⚠️ 以下所有内容总结都来自于 大语言模型的能力,如有错误,仅供参考,谨慎使用
🔴 请注意:千万不要用于严肃的学术场景,只能用于论文阅读前的初筛!
💗 如果您觉得我们的项目对您有帮助 ChatPaperFree ,还请您给我们一些鼓励!⭐️ HuggingFace免费体验
2025-02-06 更新
A Self-Supervised Framework for Improved Generalisability in Ultrasound B-mode Image Segmentation
Authors:Edward Ellis, Andrew Bulpitt, Nasim Parsa, Michael F Byrne, Sharib Ali
Ultrasound (US) imaging is clinically invaluable due to its noninvasive and safe nature. However, interpreting US images is challenging, requires significant expertise, and time, and is often prone to errors. Deep learning offers assistive solutions such as segmentation. Supervised methods rely on large, high-quality, and consistently labeled datasets, which are challenging to curate. Moreover, these methods tend to underperform on out-of-distribution data, limiting their clinical utility. Self-supervised learning (SSL) has emerged as a promising alternative, leveraging unlabeled data to enhance model performance and generalisability. We introduce a contrastive SSL approach tailored for B-mode US images, incorporating a novel Relation Contrastive Loss (RCL). RCL encourages learning of distinct features by differentiating positive and negative sample pairs through a learnable metric. Additionally, we propose spatial and frequency-based augmentation strategies for the representation learning on US images. Our approach significantly outperforms traditional supervised segmentation methods across three public breast US datasets, particularly in data-limited scenarios. Notable improvements on the Dice similarity metric include a 4% increase on 20% and 50% of the BUSI dataset, nearly 6% and 9% improvements on 20% and 50% of the BrEaST dataset, and 6.4% and 3.7% improvements on 20% and 50% of the UDIAT dataset, respectively. Furthermore, we demonstrate superior generalisability on the out-of-distribution UDIAT dataset with performance boosts of 20.6% and 13.6% compared