⚠️ 以下所有内容总结都来自于 大语言模型的能力,如有错误,仅供参考,谨慎使用
🔴 请注意:千万不要用于严肃的学术场景,只能用于论文阅读前的初筛!
💗 如果您觉得我们的项目对您有帮助 ChatPaperFree ,还请您给我们一些鼓励!⭐️ HuggingFace免费体验
2025-01-31 更新
Transformer-Based Auxiliary Loss for Face Recognition Across Age Variations
Authors:Pritesh Prakash, Ashish Jacob Sam, S Umamaheswaran
Aging presents a significant challenge in face recognition, as changes in skin texture and tone can alter facial features over time, making it particularly difficult to compare images of the same individual taken years apart, such as in long-term identification scenarios. Transformer networks have the strength to preserve sequential spatial relationships caused by aging effect. This paper presents a technique for loss evaluation that uses a transformer network as an additive loss in the face recognition domain. The standard metric loss function typically takes the final embedding of the main CNN backbone as its input. Here, we employ a transformer-metric loss, a combined approach that integrates both transformer-loss and metric-loss. This research intends to analyze the transformer behavior on the convolution output when the CNN outcome is arranged in a sequential vector. These sequential vectors have the potential to overcome the texture or regional structure referred to as wrinkles or sagging skin affected by aging. The transformer encoder