⚠️ 以下所有内容总结都来自于 大语言模型的能力,如有错误,仅供参考,谨慎使用
🔴 请注意:千万不要用于严肃的学术场景,只能用于论文阅读前的初筛!
💗 如果您觉得我们的项目对您有帮助 ChatPaperFree ,还请您给我们一些鼓励!⭐️ HuggingFace免费体验
2025-02-06 更新
AAD-DCE: An Aggregated Multimodal Attention Mechanism for Early and Late Dynamic Contrast Enhanced Prostate MRI Synthesis
Authors:Divya Bharti, Sriprabha Ramanarayanan, Sadhana S, Kishore Kumar M, Keerthi Ram, Harsh Agarwal, Ramesh Venkatesan, Mohanasankar Sivaprakasam
Dynamic Contrast-Enhanced Magnetic Resonance Imaging (DCE-MRI) is a medical imaging technique that plays a crucial role in the detailed visualization and identification of tissue perfusion in abnormal lesions and radiological suggestions for biopsy. However, DCE-MRI involves the administration of a Gadolinium based (Gad) contrast agent, which is associated with a risk of toxicity in the body. Previous deep learning approaches that synthesize DCE-MR images employ unimodal non-contrast or low-dose contrast MRI images lacking focus on the local perfusion information within the anatomy of interest. We propose AAD-DCE, a generative adversarial network (GAN) with an aggregated attention discriminator module consisting of global and local discriminators. The discriminators provide a spatial embedded attention map to drive the generator to synthesize early and late response DCE-MRI images. Our method employs multimodal inputs - T2 weighted (T2W), Apparent Diffusion Coefficient (ADC), and T1 pre-contrast for image synthesis. Extensive comparative and ablation studies on the ProstateX dataset show that our model (i) is agnostic to various generator benchmarks and (ii) outperforms other DCE-MRI synthesis approaches with improvement margins of +0.64 dB PSNR, +0.0518 SSIM, -0.015 MAE for early response and +0.1 dB PSNR, +0.0424 SSIM, -0.021 MAE for late response, and (ii) emphasize the importance of attention ensembling. Our code is available at https://github.com/bhartidivya/AAD-DCE.
