⚠️ 以下所有内容总结都来自于 大语言模型的能力,如有错误,仅供参考,谨慎使用
🔴 请注意:千万不要用于严肃的学术场景,只能用于论文阅读前的初筛!
💗 如果您觉得我们的项目对您有帮助 ChatPaperFree ,还请您给我们一些鼓励!⭐️ HuggingFace免费体验
2025-01-31 更新
VoicePrompter: Robust Zero-Shot Voice Conversion with Voice Prompt and Conditional Flow Matching
Authors:Ha-Yeong Choi, Jaehan Park
Despite remarkable advancements in recent voice conversion (VC) systems, enhancing speaker similarity in zero-shot scenarios remains challenging. This challenge arises from the difficulty of generalizing and adapting speaker characteristics in speech within zero-shot environments, which is further complicated by mismatch between the training and inference processes. To address these challenges, we propose VoicePrompter, a robust zero-shot VC model that leverages in-context learning with voice prompts. VoicePrompter is composed of (1) a factorization method that disentangles speech components and (2) a DiT-based conditional flow matching (CFM) decoder that conditions on these factorized features and voice prompts. Additionally, (3) latent mixup is used to enhance in-context learning by combining various speaker features. This approach improves speaker similarity and naturalness in zero-shot VC by applying mixup to latent representations. Experimental results demonstrate that VoicePrompter outperforms existing zero-shot VC systems in terms of speaker similarity, speech intelligibility, and audio quality. Our demo is available at \url{https://hayeong0.github.io/VoicePrompter-demo/}.
尽管近期语音转换(VC)系统取得了显著进展,但在零样本场景中增强说话人相似性仍然具有挑战性。这一挑战源于在零样本环境中对语音的说话人特性进行通用化和适应的困难,而训练和推理过程之间的不匹配进一步加剧了这一挑战。为了解决这些挑战,我们提出了VoicePrompter,一个稳健的零样本语音转换模型,它利用语境学习配合语音提示。VoicePrompter由以下部分组成:(1)一种分解方法,用于分解语音成分;(2)一个基于DiT的条件流匹配(CFM)解码器,该解码器基于这些分解特征和语音提示进行条件设置。此外,(3)潜混合方法用于通过结合各种说话人特征来增强语境学习。通过将对潜在表示应用混合方法,这种方法提高了零样本语音转换中的说话人相似性和自然度。实验结果表明,VoicePrompter在说话人相似性、语音清晰度和音频质量方面优于现有的零样本VC系统。我们的演示作品可在[https://hayeong0.github.io/VoicePrompter-demo/]上查看。