takes input from the contextual vectors obtained from the final convolution layer of the network. The learned features can be more age-invariant, complementing the discriminative power of the standard metric loss embedding. With this technique, we use transformer loss with various base metric-loss functions to evaluate the effect of the combined loss functions. We observe that such a configuration allows the network to achieve SoTA results in LFW and age-variant datasets (CA-LFW and AgeDB). This research expands the role of transformers in the machine vision domain and opens new possibilities for exploring transformers as a loss function.
人脸识别中,衰老成为一大挑战。随着时间的推移,皮肤纹理和色调的变化会改变面部特征,这使得多年后才获得同一个体的图像比对变得尤其困难,特别是在长期识别场景中。Transformer网络具有保持因衰老效应引起的连续空间关系的能力。本文提出了一种损失评估技术,该技术使用transformer网络作为人脸识别领域中的附加损失。标准的度量损失函数通常将主CNN骨干的最终嵌入作为其输入。在这里,我们采用了transformer-metric损失,这是一种结合了transformer损失和度量损失的综合方法。本研究旨在分析CNN输出被排列成顺序向量时transformer在卷积输出上的行为。这些顺序向量具有克服由衰老引起的纹理或称为皱纹或松弛的皮肤等区域性结构的潜力。Transformer编码器从网络最终卷积层获得的上下文向量中获取输入。所学习的特征可能具有更强的抗老化性,补充了标准度量损失嵌入的辨别力。使用这种技术,我们将transformer损失与各种基本度量损失函数结合,以评估组合损失函数的效果。我们观察到,这种配置使网络能够在LFW和年龄差异数据集(CA-LFW和AgeDB)上实现最先进的性能。该研究扩展了transformer在机器视觉领域的应用,为探索将transformer作为损失函数提供了新的可能性。
论文及项目相关链接
PDF Face Recognition for Age-variant Datasets
Summary
