⚠️ 以下所有内容总结都来自于 大语言模型的能力,如有错误,仅供参考,谨慎使用
🔴 请注意:千万不要用于严肃的学术场景,只能用于论文阅读前的初筛!
💗 如果您觉得我们的项目对您有帮助 ChatPaperFree ,还请您给我们一些鼓励!⭐️ HuggingFace免费体验
2025-01-31 更新
VICCA: Visual Interpretation and Comprehension of Chest X-ray Anomalies in Generated Report Without Human Feedback
Authors:Sayeh Gholipour Picha, Dawood Al Chanti, Alice Caplier
As artificial intelligence (AI) becomes increasingly central to healthcare, the demand for explainable and trustworthy models is paramount. Current report generation systems for chest X-rays (CXR) often lack mechanisms for validating outputs without expert oversight, raising concerns about reliability and interpretability. To address these challenges, we propose a novel multimodal framework designed to enhance the semantic alignment and localization accuracy of AI-generated medical reports. Our framework integrates two key modules: a Phrase Grounding Model, which identifies and localizes pathologies in CXR images based on textual prompts, and a Text-to-Image Diffusion Module, which generates synthetic CXR images from prompts while preserving anatomical fidelity. By comparing features between the original and generated images, we introduce a dual-scoring system: one score quantifies localization accuracy, while the other evaluates semantic consistency. This approach significantly outperforms existing methods, achieving state-of-the-art results in pathology localization and text-to-image alignment. The integration of phrase grounding with diffusion models, coupled with the dual-scoring evaluation system, provides a robust mechanism for validating report quality, paving the way for more trustworthy and transparent AI in medical imaging.
随着人工智能(AI)在医疗保健中越来越占据核心地位,对可解释和可靠模型的需求变得至关重要。当前用于胸部X射线(CXR)的报告生成系统由于缺乏在无需专家监督的情况下验证输出的机制,人们对它们的可靠性和可解释性表示担忧。为了应对这些挑战,我们提出了一种新型的多模式框架,旨在提高AI生成的医疗报告的语义对齐和定位准确性。我们的框架集成了两个关键模块:短语定位模型,该模型根据文本提示识别和定位CXR图像中的病理;文本到图像扩散模块,该模块从提示生成合成CXR图像,同时保留解剖学的真实性。通过比较原始图像和生成图像之间的特征,我们引入了一种双重评分系统:一个评分量化定位准确性,另一个评分评估语义一致性。该方法显著优于现有方法,在病理定位和文本到图像对齐方面达到了最新水平的结果。短语定位与扩散模型的集成,加上双重评分评估系统,为验证报告质量提供了稳健的机制,为医疗成像中更可靠、更透明的AI铺平了道路。
论文及项目相关链接
Summary
随着人工智能在医疗领域的广泛应用,对可解释性和可靠性的模型需求愈发重要。当前,针对胸部X光射线(CXR)的报告生成系统缺乏验证输出的机制,亟需改进。为此,我们提出一种新型的多模式框架,旨在提高AI生成的医疗报告的语义对齐和定位准确性。该框架包含两个核心模块:短语定位模型和文本到图像扩散模块。短语定位模型根据文本提示识别并定位CXR图像中的病理,而文本到图像扩散模块则从提示生成合成CXR图像,保持解剖结构的准确性。