⚠️ 以下所有内容总结都来自于 大语言模型的能力,如有错误,仅供参考,谨慎使用
🔴 请注意:千万不要用于严肃的学术场景,只能用于论文阅读前的初筛!
💗 如果您觉得我们的项目对您有帮助 ChatPaperFree ,还请您给我们一些鼓励!⭐️ HuggingFace免费体验
2025-02-01 更新
Assessing the Capability of YOLO- and Transformer-based Object Detectors for Real-time Weed Detection
Authors:Alicia Allmendinger, Ahmet Oğuz Saltık, Gerassimos G. Peteinatos, Anthony Stein, Roland Gerhards
Spot spraying represents an efficient and sustainable method for reducing the amount of pesticides, particularly herbicides, used in agricultural fields. To achieve this, it is of utmost importance to reliably differentiate between crops and weeds, and even between individual weed species in situ and under real-time conditions. To assess suitability for real-time application, different object detection models that are currently state-of-the-art are compared. All available models of YOLOv8, YOLOv9, YOLOv10, and RT-DETR are trained and evaluated with images from a real field situation. The images are separated into two distinct datasets: In the initial data set, each species of plants is trained individually; in the subsequent dataset, a distinction is made between monocotyledonous weeds, dicotyledonous weeds, and three chosen crops. The results demonstrate that while all models perform equally well in the metrics evaluated, the YOLOv9 models, particularly the YOLOv9s and YOLOv9e, stand out in terms of their strong recall scores (66.58 % and 72.36 %), as well as mAP50 (73.52 % and 79.86 %), and mAP50-95 (43.82 % and 47.00 %) in dataset 2. However, the RT-DETR models, especially RT-DETR-l, excel in precision with reaching 82.44 % on dataset 1 and 81.46 % in dataset 2, making them particularly suitable for scenarios where