⚠️ 以下所有内容总结都来自于 大语言模型的能力,如有错误,仅供参考,谨慎使用
🔴 请注意:千万不要用于严肃的学术场景,只能用于论文阅读前的初筛!
💗 如果您觉得我们的项目对您有帮助 ChatPaperFree ,还请您给我们一些鼓励!⭐️ HuggingFace免费体验
2025-01-31 更新
Better Slow than Sorry: Introducing Positive Friction for Reliable Dialogue Systems
Authors:Mert İnan, Anthony Sicilia, Suvodip Dey, Vardhan Dongre, Tejas Srinivasan, Jesse Thomason, Gökhan Tür, Dilek Hakkani-Tür, Malihe Alikhani
While theories of discourse and cognitive science have long recognized the value of unhurried pacing, recent dialogue research tends to minimize friction in conversational systems. Yet, frictionless dialogue risks fostering uncritical reliance on AI outputs, which can obscure implicit assumptions and lead to unintended consequences. To meet this challenge, we propose integrating positive friction into conversational AI, which promotes user reflection on goals, critical thinking on system response, and subsequent re-conditioning of AI systems. We hypothesize systems can improve goal alignment, modeling of user mental states, and task success by deliberately slowing down conversations in strategic moments to ask questions, reveal assumptions, or pause. We present an ontology of positive friction and collect expert human annotations on multi-domain