论文及项目相关链接
PDF Accepted at ICASSP 2025
Summary
近期语音转换(VC)系统在技术上取得了显著进展,但在零样本场景(zero-shot scenarios)下提高说话人相似性仍然具有挑战性。为应对这一挑战,提出了一种名为VoicePrompter的稳健零样本VC模型,该模型结合语境学习(in-context learning)与语音提示(voice prompts)。模型包含因子分解方法和基于扩散Transformer(DiT)的条件流匹配(CFM)解码器,该解码器通过利用这些分解特性和语音提示来实现调控。此外,通过潜在混合(latent mixup)技术增强语境学习,结合不同说话人的特征。此方法提高了零样本VC的说话人相似性和自然度。实验结果显示,VoicePrompter在零样本语音转换方面相较于现有系统更胜一筹,显著提高了说话人相似性、语音清晰度和音质。我们的演示模型可通过以下网址访问:https://hayeong0.github.io/VoicePrompter-demo/。。
Key Takeaways
- VoicePrompter是一个针对零样本场景下的语音转换(VC)挑战而设计的模型。
- 该模型结合了语境学习(in-context learning)和语音提示(voice prompts),以提高说话人相似性。
- VoicePrompter包括因子分解方法,用于分离语音成分。
- 使用基于扩散Transformer(DiT)的条件流匹配(CFM)解码器调控分离后的语音成分和语音提示。
- 通过潜在混合(latent mixup)技术增强语境学习,通过结合不同说话人的特征来提高性能。
- VoicePrompter显著提高了零样本VC的说话人相似性、语音清晰度和音质。
点此查看论文截图
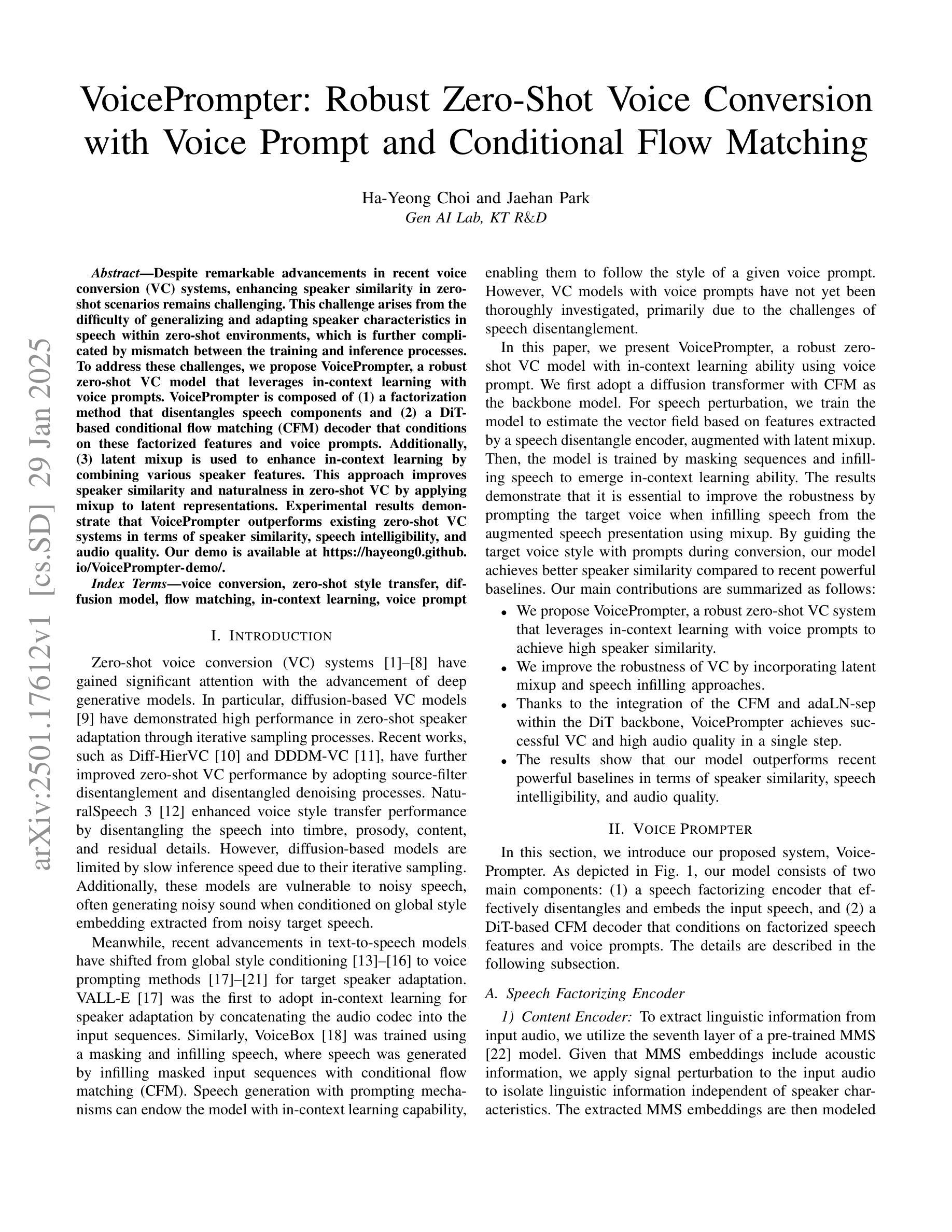
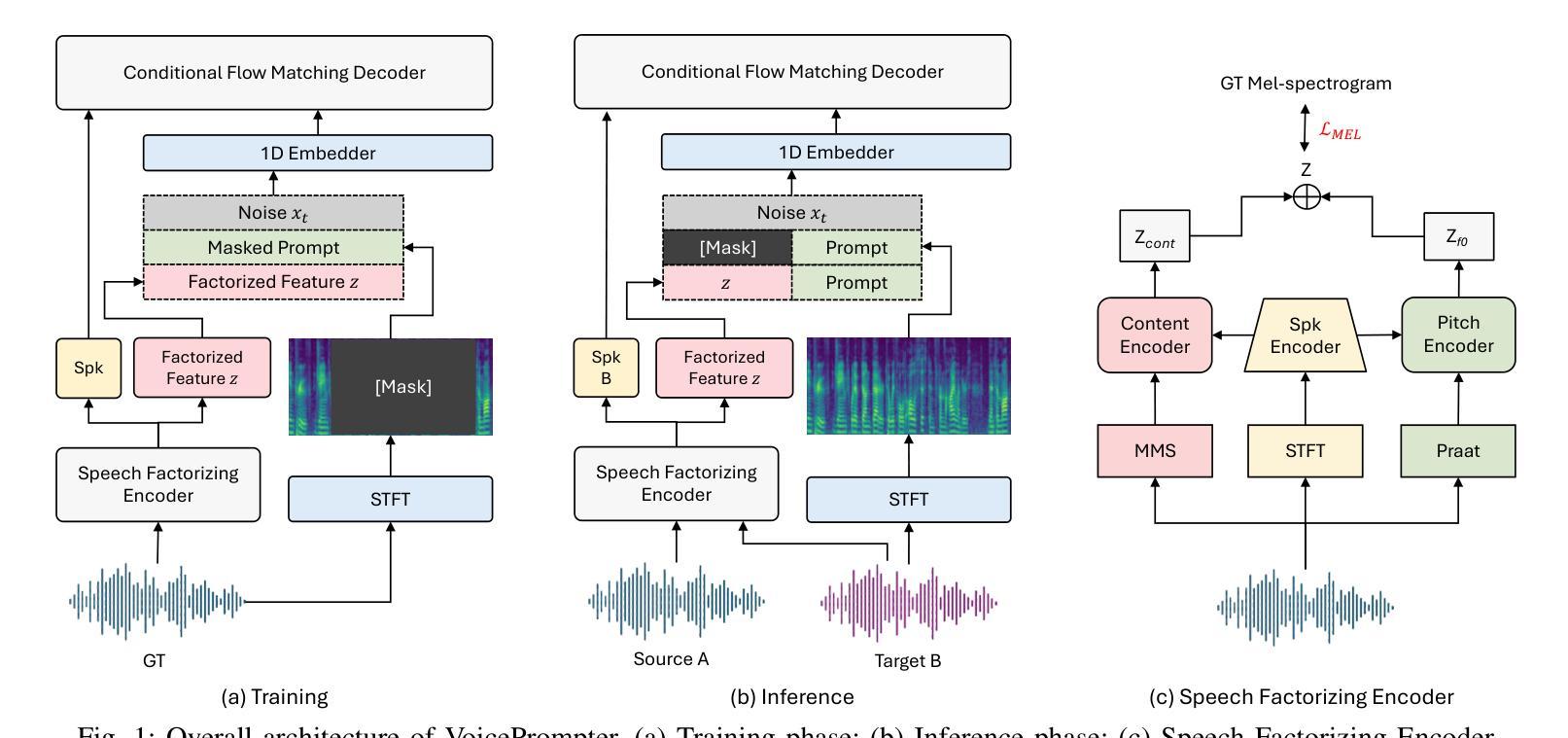
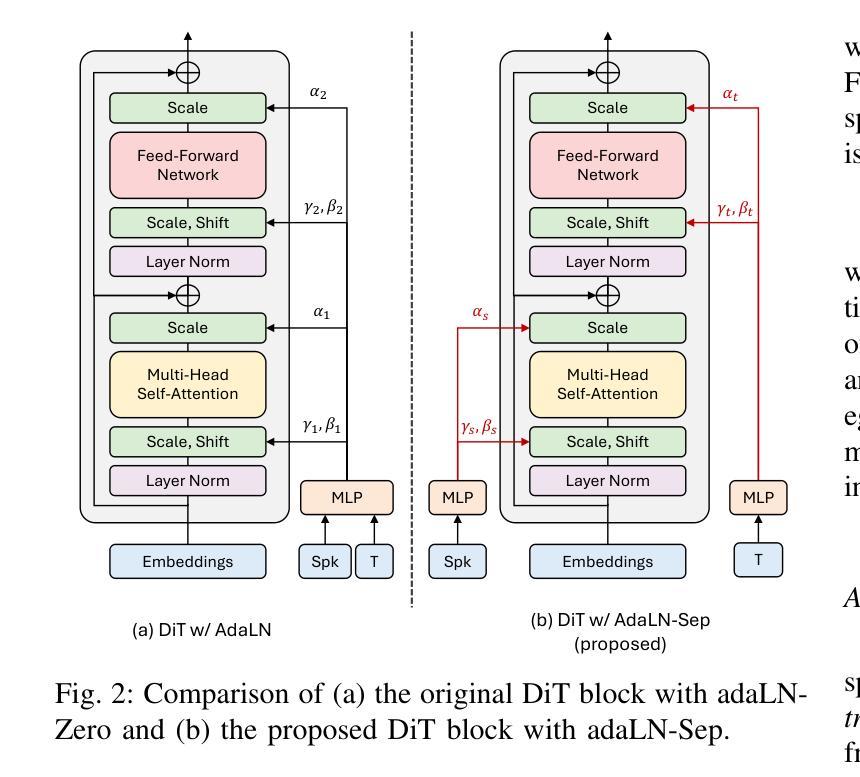
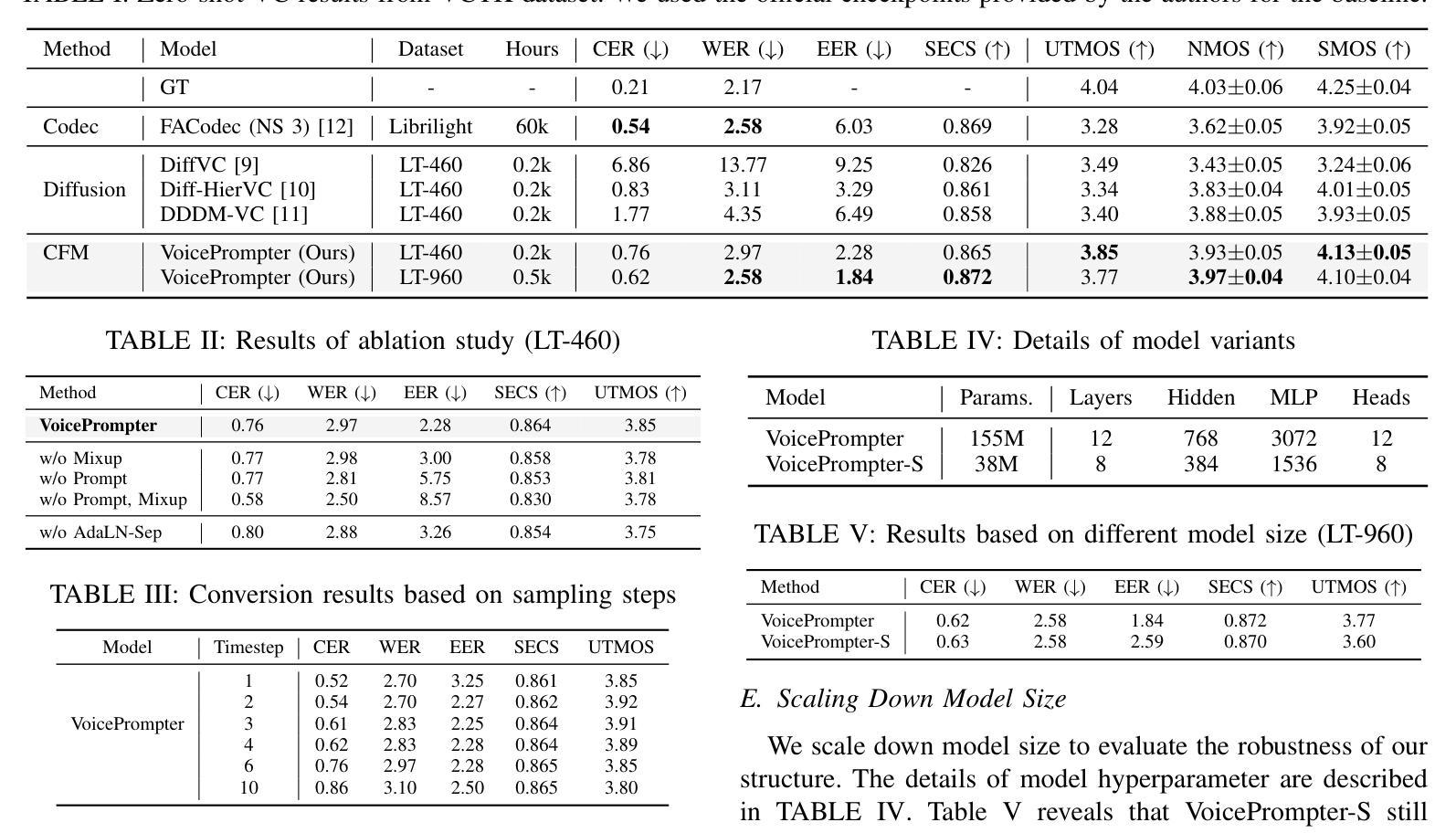
SCDiar: a streaming diarization system based on speaker change detection and speech recognition
Authors:Naijun Zheng, Xucheng Wan, Kai Liu, Zhou Huan
In hours-long meeting scenarios, real-time speech stream often struggles with achieving accurate speaker diarization, commonly leading to speaker identification and speaker count errors. To address this challenge, we propose SCDiar, a system that operates on speech segments, split at the token level by a speaker change detection (SCD) module. Building on these segments, we introduce several enhancements to efficiently select the best available segment for each speaker. These improvements lead to significant gains across various benchmarks. Notably, on real-world meeting data involving more than ten participants, SCDiar outperforms previous systems by up to 53.6% in accuracy, substantially narrowing the performance gap between online and offline systems.