该论文探讨了使用Transformer网络在人脸识别领域中的损失评估技术。针对因年龄增长导致的面部特征变化,该研究提出了一种结合Transformer损失和度量损失的混合方法。通过利用Transformer网络处理CNN输出的卷积序列向量,该方法能够减少老化对皱纹和皮肤松弛等面部纹理和结构的影响,进而提高年龄不变的人脸识别性能。
Key Takeaways
- 年龄增长对面部识别带来挑战,皮肤纹理和色调的变化会改变面部特征。
- Transformer网络有能力保持由老化引起的序列空间关系。
- 该研究提出了结合Transformer损失和度量损失的混合方法,以应对老化对面部识别的影响。
- Transformer网络处理CNN输出的卷积序列向量,有助于克服老化引起的皱纹和皮肤松弛等面部纹理问题。
- 这种方法使得网络在LFW和年龄变化的数据集(CA-LFW和AgeDB)上取得了最先进的识别结果。
- 此研究扩展了Transformer在机器视觉领域的应用,并开启了探索其作为损失函数的新可能性。
点此查看论文截图
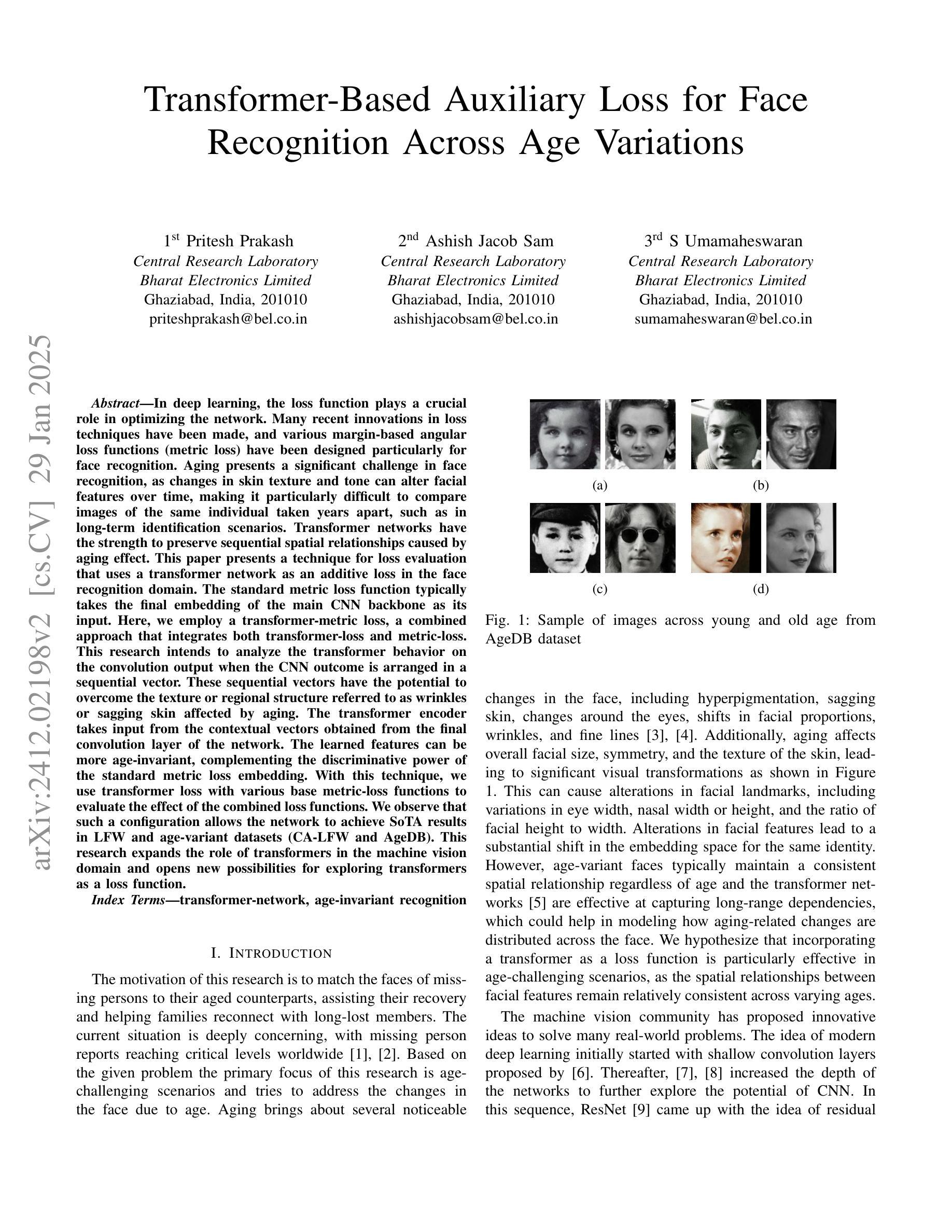
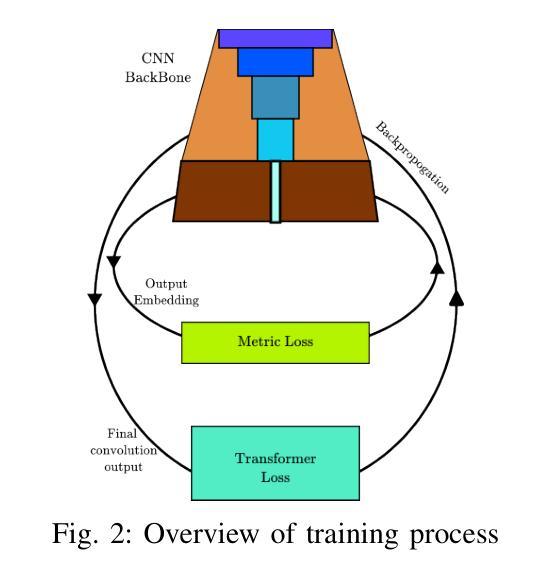
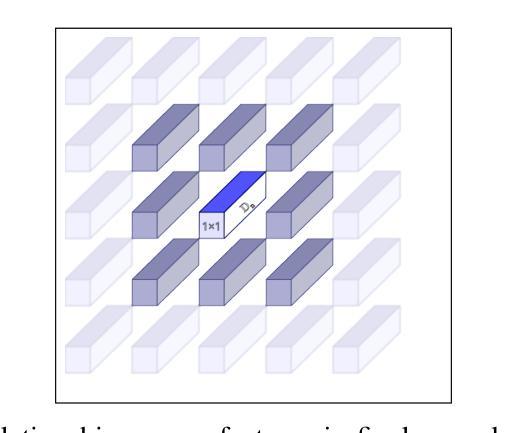
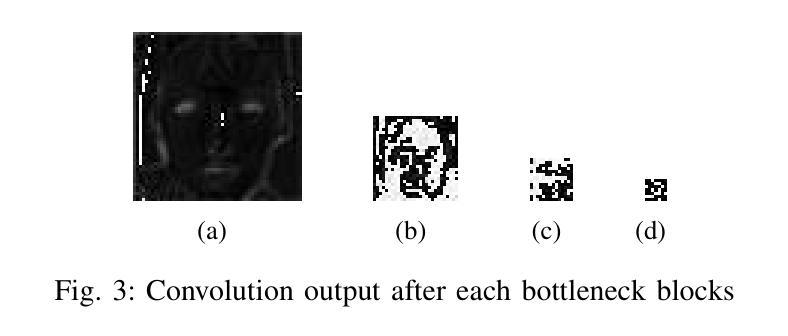
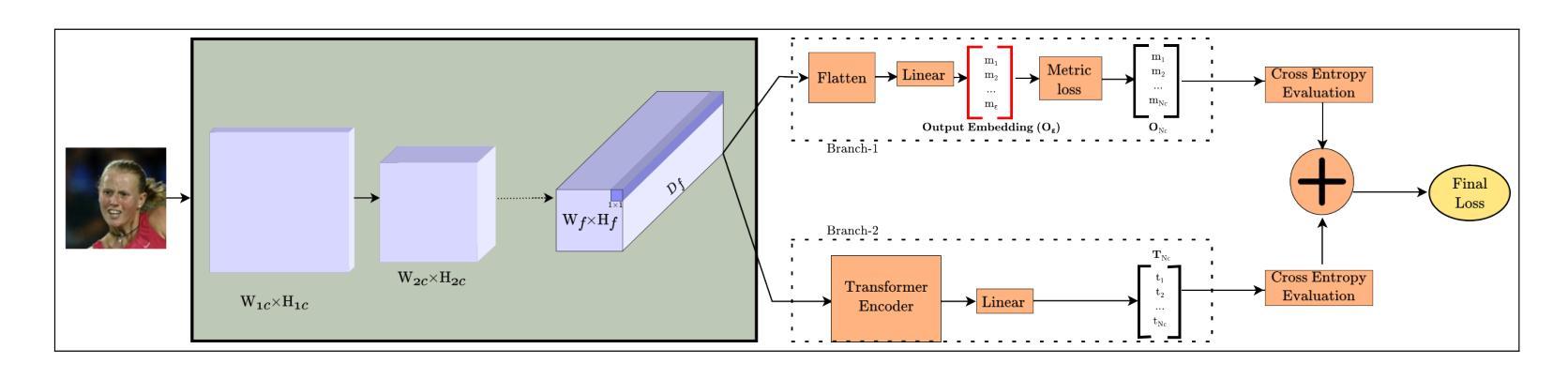
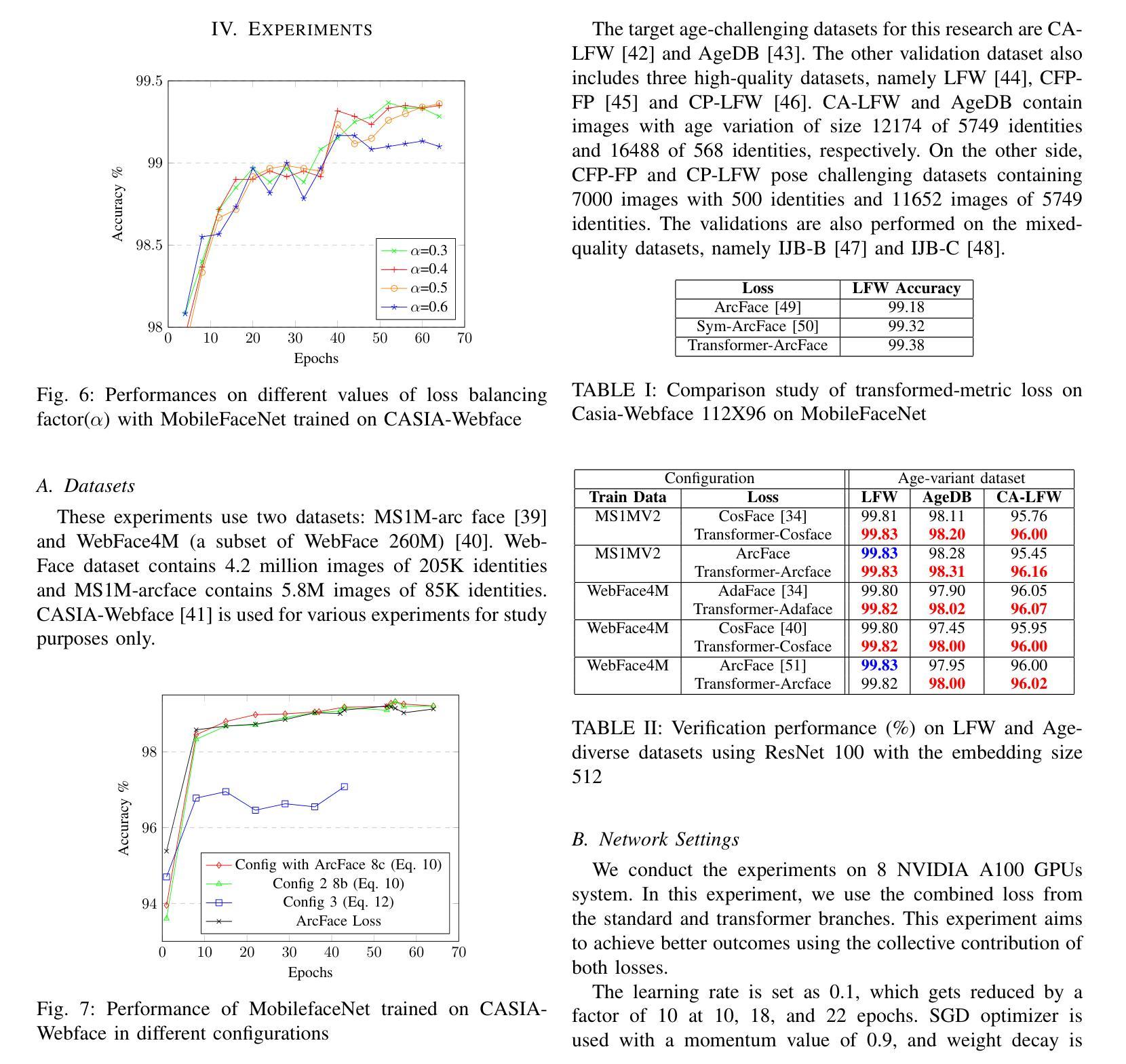
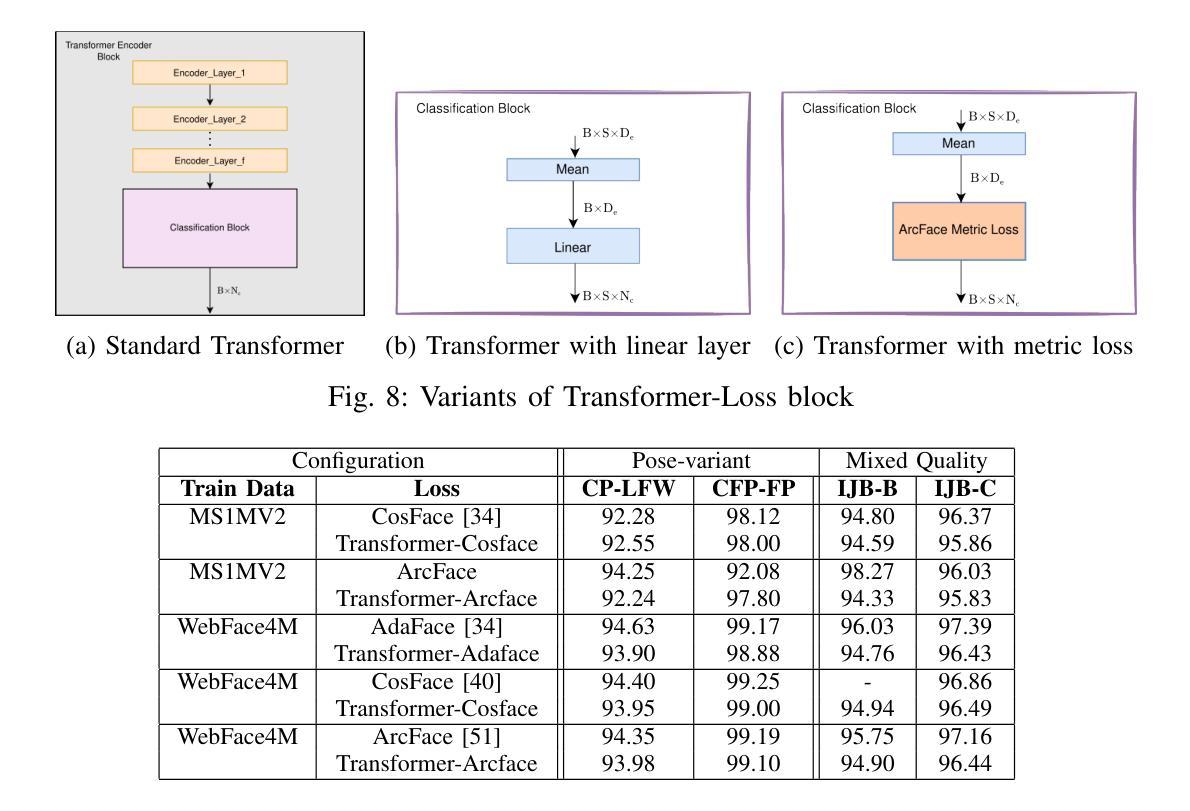