and embodied goal-oriented corpora. Experiments on these corpora, along with simulated interactions using state-of-the-art systems, suggest incorporating friction not only fosters accountable decision-making, but also enhances machine understanding of user beliefs and goals, and increases task success rates.
长期以来,话语理论和认知科学已经认识到从容的节奏的价值,而最近的对话研究倾向于减少会话系统中的摩擦。然而,无摩擦的对话可能会导致对AI输出的无批判性依赖,这可能会掩盖隐含的假设并导致意想不到的后果。为了应对这一挑战,我们提议将在对话AI中融入积极的摩擦,这可以促进用户对目标的反思、对系统响应的批判性思考以及对AI系统的后续调整。我们假设通过故意在关键时刻放慢对话速度来提出问题、揭示假设或暂停,可以促使系统改善目标对齐、用户心理状态的建模和任务成功率。我们展示了积极的摩擦的本体论,并在多领域和具体目标导向的语料库上收集了专家的人类注释。这些语料库的实验以及与最新系统的模拟交互表明,融入摩擦不仅促进了负责任的决策制定,还增强了机器对用户信念和目标的了解,提高了任务成功率。
论文及项目相关链接
Summary
本文主张在对话AI中融入适度摩擦,以促进用户对目标的反思和对系统回应的批判性思维,从而避免过度依赖AI输出导致的隐含假设模糊和意外后果。通过战略性地放慢对话速度,提出假设、揭示假设或暂停,可以改善目标对齐、用户心理状态建模和任务成功率。
Key Takeaways
- 摩擦在对话AI中具有重要作用,适度的摩擦可以促使用户反思目标和系统响应的批判性思维。
- 完全的摩擦无碍对话可能导致用户对AI输出的过度依赖,导致隐含假设模糊和意外后果。
- 通过在关键时候放慢对话速度,可以增加用户对任务目标的清晰度,改善目标对齐。
- 积极摩擦有助于建模用户的心理状态,提高任务成功率。
- 积极摩擦不仅促进负责任的决策制定,还增强了机器对用户信念和目标的了解。
- 专家注解和实验证明积极摩擦在对话AI中的有效性。
点此查看论文截图
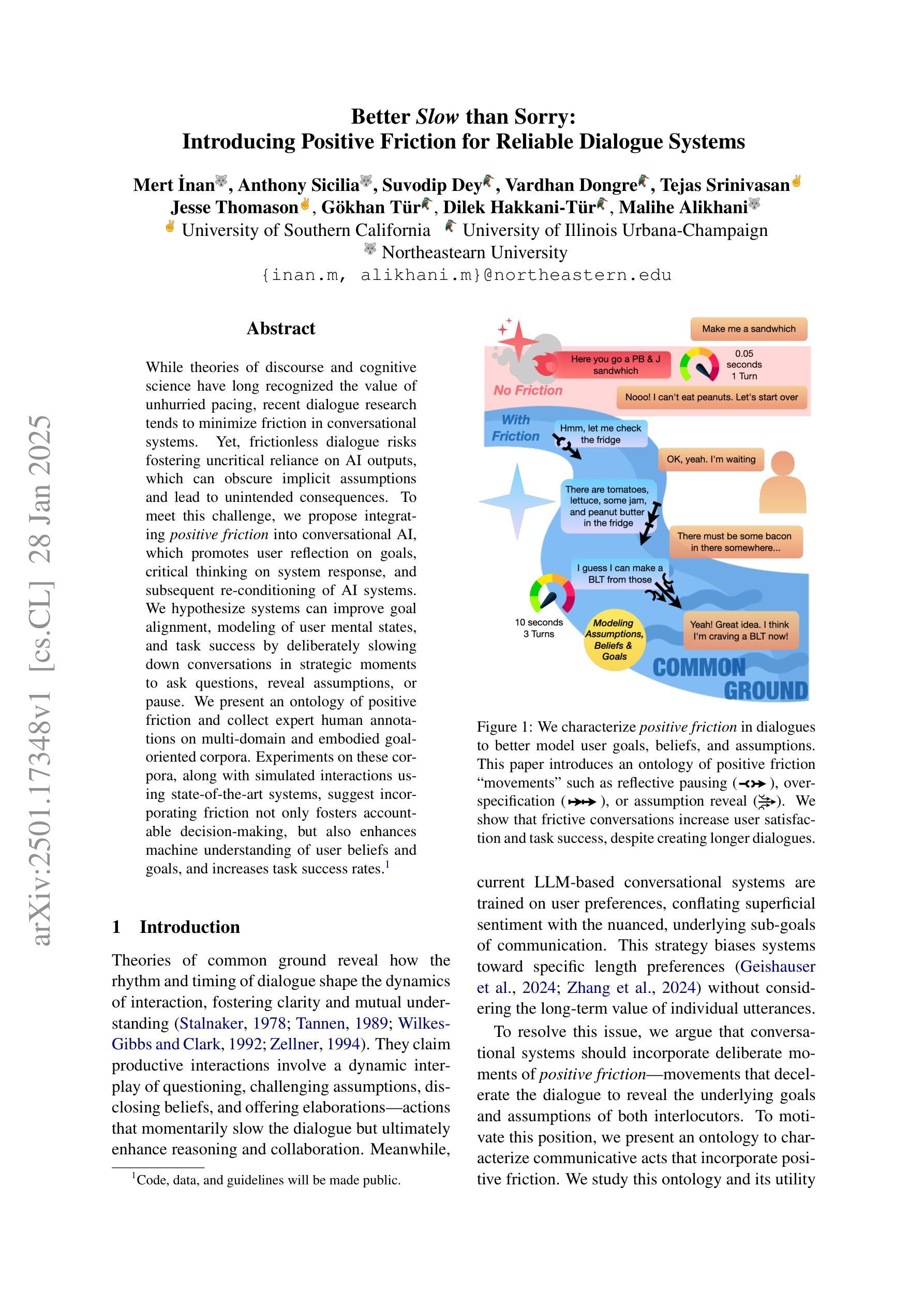
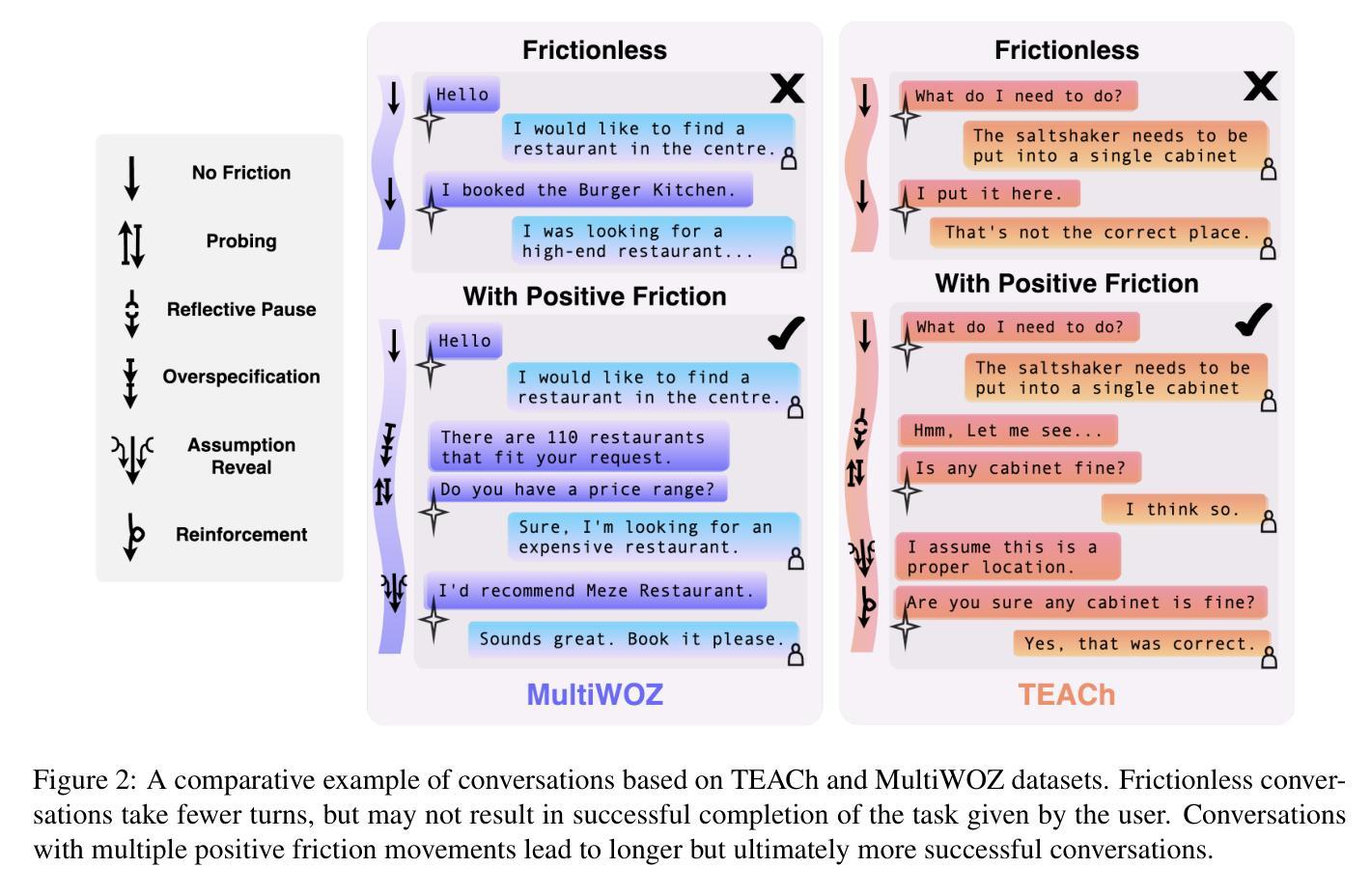
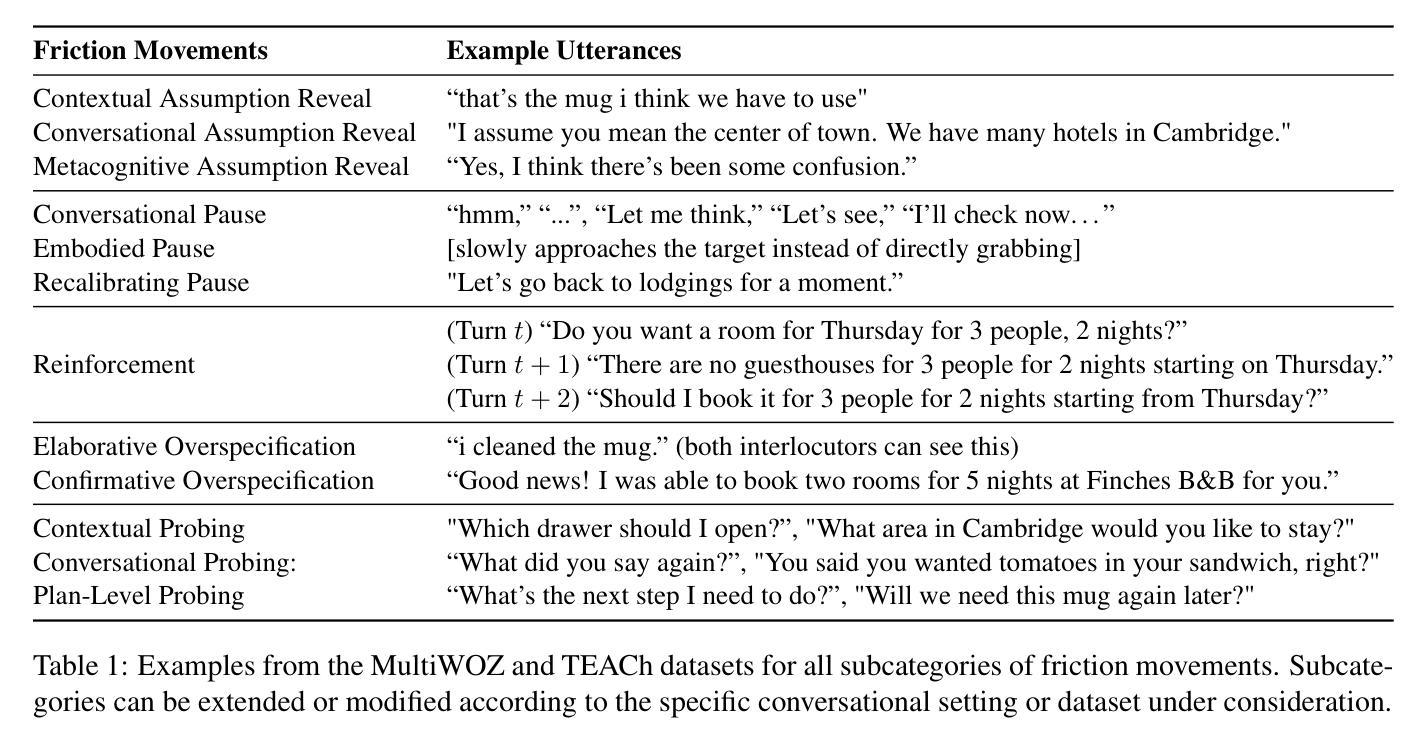
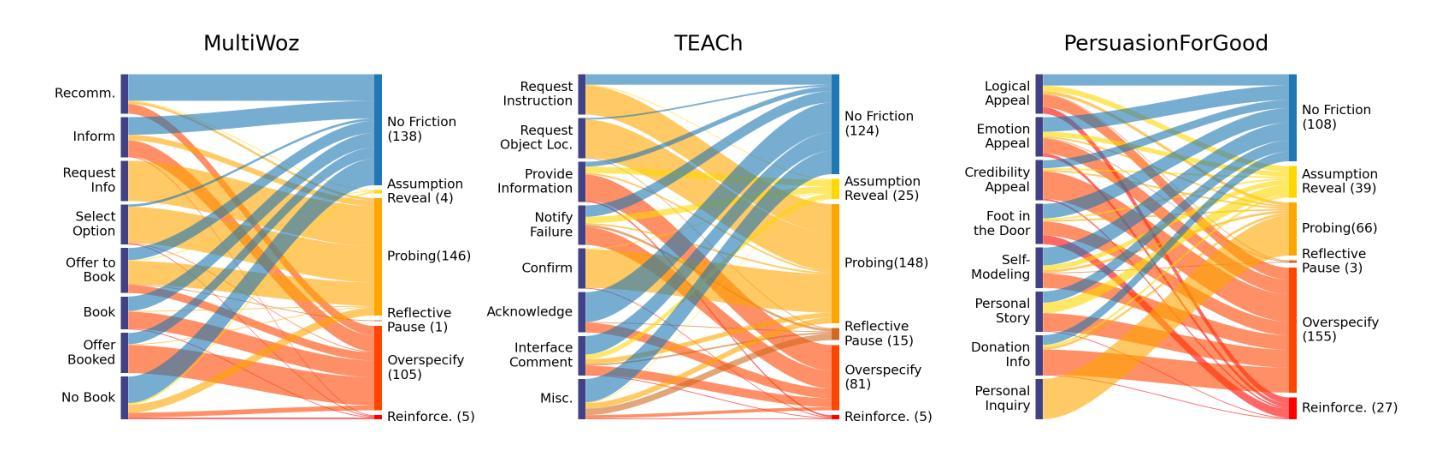
EmoDynamiX: Emotional Support Dialogue Strategy Prediction by Modelling MiXed Emotions and Discourse Dynamics
Authors:Chenwei Wan, Matthieu Labeau, Chloé Clavel
Designing emotionally intelligent conversational systems to provide comfort and advice to people experiencing distress is a compelling area of research. Recently, with advancements in large language models (LLMs), end-to-end dialogue agents without explicit strategy prediction steps have become prevalent. However, implicit strategy planning lacks transparency, and recent studies show that LLMs’ inherent preference bias towards certain socio-emotional strategies hinders the delivery of high-quality emotional support. To address this challenge, we propose decoupling strategy prediction from language generation, and introduce a novel dialogue strategy prediction framework, EmoDynamiX, which models the discourse dynamics between user fine-grained emotions and system strategies using a heterogeneous graph for better performance and transparency. Experimental results on two ESC datasets show EmoDynamiX outperforms previous state-of-the-art methods with a significant margin (better proficiency and lower preference bias). Our approach also exhibits better transparency by allowing backtracing of decision making.