通过比较原始和生成图像的特征,我们引入双重评分系统:一个评分量化定位准确性,另一个评分评估语义一致性。该方法显著优于现有方法,在病理定位和文本到图像对齐方面达到最新结果。短语定位与扩散模型的结合,加上双重评分评估系统,为报告质量提供了稳健的验证机制,为医疗成像领域更可靠、透明的AI应用铺平了道路。
Key Takeaways
- 人工智能在医疗领域的需求:需要可解释性和可靠的模型。
- 现有CXR报告生成系统的挑战:缺乏验证输出的机制,存在可靠性和可解释性问题。
- 新型多模式框架的提出:旨在提高AI生成的医疗报告的语义对齐和定位准确性。
- 框架包含两个核心模块:短语定位模型和文本到图像扩散模块。
- 双重评分系统:一个评分量化定位准确性,另一个评分评估语义一致性。
- 方法显著优于现有方法,达到最新结果。
点此查看论文截图
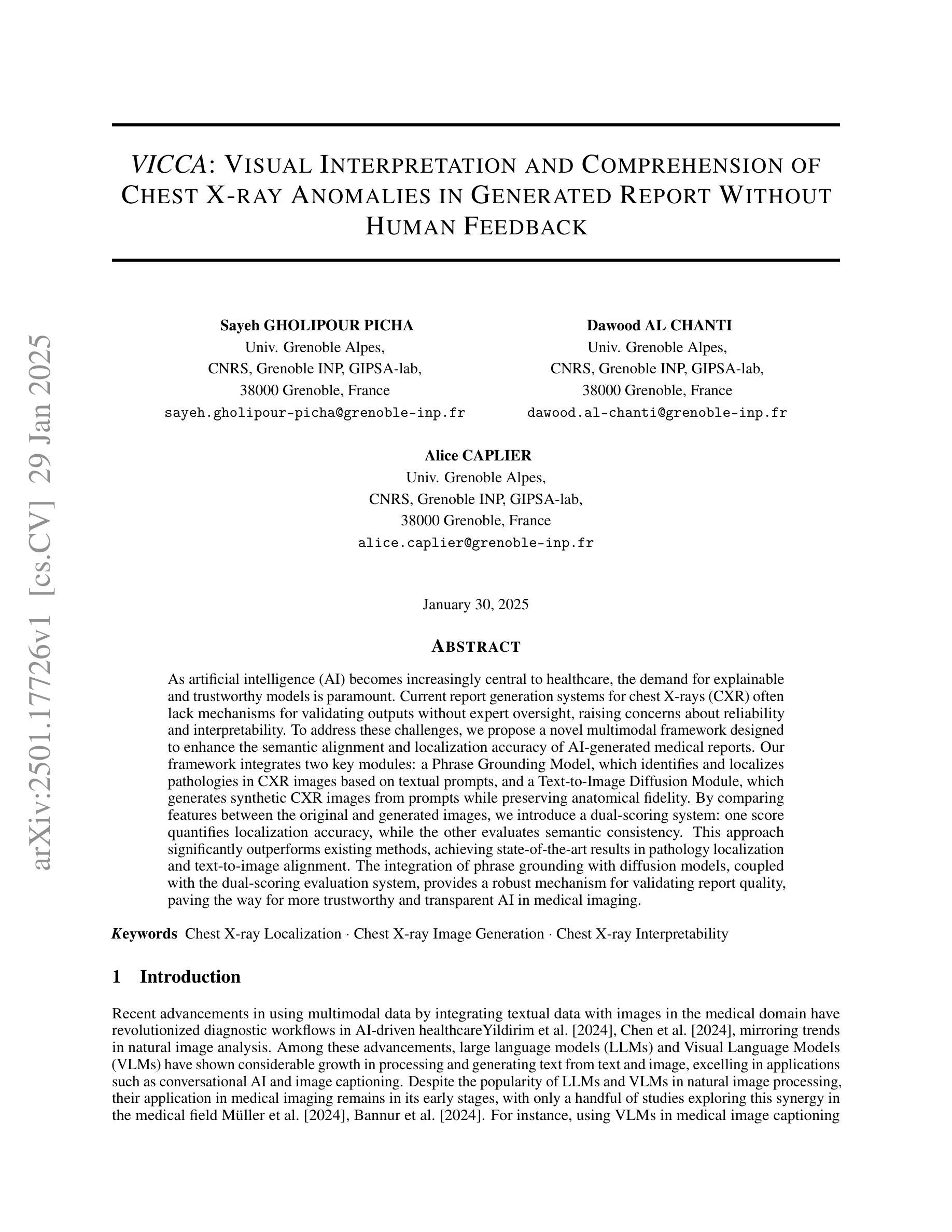
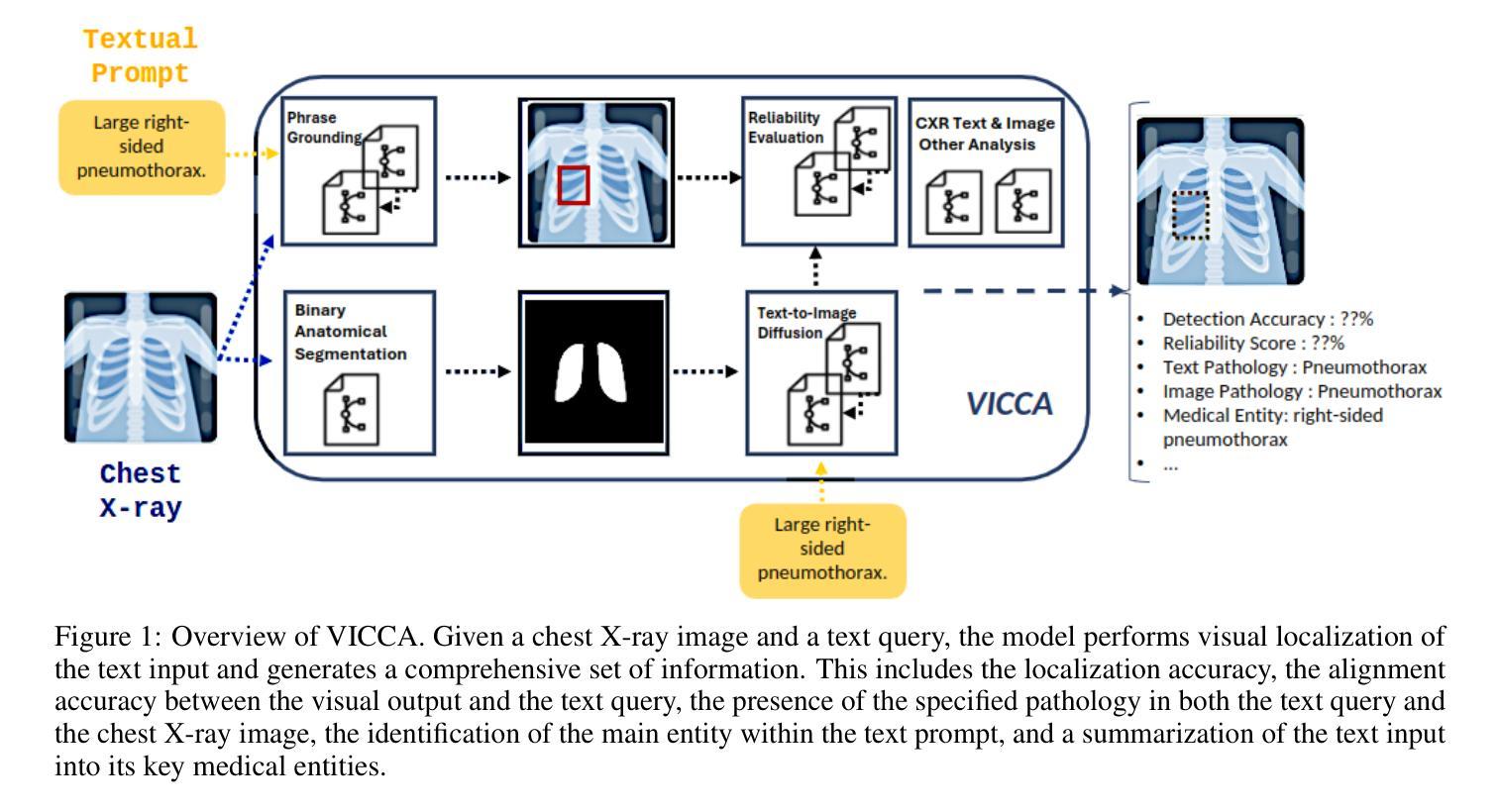
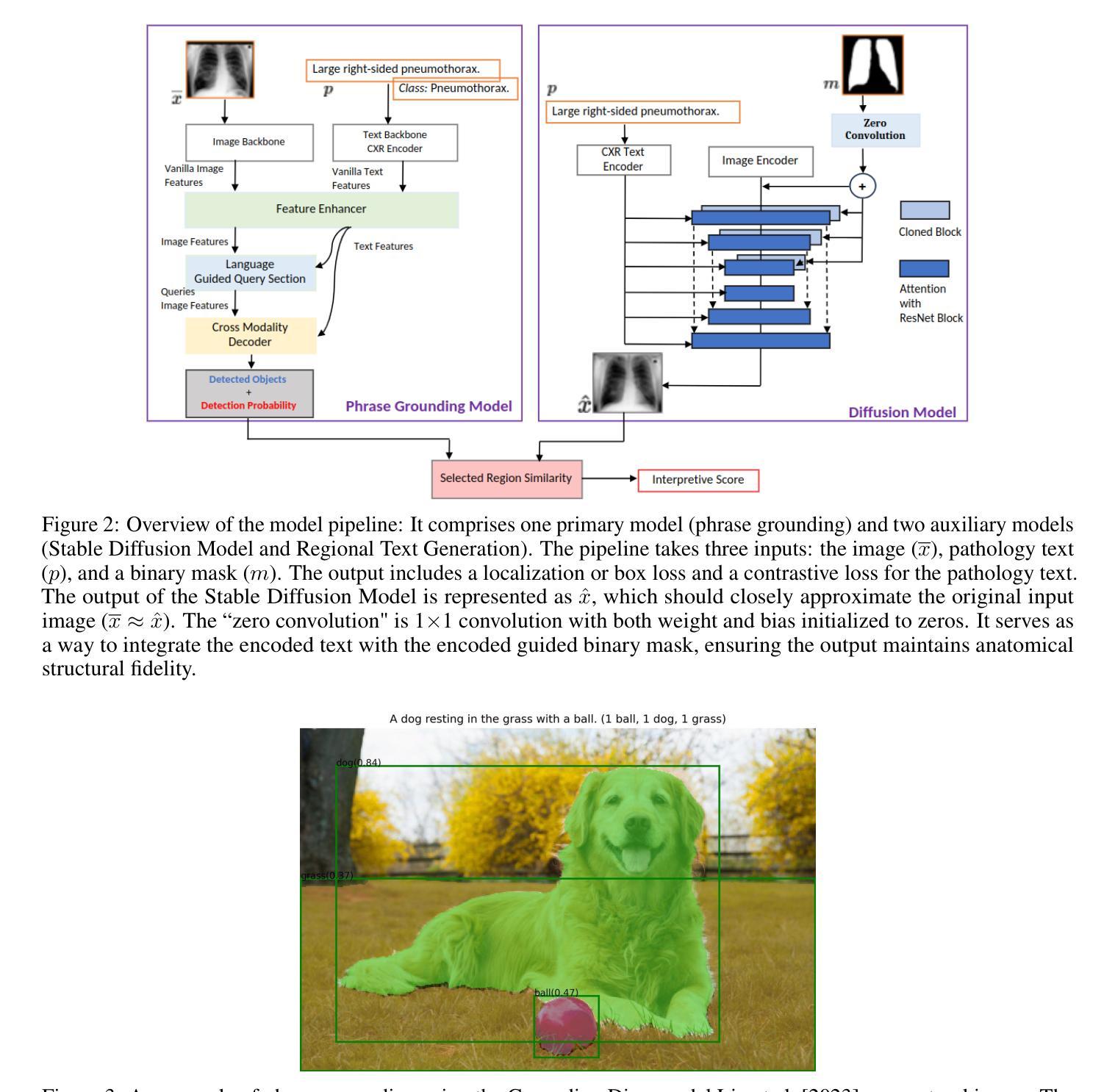
Zero-Shot Medical Phrase Grounding with Off-the-shelf Diffusion Models
Authors:Konstantinos Vilouras, Pedro Sanchez, Alison Q. O’Neil, Sotirios A. Tsaftaris
Localizing the exact pathological regions in a given medical scan is an important imaging problem that traditionally requires a large amount of bounding box ground truth annotations to be accurately solved. However, there exist alternative, potentially weaker, forms of supervision, such as accompanying free-text reports, which are readily available.The task of performing localization with textual guidance is commonly referred to as phrase grounding. In this work, we use a publicly available Foundation Model, namely the Latent Diffusion Model, to perform this challenging task. This choice is supported by the fact that the Latent Diffusion Model, despite being generative in nature, contains cross-attention mechanisms that implicitly align visual and textual features, thus leading to intermediate representations that are suitable for the task at hand. In addition, we aim to perform this task in a zero-shot manner, i.e., without any training on the target task, meaning that the model’s weights remain frozen. To this end, we devise strategies to select features and also refine them via post-processing without extra learnable parameters. We compare our proposed method with state-of-the-art approaches which explicitly enforce image-text alignment in a joint embedding space via contrastive learning. Results on a popular chest X-ray benchmark indicate that our method is competitive with SOTA on different types of pathology, and even outperforms them on average in terms of two metrics (mean IoU and AUC-ROC). Source code will be released upon acceptance at \url{https://github.com/vios-s}.