在长达数小时的会议场景中,实时语音流通常难以实现准确的说话人日记化,这通常会导致说话人识别和说话人数量的错误。为了应对这一挑战,我们提出了SCDiar系统,它采用语音分段操作,每个分段都在词元层面由说话人变更检测(SCD)模块进行分割。基于这些分段,我们对如何有效地为每位说话人选择最佳可用分段进行了多项改进。这些改进在多个基准测试中带来了显著的提升。值得注意的是,在涉及超过十位参与者的真实会议数据中,SCDiar的准确率相较于之前的系统提高了高达53.6%,大大缩小了在线和离线系统之间的性能差距。
论文及项目相关链接
PDF Accepted at ICASSP 2025
Summary
本文提出了一个名为SCDiar的系统,用于解决长时间会议场景中实时语音流的准确说话人识别问题。通过分割语音段并选择最佳可用的段来为每个说话者提供数据,提高了识别效果。在不同基准测试上的结果表现显著,尤其在涉及超过十位参与者的真实会议数据中,SCDiar系统的准确率相较于前系统提升了高达53.6%,大幅缩小了在线与离线系统之间的性能差距。
Key Takeaways
- 在长时间会议场景中,实时语音流面临准确说话人识别的挑战。
- SCDiar系统通过分割语音段,并采用说话人变化检测模块在标记级别进行分割来解决此问题。
- 通过增强选择最佳可用段的功能,为每位说话者提供数据,提高了识别效果。
- 在多个基准测试中,SCDiar系统表现显著。
- 在涉及超过十位参与者的真实会议数据中,SCDiar系统的准确率提升显著。
- SCDiar系统的应用缩小了在线与离线系统之间的性能差距。
点此查看论文截图
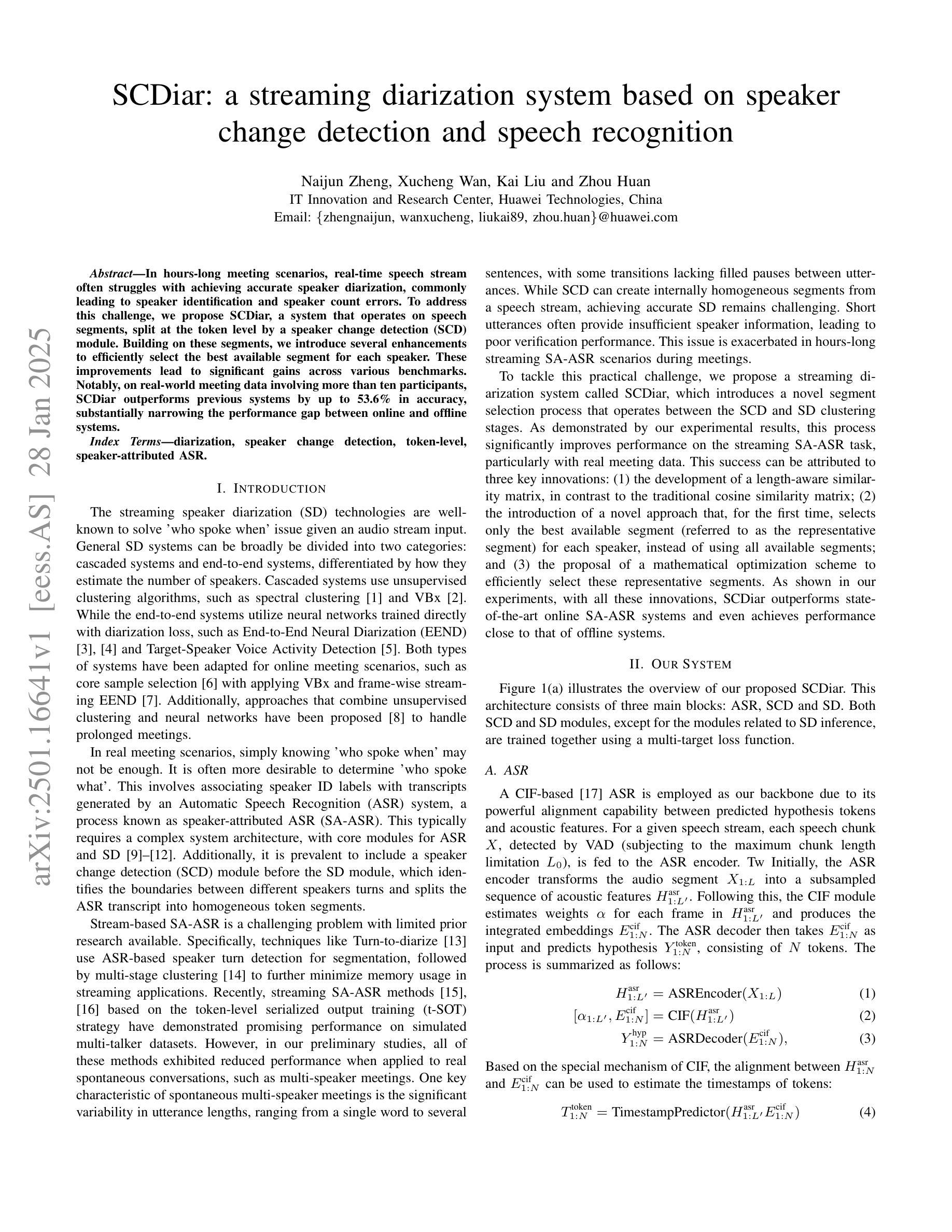
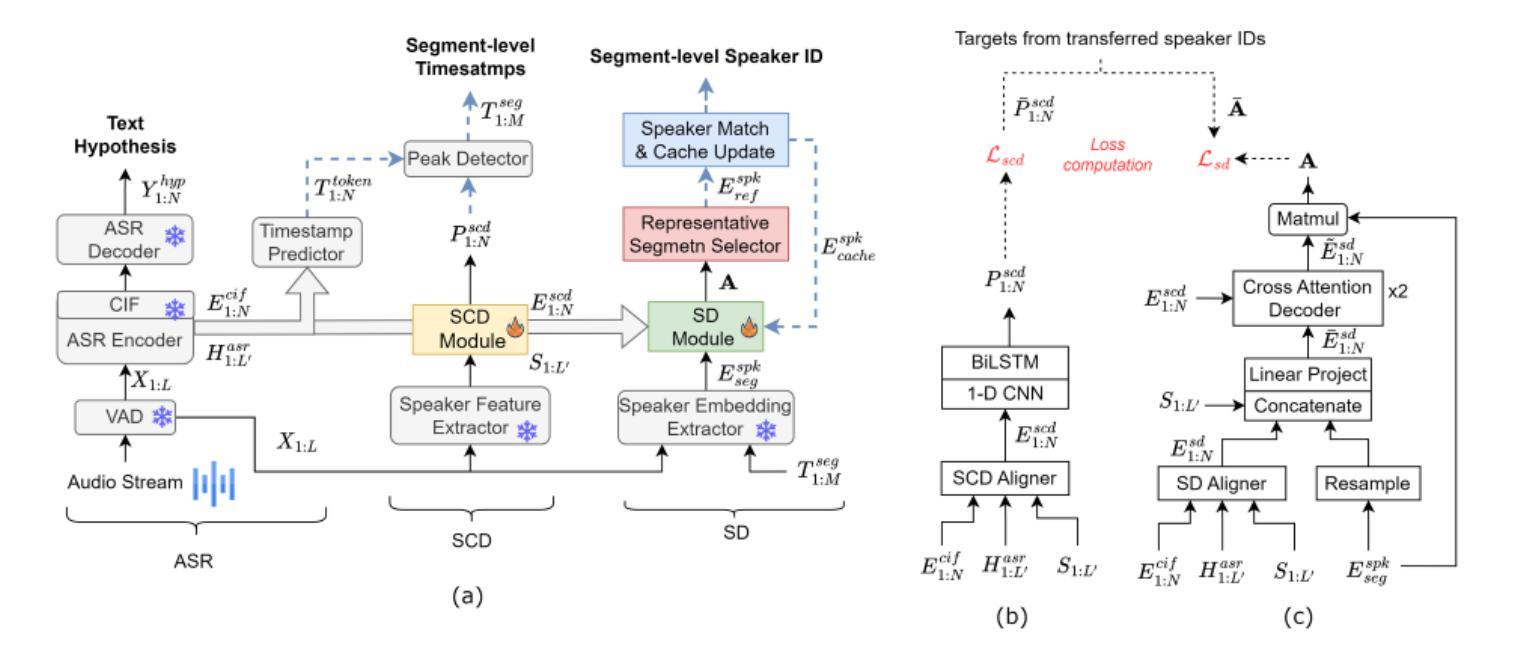
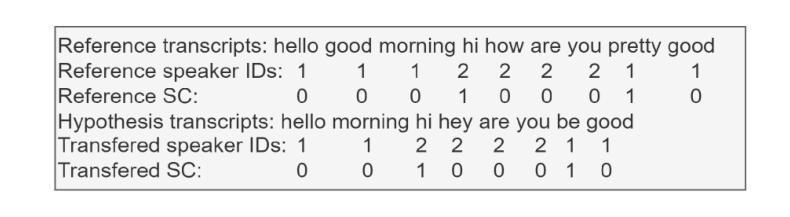
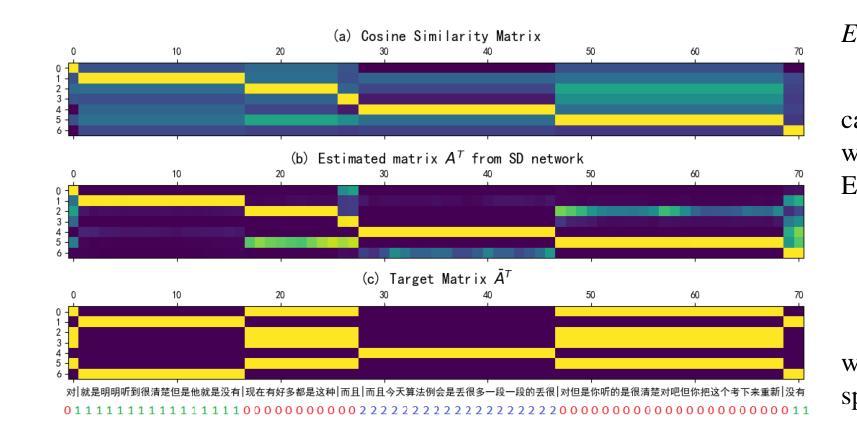
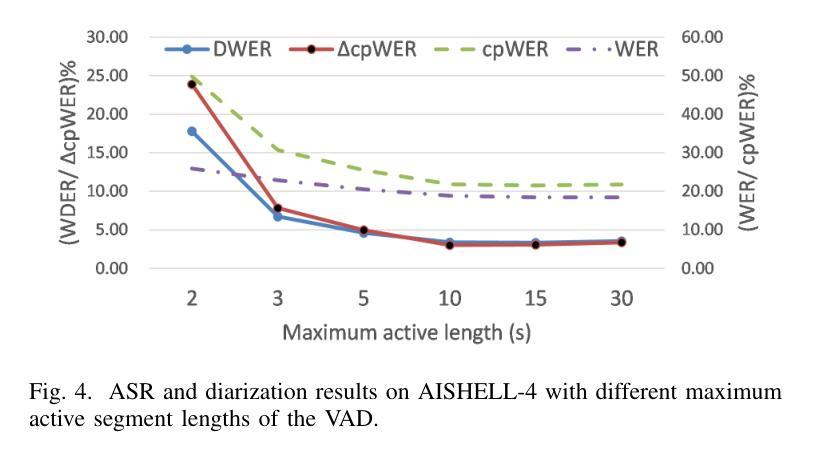
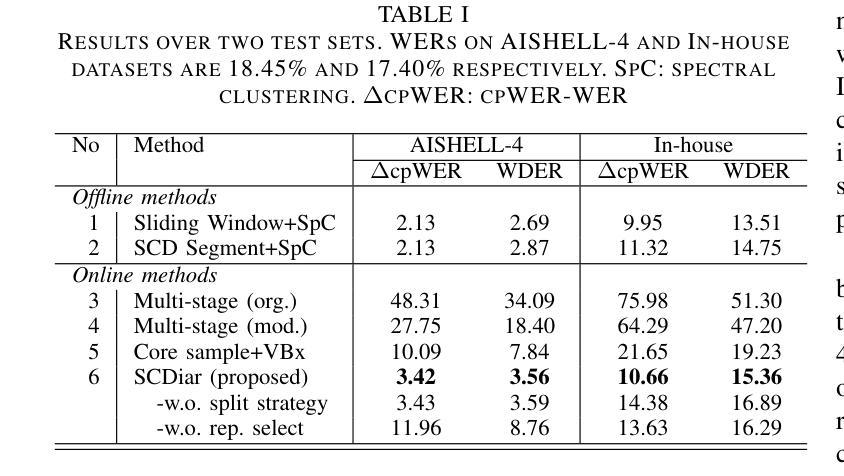
GLDiTalker: Speech-Driven 3D Facial Animation with Graph Latent Diffusion Transformer
Authors:Yihong Lin, Zhaoxin Fan, Xianjia Wu, Lingyu Xiong, Liang Peng, Xiandong Li, Wenxiong Kang, Songju Lei, Huang Xu
