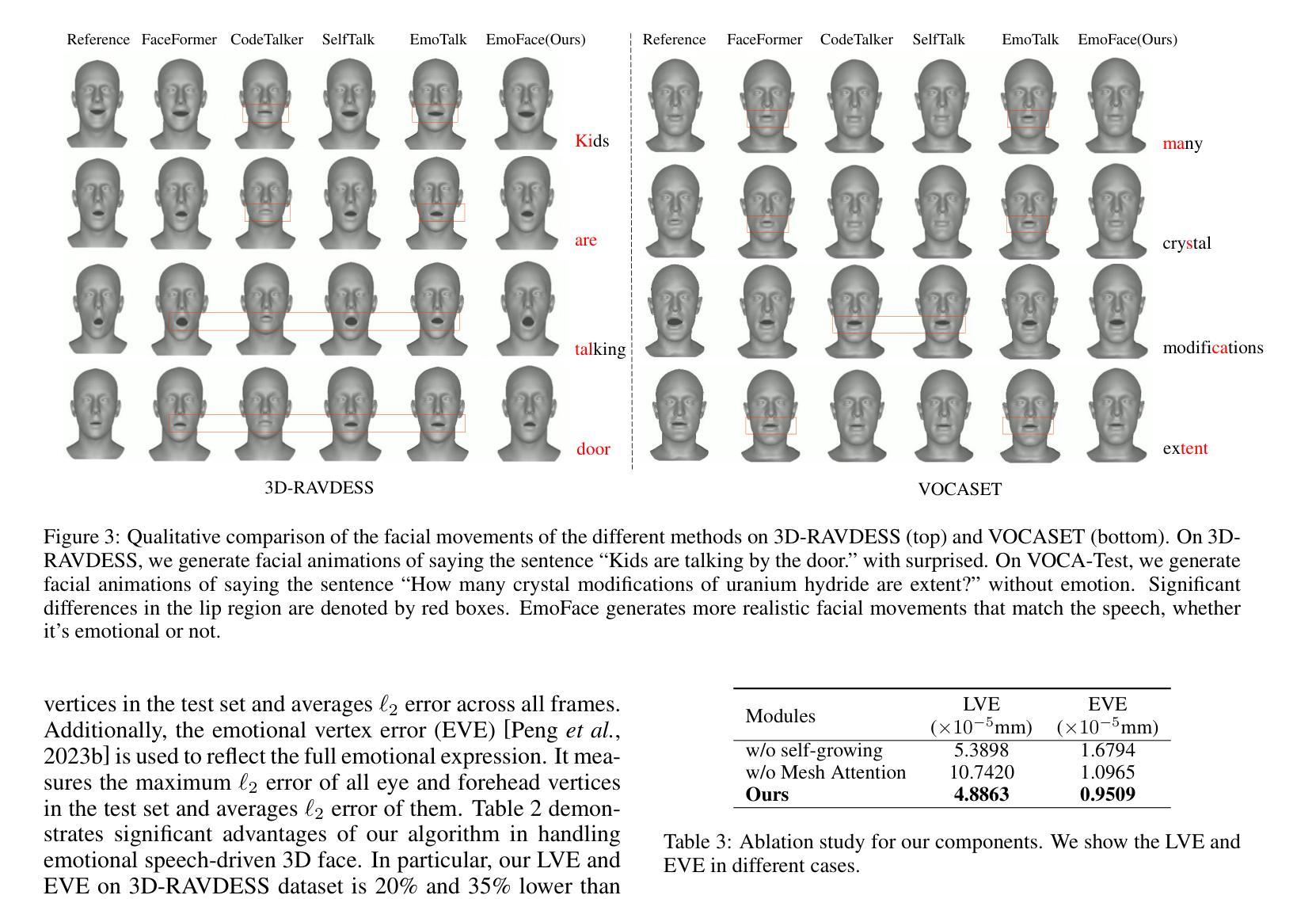
GLDiTalker: Speech-Driven 3D Facial Animation with Graph Latent Diffusion Transformer
Authors:Yihong Lin, Zhaoxin Fan, Xianjia Wu, Lingyu Xiong, Liang Peng, Xiandong Li, Wenxiong Kang, Songju Lei, Huang Xu
Speech-driven talking head generation is a critical yet challenging task with applications in augmented reality and virtual human modeling. While recent approaches using autoregressive and diffusion-based models have achieved notable progress, they often suffer from modality inconsistencies, particularly misalignment between audio and mesh, leading to reduced motion diversity and lip-sync accuracy. To address this, we propose GLDiTalker, a novel speech-driven 3D facial animation model based on a Graph Latent Diffusion Transformer. GLDiTalker resolves modality misalignment by diffusing signals within a quantized spatiotemporal latent space. It employs a two-stage training pipeline: the Graph-Enhanced Quantized Space Learning Stage ensures lip-sync accuracy, while the Space-Time Powered Latent Diffusion Stage enhances motion diversity. Together, these stages enable GLDiTalker to generate realistic, temporally stable 3D facial animations. Extensive evaluations on standard benchmarks demonstrate that GLDiTalker outperforms existing methods, achieving superior results in both lip-sync accuracy and motion diversity.
