⚠️ 以下所有内容总结都来自于 大语言模型的能力,如有错误,仅供参考,谨慎使用
🔴 请注意:千万不要用于严肃的学术场景,只能用于论文阅读前的初筛!
💗 如果您觉得我们的项目对您有帮助 ChatPaperFree ,还请您给我们一些鼓励!⭐️ HuggingFace免费体验
2025-02-06 更新
MaintaAvatar: A Maintainable Avatar Based on Neural Radiance Fields by Continual Learning
Authors:Shengbo Gu, Yu-Kun Qiu, Yu-Ming Tang, Ancong Wu, Wei-Shi Zheng
The generation of a virtual digital avatar is a crucial research topic in the field of computer vision. Many existing works utilize Neural Radiance Fields (NeRF) to address this issue and have achieved impressive results. However, previous works assume the images of the training person are available and fixed while the appearances and poses of a subject could constantly change and increase in real-world scenarios. How to update the human avatar but also maintain the ability to render the old appearance of the person is a practical challenge. One trivial solution is to combine the existing virtual avatar models based on NeRF with continual learning methods. However, there are some critical issues in this approach: learning new appearances and poses can cause the model to forget past information, which in turn leads to a degradation in the rendering quality of past appearances, especially color bleeding issues, and incorrect human body poses. In this work, we propose a maintainable avatar (MaintaAvatar) based on neural radiance fields by continual learning, which resolves the issues by utilizing a Global-Local Joint Storage Module and a Pose Distillation Module. Overall, our model requires only limited data collection to quickly fine-tune the model while avoiding catastrophic forgetting, thus achieving a maintainable virtual avatar. The experimental results validate the effectiveness of our MaintaAvatar model.