Speech-driven talking head generation is a critical yet challenging task with applications in augmented reality and virtual human modeling. While recent approaches using autoregressive and diffusion-based models have achieved notable progress, they often suffer from modality inconsistencies, particularly misalignment between audio and mesh, leading to reduced motion diversity and lip-sync accuracy. To address this, we propose GLDiTalker, a novel speech-driven 3D facial animation model based on a Graph Latent Diffusion Transformer. GLDiTalker resolves modality misalignment by diffusing signals within a quantized spatiotemporal latent space. It employs a two-stage training pipeline: the Graph-Enhanced Quantized Space Learning Stage ensures lip-sync accuracy, while the Space-Time Powered Latent Diffusion Stage enhances motion diversity. Together, these stages enable GLDiTalker to generate realistic, temporally stable 3D facial animations. Extensive evaluations on standard benchmarks demonstrate that GLDiTalker outperforms existing methods, achieving superior results in both lip-sync accuracy and motion diversity.
语音驱动的头部生成是一项至关重要且具有挑战性的任务,在增强现实和虚拟人类建模等领域具有广泛的应用。虽然最近使用自回归和扩散模型的方法取得了显著的进步,但它们经常遭受模态不一致的问题,特别是音频和网格之间的不匹配,导致运动多样性和唇同步准确性降低。为了解决这一问题,我们提出了GLDiTalker,这是一个基于图潜在扩散变压器的新型语音驱动3D面部动画模型。GLDiTalker通过量化时空潜在空间内的信号扩散来解决模态不匹配问题。它采用两阶段训练流程:图增强量化空间学习阶段确保唇同步准确性,而时空动力潜在扩散阶段增强运动多样性。这两个阶段共同作用,使GLDiTalker能够生成逼真、时间稳定的3D面部动画。在标准基准测试上的广泛评估表明,GLDiTalker优于现有方法,在唇同步准确性和运动多样性方面都取得了优越的结果。