设计能够给经历痛苦的人提供安慰和建议的情绪智能对话系统是一个引人注目的研究领域。最近,随着大型语言模型(LLM)的进步,不需要明确策略预测步骤的端到端对话代理已经普遍存在。然而,隐式策略规划缺乏透明度,最近的研究表明,LLM对某些社会情感策略的固有偏好偏向阻碍了高质量情感支持的实现。为了解决这一挑战,我们提出了将策略预测与语言生成相分离,并引入了一种新型的对话策略预测框架EmoDynamiX。该框架使用异构图对用户精细情绪与系统策略之间的对话动态进行建模,以实现更好的性能和透明度。在两个ESC数据集上的实验结果表明,EmoDynamiX显著优于以前的最先进方法(具有更好的熟练程度和更低的偏好偏见)。我们的方法还通过允许回溯决策制定来提供更好的透明度。
论文及项目相关链接
PDF Accepted to NAACL 2025 main, camera ready version
Summary
设计具有情感智能的对话系统,为经历困扰的人提供安慰和建议是一个引人入胜的研究领域。随着大型语言模型(LLMs)的进步,端到端的对话代理(没有明确的策略预测步骤)越来越普遍。然而,这种隐式策略规划缺乏透明度,且最近的研究表明LLMs对某些社会情感策略的固有偏好偏向阻碍了高质量情感支持的实现。为解决这一挑战,我们提出了策略预测与语言生成解耦的方法,并引入了一种新颖的对话策略预测框架——EmoDynamiX。它通过异质图建模用户精细情绪与系统策略之间的对话动态,以实现更好的性能和透明度。在两份情感支持对话数据集的测试结果显示,EmoDynamiX显著优于先前的一流方法(更高的专业能力和更低的偏好偏向)。此外,我们的方法还通过允许回溯决策制定过程提高了透明度。
Key Takeaways
- 设计情感智能对话系统为经历困扰的人提供情感支持是一个重要研究领域。
- 大型语言模型的进步推动了端到端对话代理的发展,但隐式策略规划缺乏透明度。
- LLMs对特定社会情感策略的固有偏好可能影响提供高质量的情感支持。
- EmoDynamiX是一个新颖的对话策略预测框架,旨在解决上述问题。
- EmoDynamiX通过异质图建模用户精细情绪与系统策略之间的对话动态。
- EmoDynamiX在情感支持对话数据集上的测试结果显著优于先前方法。
点此查看论文截图
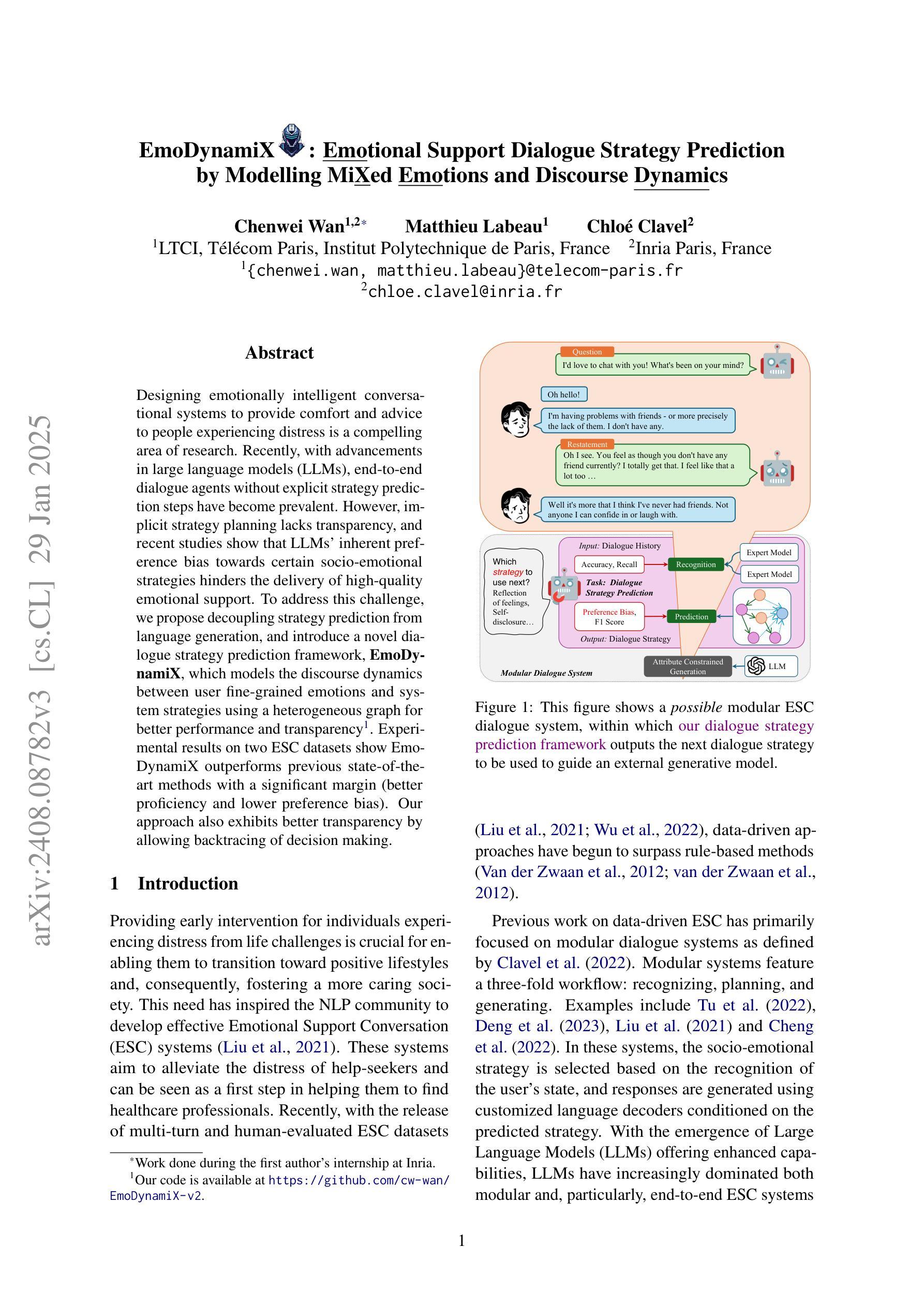
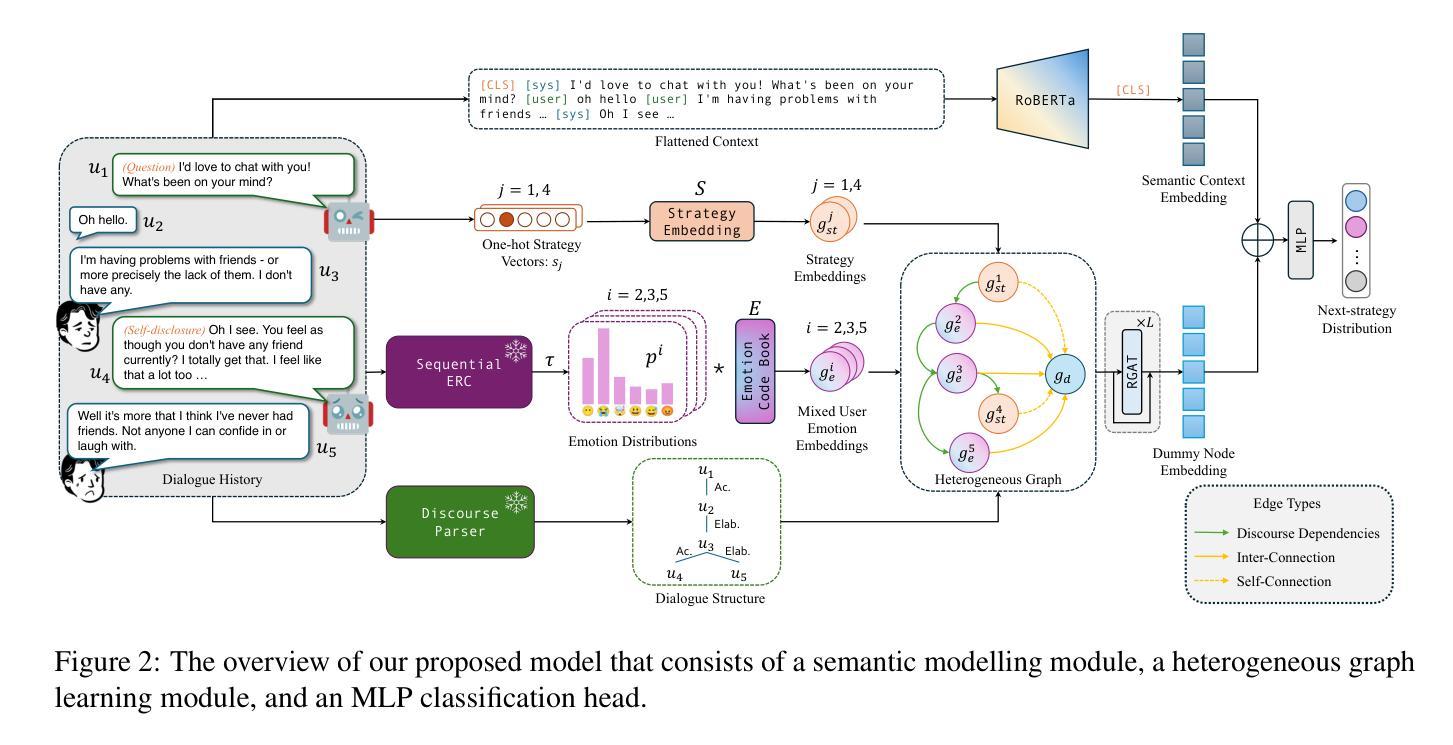
Towards Lifelong Dialogue Agents via Timeline-based Memory Management
Authors:Kai Tzu-iunn Ong, Namyoung Kim, Minju Gwak, Hyungjoo Chae, Taeyoon Kwon, Yohan Jo, Seung-won Hwang, Dongha Lee, Jinyoung Yeo
To achieve lifelong human-agent interaction, dialogue agents need to constantly memorize perceived information and properly retrieve it for response generation (RG). While prior studies focus on getting rid of outdated memories to improve retrieval quality, we argue that such memories provide rich, important contextual cues for RG (e.g., changes in user behaviors) in long-term conversations. We present THEANINE, a framework for LLM-based lifelong dialogue agents. THEANINE discards memory removal and manages large-scale memories by linking them based on their temporal and cause-effect relation. Enabled by this linking structure, THEANINE augments RG with memory timelines - series of memories representing the evolution or causality of relevant past events. Along with THEANINE, we introduce TeaFarm, a counterfactual-driven evaluation scheme, addressing the limitation of G-Eval and human efforts when assessing agent performance in integrating past memories into RG. A supplementary video for THEANINE and data for TeaFarm are at https://huggingface.co/spaces/ResearcherScholar/Theanine.