动态对比增强磁共振成像(DCE-MRI)是一种医学成像技术,在异常病变的详细可视化和组织灌注识别中发挥着关键作用,并为活检提供放射学建议。然而,DCE-MRI需要注射基于钆(Gad)的造影剂,这增加了体内毒性风险。先前合成DCE-MR图像的深度学习方法是使用非对比或低剂量对比MRI图像的单模态方法,并不专注于感兴趣解剖结构内的局部灌注信息。我们提出了AAD-DCE,这是一种生成对抗网络(GAN),包含一个聚合注意力判别器模块,它由全局和局部判别器组成。判别器提供空间嵌入注意力图,以驱动生成器合成早期和晚期响应DCE-MRI图像。我们的方法采用多模态输入,包括T2加权(T2W)、表观扩散系数(ADC)和T1预对比用于图像合成。在ProstateX数据集上进行的大量比较和消融研究表明:(i)我们的模型对各种生成器基准测试不敏感;(ii)与其他DCE-MRI合成方法相比,我们的模型在早反应和晚反应方面分别提高了+0.64 dB PSNR,+ 0.0518 SSIM,- 0.015 MAE和+ 0.1 dB PSNR,+ 0.0424 SSIM,- 0.021 MAE;(iii)强调了注意力集成的重要性。我们的代码可在https://github.com/bhartidivya/AAD-DCE上找到。
论文及项目相关链接
Summary
本研究提出了AAD-DCE模型,该模型使用生成对抗网络(GAN)进行动态对比增强磁共振成像(DCE-MRI)合成。采用聚集注意力判别器模块,包含全局和局部判别器,可提供空间嵌入注意力图,引导生成器合成早期和晚期响应DCE-MRI图像。采用多模态输入(T2加权、表观扩散系数和T1预对比),在前列腺数据集上的广泛对比和消融研究表明,该模型对生成器基准测试具有不敏感性,且在早期和晚期响应的DCE-MRI合成方面优于其他方法,强调了注意力集成的重要性。
Key Takeaways
- AAD-DCE模型利用GAN进行DCE-MRI图像合成。
- 聚集注意力判别器模块包含全局和局部判别器,提供空间嵌入注意力图。
- 多模态输入(T2加权、表观扩散系数和T1预对比)用于图像合成。
- 在前列腺数据集上的研究表明,该模型对生成器基准测试具有不敏感性。
- 与其他DCE-MRI合成方法相比,AAD-DCE模型在PSNR、SSIM和MAE等评价指标上表现更优。
点此查看论文截图
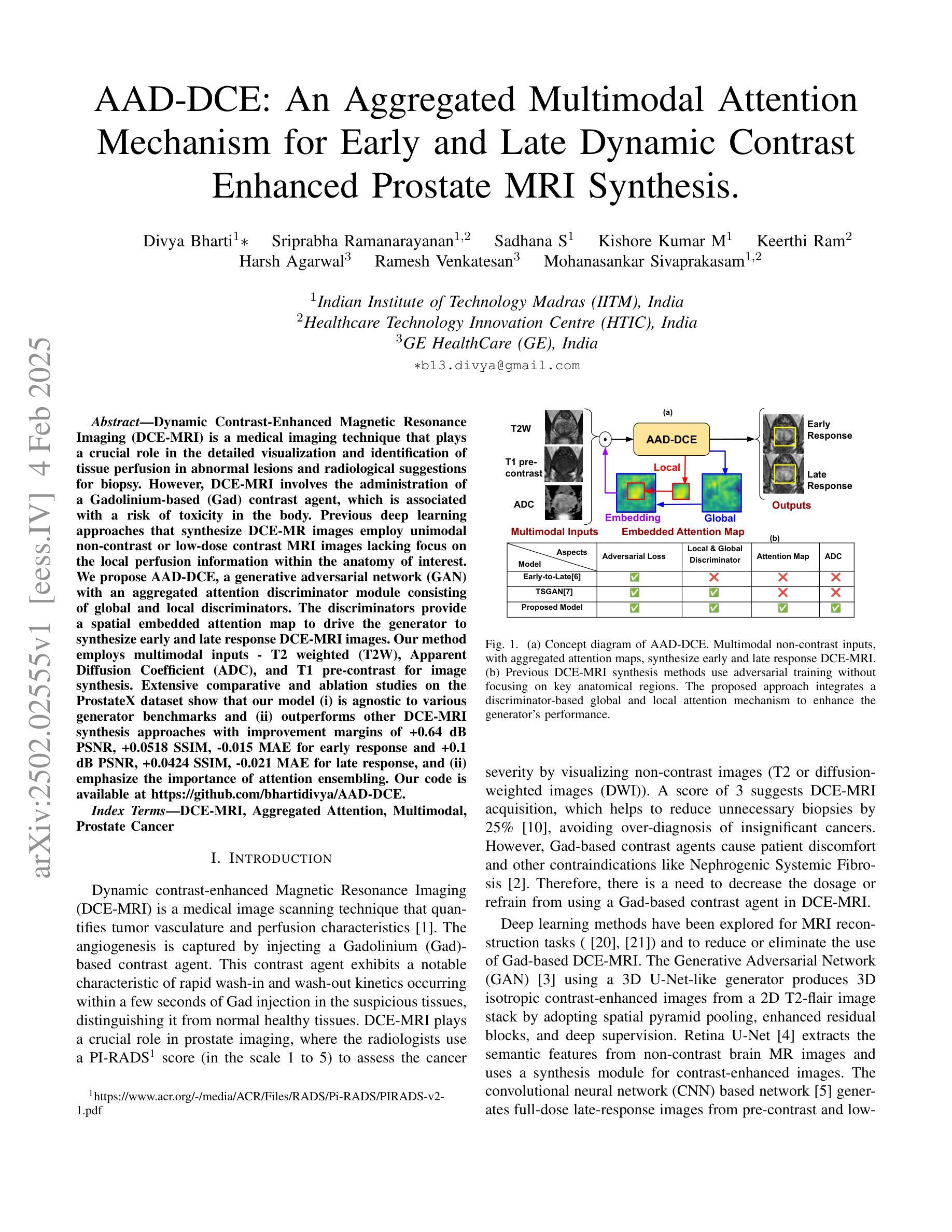
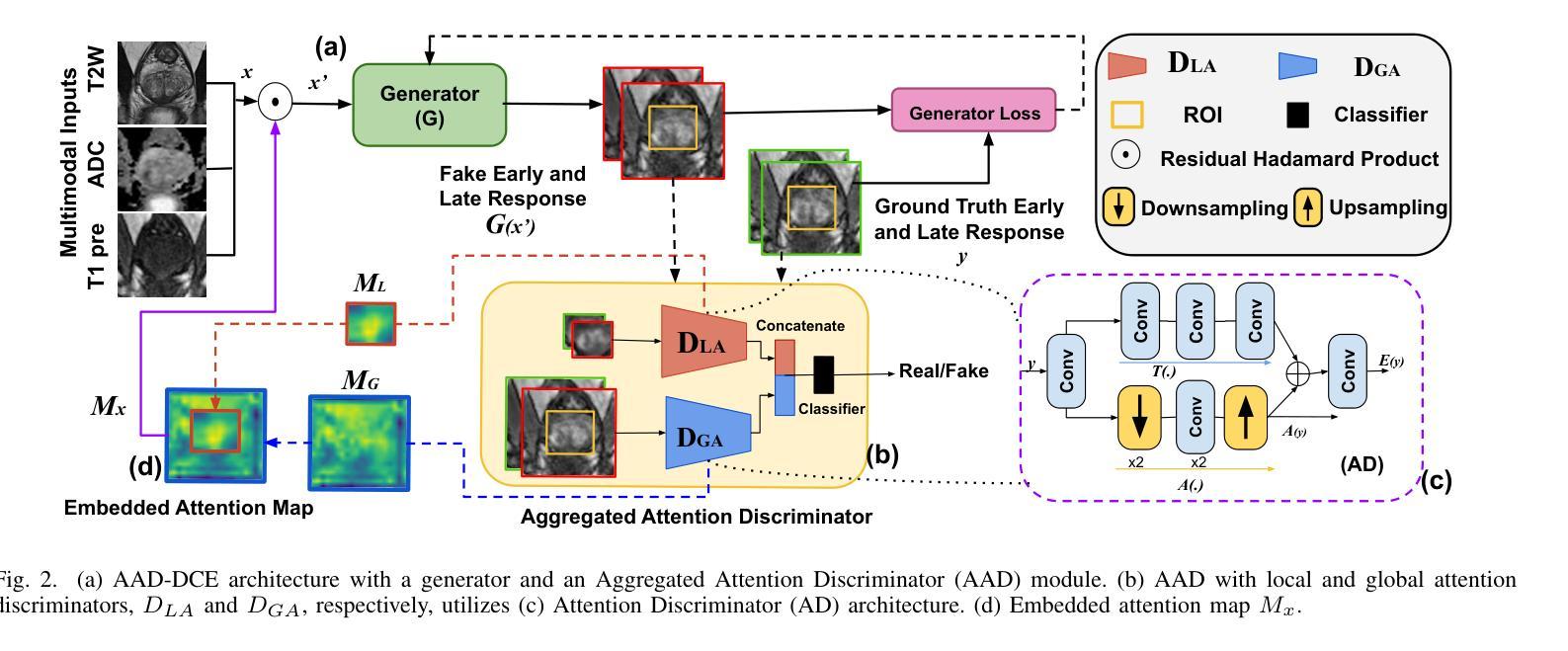
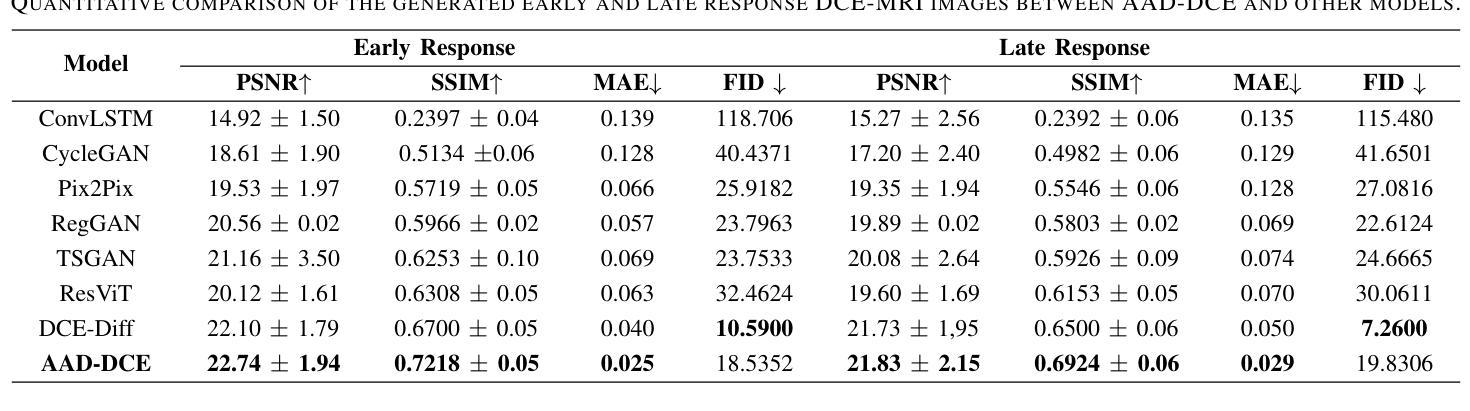
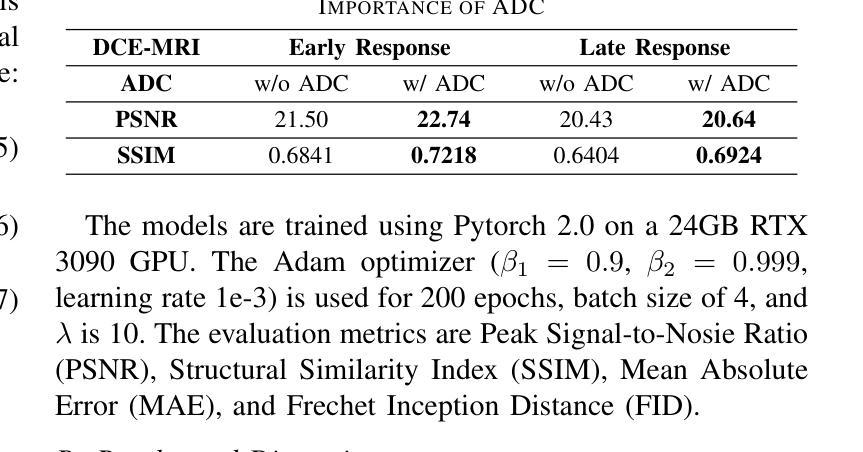
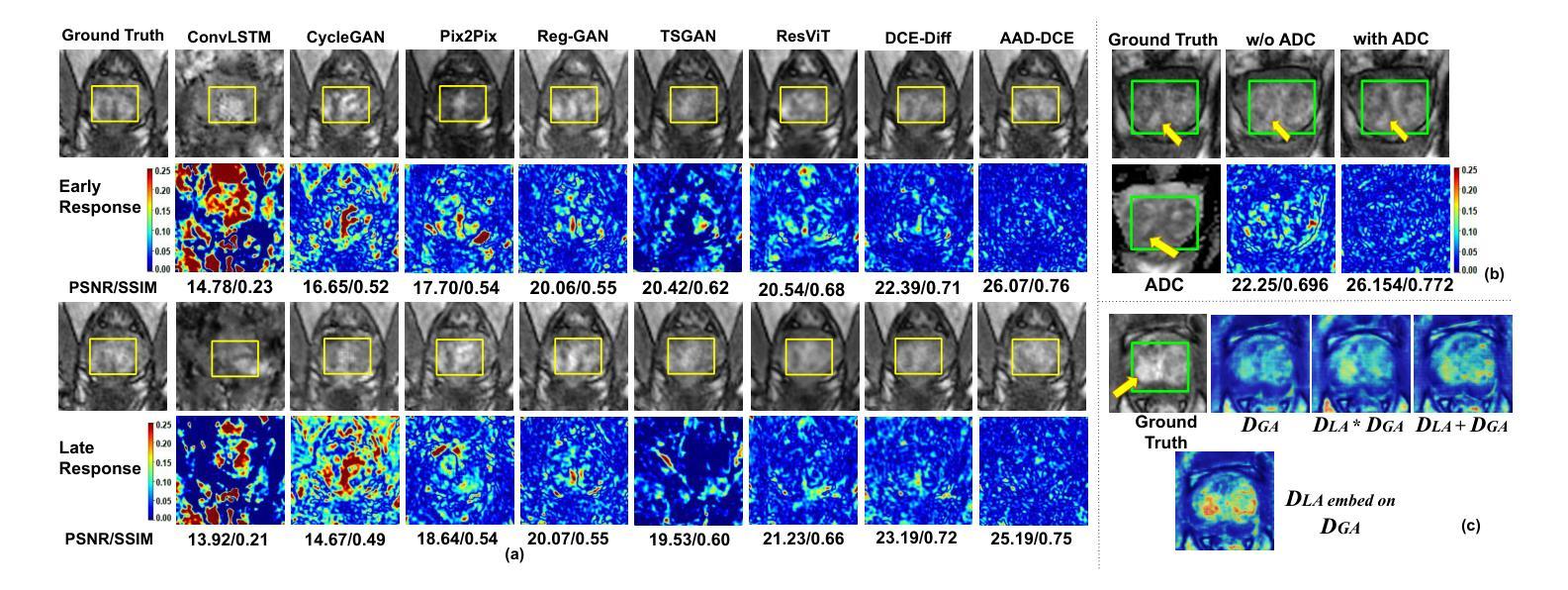
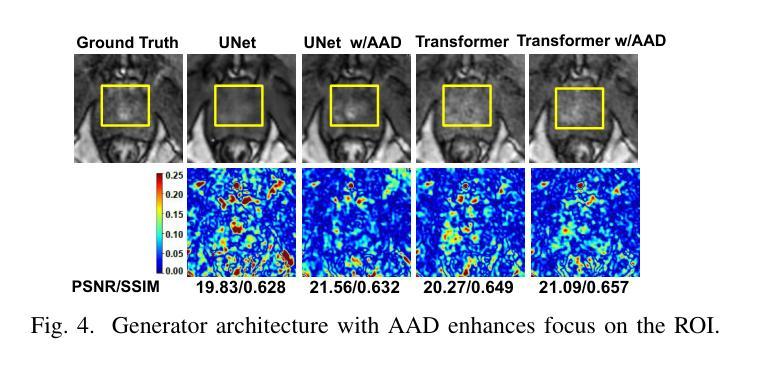
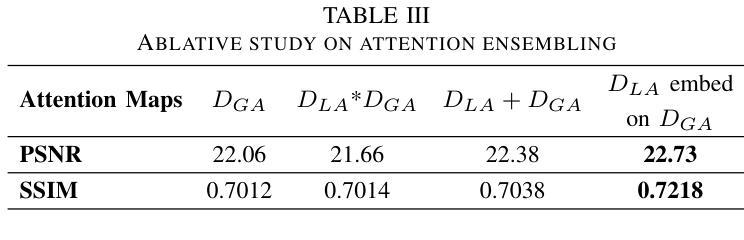
Multi-level Supervised Contrastive Learning
Authors:Naghmeh Ghanooni, Barbod Pajoum, Harshit Rawal, Sophie Fellenz, Vo Nguyen Le Duy, Marius Kloft