to the supervised baseline using 20% and 50% of the BUSI and BrEaST training data, respectively. Our research highlights that domain-inspired SSL can improve US segmentation, especially under data-limited conditions.
超声(US)成像因其无创且安全的特点而在临床上具有非常重要的价值。然而,解读超声图像具有挑战性,需要丰富的专业知识和大量的时间,并且容易出错。深度学习提供了辅助解决方案,例如分割技术。监督学习方法依赖于大量高质量且标签一致的数据集,这些数据集的整理具有挑战性。此外,这些方法在超出分布范围的数据上表现往往不佳,从而限制了其临床应用价值。自监督学习(SSL)已成为一种有前景的替代方法,它利用未标记的数据来提高模型的性能和泛化能力。我们针对B模式超声图像引入了一种对比自监督学习方法,并结合了一种新型的关系对比损失(RCL)。RCL通过可学习的度量标准来区分正负样本对,从而鼓励学习独特特征。此外,我们还提出了基于空间和频率的增强策略,用于超声图像的表示学习。我们的方法在三组公开乳腺超声数据集中显著优于传统的监督分割方法,特别是在数据有限的情况下。在Dice相似度指标上的显著改进包括在BUSI数据集20%和50%的数据上分别提高了4%和近6%;在BrEaST数据集20%和50%的数据上分别提高了近6%和近9%;在UDIAT数据集上分别提高了6.4%和近3.7%。此外,我们在分布外的UDIAT数据集上展示了出色的泛化能力,与使用BUSI和BrEaST训练数据集的监督基线相比,性能分别提高了近20.6%和近13.6%。我们的研究表明,领域启发式的自监督学习能够改善超声分割技术,特别是在数据有限的情况下。
论文及项目相关链接
PDF 12
摘要