为实现终身的人机交互,对话智能体需要不断记忆感知到的信息,并适当检索以生成响应(RG)。虽然先前的研究专注于消除过时记忆以提高检索质量,但我们认为这些记忆为长期对话中的RG提供了丰富且重要的上下文线索(例如用户行为的改变)。我们提出了THEANINE,这是一个基于大型语言模型的终身对话智能体框架。THEANINE摒弃了记忆删除,并通过基于其时间和因果关系的链接来管理大规模记忆。借助这种链接结构,THEANINE通过记忆时间线增强RG,记忆时间线是一系列代表相关过去事件演变或因果关系的记忆。与THEANINE一起,我们引入了TeaFarm,这是一种以反事实驱动的评价方案,解决了在评估智能体将过去记忆融入RG的性能时G-Eval和人类努力的局限性。THEANINE的补充视频和TeaFarm的数据可在https://huggingface.co/spaces/ResearcherScholar/Theanine找到。
论文及项目相关链接
PDF Accepted to NAACL 2025
Summary
对话智能体实现终身人机交互的关键在于不断记忆感知信息并正确检索以用于响应生成。现有研究主要关注消除过时记忆以提高检索质量,但我们认为这些记忆为长期对话中的响应生成提供了丰富的上下文线索。为此,我们提出了基于大模型的终身对话智能体框架THEANINE,它不再删除记忆,而是通过时间和因果关系链接大规模记忆。THEANINE通过引入记忆时间线来增强响应生成能力,展示相关过去事件的演变或因果关系。此外,我们还引入了TeaFarm评估方案,以解决现有评估方法在面对集成过去记忆到响应生成时的局限性问题。相关补充视频和数据可在链接中找到。
Key Takeaways
- 对话智能体实现终身人机交互需要持续记忆并正确检索感知信息。
- 过时记忆对于长期对话的响应生成具有重要价值,提供丰富的上下文线索。
- THEANINE框架通过时间和因果关系链接大规模记忆,而不是删除旧记忆。