论文及项目相关链接
PDF 9 pages, 5 figures
Summary
文本提出了一种基于Graph Latent Diffusion Transformer的语音驱动3D面部动画模型GLDiTalker。为解决模态不一致问题,该模型在量化时空潜在空间内扩散信号,采用两阶段训练管道,确保唇同步准确性并增强运动多样性,生成逼真、时间稳定的3D面部动画。
Key Takeaways
- 文本介绍了语音驱动的头部生成任务的重要性和挑战性,以及其在增强现实和虚拟人类建模中的应用。
- 现有方法使用自回归和扩散模型取得显著进展,但存在模态不一致问题,特别是音频与网格之间的不匹配,导致运动多样性和唇同步准确性降低。
- GLDiTalker是一个基于Graph Latent Diffusion Transformer的语音驱动3D面部动画模型,旨在解决模态不匹配问题。
- GLDiTalker通过在量化时空潜在空间内扩散信号来解决模态不一致问题。
- 模型采用两阶段训练管道,第一阶段确保唇同步准确性,第二阶段增强运动多样性。
- GLDiTalker能够生成逼真、时间稳定的3D面部动画。
点此查看论文截图
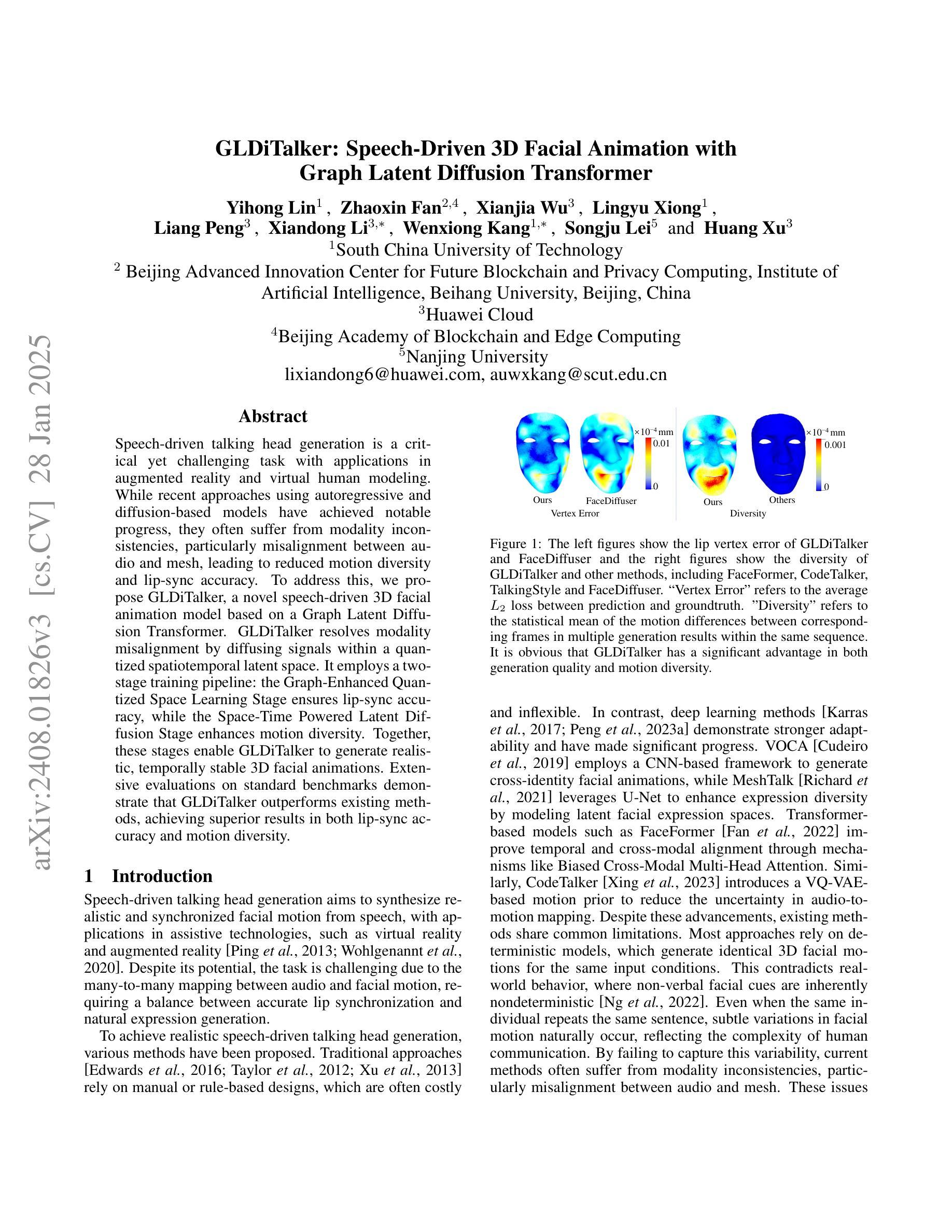
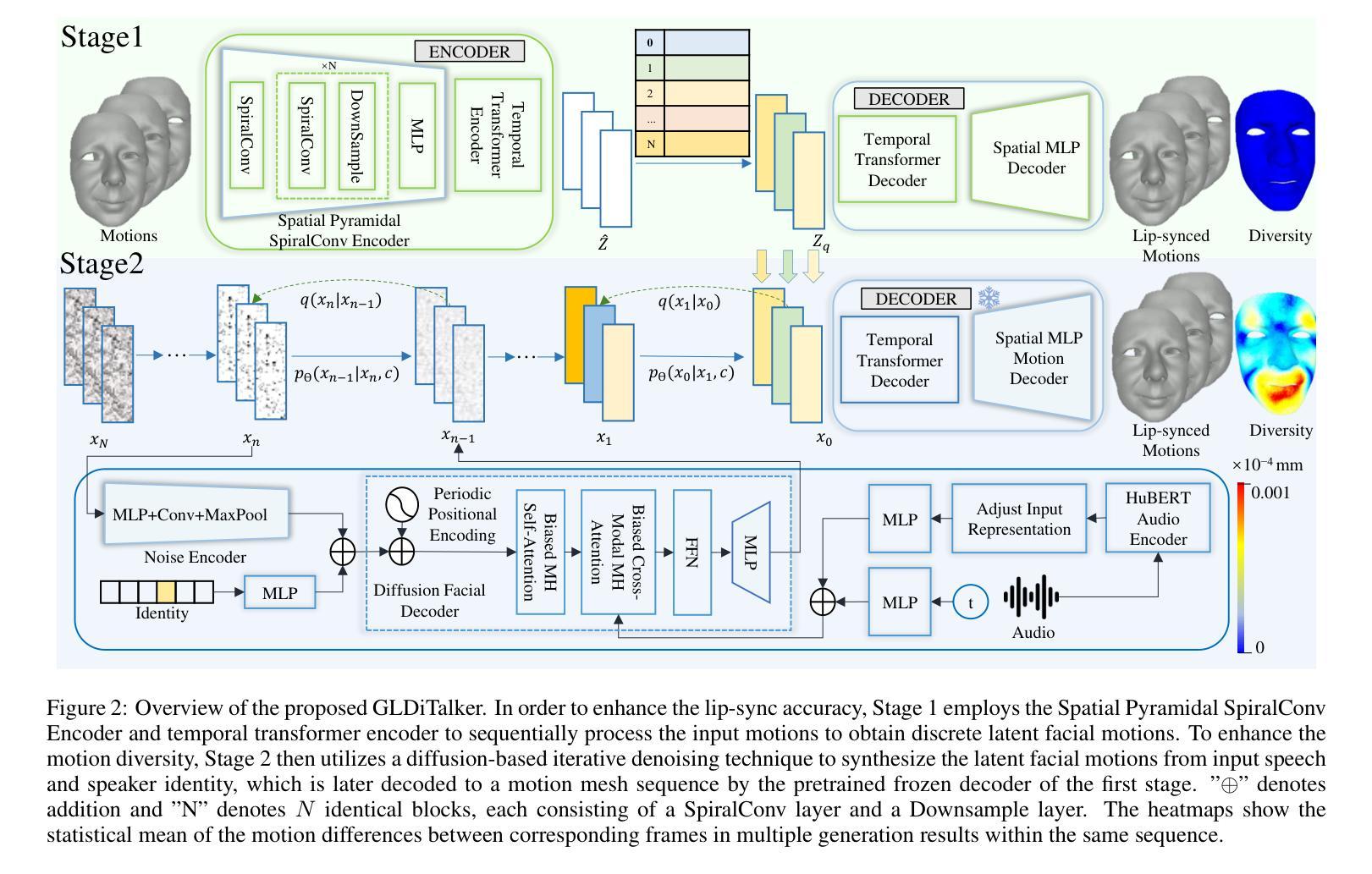
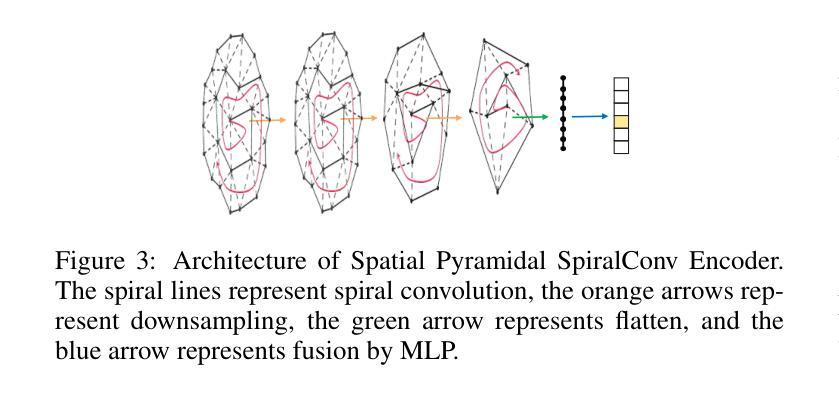
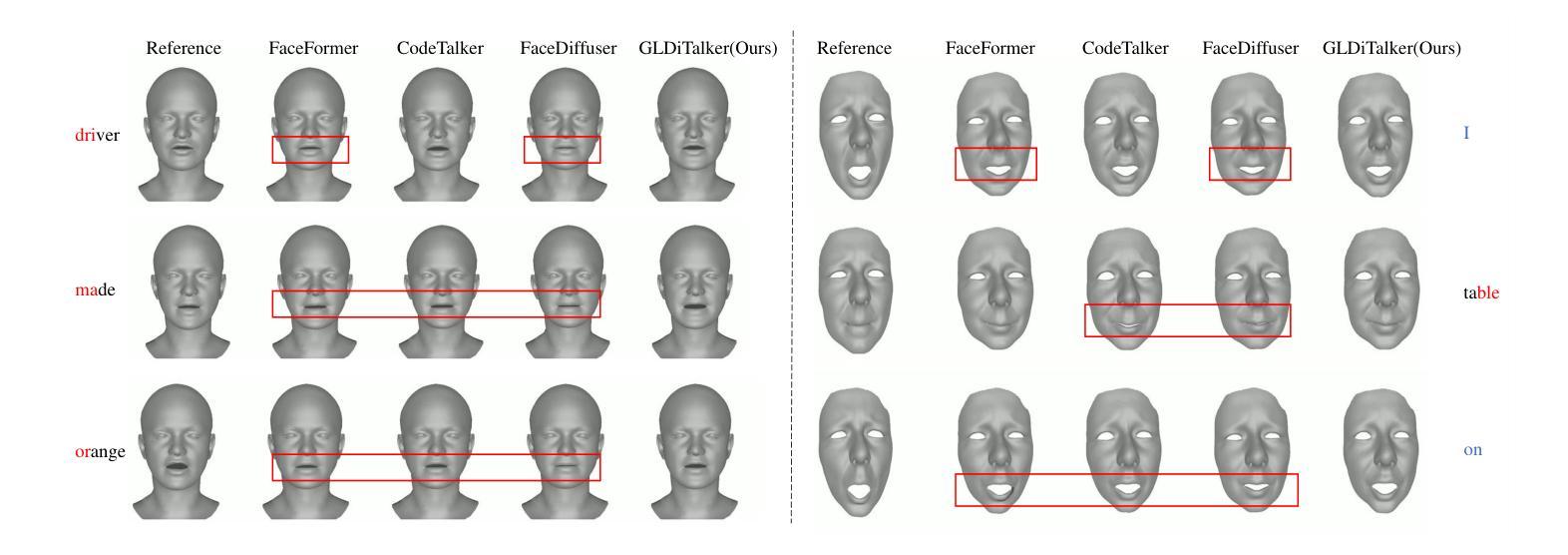
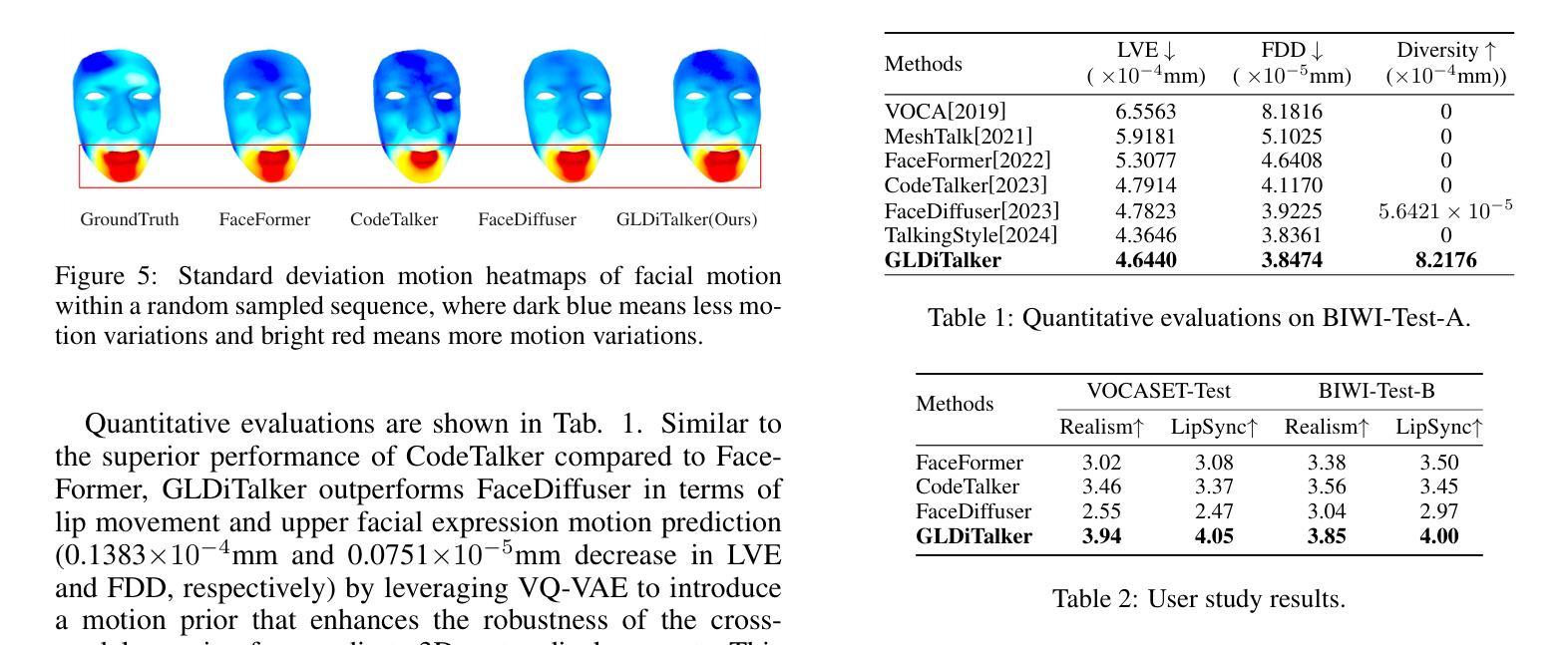
Continuously Learning New Words in Automatic Speech Recognition
Authors:Christian Huber, Alexander Waibel
Despite recent advances, Automatic Speech Recognition (ASR) systems are still far from perfect. Typical errors include acronyms, named entities, and domain-specific special words for which little or no labeled data is available. To address the problem