Contrastive learning is a well-established paradigm in representation learning. The standard framework of contrastive learning minimizes the distance between “similar” instances and maximizes the distance between dissimilar ones in the projection space, disregarding the various aspects of similarity that can exist between two samples. Current methods rely on a single projection head, which fails to capture the full complexity of different aspects of a sample, leading to suboptimal performance, especially in scenarios with limited training data. In this paper, we present a novel supervised contrastive learning method in a unified framework called multilevel contrastive learning (MLCL), that can be applied to both multi-label and hierarchical classification tasks. The key strength of the proposed method is the ability to capture similarities between samples across different labels and/or hierarchies using multiple projection heads. Extensive experiments on text and image datasets demonstrate that the proposed approach outperforms state-of-the-art contrastive learning methods
对比学习是表征学习中的一个成熟范式。对比学习的标准框架旨在最小化“相似”实例之间的距离,并最大化不同实例在投影空间之间的距离,同时忽略两个样本之间可能存在的各种相似性方面。当前的方法依赖于单个投影头,无法捕捉样本不同方面的全部复杂性,导致性能不佳,特别是在训练数据有限的情况下。在本文中,我们提出了一种新型的有监督对比学习方法,在一个统一框架中称为多层次对比学习(MLCL),可应用于多标签和层次分类任务。所提方法的关键优势在于能够使用多个投影头捕捉不同标签和/或层次之间的样本相似性。在文本和图像数据集上的大量实验表明,该方法优于最新的对比学习方法。
论文及项目相关链接
Summary
本文介绍了一种名为多层次对比学习(MLCL)的新型有监督对比学习方法,该方法可以在多标签和层次分类任务中表现出色。其关键优势在于能够利用多个投影头捕捉不同标签和/或层次之间的样本相似性,从而提高对比学习的性能,特别是在训练数据有限的情况下。实验证明,该方法在文本和图像数据集上的表现优于最新的对比学习方法。
Key Takeaways
- 对比学习是表示学习中的成熟范式。
- 传统对比学习方法使用单一投影头,无法捕捉样本的复杂性和不同方面的相似性。
- 提出的多层次对比学习方法(MLCL)能够捕捉不同标签和/或层次间的样本相似性。
- MLCL方法通过多个投影头实现,适用于多标签和层次分类任务。
- 实验证明,MLCL在文本和图像数据集上的性能优于其他先进的对比学习方法。
