⚠️ 以下所有内容总结都来自于 大语言模型的能力,如有错误,仅供参考,谨慎使用
🔴 请注意:千万不要用于严肃的学术场景,只能用于论文阅读前的初筛!
💗 如果您觉得我们的项目对您有帮助 ChatPaperFree ,还请您给我们一些鼓励!⭐️ HuggingFace免费体验
2025-02-06 更新
AAD-DCE: An Aggregated Multimodal Attention Mechanism for Early and Late Dynamic Contrast Enhanced Prostate MRI Synthesis
Authors:Divya Bharti, Sriprabha Ramanarayanan, Sadhana S, Kishore Kumar M, Keerthi Ram, Harsh Agarwal, Ramesh Venkatesan, Mohanasankar Sivaprakasam
Dynamic Contrast-Enhanced Magnetic Resonance Imaging (DCE-MRI) is a medical imaging technique that plays a crucial role in the detailed visualization and identification of tissue perfusion in abnormal lesions and radiological suggestions for biopsy. However, DCE-MRI involves the administration of a Gadolinium based (Gad) contrast agent, which is associated with a risk of toxicity in the body. Previous deep learning approaches that synthesize DCE-MR images employ unimodal non-contrast or low-dose contrast MRI images lacking focus on the local perfusion information within the anatomy of interest. We propose AAD-DCE, a generative adversarial network (GAN) with an aggregated attention discriminator module consisting of global and local discriminators. The discriminators provide a spatial embedded attention map to drive the generator to synthesize early and late response DCE-MRI images. Our method employs multimodal inputs - T2 weighted (T2W), Apparent Diffusion Coefficient (ADC), and T1 pre-contrast for image synthesis. Extensive comparative and ablation studies on the ProstateX dataset show that our model (i) is agnostic to various generator benchmarks and (ii) outperforms other DCE-MRI synthesis approaches with improvement margins of +0.64 dB PSNR, +0.0518 SSIM, -0.015 MAE for early response and +0.1 dB PSNR, +0.0424 SSIM, -0.021 MAE for late response, and (ii) emphasize the importance of attention ensembling. Our code is available at https://github.com/bhartidivya/AAD-DCE.