minimizing false positives is critical. In particular, the smallest variants of the YOLO models (YOLOv8n, YOLOv9t, and YOLOv10n) achieve substantially faster inference times down to 7.58 ms for dataset 2 on the NVIDIA GeForce RTX 4090 GPU for analyzing one frame, while maintaining competitive accuracy, highlighting their potential for deployment in resource-constrained embedded computing devices as typically used in productive setups.
点喷技术是一种高效的可持续方法,可以减少农田中农药,特别是除草剂的用量。为实现这一点,在实时条件下可靠地区分作物和杂草,甚至区分不同的杂草种类是至关重要的。为评估实时应用的适用性,对目前最先进的各种目标检测模型进行了比较。使用来自实际现场情况的图像,对YOLOv8、YOLOv9、YOLOv10和RT-DETR的所有可用模型进行了训练和评估。这些图像被分为两个独立的数据集:在初始数据集中,每种植物分别进行训练;在后续的数据集中,区分单子叶杂草、双子叶杂草和三种选定作物。结果表明,虽然所有模型在评估的指标上表现同样出色,但在数据集2中,YOLOv9模型(特别是YOLOv9s和YOLOv9e)在召回率方面表现出色(分别为66.58%和72.36%),以及mAP50(分别为73.52%和79.86%)和mAP50-95(分别为43.82%和47.00%)。