- MLCL方法能够提高对比学习的性能,特别是在训练数据有限的情况下。
点此查看论文截图
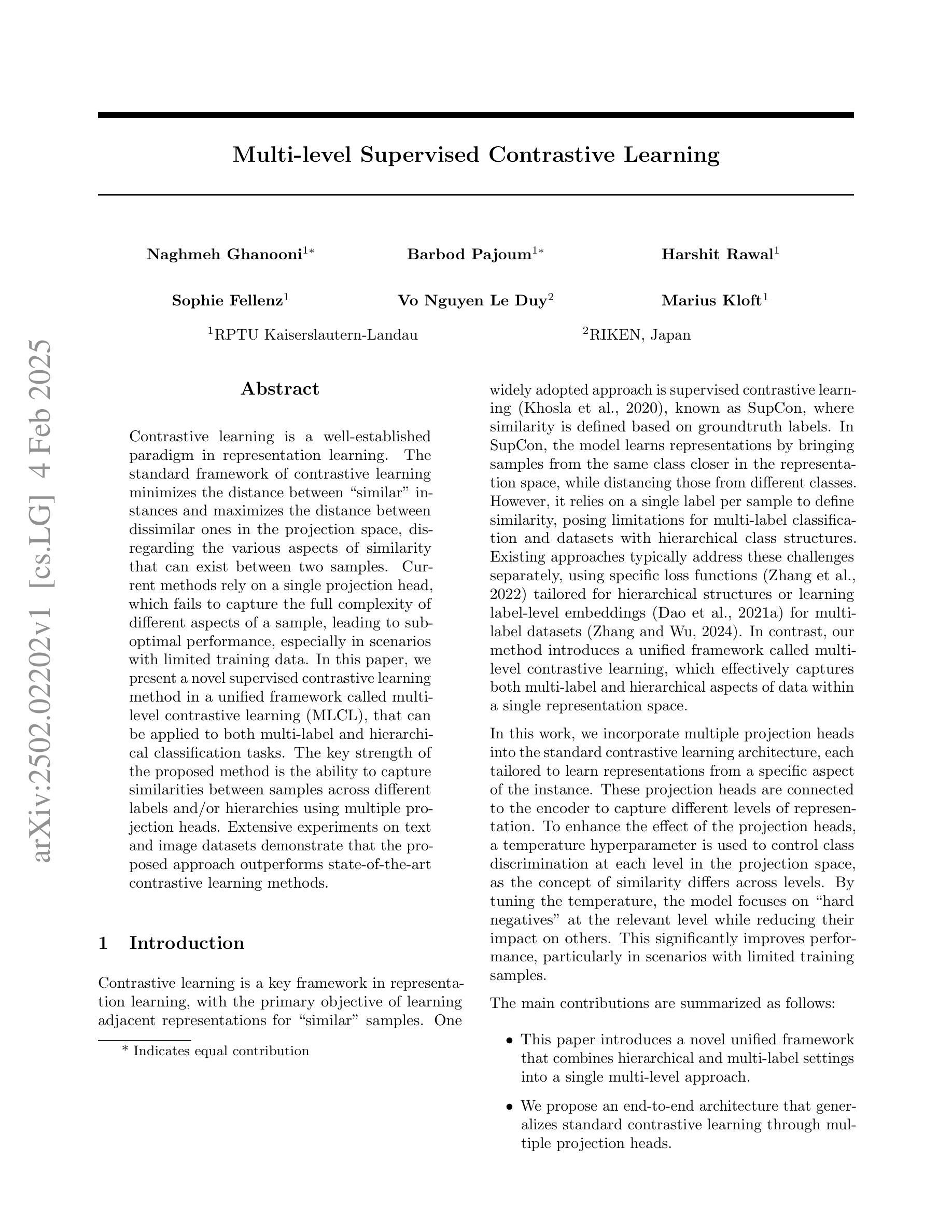
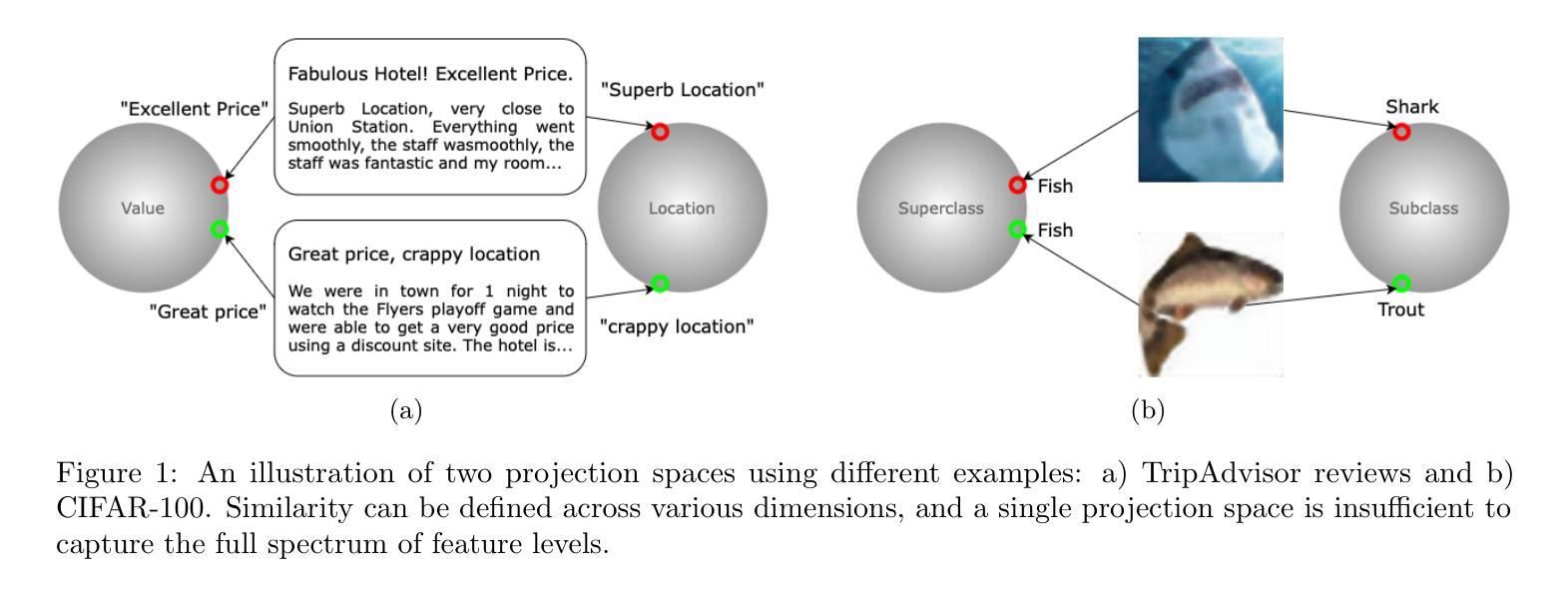
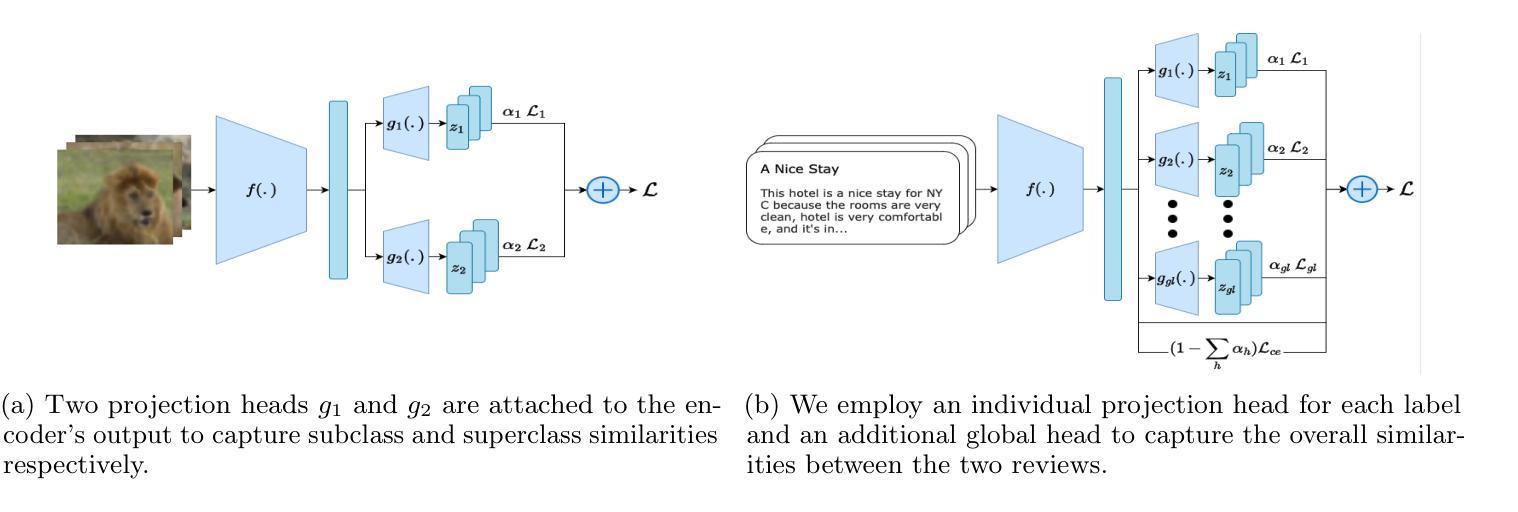
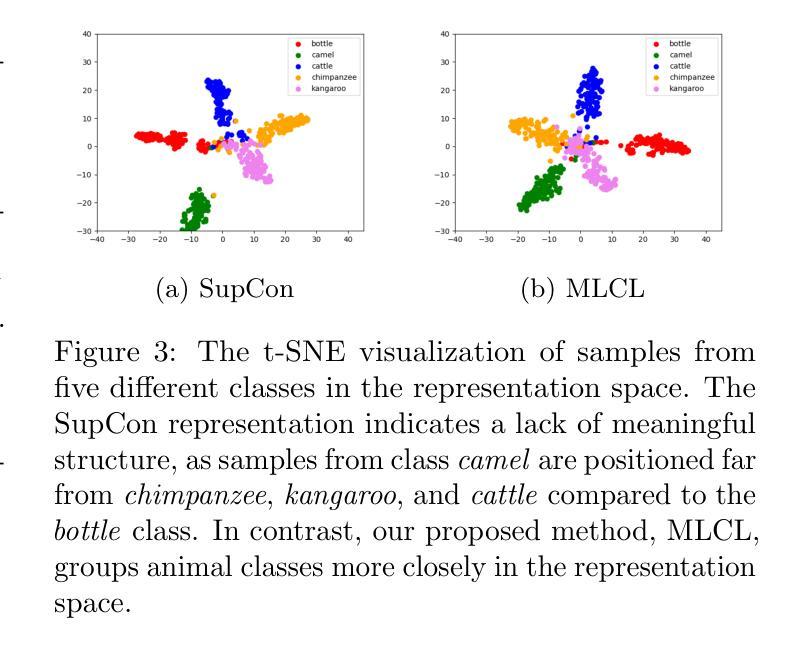
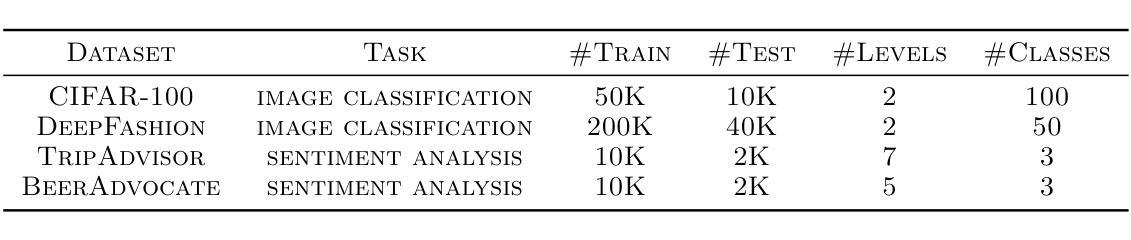
Detecting Backdoor Samples in Contrastive Language Image Pretraining
Authors:Hanxun Huang, Sarah Erfani, Yige Li, Xingjun Ma, James Bailey
Contrastive language-image pretraining (CLIP) has been found to be vulnerable to poisoning backdoor attacks where the adversary can achieve an almost perfect attack success rate on CLIP models by poisoning only 0.01% of the training dataset. This raises security concerns on the current practice of pretraining large-scale models on unscrutinized web data using CLIP. In this work, we analyze the representations of backdoor-poisoned samples learned by CLIP models and find that they exhibit unique characteristics in their local subspace, i.e., their local neighborhoods are far more sparse than that of clean samples. Based on this finding, we conduct a systematic study on detecting CLIP backdoor attacks and show that these attacks can be easily and efficiently detected by traditional density ratio-based local outlier detectors, whereas existing backdoor sample detection methods fail. Our experiments also reveal that an unintentional backdoor already exists in the original CC3M dataset and has been trained into a popular open-source model released by OpenCLIP. Based on our detector, one can clean up a million-scale web dataset (e.g., CC3M) efficiently within 15 minutes using 4 Nvidia A100 GPUs. The code is publicly available in our \href{https://github.com/HanxunH/Detect-CLIP-Backdoor-Samples}{GitHub repository}.