动态对比增强磁共振成像(DCE-MRI)是一种医学成像技术,在异常病变组织灌注的详细可视化和识别中起着至关重要的作用,并为活检提供放射学建议。然而,DCE-MRI需要使用基于钆(Gad)的造影剂,这带来了体内毒性的风险。之前合成DCE-MR图像的深度学习方法主要使用非对比或低剂量对比MRI图像,缺乏对焦于感兴趣部位的局部灌注信息。我们提出了AAD-DCE,这是一个生成对抗网络(GAN),其中包含一个聚合注意力判别器模块,该模块由全局和局部判别器组成。判别器提供空间嵌入注意力图,以驱动生成器合成早期和晚期响应DCE-MRI图像。我们的方法采用多模态输入,包括T2加权(T2W)、表观扩散系数(ADC)和T1预对比图像进行图像合成。在ProstateX数据集上的广泛比较和消融研究表明,我们的模型(i)不受各种生成器基准测试的影响;(ii)在早反应和晚反应方面,与其他DCE-MRI合成方法相比,我们的模型分别提高了+0.64 dB的峰值信噪比(PSNR)、+0.0518的结构相似性度量(SSIM)和-0.015的平均绝对误差(MAE),以及+0.1 dB的PSNR、+0.0424的SSIM和-0.021的MAE,并强调了注意力集成的重要性。我们的代码可在https://github.com/bhartidivya/AAD-DCE上找到。
论文及项目相关链接
Summary:基于生成对抗网络(GAN)的AAD-DCE模型,利用多模态输入信息合成DCE-MRI图像。该模型采用全局和局部鉴别器组成的聚合注意力鉴别器模块,提供空间嵌入注意力图以指导生成器合成早期和晚期响应的DCE-MRI图像。在ProstateX数据集上的广泛对比和消融研究表明,该模型在性能上优于其他DCE-MRI合成方法。
Key Takeaways:
- AAD-DCE模型利用生成对抗网络(GAN)技术,旨在合成动态对比增强磁共振成像(DCE-MRI)图像。
- 该模型通过采用聚合注意力鉴别器模块,包含全局和局部鉴别器,以提高图像合成的质量。
- AAD-DCE模型采用多模态输入,包括T2加权(T2W)、表观扩散系数(ADC)和T1预对比图像。
- 在ProstateX数据集上的研究结果显示,AAD-DCE模型在性能上优于其他DCE-MRI图像合成方法。