生成虚拟数字角色是计算机视觉领域的重要研究课题。许多现有作品利用神经辐射场(NeRF)来解决这个问题,并取得了令人印象深刻的结果。然而,以前的研究假设训练人员的图像是可用的并且是固定的,而在现实世界的场景中,主体的外观和姿势可能会不断发生变化并增加。如何更新虚拟角色,同时保持渲染人物旧外观的能力是一个实际挑战。一种简单的解决方案是将基于NeRF的现有虚拟角色模型与持续学习方法相结合。然而,这种方法存在一些关键问题:学习新的外观和姿势会导致模型忘记过去的信息,进而降低过去外观的渲染质量,特别是出现色彩混合问题和人体姿势不正确的问题。在这项工作中,我们提出了一种基于神经辐射场的可持续角色(MaintaAvatar),通过持续学习来解决这些问题,利用全局-局部联合存储模块和姿势蒸馏模块来解决这些问题。总的来说,我们的模型只需要有限的数据收集就能快速微调模型,同时避免灾难性遗忘,从而实现可持续的虚拟角色。实验结果验证了我们的MaintaAvatar模型的有效性。
论文及项目相关链接
PDF AAAI 2025. 9 pages
Summary
本研究探讨了利用神经辐射场(NeRF)技术生成虚拟数字头像的重要性,并提出了一种基于持续学习的可维护头像(MaintaAvatar)模型。该模型通过全局局部联合存储模块和姿态蒸馏模块解决了学习新外观和姿态时遗忘过去信息的问题,提高了虚拟头像的渲染质量和姿态准确性。研究结果表明,该模型在有限的数据采集下可以快速微调,避免了灾难性遗忘,实现了可维护的虚拟头像。