of recognizing these words, we propose a self-supervised continual learning approach: Given the audio of a lecture talk with the corresponding slides, we bias the model towards decoding new words from the slides by using a memory-enhanced ASR model from the literature. Then, we perform inference on the talk, collecting utterances that contain detected new words into an adaptation data set. Continual learning is then performed by training adaptation weights added to the model on this data set. The whole procedure is iterated for many talks. We show that with this approach, we obtain increasing performance on the new words when they occur more frequently (more than 80% recall) while preserving the general performance of the model.
尽管最近的进展很大,但自动语音识别(ASR)系统仍然远非完美。常见的错误包括缩写词、命名实体和特定领域的特殊词汇,对于这些词汇,可用的小标签数据很少或根本没有。为了解决识别这些单词的问题,我们提出了一种基于自监督的连续学习方法:给定带有相应幻灯片的讲座音频,我们通过使用文献中的带有内存的ASR模型,使模型偏向于从幻灯片中解码新单词。然后,我们对讲座进行推理,收集包含检测到的新词的语音片段,并将其放入适应数据集。随后,通过在此数据集上训练模型的附加适应权重来执行连续学习。整个过程针对多次讲座进行迭代。我们表明,通过这种方法,当新单词出现更频繁时,我们在新单词上的性能会得到提高(召回率超过80%),同时保持模型的整体性能。
论文及项目相关链接
PDF Accepted at ICASSP 2025
Summary
本文提出一种基于自监督的连续学习方法来解决自动语音识别(ASR)系统中存在的问题,特别是针对识别术语、专有名词和特定领域词汇的挑战。该方法通过结合音频讲座和对应幻灯片内容,训练模型偏向从幻灯片解码新词。在讲座中进行推理,收集包含检测到的新词的语句作为适应数据集。通过连续学习,在适应数据集上训练模型,并多次迭代该过程。实验表明,该方法在新词出现频率增加时取得了良好的性能提升(召回率超过80%),同时保持了模型的总体性能。