- 该模型的性能改进体现在早期和晚期响应的PSNR、SSIM和MAE等指标上。
- AAD-DCE模型的代码已公开可用。
点此查看论文截图
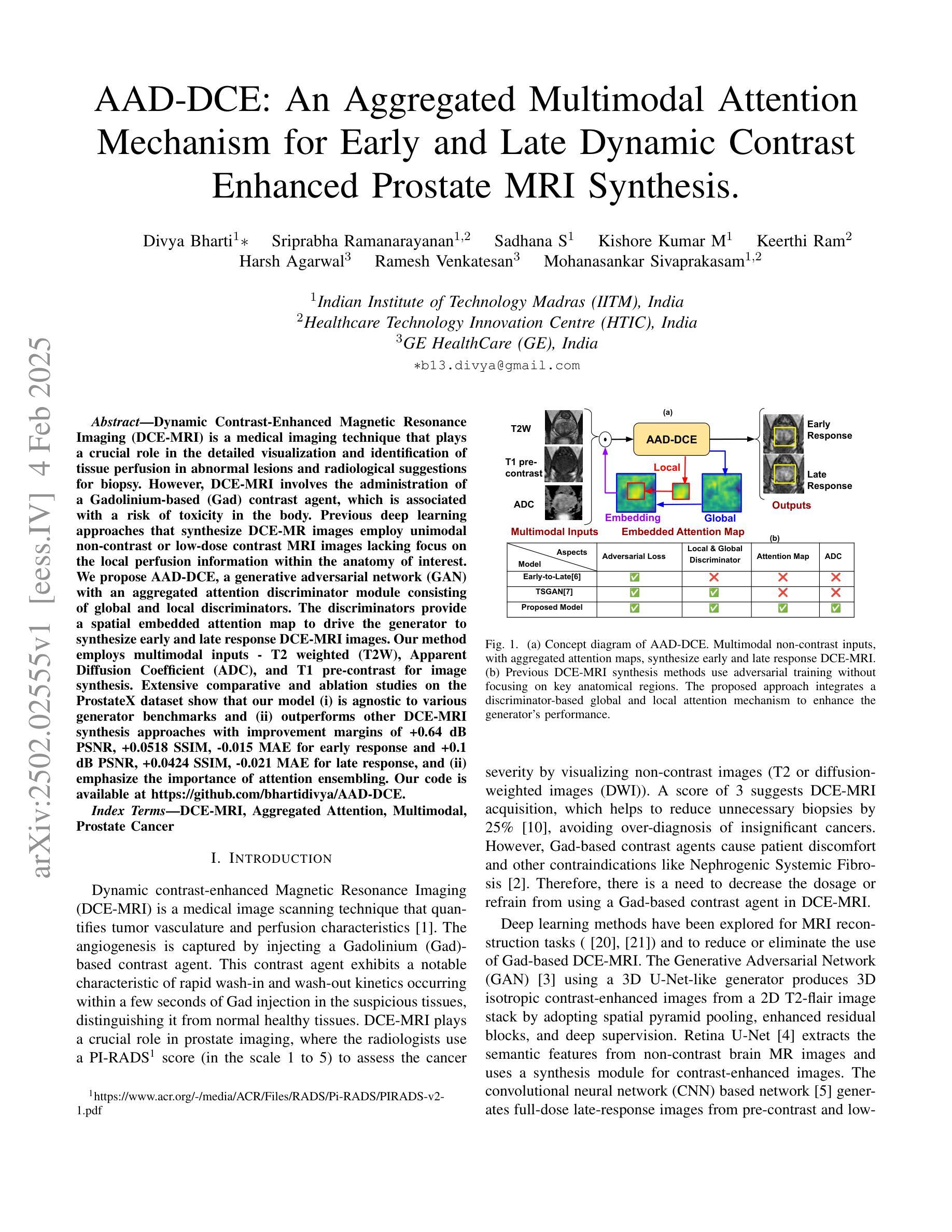
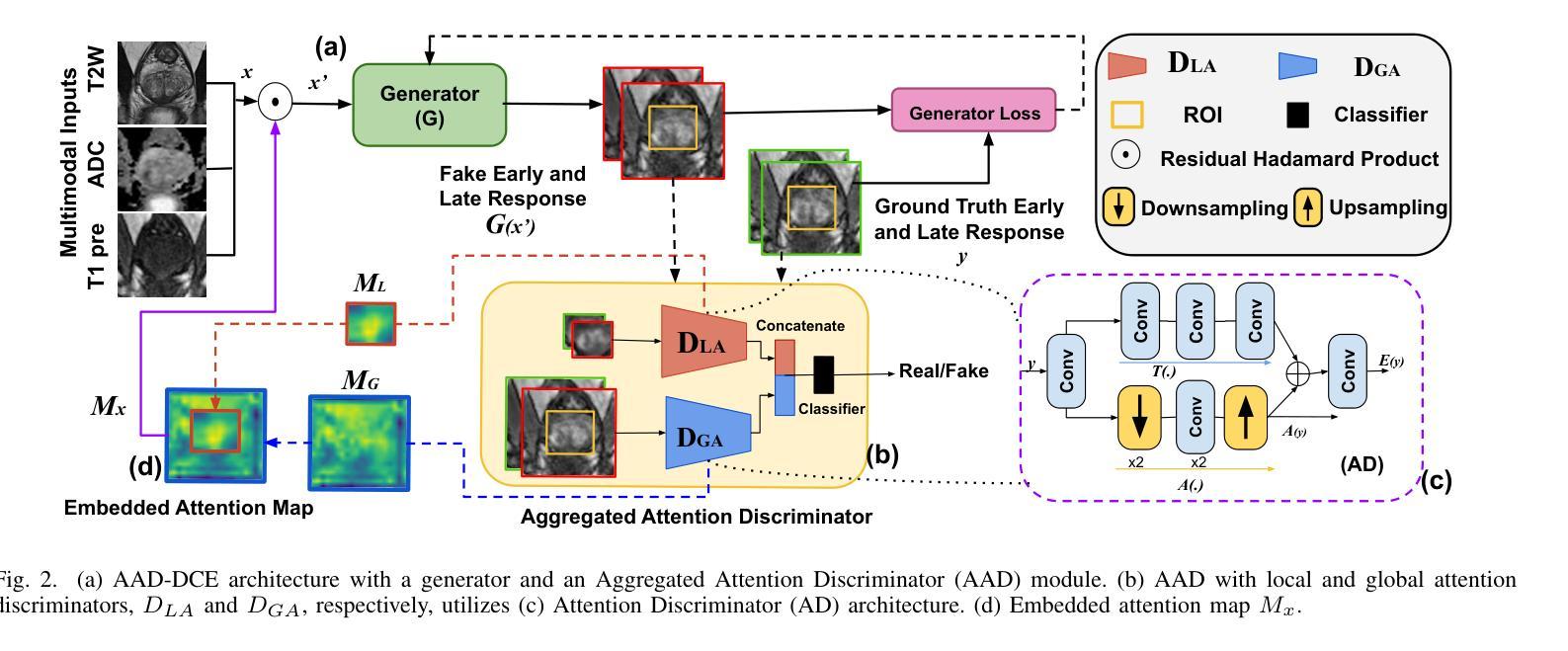
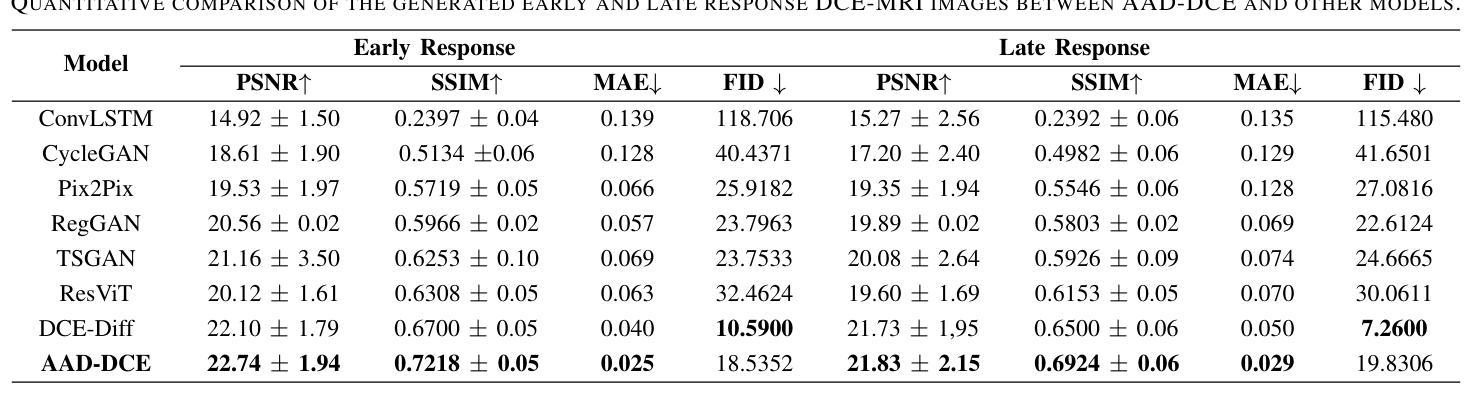
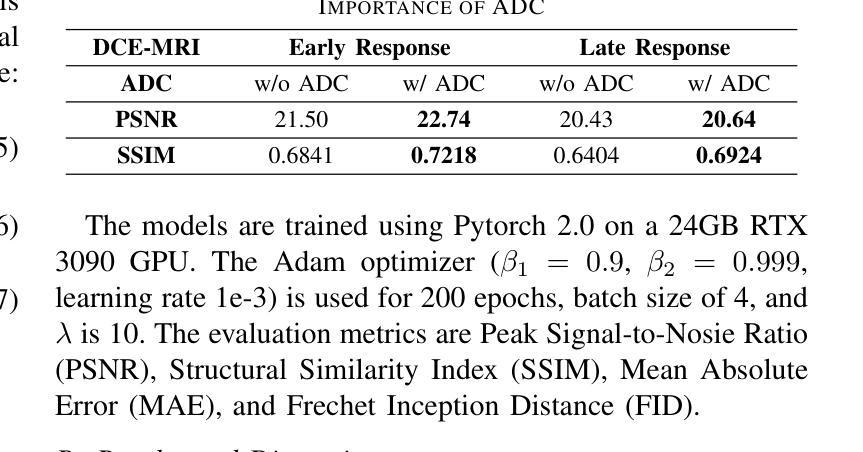
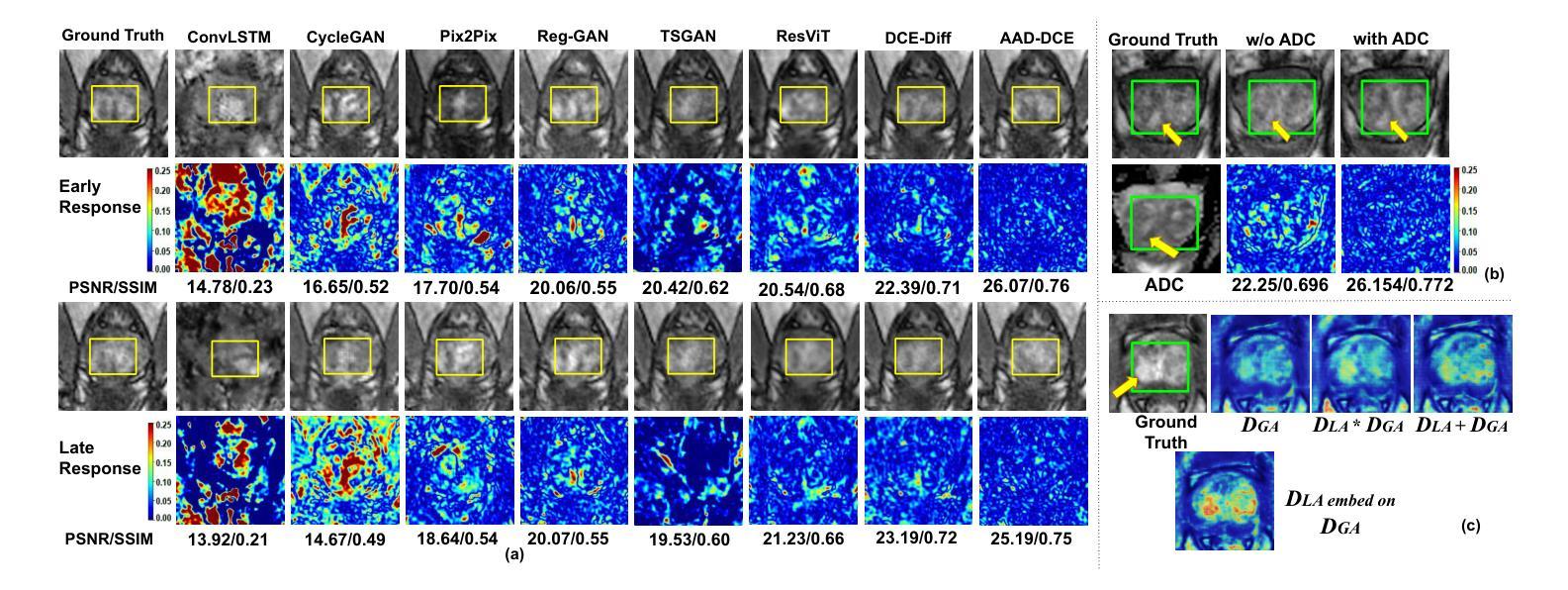
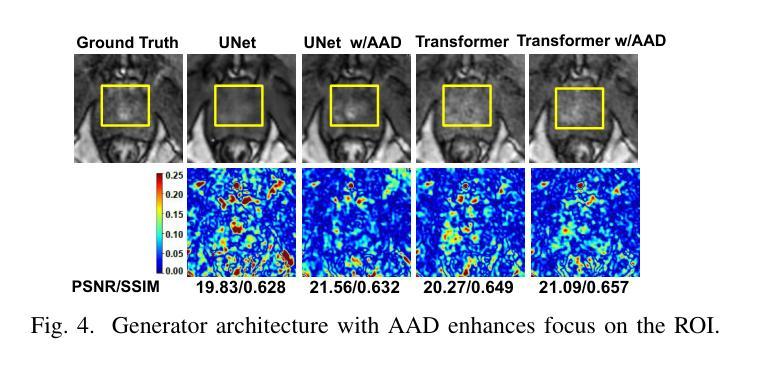
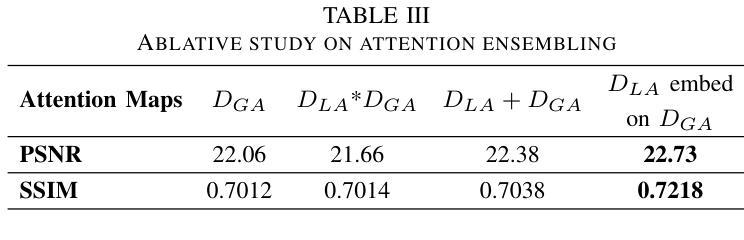
BC-GAN: A Generative Adversarial Network for Synthesizing a Batch of Collocated Clothing
Authors:Dongliang Zhou, Haijun Zhang, Jianghong Ma, Jianyang Shi
Collocated clothing synthesis using generative networks has become an emerging topic in the field of fashion intelligence, as it has significant potential economic value to increase revenue in the fashion industry. In previous studies, several works have attempted to synthesize visually-collocated clothing based on a given clothing item using generative adversarial networks (GANs) with promising results. These works, however, can only accomplish the synthesis of one collocated