对比语言图像预训练(CLIP)容易受到后门攻击的影响,攻击者只需对训练数据集进行0.01%的毒化,便可在CLIP模型上实现近乎完美的攻击成功率。这引发了人们对当前在未经严格审查的网页数据上使用CLIP预训练大规模模型的实践的担忧。在这项工作中,我们分析了被CLIP模型学习到的后门毒化样本的表示,发现它们在局部子空间具有独特特征,即它们的局部邻域比清洁样本更为稀疏。基于这一发现,我们对检测CLIP后门攻击进行了系统研究,并表明这些攻击可以通过传统的基于密度比的局部异常检测器轻松有效地检测出来,而现有的后门样本检测方法则失败了。我们的实验还表明,原始CC3M数据集中已经存在无意中植入的后门,并已训练成OpenCLIP发布的流行开源模型。基于我们的检测器,使用4个Nvidia A100 GPU,可以在15分钟内高效清理百万级别的网页数据集(例如CC3M)。代码已在我们位于https://github.com/HanxunH/Detect-CLIP-Backdoor-Samples的GitHub仓库中公开可用。
论文及项目相关链接
PDF ICLR2025
Summary
本文揭示了对比语言图像预训练模型(CLIP)存在的安全隐患,即只需对训练数据集进行极少量的(0.01%)后门攻击即可达到几乎完美的攻击成功率。通过分析受后门攻击影响的样本在CLIP模型中的表现特征,发现它们具有独特的局部子空间特征,即其局部邻域比清洁样本更为稀疏。研究结果表明,这类攻击可通过传统的基于密度比的局部异常检测器轻松有效地检测出来,而现有的后门样本检测方法则无法识别。实验还显示,原始CC3M数据集中已存在无意中的后门,并已植入一个流行的开源模型中。利用检测器,可在短时间内高效清理大规模网络数据集(如CC3M)。
Key Takeaways
- CLIP模型面临后门攻击风险,攻击者只需对极少量数据进行后门注入即可实现近乎完美的攻击效果。
- 受后门影响的CLIP模型样本在局部子空间表现出独特特征,其邻域比正常样本更为稀疏。
- 传统基于密度比的局部异常检测器能有效检测CLIP模型中的后门攻击。
- 现有后门样本检测方法在识别CLIP模型中的后门攻击时效果不佳。
- 原始CC3M数据集中存在无意中的后门,并已影响某些开源模型。
- 使用检测器能高效清理大规模网络数据集,如CC3M。
点此查看论文截图
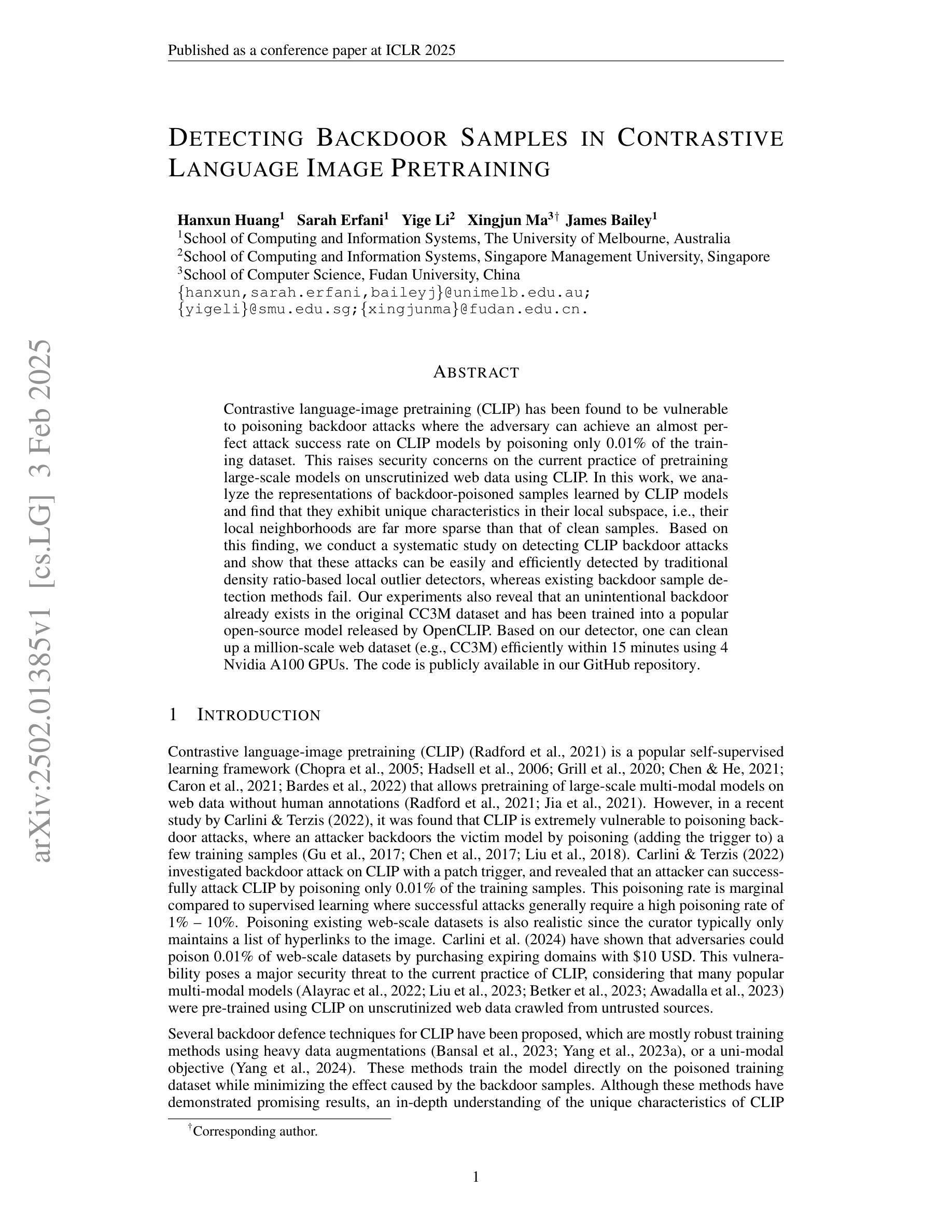
RealRAG: Retrieval-augmented Realistic Image Generation via Self-reflective Contrastive Learning
Authors:Yuanhuiyi Lyu, Xu Zheng, Lutao Jiang, Yibo Yan, Xin Zou, Huiyu Zhou, Linfeng Zhang, Xuming Hu
Recent text-to-image generative models, e.g., Stable Diffusion V3 and Flux, have achieved notable progress. However, these models are strongly restricted to their limited knowledge, a.k.a., their own fixed parameters, that are trained with closed datasets. This leads to significant hallucinations or distortions when facing fine-grained and unseen novel real-world objects, e.g., the appearance of the Tesla Cybertruck. To this end, we present the first real-object-based retrieval-augmented generation framework (RealRAG), which augments fine-grained and unseen novel object generation by learning and retrieving real-world images to overcome the knowledge gaps of generative models. Specifically, to integrate missing memory for unseen novel object generation, we train a reflective retriever by self-reflective contrastive learning, which injects the generator’s knowledge into the sef-reflective negatives, ensuring that the retrieved augmented images compensate for the model’s missing knowledge. Furthermore, the real-object-based framework integrates fine-grained visual knowledge for the generative models, tackling the distortion problem and improving the realism for fine-grained object generation. Our Real-RAG is superior in its modular application to all types of state-of-the-art text-to-image generative models and also delivers remarkable performance boosts with all of them, such as a gain of 16.18% FID score with the auto-regressive model on the Stanford Car benchmark.