定位给定医学扫描中的精确病理区域是一个重要的成像问题,传统上需要大量的边界框真实标注来准确解决。然而,存在替代的、可能较弱的监督形式,例如伴随的自由文本报告,这些报告很容易获得。执行带有文本指导的定位任务通常被称为短语定位。在这项工作中,我们使用一个公开可用的基础模型,即潜在扩散模型,来完成这项具有挑战性的任务。我们选择这个模型是因为尽管潜在扩散模型本质上是生成式的,但它包含交叉注意机制,可以隐式地对齐视觉和文本特征,从而产生适合当前任务的中间表示。此外,我们旨在以零样本方式进行此任务,即无需对目标任务进行任何训练,这意味着模型的权重保持冻结。为此,我们制定了选择特征的策略,并通过后处理对特征进行提炼,无需额外的可学习参数。我们将所提出的方法与最先进的方法进行了比较,最先进的方法通过对比学习在联合嵌入空间中显式强制执行图像文本对齐。在流行的胸部X射线基准测试上的结果表明,我们的方法在不同类型的病理上与最先进的方法具有竞争力,甚至在两个指标(平均IoU和AUC-ROC)上的平均表现超过了它们。源代码将在接受后发布于https://github.com/vios-s。
论文及项目相关链接
PDF 10 pages, 3 figures, IEEE J-BHI Special Issue on Foundation Models in Medical Imaging
Summary
基于文本指导进行定位任务的“词基定位”(Phrase Grounding)方法逐渐受到关注。该研究使用预训练好的开源模型Latent Diffusion Model(LDM)应对这一挑战任务,该方法生成的特性使其成为良好的候选者,因为该模型具有跨注意力机制,可隐式对齐视觉和文本特征。该研究还尝试以零样本方式进行任务操作,无需针对目标任务进行训练,并保持模型权重冻结状态。他们选择策略选取和提炼特征并通过后处理强化这些方法效果。对广泛使用的胸片等效评分表的实验结果比较表明,此方法不仅在各类疾病上的表现具有竞争力,而且在平均意义上超过当前顶尖技术的两项指标(平均IoU和AUC-ROC)。该研究的源代码将在接受后发布于GitHub上。