然而,RT-DETR模型(尤其是RT-DETR-l)在精确性方面表现出色,数据集1和数据集2的精确度分别达到了82.44%和81.46%,因此它们在尽量减少误报的关键场景中特别适用。特别是YOLO模型的最小变体(YOLOv8n、YOLOv9t和YOLOv10n),在NVIDIA GeForce RTX 4090 GPU上分析一帧的时间大大加快,达到数据集2的7.58毫秒,同时保持竞争力准确度,这突显了它们在资源受限的嵌入式计算设备中部署的潜力,通常用于生产环境中。
论文及项目相关链接
Summary
实时喷施技术能有效减少农业中的农药使用量,特别是除草剂。为准确区分农作物与杂草,甚至不同种类的杂草,采用先进的物体检测模型进行评估。对比了YOLOv8、YOLOv9、YOLOv10和RT-DETR等模型的实际应用效果。结果显示,YOLOv9模型在召回率和mAP(平均精度均值)方面表现突出,而RT-DETR模型在精确度上表现优异。此外,较小的YOLO模型在推理速度上更快,且维持较高准确率,适合用于资源有限的嵌入式计算设备。
Key Takeaways
- 实时喷施技术有助于减少农药使用,特别是除草剂。
- 区分农作物与杂草是此技术的关键。
- 对比了多种先进的物体检测模型的效果。
- YOLOv9模型在召回率和mAP方面表现优异。
- RT-DETR模型在精确度上更胜一筹。
- 较小的YOLO模型具有更快的推理速度且维持高准确率。
点此查看论文截图
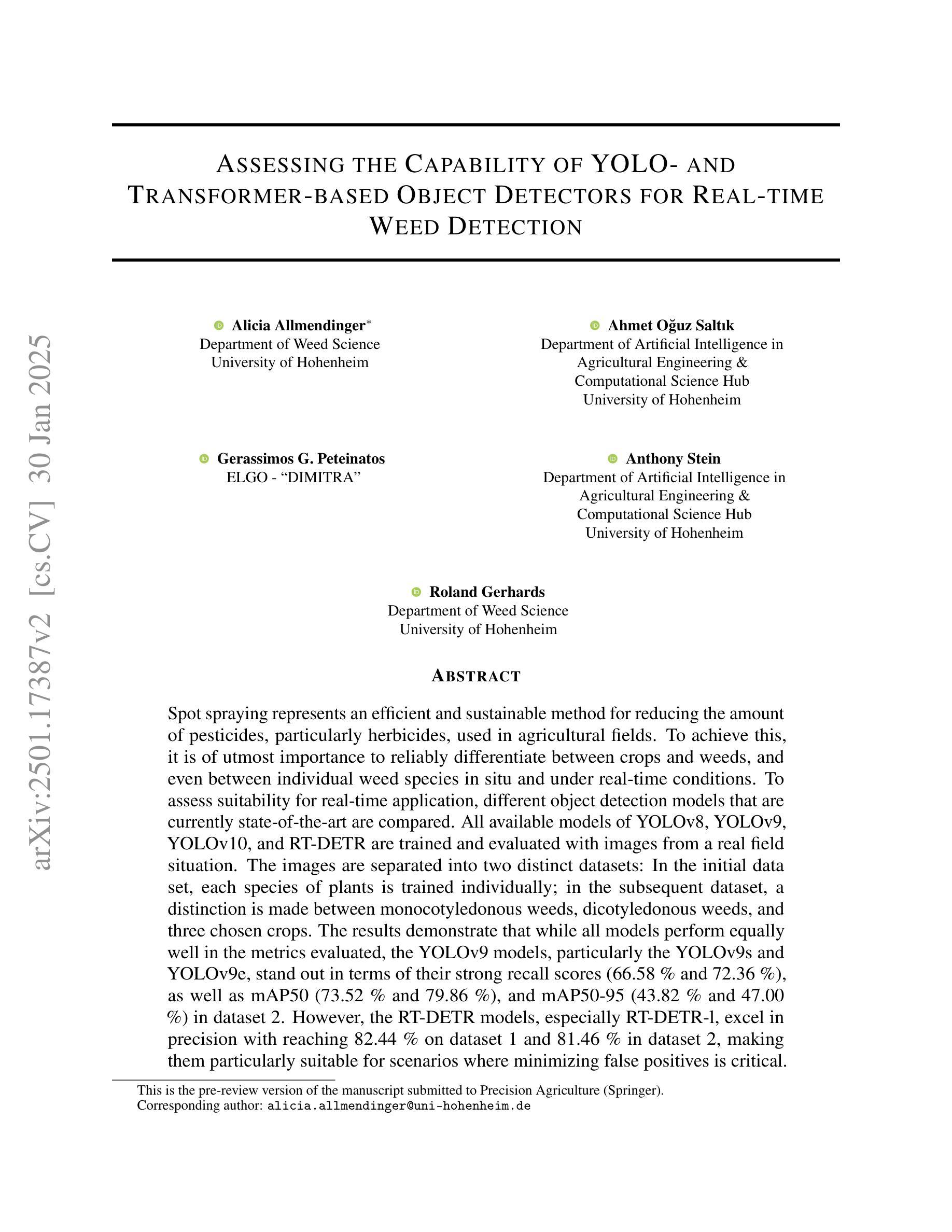
PV-S3: Advancing Automatic Photovoltaic Defect Detection using Semi-Supervised Semantic Segmentation of Electroluminescence Images
Authors:Abhishek Jha, Yogesh Rawat, Shruti Vyas