Key Takeaways
- 虚拟数字头像生成是计算机视觉领域的重要研究话题。
- 现有研究多利用神经辐射场(NeRF)技术来处理该问题,并取得显著成果。
- 现实世界中,人的外观和姿态会不断变化,如何更新虚拟头像并保持其渲染能力是实际挑战。
- 现有结合NeRF的虚拟头像模型与持续学习方法存在关键挑战,如新信息学习导致的过去信息遗忘、渲染质量下降及色彩溢出等问题。
- MaintaAvatar模型通过全局局部联合存储模块和姿态蒸馏模块解决了上述问题。
- MaintaAvatar模型在有限数据采集下可以快速微调,避免了灾难性遗忘。
点此查看论文截图
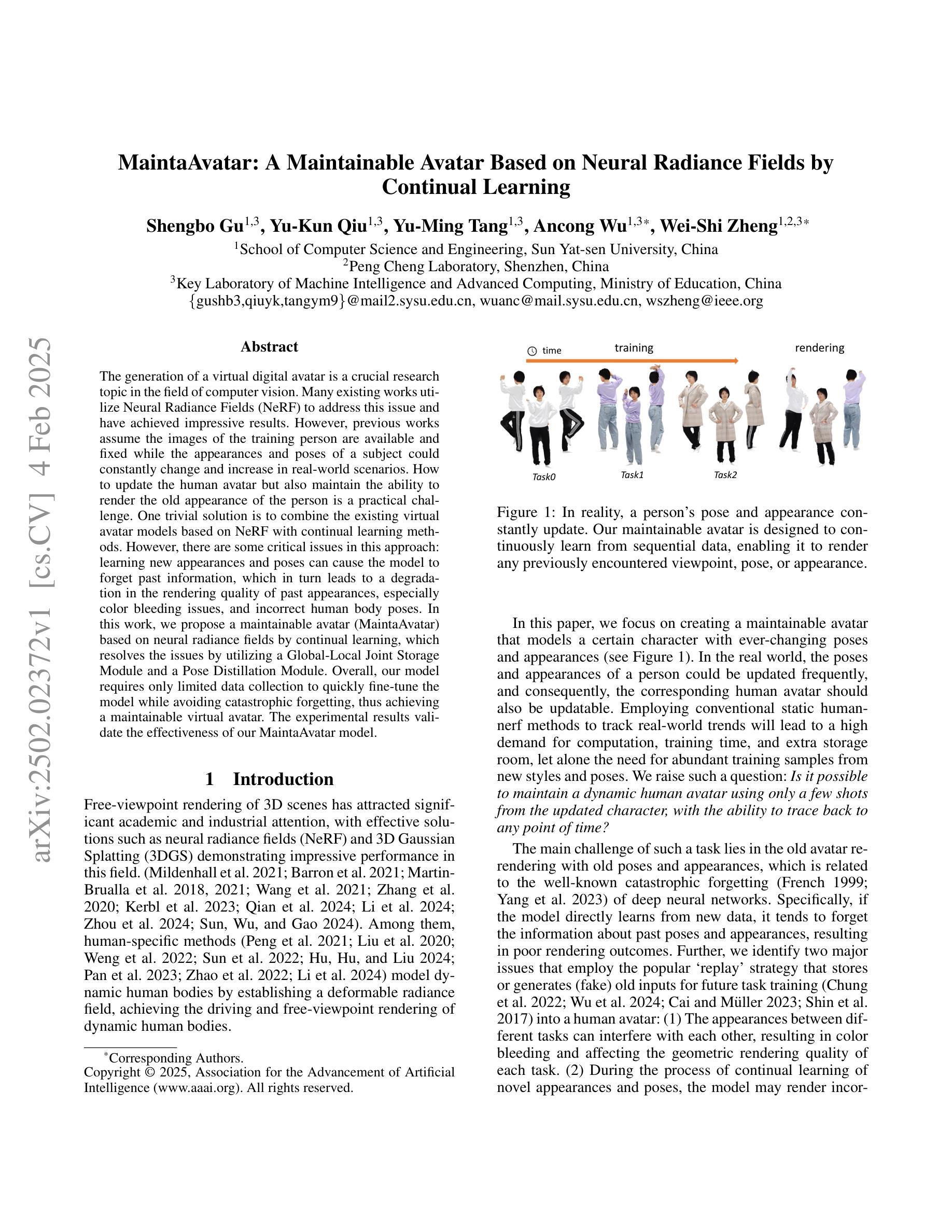
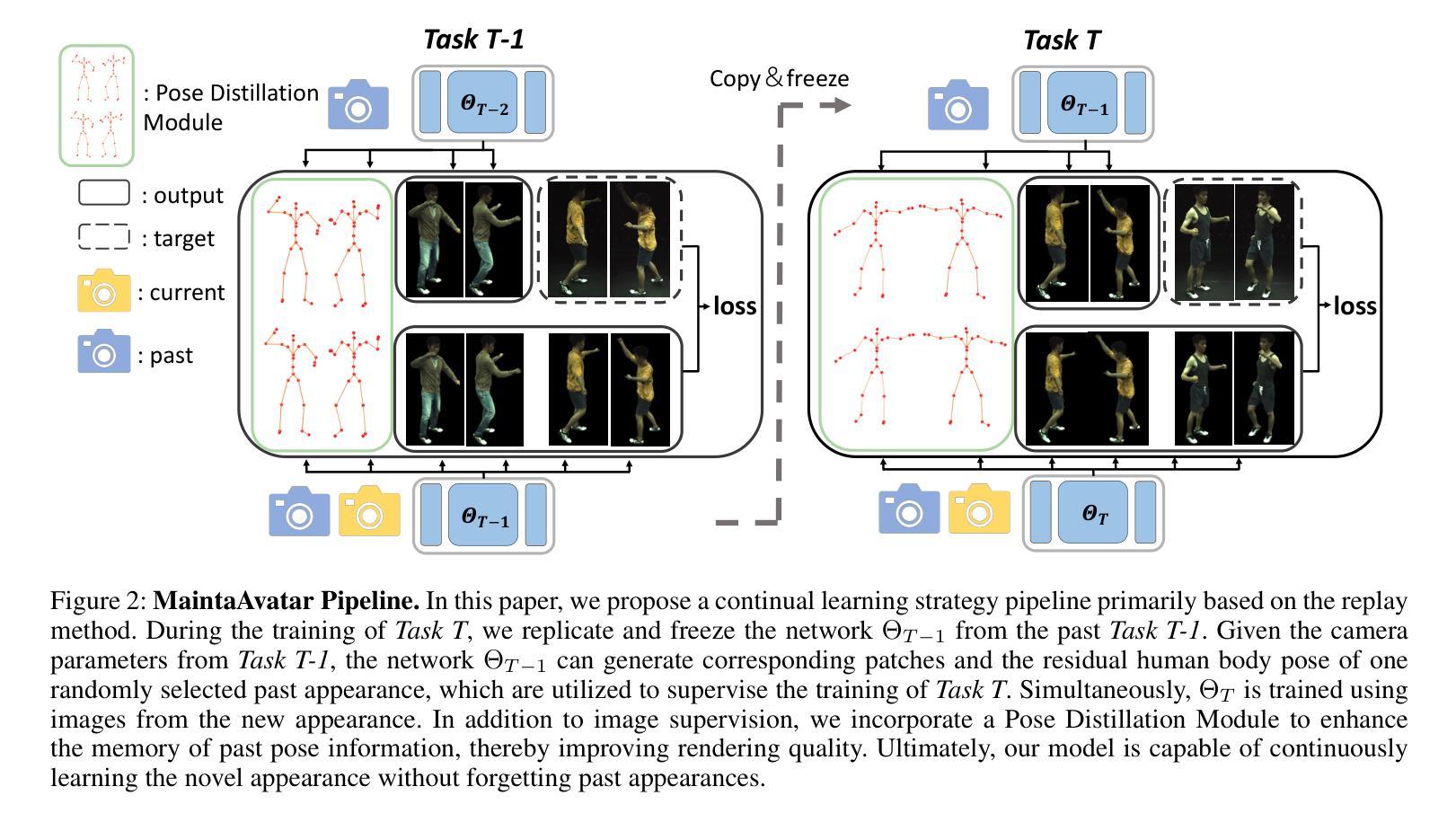
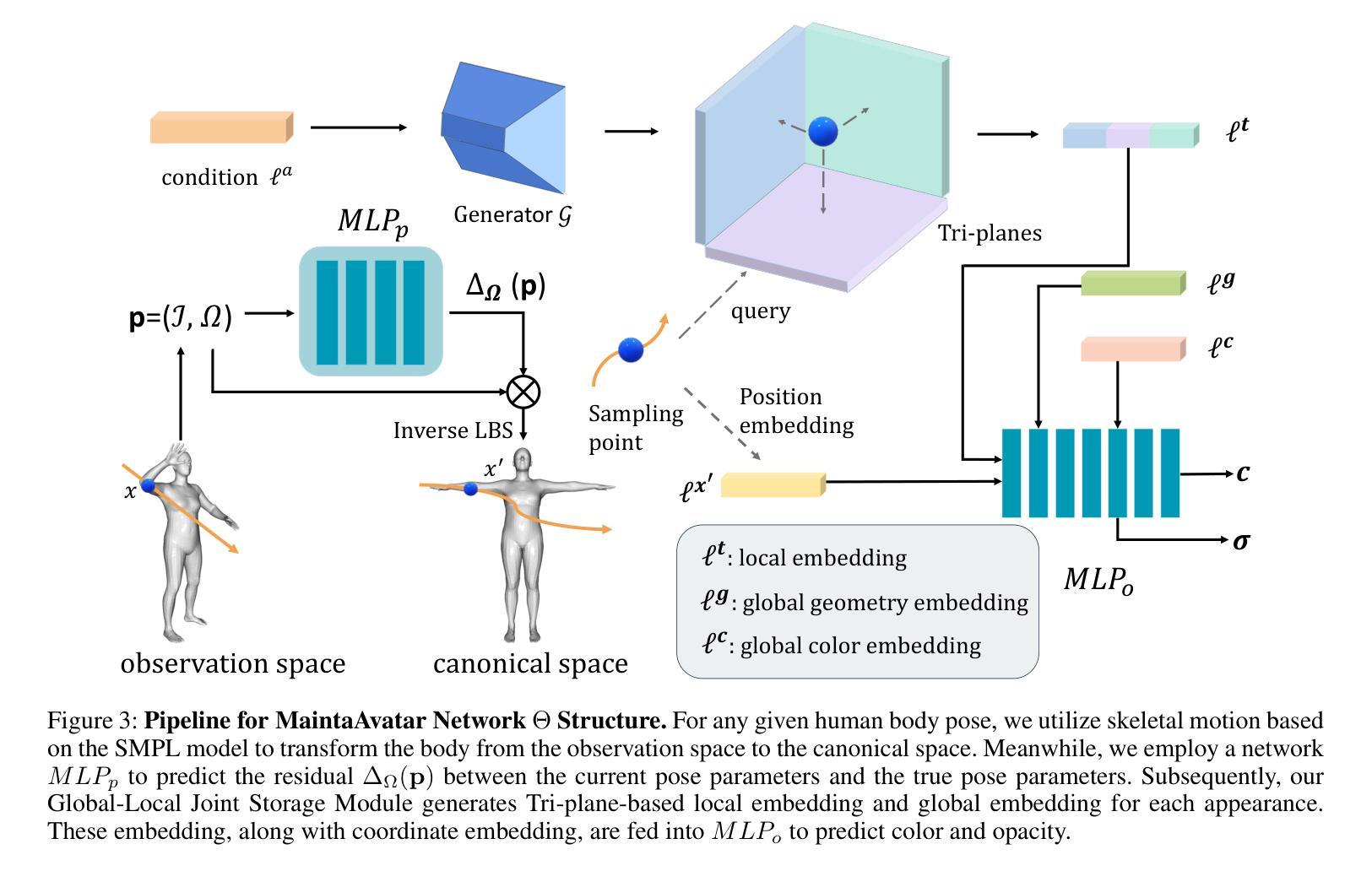
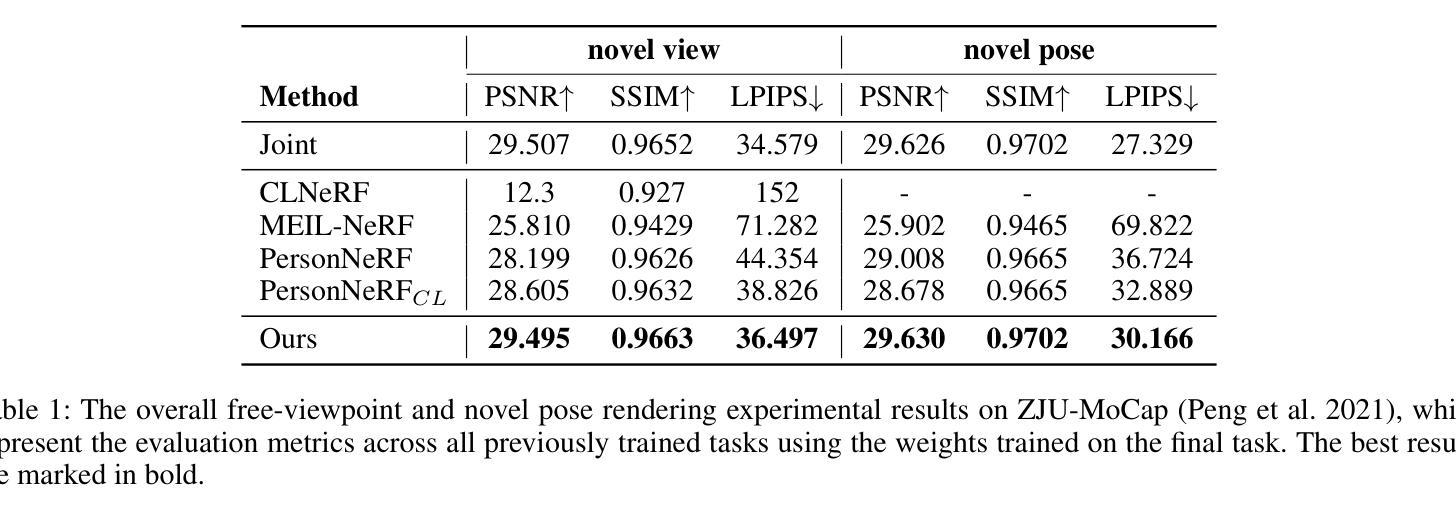
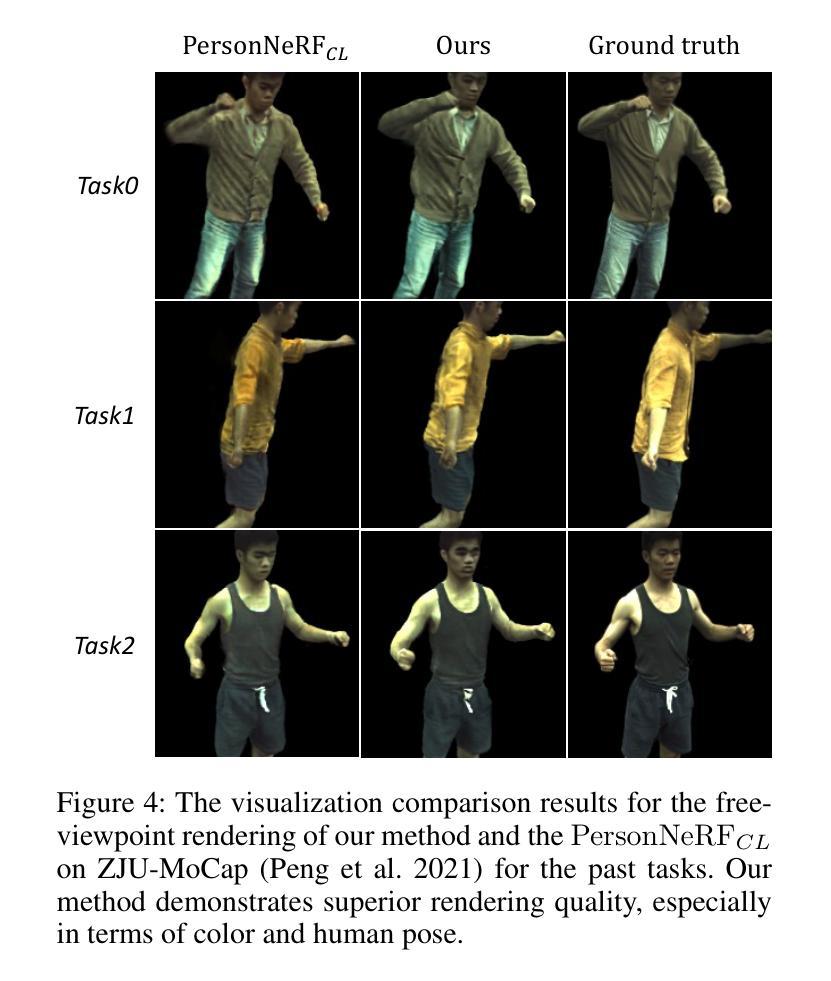
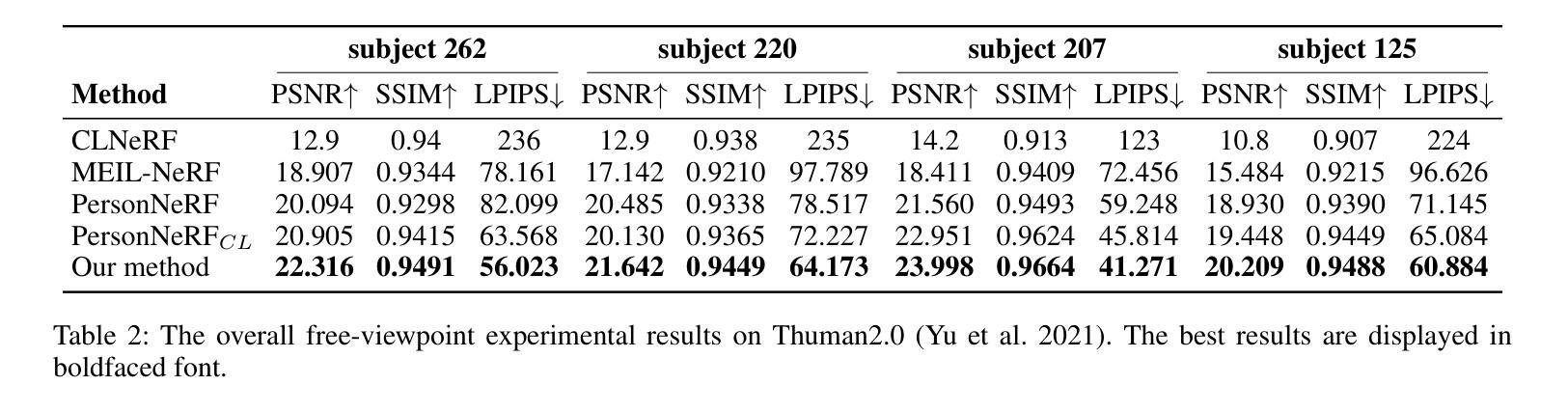