- THEANINE通过引入记忆时间线增强了响应生成能力,展示过去事件的演变和因果关系。
- 现有评估方法在面对集成过去记忆到响应生成时存在局限性。
- TeaFarm评估方案解决了这一问题,并提供了更加全面和有效的评估方式。
点此查看论文截图
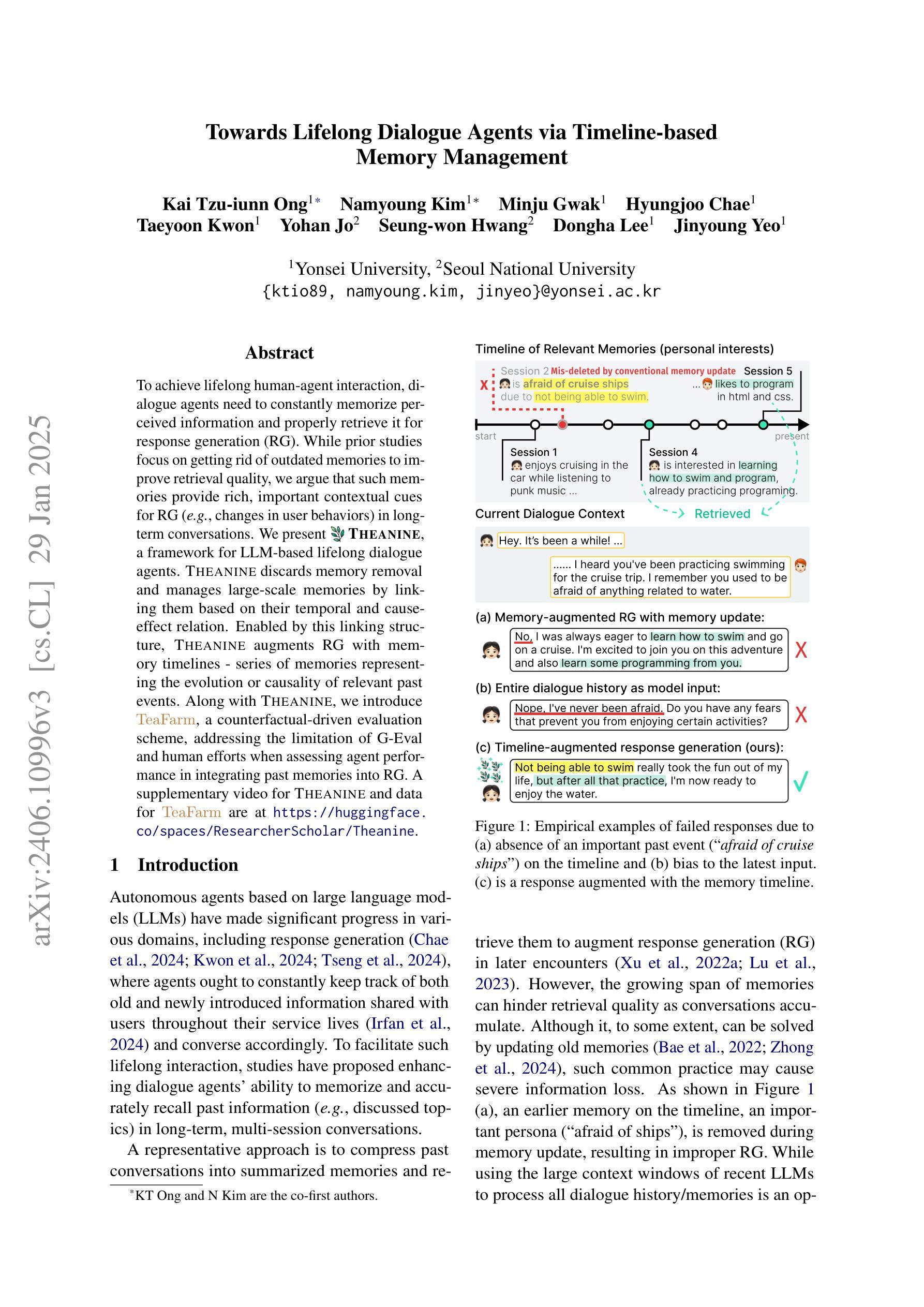
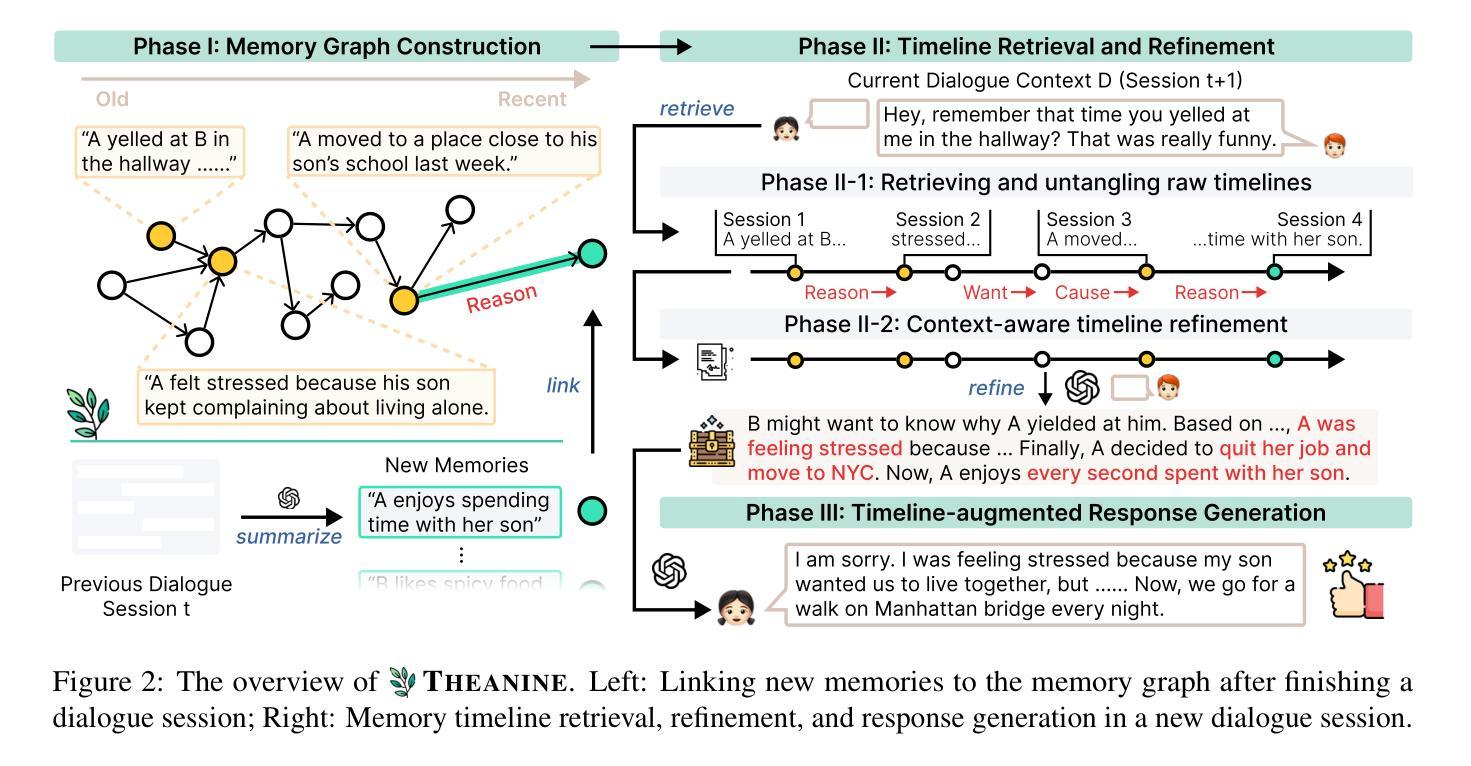
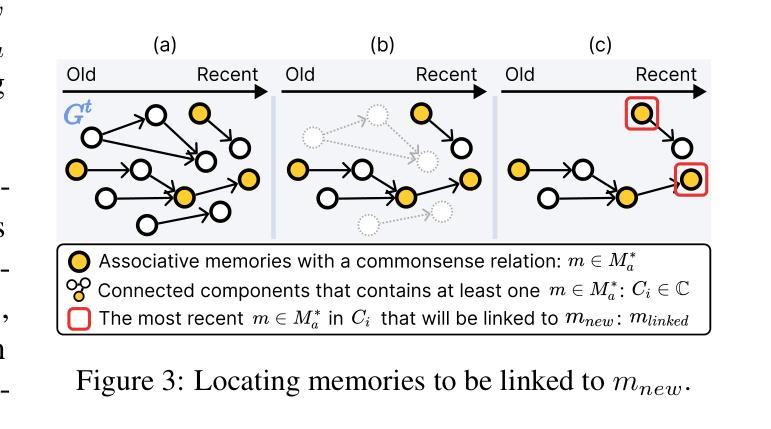
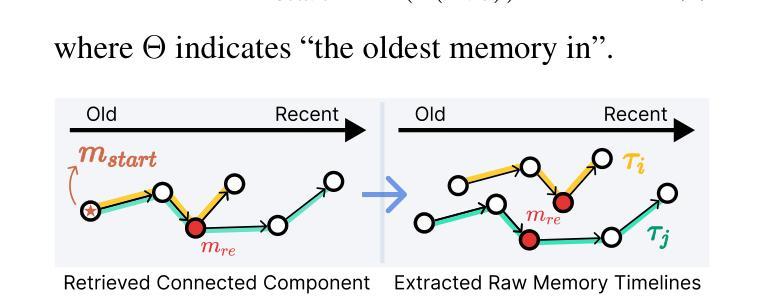
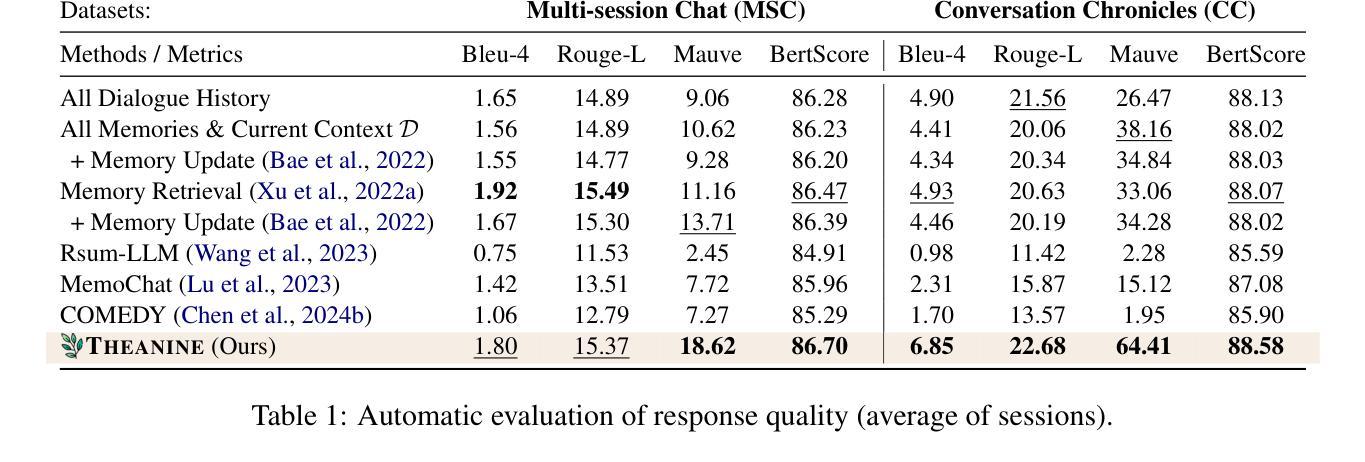
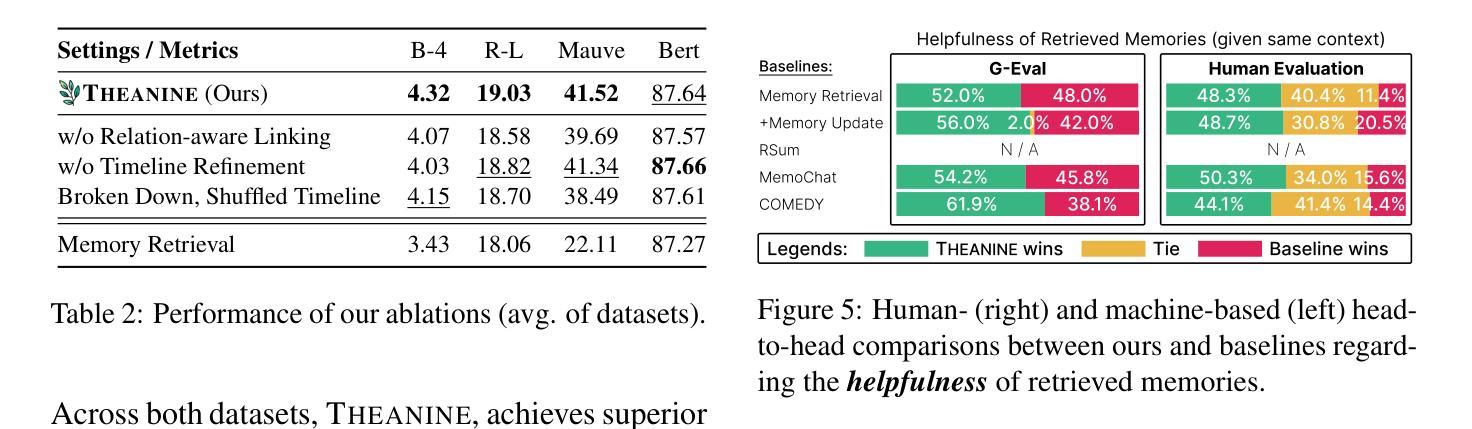
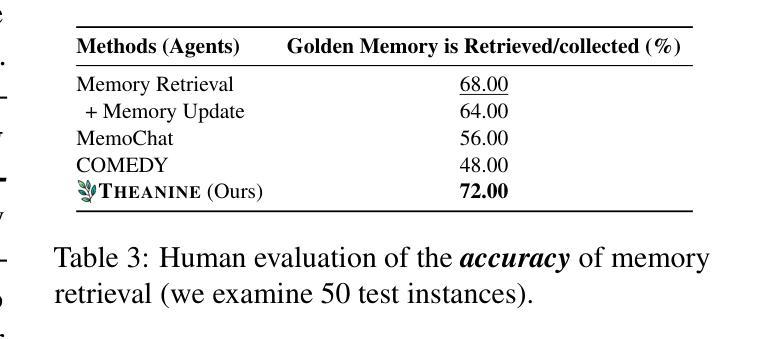