Photovoltaic (PV) systems allow us to tap into all abundant solar energy, however they require regular maintenance for high efficiency and to prevent degradation. Traditional manual health check, using Electroluminescence (EL) imaging, is expensive and logistically challenging which makes automated defect detection essential. Current automation approaches require extensive manual expert labeling, which is time-consuming, expensive, and prone to errors. We propose PV-S3 (Photovoltaic-Semi Supervised Segmentation), a Semi-Supervised Learning approach for semantic segmentation of defects in EL images that reduces reliance on extensive labeling. PV-S3 is a Deep learning model trained using a few labeled images along with numerous unlabeled images. We introduce a novel Semi Cross-Entropy loss function to deal with class imbalance. We evaluate PV-S3 on multiple datasets and demonstrate its effectiveness and adaptability. With merely 20% labeled samples, we achieve an absolute improvement of 9.7% in IoU, 13.5% in Precision, 29.15% in Recall, and 20.42% in F1-Score over prior state-of-the-art supervised method (which uses 100% labeled samples) on UCF-EL dataset (largest dataset available for semantic segmentation of EL images) showing improvement in performance while reducing the annotation costs by 80%. For more details, visit our GitHub repository:https://github.com/abj247/PV-S3.
光伏(PV)系统使我们能够利用所有丰富的太阳能,然而,为了获得高效率并防止性能退化,它们需要进行定期维护。传统的健康检查方式采用电致发光(EL)成像进行手动检测,这种方法既昂贵又难以实施,因此自动化缺陷检测至关重要。当前的自动化方法需要大量手动专家标注,既耗时又昂贵,还容易出错。我们提出PV-S3(光伏半监督分割),这是一种用于EL图像缺陷语义分割的半监督学习方法,减少对大量标注的依赖。PV-S3是一个深度学习模型,仅使用少量标注图像和大量未标注图像进行训练。我们引入了一种新的半交叉熵损失函数来处理类别不平衡问题。我们在多个数据集上评估了PV-S3,证明了其有效性和适应性。