语音驱动说话人头部生成是一项重要且具有挑战性的任务,在增强现实和虚拟人类建模中具有广泛的应用。虽然最近使用自回归和扩散模型的方法已经取得了明显的进步,但它们经常遭受模态不一致的问题,特别是音频和网格之间的不匹配,导致运动多样性和唇同步准确性降低。为了解决这一问题,我们提出了GLDiTalker,这是一个基于图潜在扩散变压器的新型语音驱动3D面部动画模型。GLDiTalker通过量化时空潜在空间内的信号扩散来解决模态不匹配问题。它采用两阶段训练管道:图增强量化空间学习阶段确保唇同步准确性,而时空动力潜在扩散阶段增强运动多样性。这两个阶段共同使GLDiTalker能够生成逼真、时间稳定的3D面部动画。在标准基准测试上的广泛评估表明,GLDiTalker优于现有方法,在唇同步准确性和运动多样性方面都取得了优越的结果。
论文及项目相关链接
PDF 9 pages, 5 figures
Summary
基于Graph Latent Diffusion Transformer的语音驱动3D面部动画模型GLDiTalker解决了模态不一致的问题,特别是在音频和网格之间的不匹配问题。它通过在一个量化的时空潜在空间内扩散信号来解决模态不匹配问题。采用两阶段训练管道,提高了唇同步准确性和运动多样性,从而生成真实且时间稳定的3D面部动画。