Key Takeaways
- 自动语音识别(ASR)系统仍存在识别术语、专有名词和特定领域词汇的挑战。
- 提出一种自监督的连续学习方法来解决这些问题。
- 通过结合音频讲座和对应幻灯片内容,训练模型偏向从幻灯片解码新词。
- 收集包含检测到的新词的语句作为适应数据集。
- 连续学习通过训练适应数据集上的模型来提升对新词的识别性能。
- 实验结果显示,该方法在新词出现频率增加时性能提升显著(召回率超过80%)。
点此查看论文截图
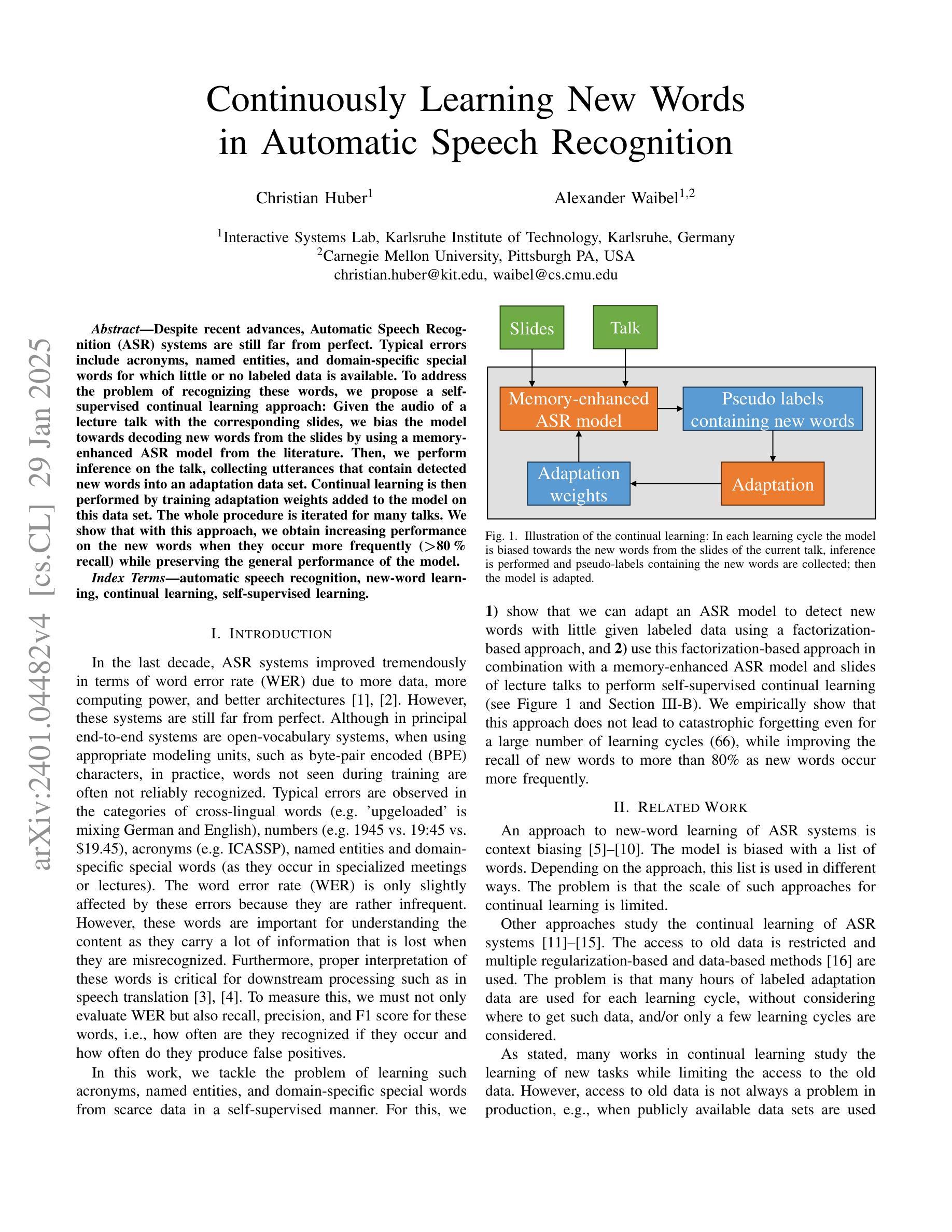
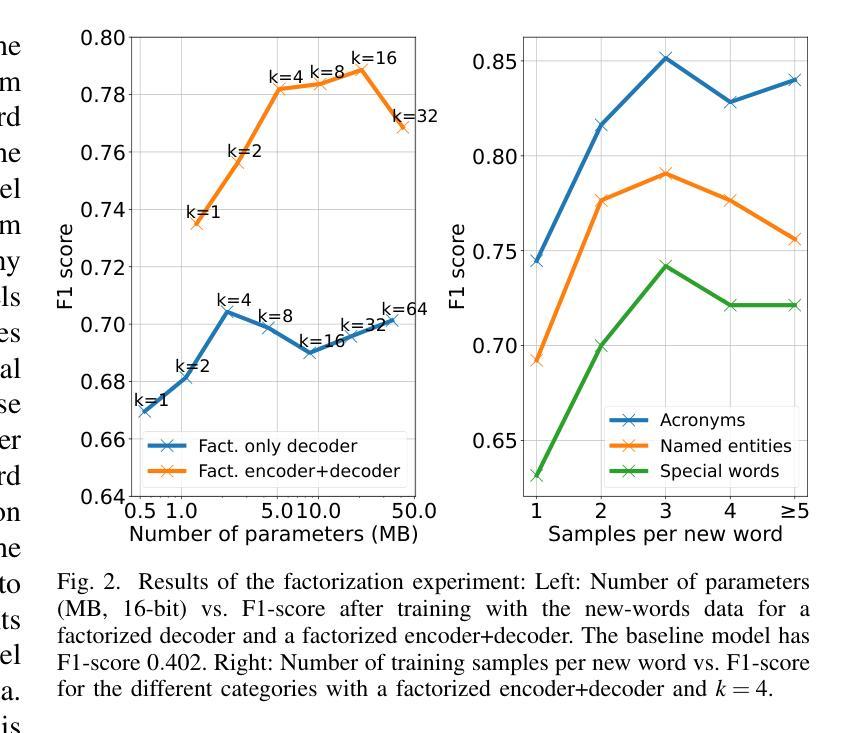
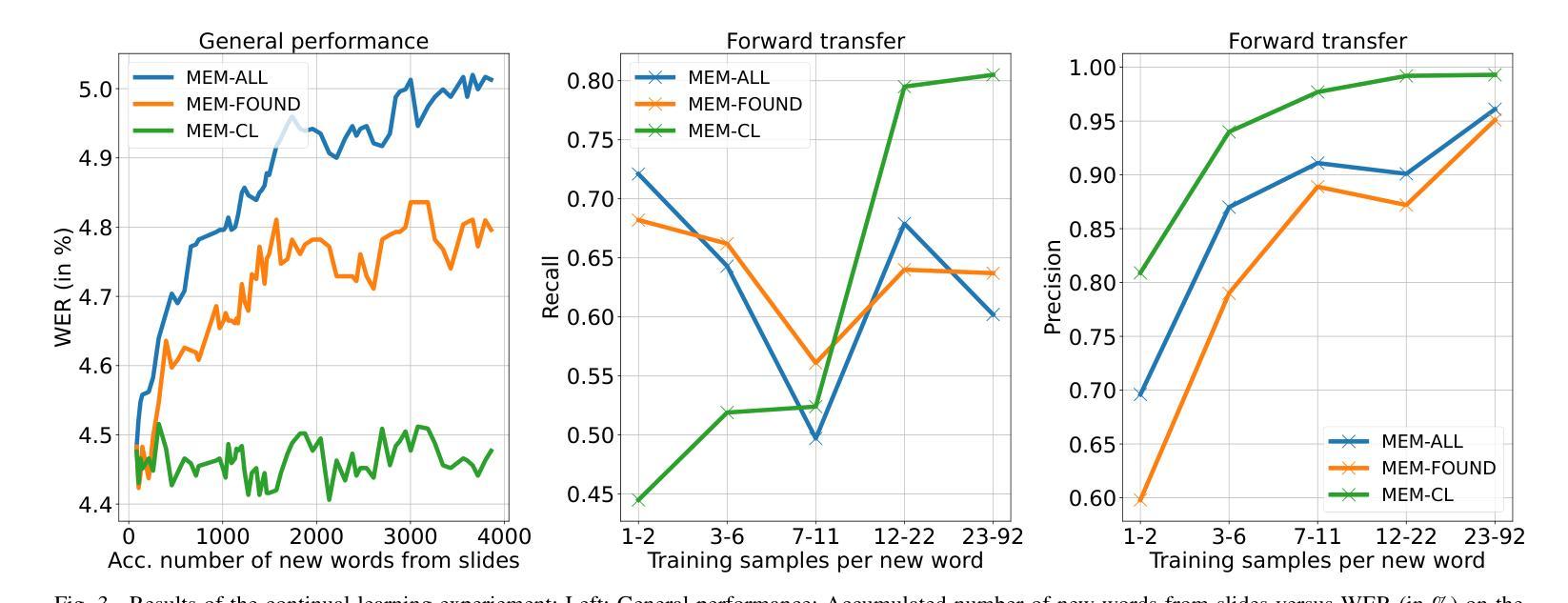
Fast Word Error Rate Estimation Using Self-Supervised Representations for Speech and Text
Authors:Chanho Park, Chengsong Lu, Mingjie Chen, Thomas Hain
Word error rate (WER) estimation aims to evaluate the quality of an automatic speech recognition (ASR) system’s output without requiring ground-truth labels. This task has gained increasing attention as advanced ASR systems are trained on large amounts of data. In this context, the computational efficiency of a WER estimator becomes essential in practice. However, previous works have not prioritised this aspect. In this paper, a Fast estimator for WER (Fe-WER) is introduced, utilizing average pooling over self-supervised learning representations for speech and text. Our results demonstrate that Fe-WER outperformed a baseline relatively by 14.10% in root mean square error