超声成像因其无创且安全的特点而具有极高的临床价值。然而,解读超声图像具有挑战性,需要大量专业知识与时间,并容易出错。深度学习提供了解辅助解决方案,如分割技术。然而,监督学习方法依赖于大量高质量且持续标注的数据集,这很难获取。此外,这些方法在超出分布范围的数据上表现不佳,限制了其临床效用。自监督学习(SSL)作为一种有前途的替代方案崭露头角,利用无标签数据提高模型性能和泛化能力。我们提出了一种针对B模式超声图像的对比自监督学习方法,并结合了一种新型关系对比损失(RCL)。RCL通过区分正负样本对,鼓励学习独特特征,从而提高模型性能。此外,我们提出了基于空间和频率的增强策略,用于超声图像上的表示学习。我们的方法在三组公开乳腺超声数据集上的表现显著优于传统的监督分割方法,特别是在数据有限的情况下。在BUSI数据集上,使用20%和50%的数据时,Dice相似度指标分别提高了4%和近6%;在BrEaST数据集上分别提高了近6%和近9%;在UDIAT数据集上也观察到相似提升趋势。此外,在跨数据集验证中表现出优秀的泛化性能:相比于仅在BUSI和BrEaST数据集上训练的监督模型,使用其训练数据的20%和50%,在UDIAT测试集上的性能分别提升了约20.6%和约13.6%。本研究强调,基于领域的自监督学习能够改善超声图像分割效果,特别是在数据有限的情况下。
关键见解
- 超声成像具有无创性和安全性等显著优势,但其图像解读具有挑战性。
- 深度学习技术(如分割)在临床影像领域具有广泛应用前景。
- 监督学习方法受限于数据质量和标注难度问题以及模型在新数据上的泛化能力问题。
- 自监督学习通过利用无标签数据提高了模型性能和泛化能力。
- 提出了一种结合对比自监督学习和关系对比损失(RCL)的方法来处理B模式超声图像,有效提高模型性能。
- 所提出的方法在不同乳腺超声数据集上的表现优于传统监督方法,特别是在数据有限的情况下表现尤为突出。
点此查看论文截图
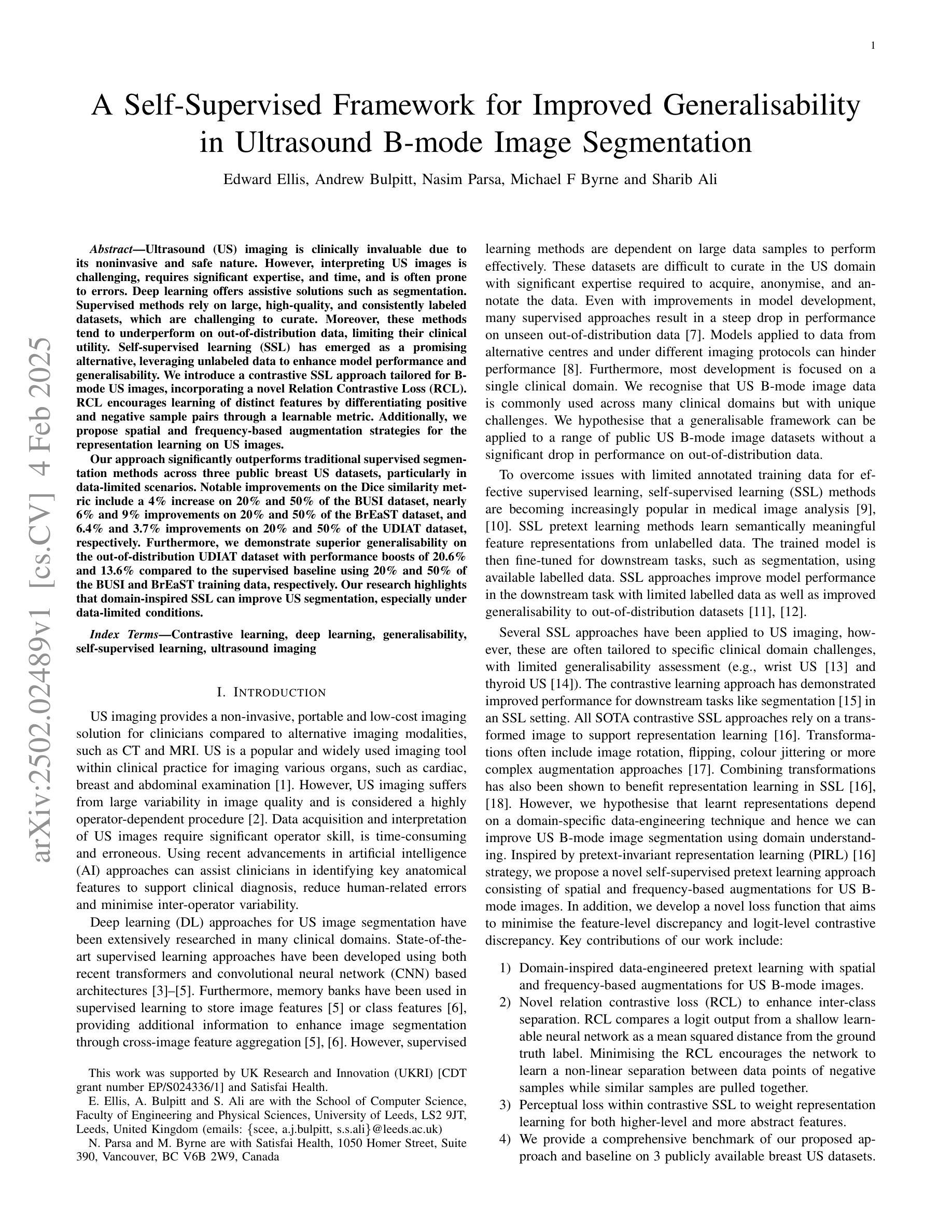
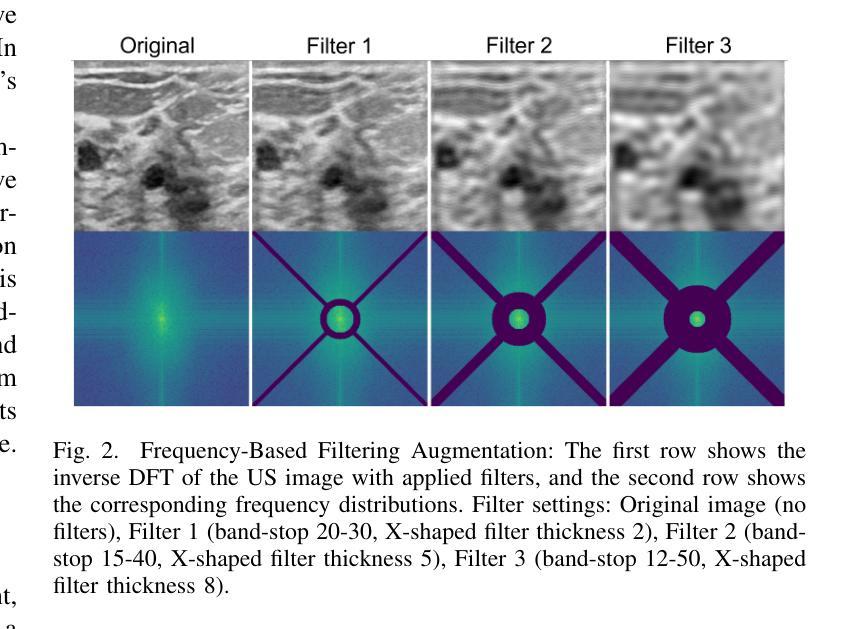
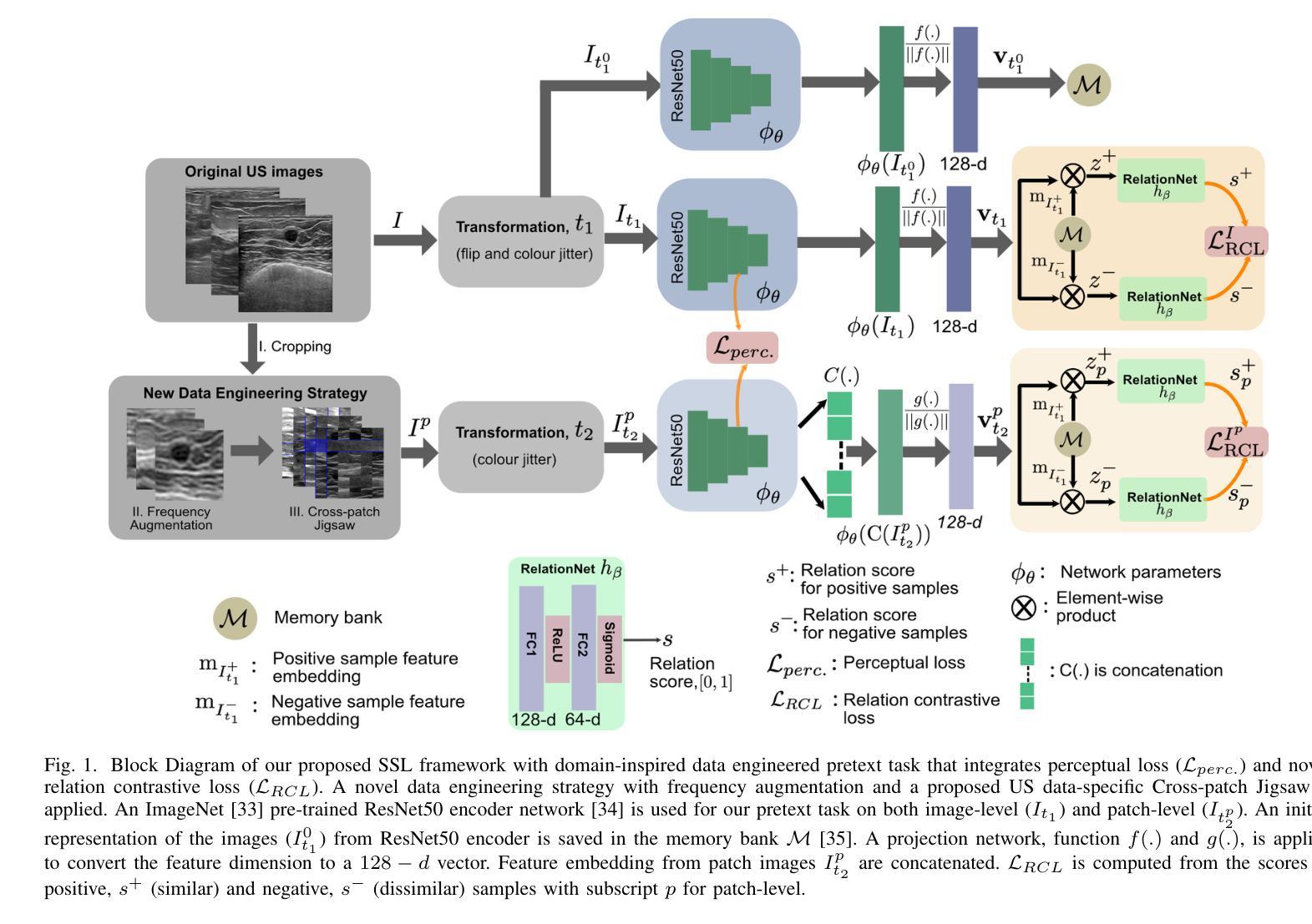
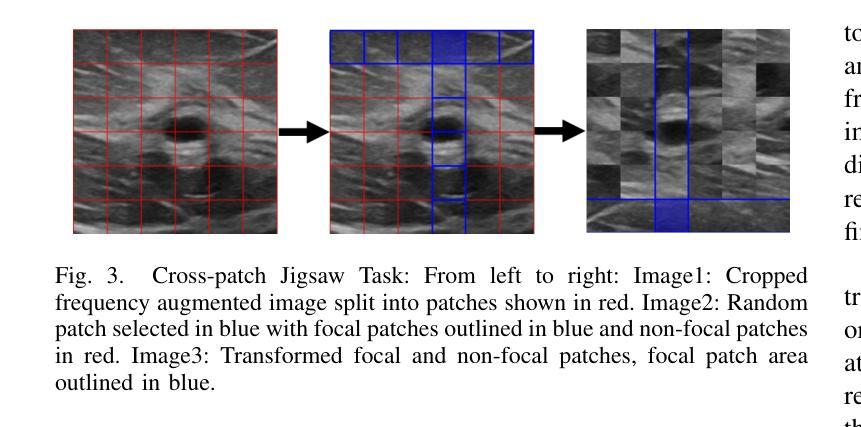
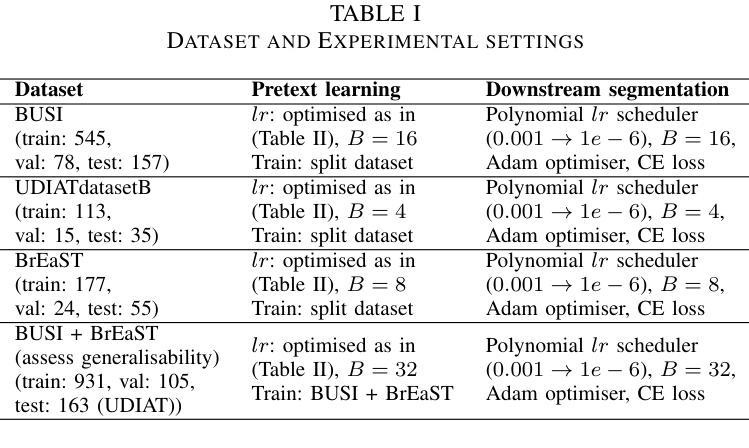
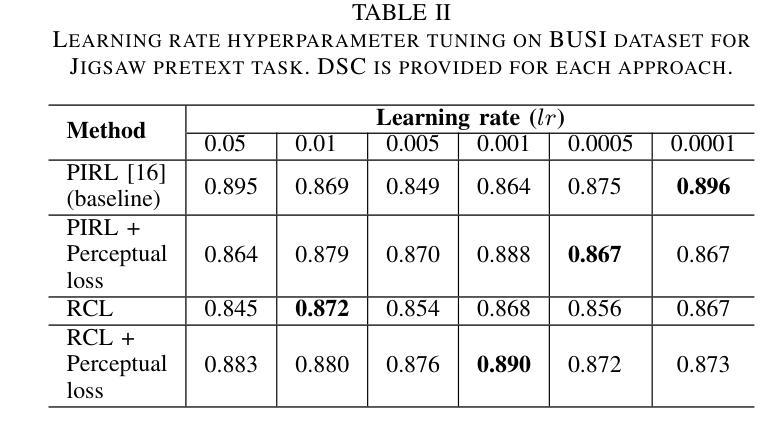