在标准基准测试上的广泛评估表明,GLDiTalker在唇同步准确性和运动多样性方面均优于现有方法。
Key Takeaways
- GLDiTalker是一种基于Graph Latent Diffusion Transformer的语音驱动3D面部动画模型。
- 该模型解决了模态不一致问题,特别是音频和网格之间的不匹配。
- GLDiTalker通过在一个量化的时空潜在空间内扩散信号来解决模态不匹配。
- 模型采用两阶段训练管道,包括Graph-Enhanced Quantized Space Learning Stage和Space-TimePowered Latent Diffusion Stage。
- 第一阶段确保唇同步准确性,第二阶段增强运动多样性。
- GLDiTalker能生成真实且时间稳定的3D面部动画。
点此查看论文截图
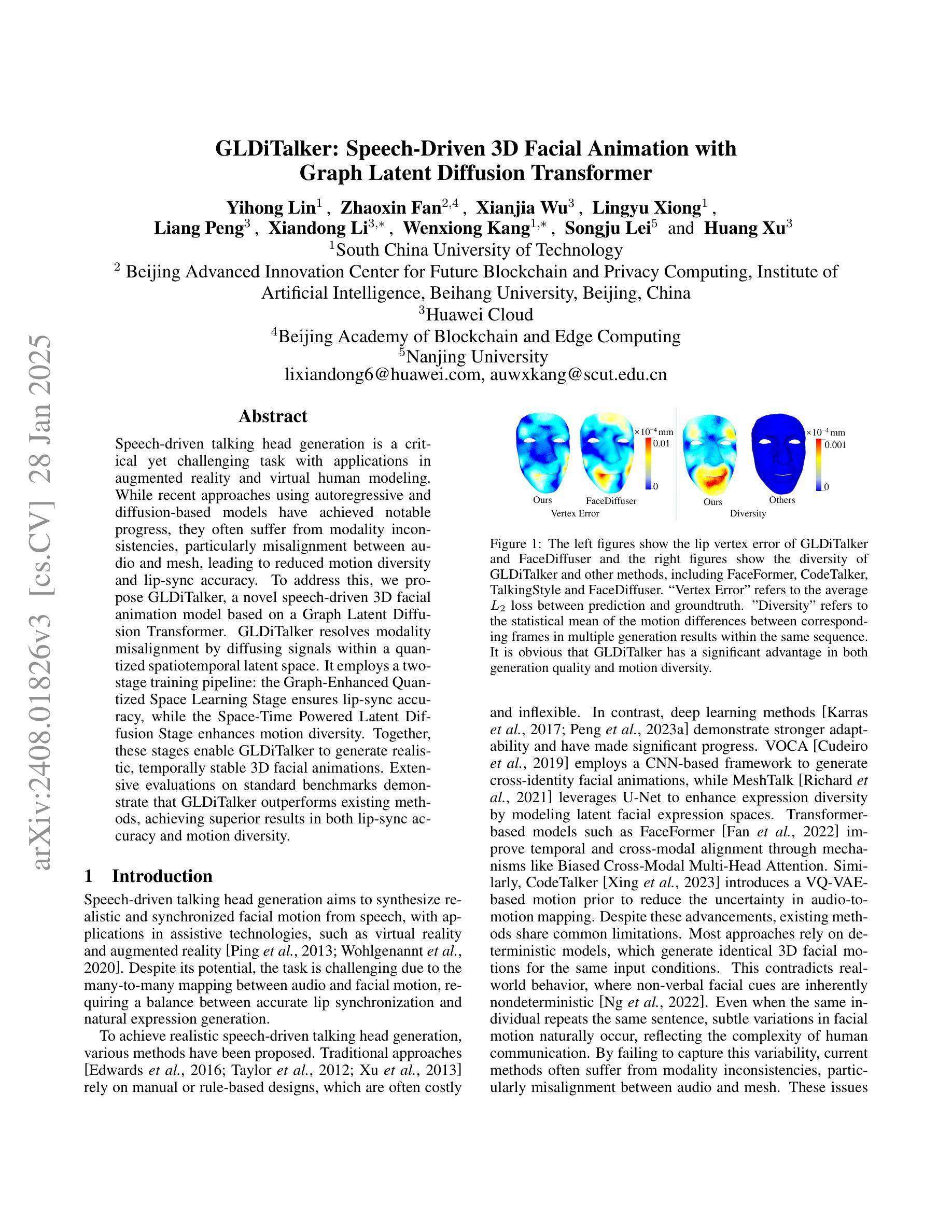
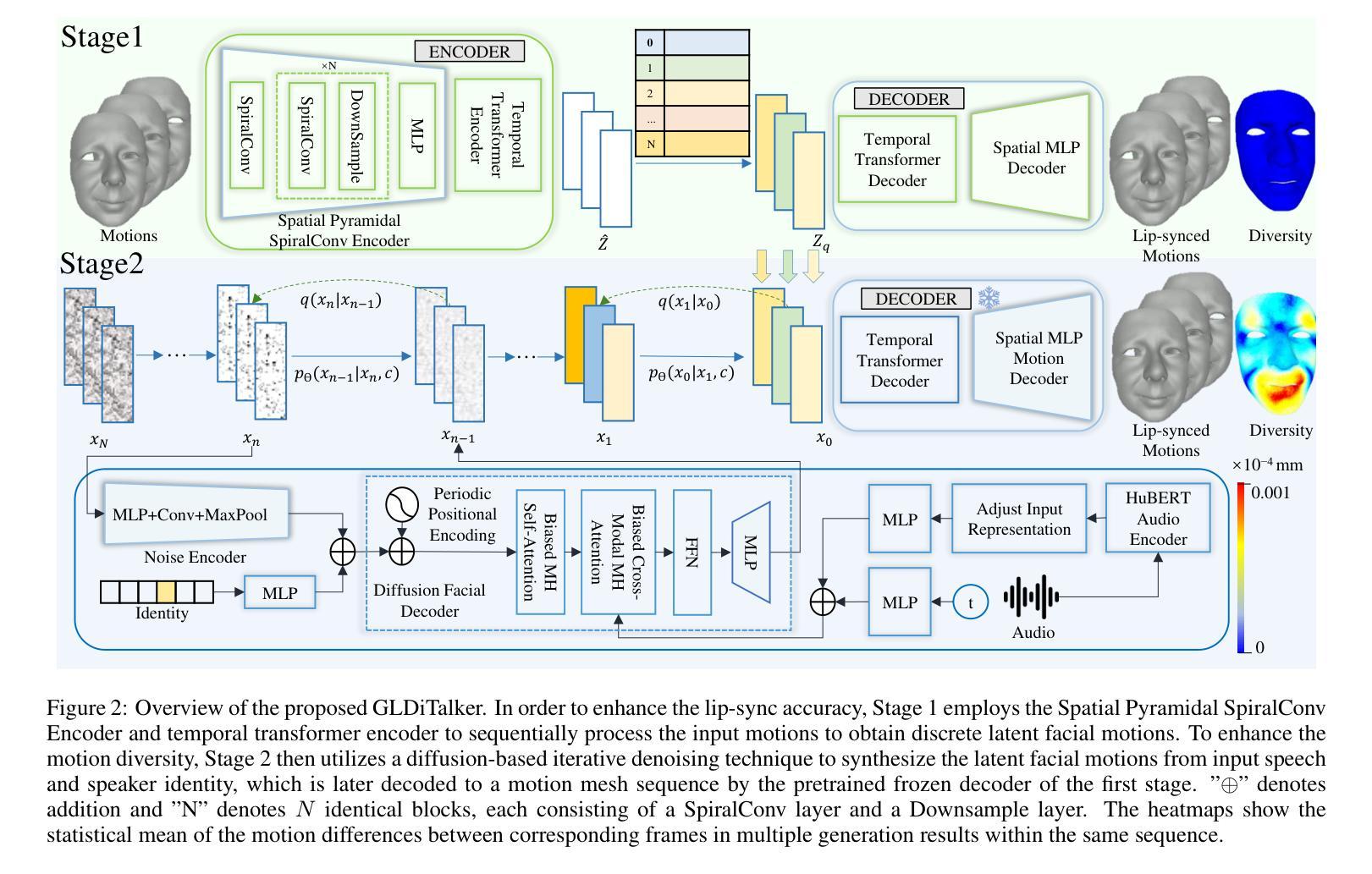
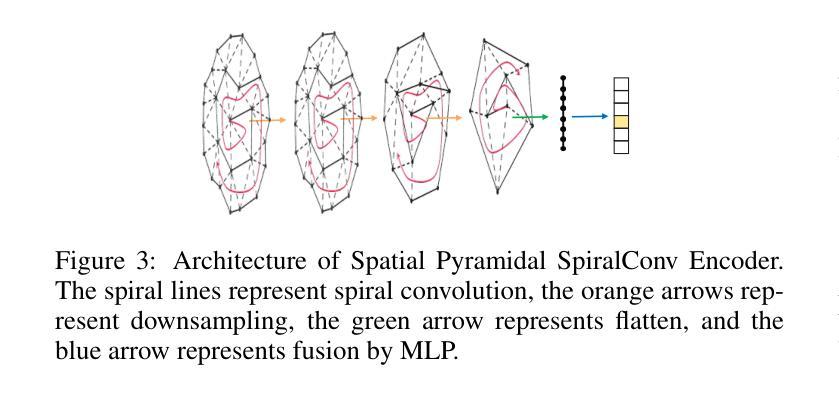
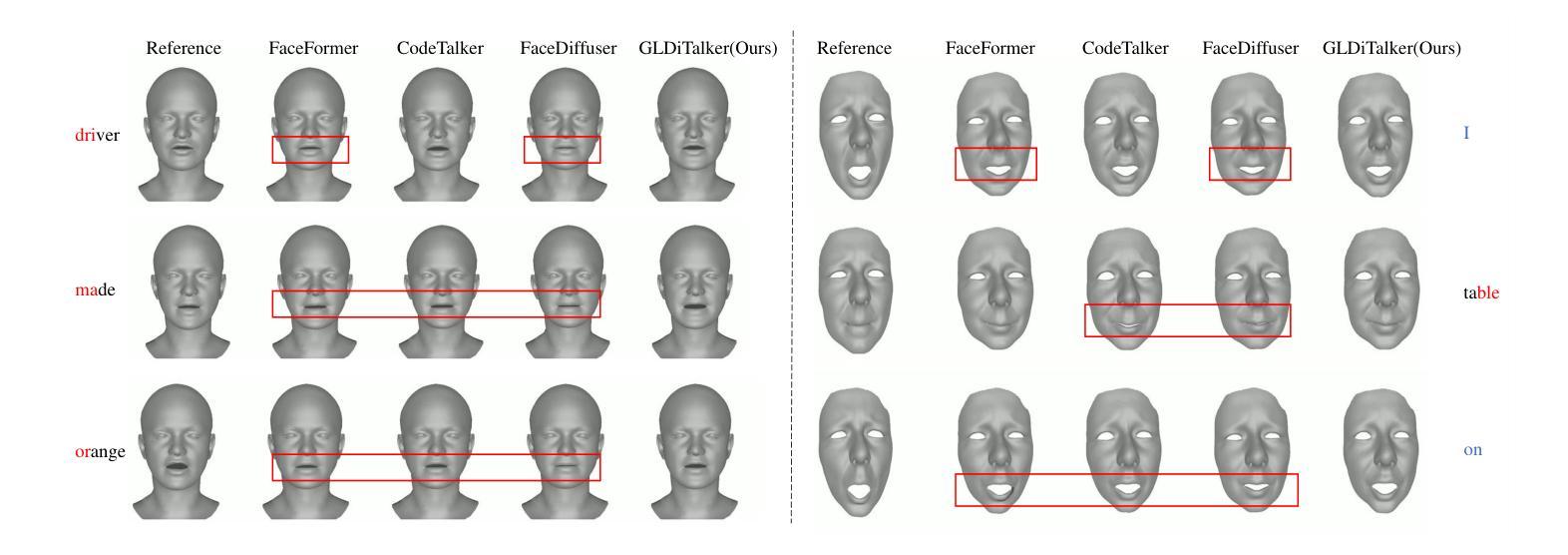
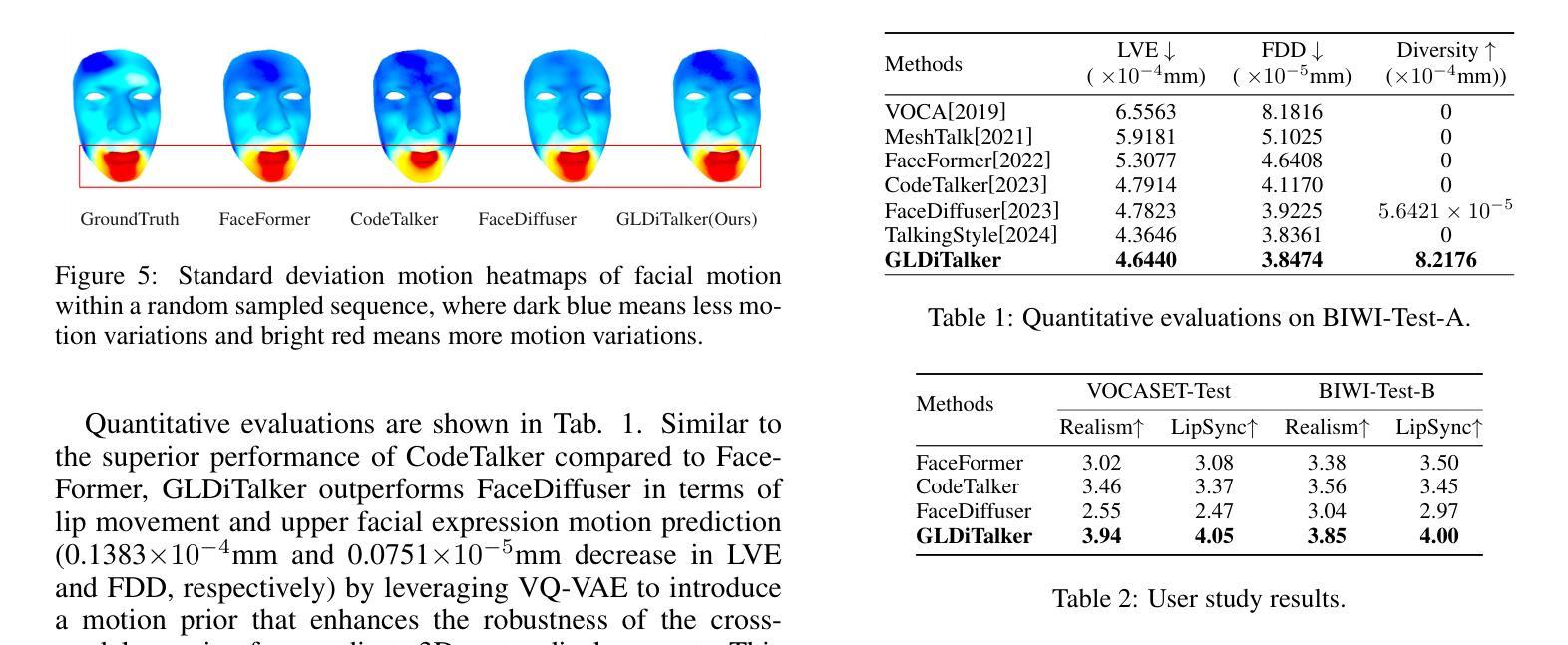