仅使用20%的标注样本,我们在UCF-EL数据集(可用于EL图像语义分割的最大数据集)上的交并比(IoU)提高了9.7%,精确度提高了13.5%,召回率提高了29.15%,F1分数提高了20.42%,超过了先前最先进的监督方法(使用100%标注样本),在降低标注成本的同时提高了性能,降低了80%的标注成本。更多详细信息,请访问我们的GitHub仓库:https://github.com/abj247/PV-S3。
论文及项目相关链接
PDF 19 pages, 10 figures
Summary:本文介绍了一种针对光伏系统的半监督学习方法PV-S3,用于在EL图像中进行缺陷语义分割。该方法使用少量标注图像和大量未标注图像进行训练,引入了一种新的半交叉熵损失函数来解决类别不平衡问题。在UCF-EL数据集上的实验结果表明,PV-S3在仅使用20%标注样本的情况下,相较于使用全部标注样本的现有监督方法在IoU、精确度、召回率和F1分数上均有所提高,同时降低了80%的标注成本。
Key Takeaways:
- 传统的手动健康检查使用电致发光(EL)成像的方式对于光伏系统维护成本高昂且难以实现自动化。
- 当前自动化方法需要大量手动标注数据,耗时长且易出错。
- 提出了一种半监督学习方法PV-S3,用于在EL图像中进行缺陷语义分割,仅使用少量标注图像和大量未标注图像进行训练。
- PV-S3使用半交叉熵损失函数来解决类别不平衡问题。
- 在UCF-EL数据集上的实验结果表明,PV-S3在减少标注样本的情况下,性能优于现有监督方法。
- PV-S3显著提高了IoU、精确度、召回率和F1分数等指标。
点此查看论文截图
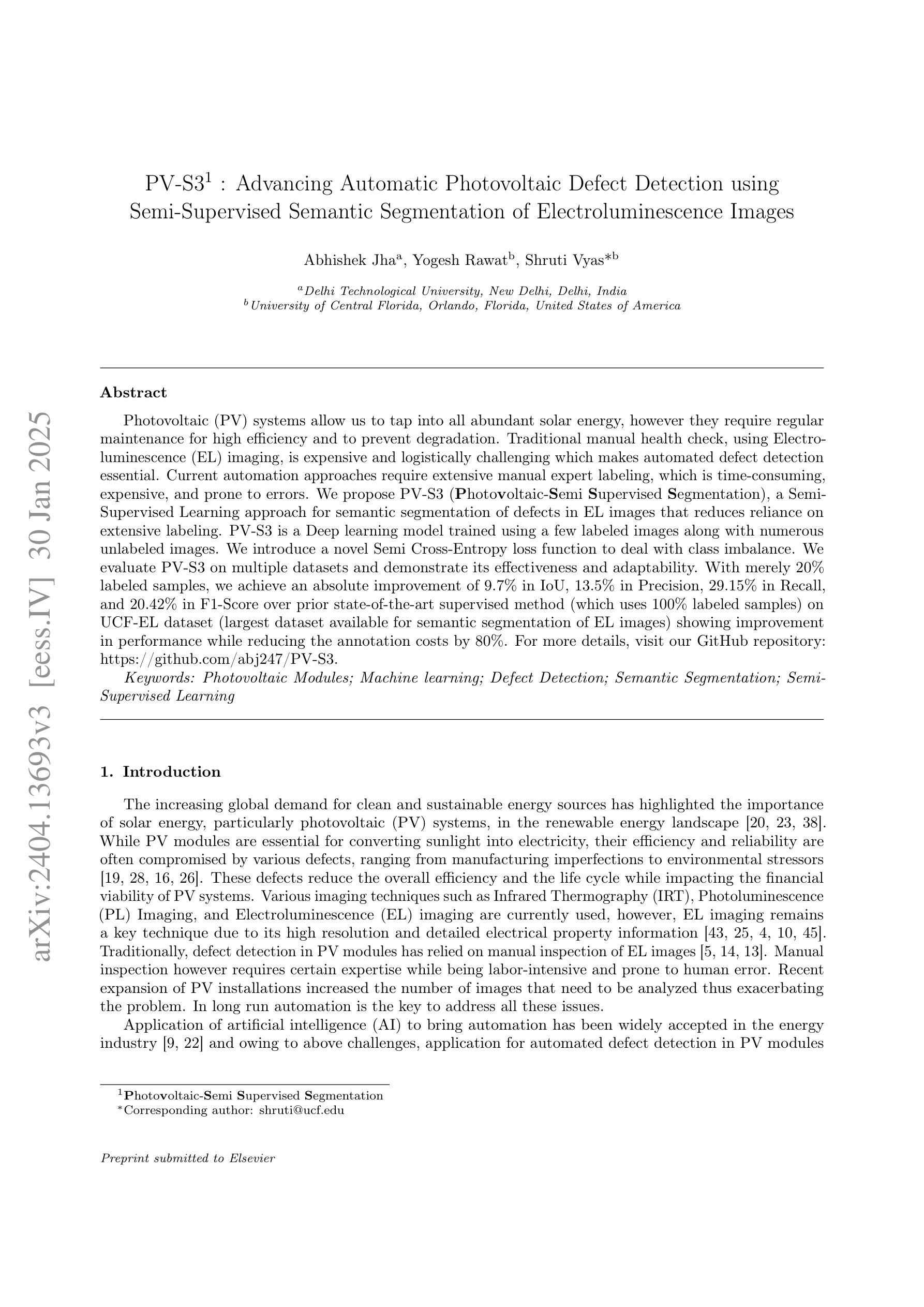
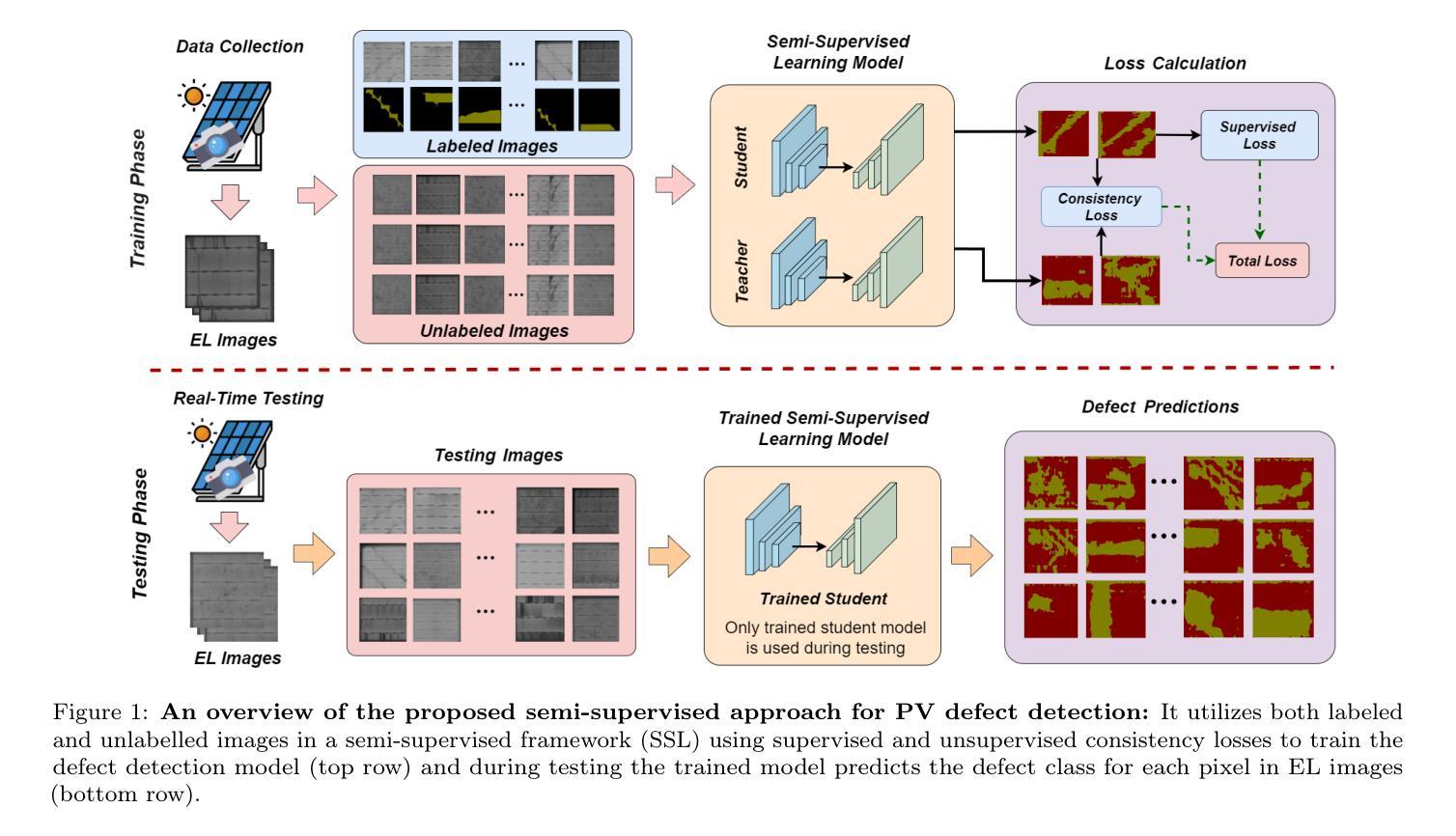
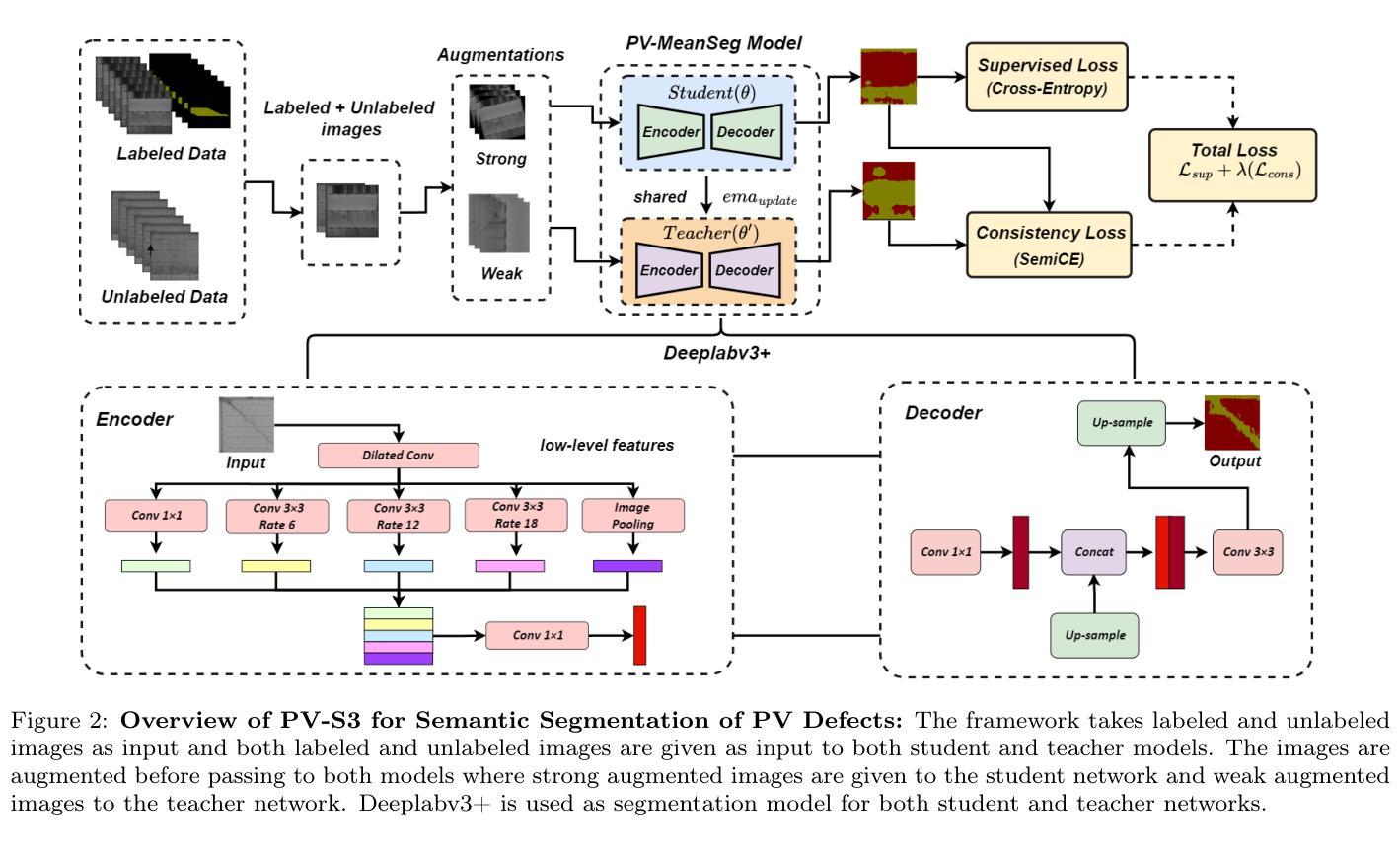
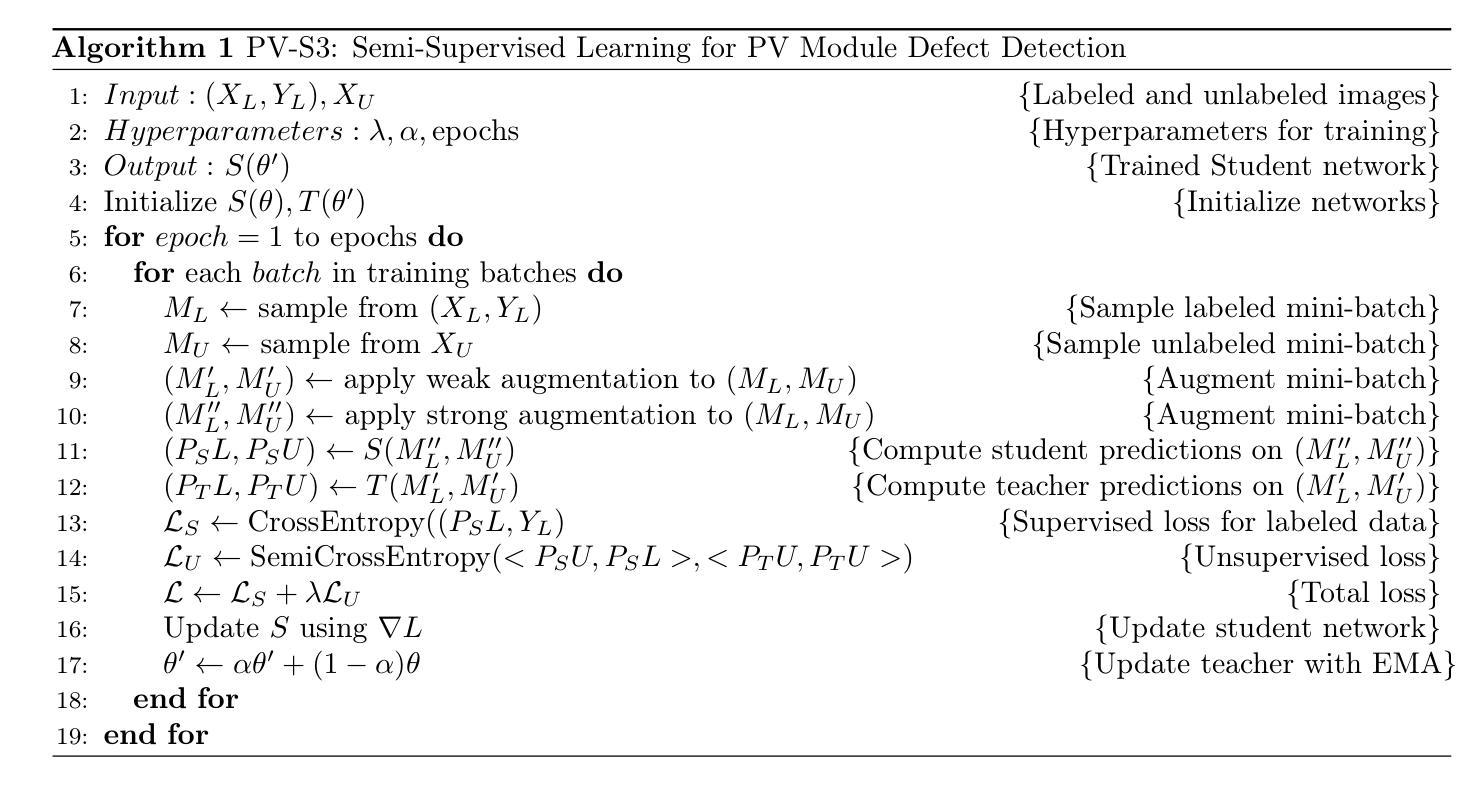