and 1.22% in Pearson correlation coefficient on Ted-Lium3. Moreover, a comparative analysis of the distributions of target WER and WER estimates was conducted, including an examination of the average values per speaker. Lastly, the inference speed was approximately 3.4 times faster in the real-time factor.
自动语音识别(ASR)系统的输出质量评估不需要真实的标签即可进行,称为词错误率(WER)估计。随着在大量数据上训练的先进ASR系统的出现,这项任务受到了越来越多的关注。在这种情况下,WER估算器的计算效率在实践中变得至关重要。然而,以前的研究并没有优先考虑这一方面。本文介绍了一种基于语音和文本自监督学习表示的WER快速估算器(Fe-WER)。我们的结果表明,在Ted-Lium3数据集上,Fe-WER相对于基线在均方根误差上提高了14.10%,在皮尔逊相关系数上提高了1.22%。此外,还对目标WER和WER估计的分布进行了对比分析,包括对每个说话人的平均值的分析。最后,其实时推理速度约为真实速度的3.4倍。
论文及项目相关链接
PDF 5 pages, accepted by ICASSP 2025
Summary
本文介绍了一种用于评估自动语音识别(ASR)系统输出质量的快速词错误率(WER)估计器(Fe-WER)。它利用自我监督学习表示的语音和文本的平均池化方法,表现出优异的性能。相较于基线模型,Fe-WER在Ted-Lium3数据集上显著降低根均方误差并提高了皮尔逊相关系数。此外,其推理速度提高了约3.4倍。
Key Takeaways
- Fe-WER旨在评估ASR系统输出质量,无需依赖真实标签。
- Fe-WER利用自我监督学习表示的语音和文本的平均池化方法。
- 在Ted-Lium3数据集上,Fe-WER相较于基线模型在性能上有所改进。
- Fe-WER提高了词错误率估计的计算效率。
- 对比分析了目标WER和WER估计的分布,以及每说话人的平均值。
- Fe-WER的推理速度比传统方法更快。
点此查看论文截图
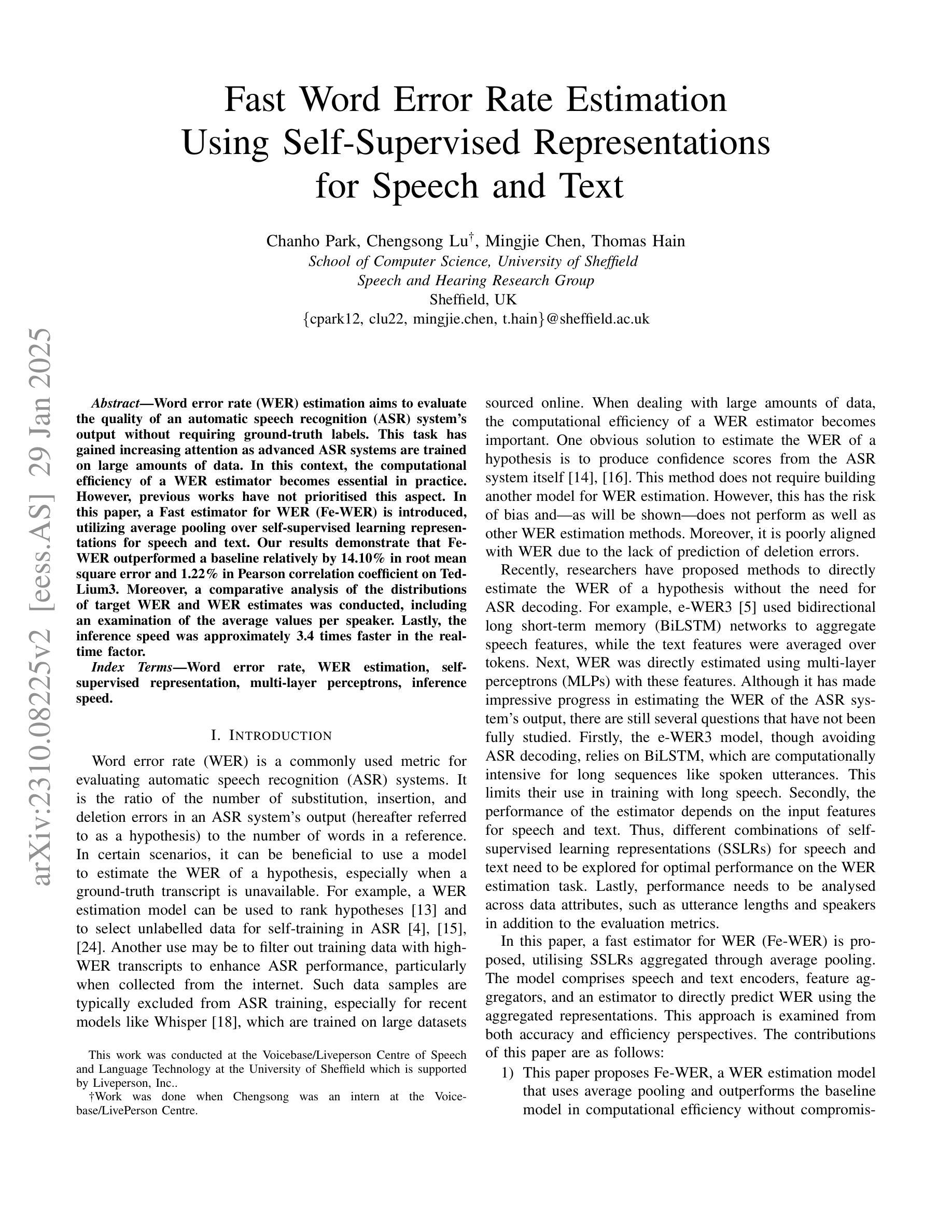


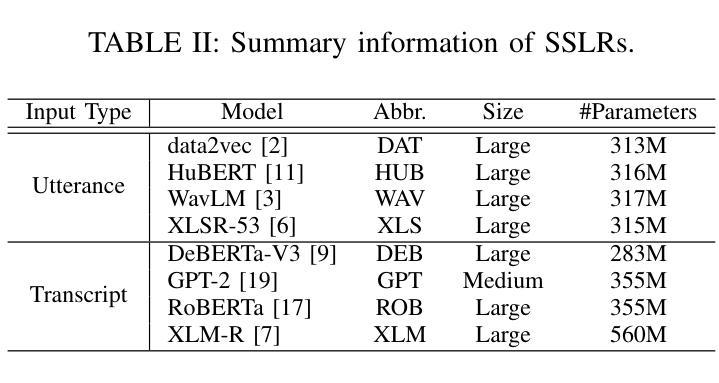
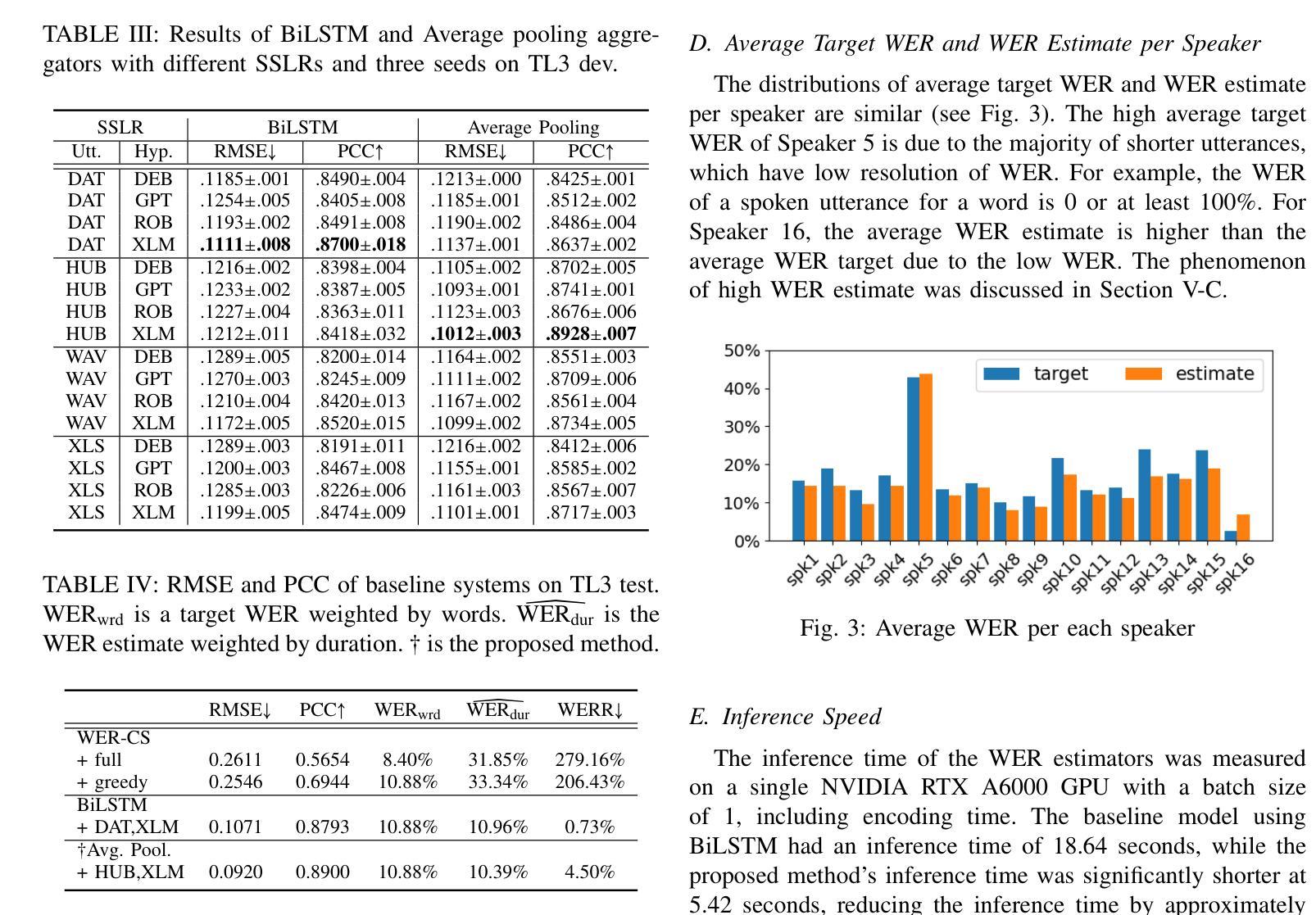
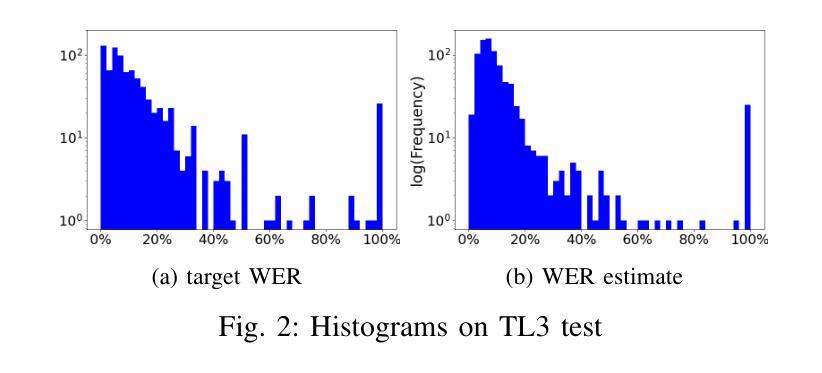
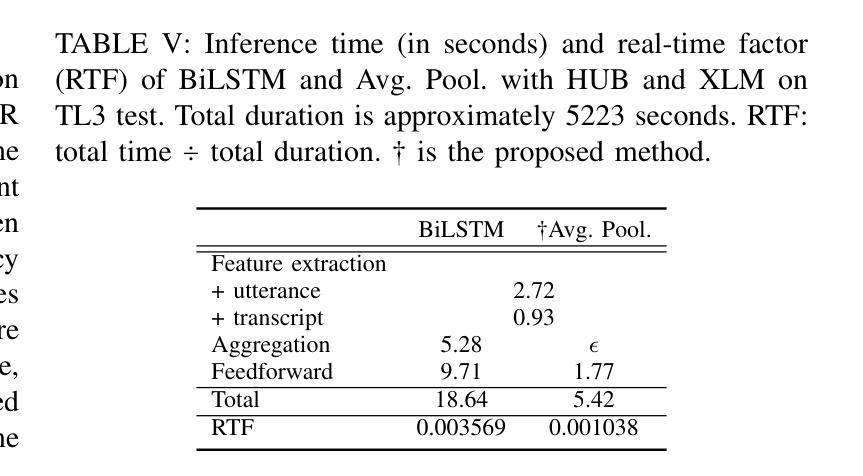