最近出现的文本到图像生成模型,如Stable Diffusion V3和Flux,已经取得了显著的进步。然而,这些模型受限于其有限的知识(即使用封闭数据集训练的固定参数)。这导致在面对细粒度和未见的新现实世界物体(例如特斯拉的赛博卡车)时,会出现明显的幻觉或失真。为此,我们首次提出了基于真实物体的检索增强生成框架(RealRAG)。它通过学习和检索真实世界的图像来增强细粒度和未见的新物体生成,以克服生成模型的知识空白。具体来说,为了整合未见新物体生成的缺失记忆,我们通过自我反射对比学习训练了一个反射检索器,将生成器的知识注入到自我反射的负样本中,确保检索到的增强图像能够补偿模型的缺失知识。此外,基于真实物体的框架为生成模型融入了细粒度视觉知识,解决了失真问题,提高了细粒度物体生成的逼真度。我们的RealRAG在模块化应用于所有最先进的文本到图像生成模型时表现出卓越的性能,并与它们一起实现了显著的性能提升。例如,在斯坦福汽车基准测试上,与自回归模型相比,我们的模型FID得分提高了16.18%。
论文及项目相关链接
Summary
本文介绍了新的文本到图像生成模型RealRAG,该模型通过学习和检索真实世界图像来增强对精细未见物体的生成能力,以克服生成模型的知识空白。RealRAG采用自我反思对比学习训练反射检索器,将生成器的知识注入自我反思负样本,确保检索到的增强图像能够补偿模型的缺失知识。此外,RealRAG整合了精细视觉知识,解决了生成模型的失真问题,提高了精细物体生成的逼真度。RealRAG可模块化应用于各种先进的文本到图像生成模型,并与其显著提升了性能,如在Stanford Car基准测试上,与自回归模型相比,FID得分提高了16.18%。
Key Takeaways
- RealRAG是一种基于真实物体的检索增强生成框架,旨在解决文本到图像生成模型在面对精细未见物体时的知识空白问题。
- 通过自我反思对比学习,RealRAG训练了一个反射检索器,将生成器的知识注入自我反思负样本,以提高生成模型的性能。
- RealRAG整合了精细视觉知识,提高了生成模型的逼真度,并解决了失真问题。
- RealRAG可模块化应用于各种先进的文本到图像生成模型,并显著提升其性能。
- RealRAG在Stanford Car基准测试上取得了显著的性能提升,与自回归模型相比,FID得分提高了16.18%。
- RealRAG的主要优势在于其能够利用真实世界图像来增强生成模型的能力,使其更能够反映真实世界中的细节和特征。
点此查看论文截图
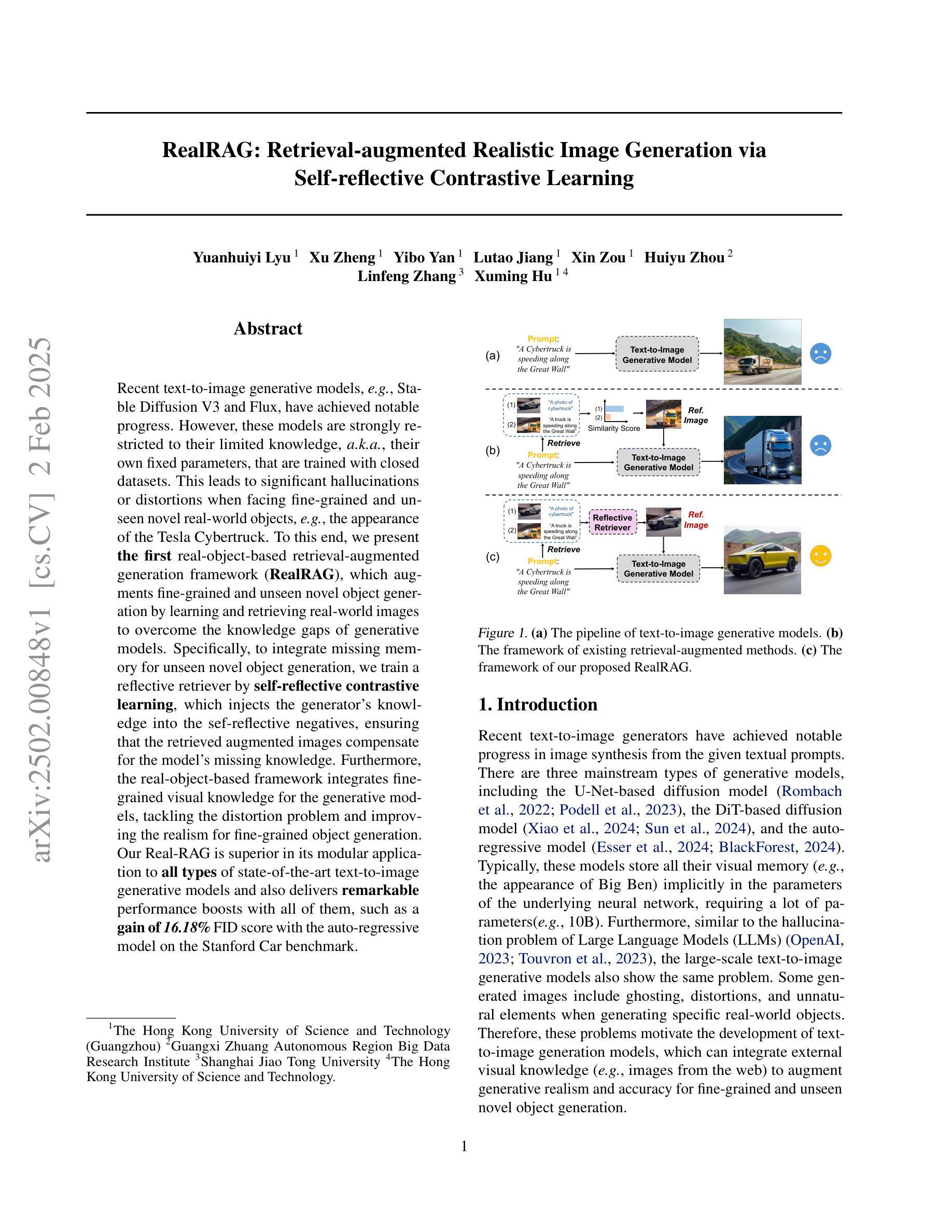
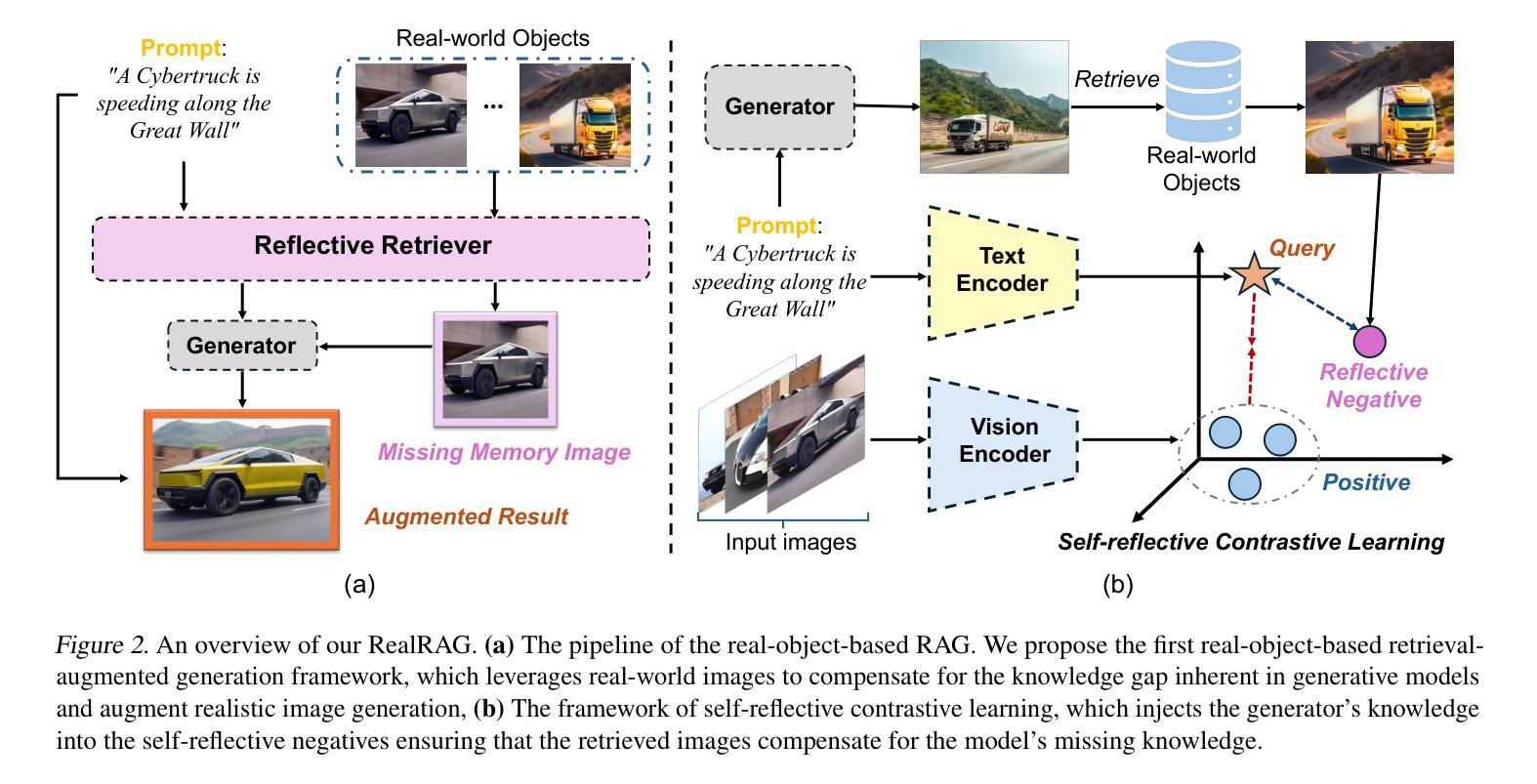
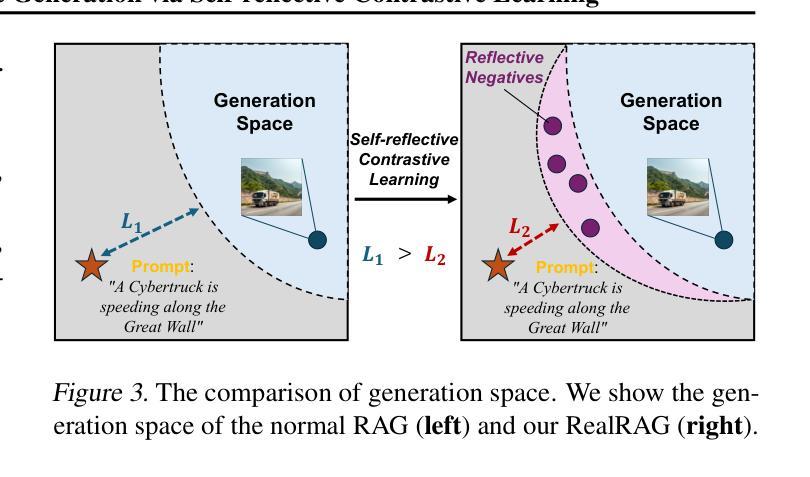
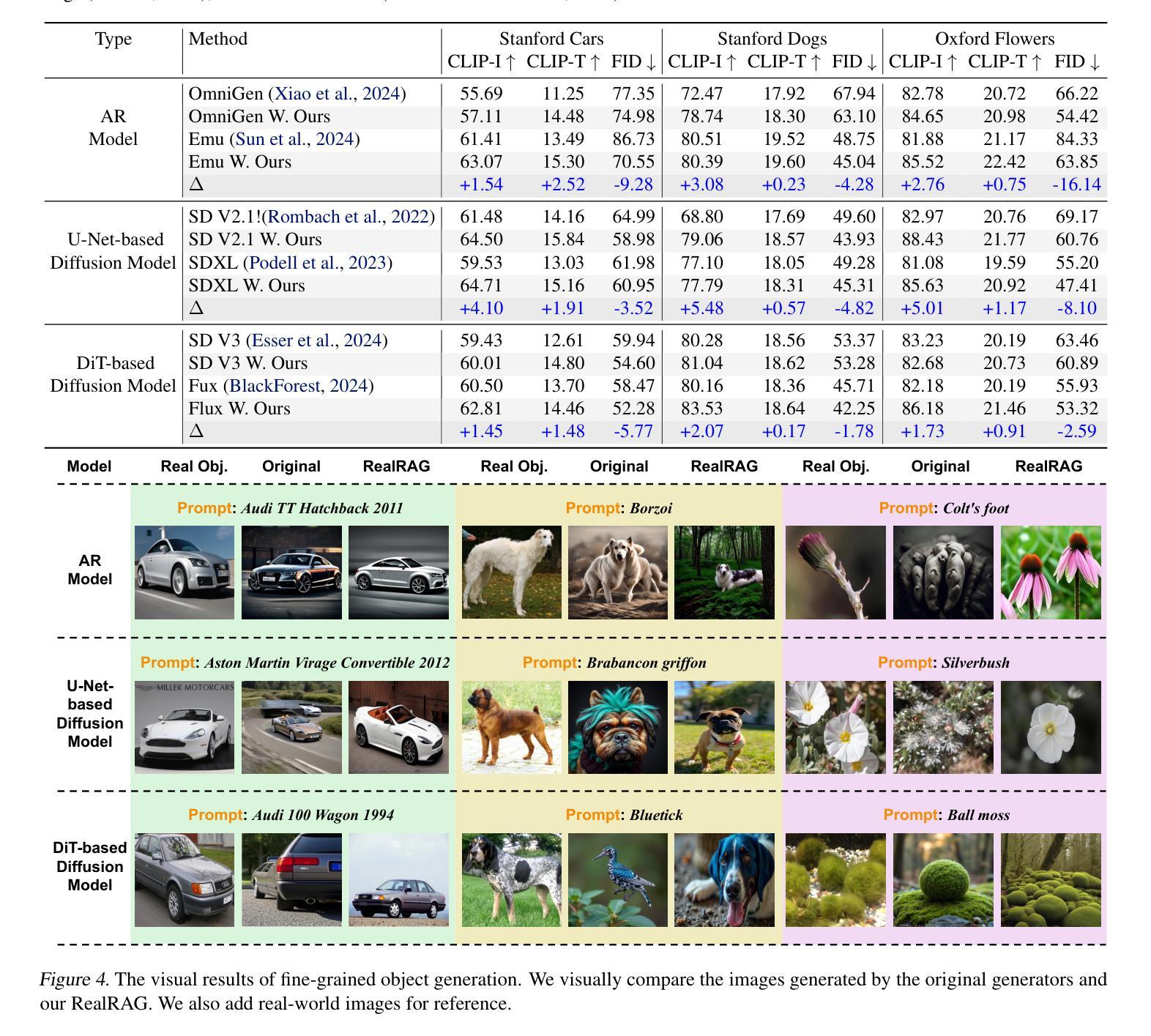
Contrastive Forward-Forward: A Training Algorithm of Vision Transformer
Authors:Hossein Aghagolzadeh, Mehdi Ezoji