Key Takeaways
- 研究关注于使用Latent Diffusion Model(LDM)解决基于文本指导的定位问题。
- LDM具有跨注意力机制,能隐式对齐视觉和文本特征,使其成为解决此任务的有力工具。
- 研究尝试零样本方式执行任务,无需针对目标任务进行训练,并保持模型权重冻结状态。
- 研究采用策略选取和提炼特征并通过后处理提升效果。
- 实验结果显示该方法在胸片上的表现具有竞争力,超过当前顶尖技术的两项指标。
- 该研究的源代码将在接受后公开于GitHub上。
点此查看论文截图
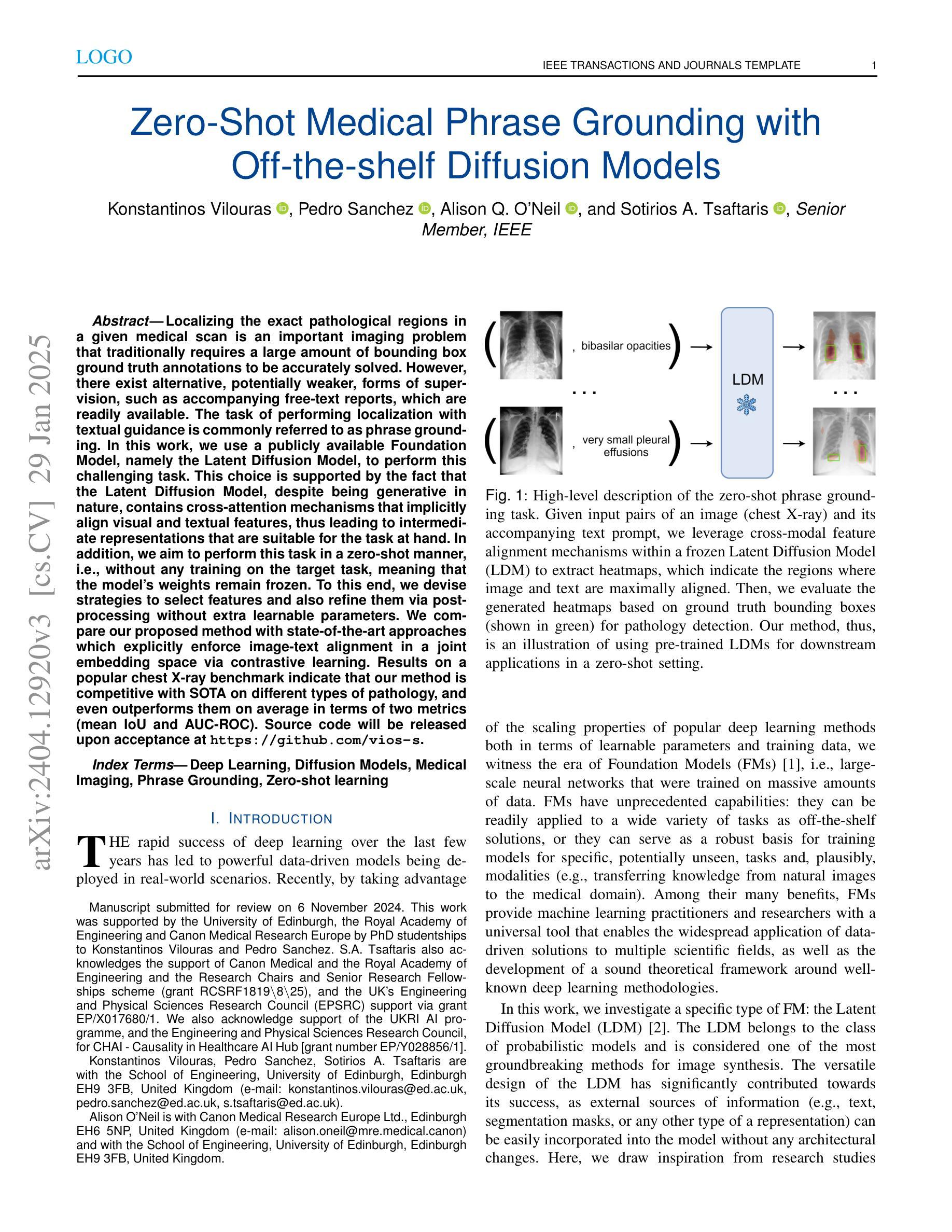
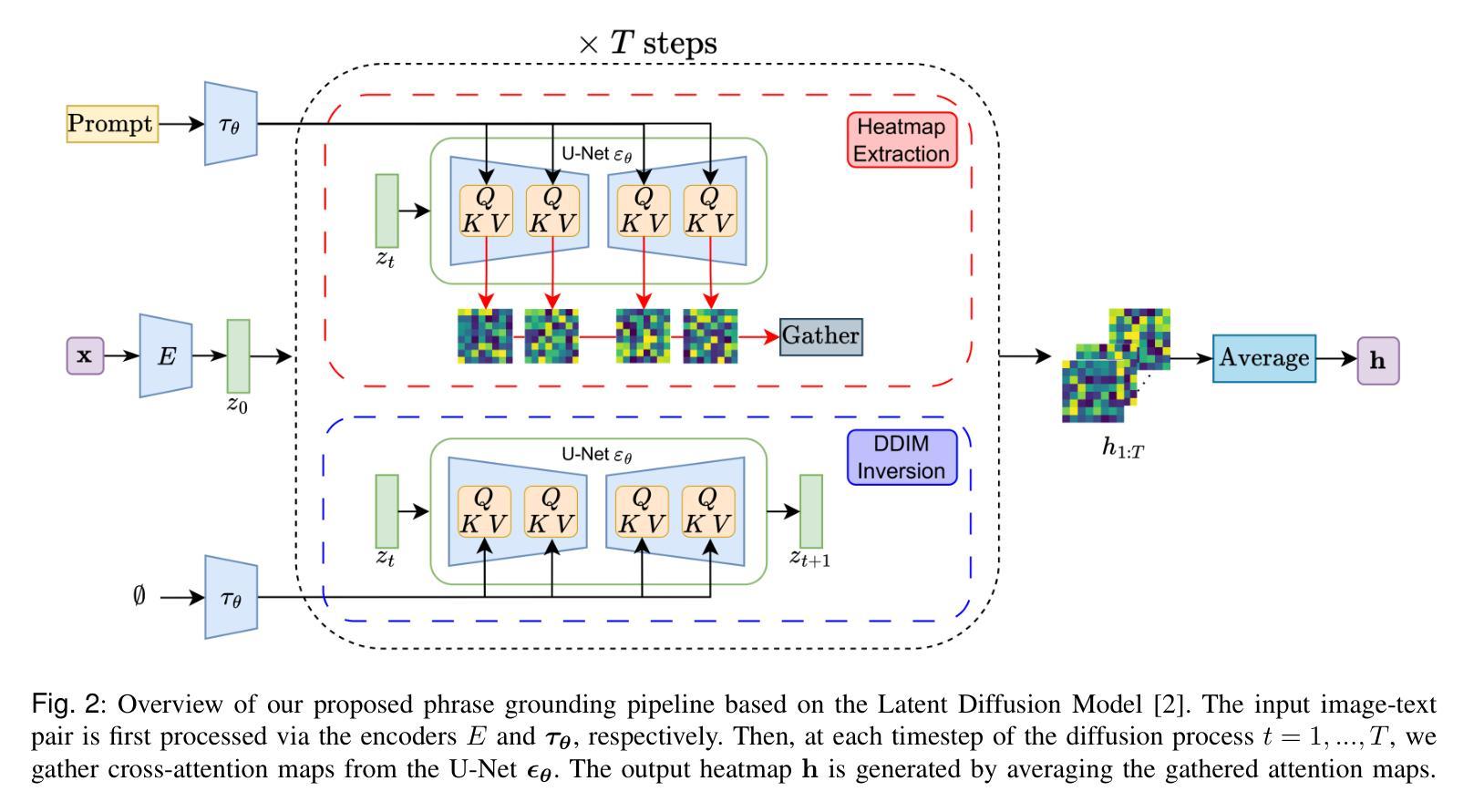
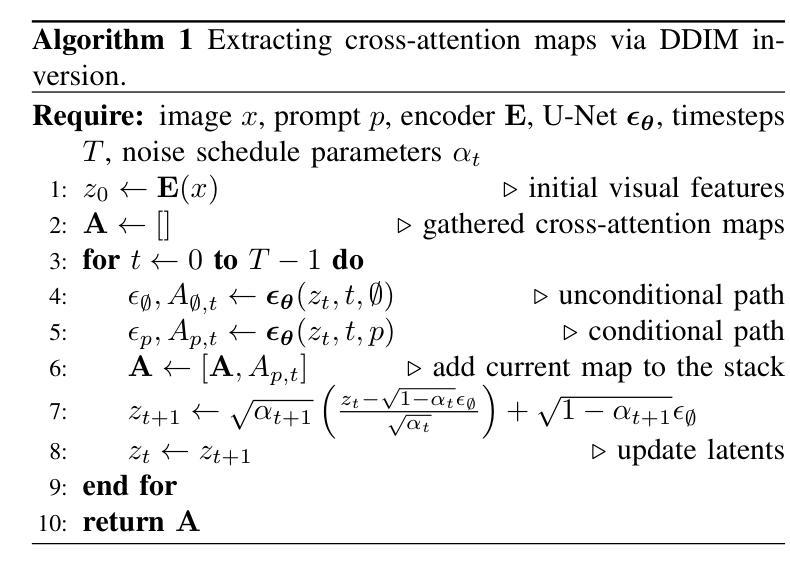