Although backpropagation is widely accepted as a training algorithm for artificial neural networks, researchers are always looking for inspiration from the brain to find ways with potentially better performance. Forward-Forward is a new training algorithm that is more similar to what occurs in the brain, although there is a significant performance gap compared to backpropagation. In the Forward-Forward algorithm, the loss functions are placed after each layer, and the updating of a layer is done using two local forward passes and one local backward pass. Forward-Forward is in its early stages and has been designed and evaluated on simple multi-layer perceptron networks to solve image classification tasks. In this work, we have extended the use of this algorithm to a more complex and modern network, namely the Vision Transformer. Inspired by insights from contrastive learning, we have attempted to revise this algorithm, leading to the introduction of Contrastive Forward-Forward. Experimental results show that our proposed algorithm performs significantly better than the baseline Forward-Forward leading to an increase of up to 10% in accuracy and boosting the convergence speed by 5 to 20 times on Vision Transformer. Furthermore, if we take Cross Entropy as the baseline loss function in backpropagation, it will be demonstrated that the proposed modifications to the baseline Forward-Forward reduce its performance gap compared to backpropagation on Vision Transformer, and even outperforms it in certain conditions, such as inaccurate supervision.
尽管反向传播被广泛接受作为人工神经网络的训练算法,研究人员总是从大脑中寻找灵感,以寻找可能具有更好性能的替代方法。Forward-Forward是一种新的训练算法,它更接近大脑中发生的事情,尽管与反向传播相比,其性能还存在较大差距。在Forward-Forward算法中,损失函数被放置在每层之后,层的更新是通过两次局部前向传递和一次局部反向传递完成的。Forward-Forward仍处于早期阶段,并已针对简单的多层感知器网络进行设计用于解决图像分类任务。在这项工作中,我们将该算法的使用扩展到了更复杂和更现代的网络,即视觉转换器。受对比学习的启发,我们尝试对此算法进行了修订,从而引入了对比Forward-Forward。实验结果表明,我们提出的算法比基线Forward-Forward表现得更好,准确率提高了高达10%,并且在视觉转换器上收敛速度提高了5到20倍。此外,如果我们以交叉熵作为反向传播的基线损失函数,将证明对基线Forward-Forward的修改减少了其与反向传播在视觉转换器上的性能差距,并且在某些条件下(例如不准确的监督)甚至超过了反向传播的性能。
论文及项目相关链接
PDF 22 pages, 8 figures, under review
Summary
基于反向传播在人工神经网络中的广泛应用,研究者不断从大脑中汲取灵感以寻找性能更佳的训练算法。Forward-Forward是一种新的训练算法,其过程更接近大脑中的机制,尽管与反向传播相比仍存在性能差距。Forward-Forward算法将损失函数置于每层之后,并通过两次局部前向传递和一次局部反向传递进行层的更新。此工作将Forward-Forward算法扩展到了更复杂的现代网络——Vision Transformer上,并受到对比学习的启发对其进行了改进,从而引入了对比Forward-Forward。实验结果表明,与基线Forward-Forward相比,新算法性能显著提高,准确率提高高达10%,并在Vision Transformer上加快了5至20倍的收敛速度。此外,在某些条件下,如在不准确监督的情况下,新算法甚至超过了使用交叉熵作为基准损失函数的反向传播的性能。
Key Takeaways
- Forward-Forward是一种新的神经网络训练算法,其设计更接近大脑的工作机制。
- 在Forward-Forward算法中,损失函数被置于每层的末端,并通过两次前向传递和一次反向传递来更新层。
- Forward-Forward算法已在简单的多层感知器网络上进行了设计和评估,用于解决图像分类任务。
- 将Forward-Forward算法扩展到了更复杂的Vision Transformer网络。
- 结合对比学习洞察对Forward-Forward算法进行了改进,提出了Contrastive Forward-Forward。
- 实验结果表明,新算法在Vision Transformer上的性能优于基线Forward-Forward,提高了准确率和收敛速度。
点此查看论文截图
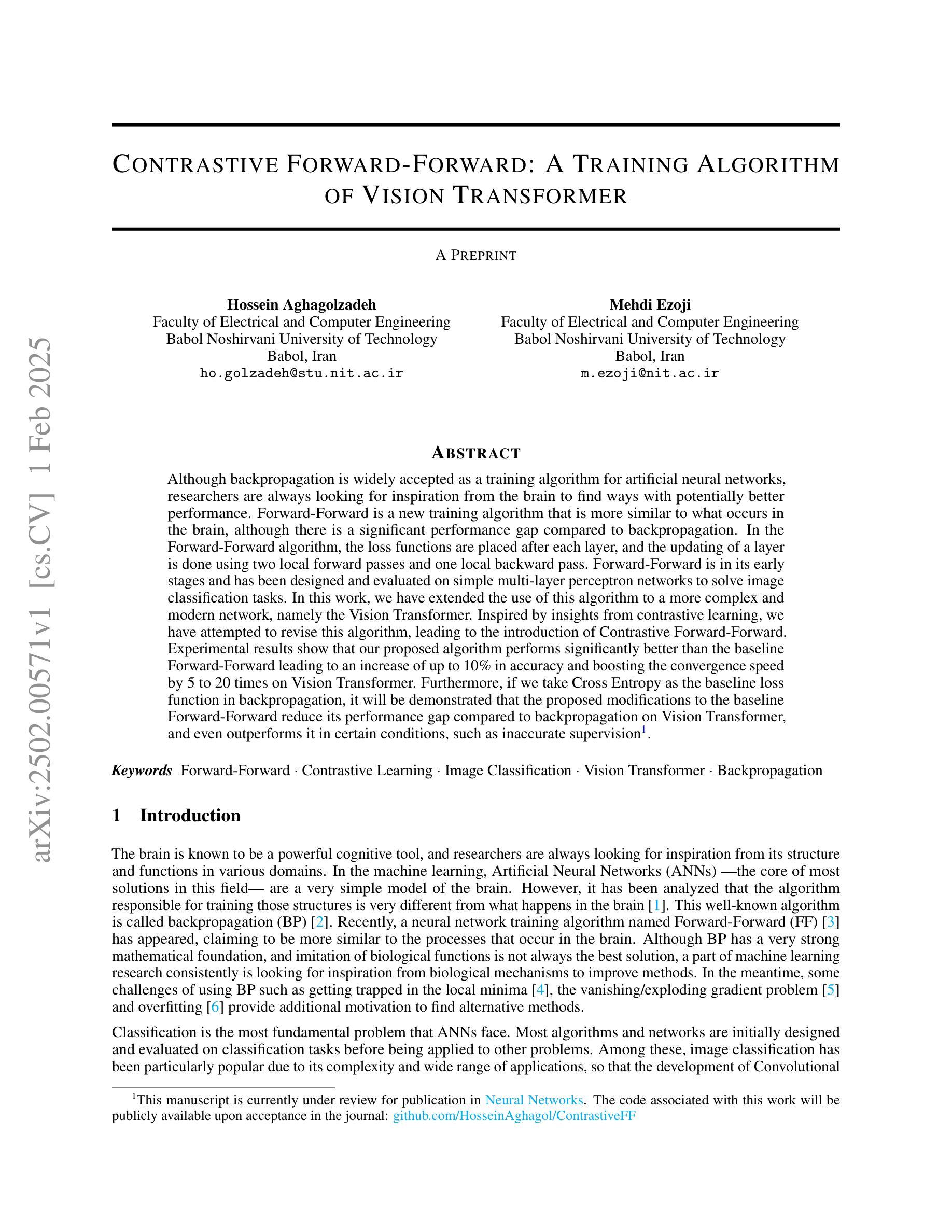
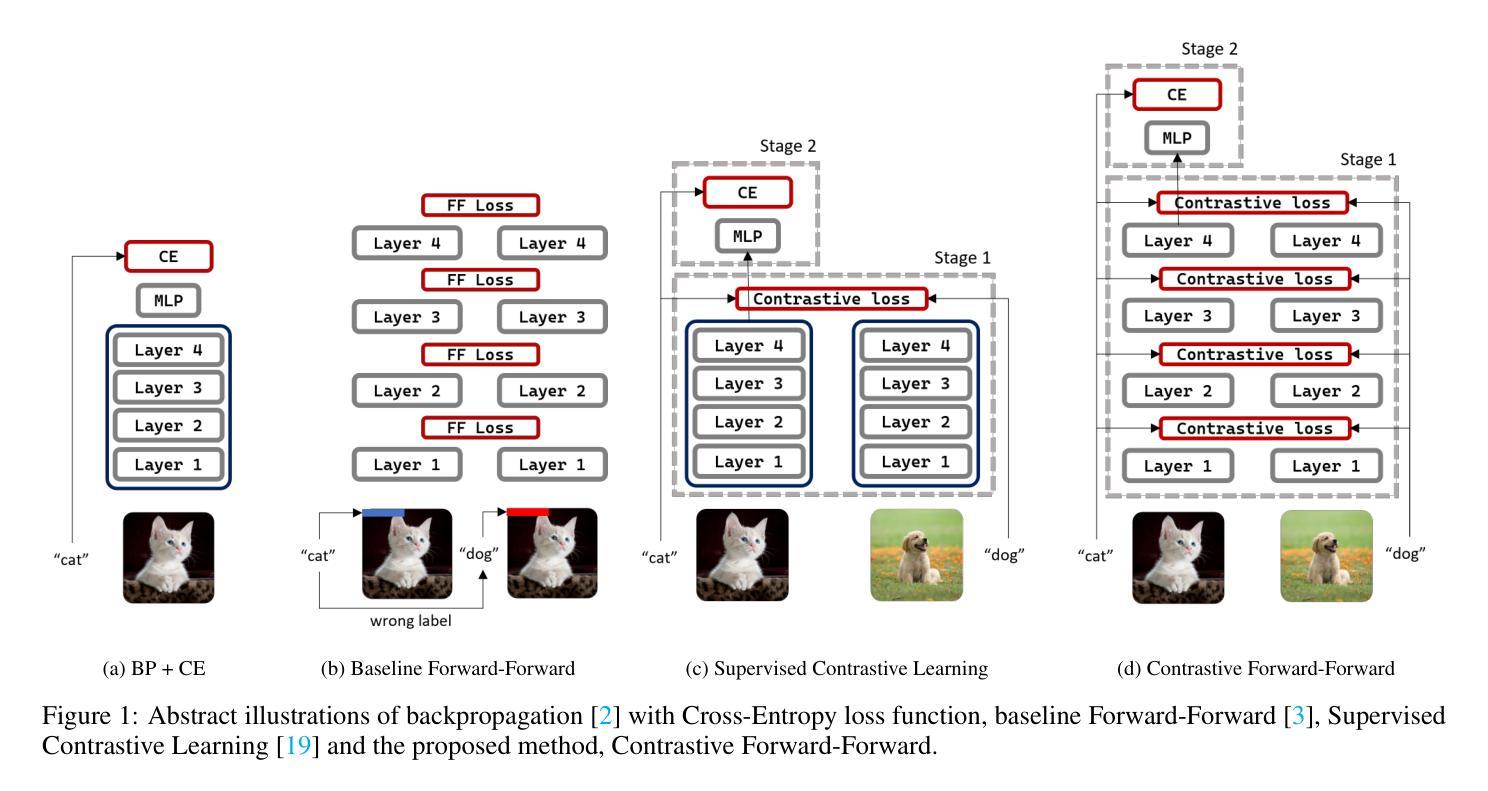
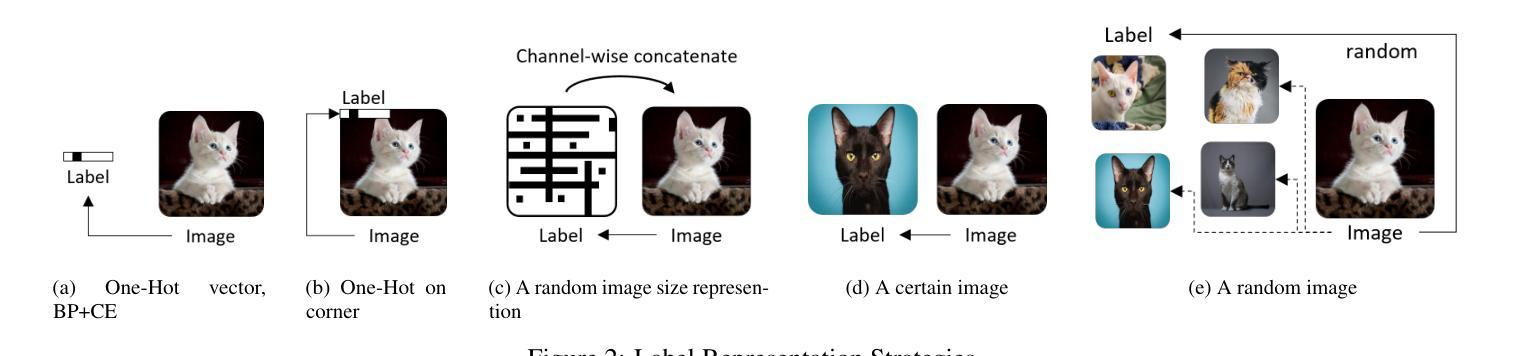