clothing item each time. Nevertheless, users may require different clothing items to meet their multiple choices due to their personal tastes and different dressing scenarios. To address this limitation, we introduce a novel batch clothing generation framework, named BC-GAN, which is able to synthesize multiple visually-collocated clothing images simultaneously. In particular, to further improve the fashion compatibility of synthetic results, BC-GAN proposes a new fashion compatibility discriminator in a contrastive learning perspective by fully exploiting the collocation relationship among all clothing items. Our model was examined in a large-scale dataset with compatible outfits constructed by ourselves. Extensive experiment results confirmed the effectiveness of our proposed BC-GAN in comparison to state-of-the-art methods in terms of diversity, visual authenticity, and fashion compatibility.
利用生成网络进行搭配服装合成已成为时尚智能领域的一个新兴话题,因为它为时尚产业带来了巨大的经济增值潜力。在先前的研究中,一些作品已经尝试使用生成对抗网络(GANs)基于给定的服装项目进行视觉搭配服装的合成,并取得了令人鼓舞的结果。然而,这些工作每次都只能完成一件搭配服装的合成。然而,由于个人口味和不同的穿衣场景,用户可能需要不同的服装项目来满足他们的多种选择。为了解决这一限制,我们引入了一个新颖的批量服装生成框架,名为BC-GAN,它能够同时合成多个视觉搭配服装图像。特别是,为了进一步提高合成结果的时尚兼容性,BC-GAN从对比学习的角度提出了一个新的时尚兼容性鉴别器,充分利用了所有服装项目之间的搭配关系。我们的模型是在我们自己构建的大规模兼容服装数据集上进行测试的。大量的实验结果证实,与最新方法相比,我们所提出的BC-GAN在多样性、视觉真实性和时尚兼容性方面都非常有效。
论文及项目相关链接
PDF This paper was accepted by IEEE TCSVT
Summary
基于生成对抗网络(GANs)的联合服装合成已成为时尚智能领域的一个新兴话题,具有巨大的经济价值。尽管之前的研究已经尝试使用GANs对给定的服装项目进行视觉上联合的服装合成,并取得了一些成果,但它们每次只能合成一件联合服装。针对用户因个人喜好和不同穿衣场景需要多种服装选择的需求,我们提出了一种新型的批量服装生成框架BC-GAN,能够同时合成多种视觉上联合的服装图像。特别是,为了进一步提高合成结果的时尚兼容性,BC-GAN从对比学习的角度提出了一个新的时尚兼容性鉴别器,充分利用所有服装项目之间的搭配关系。在由我们自己构建的大规模兼容服装数据集上进行的实验证实,与最先进的方法相比,BC-GAN在多样性、视觉真实性和时尚兼容性方面更加有效。
Key Takeaways
- 生成对抗网络(GANs)在时尚智能领域的联合服装合成成为新兴话题,具有巨大的经济价值。
- 现有研究只能单次合成一种联合服装,无法满足用户多样化的需求。
- 提出了一种新型的批量服装生成框架BC-GAN,能够同时合成多种视觉上联合的服装图像。
- BC-GAN通过引入时尚兼容性鉴别器,提高了合成结果的时尚兼容性。
- 该鉴别器从对比学习的角度出发,充分利用服装项目之间的搭配关系。
- BC-GAN在多样性、视觉真实性和时尚兼容性方面表现出优异的性能。
点此查看论文截图
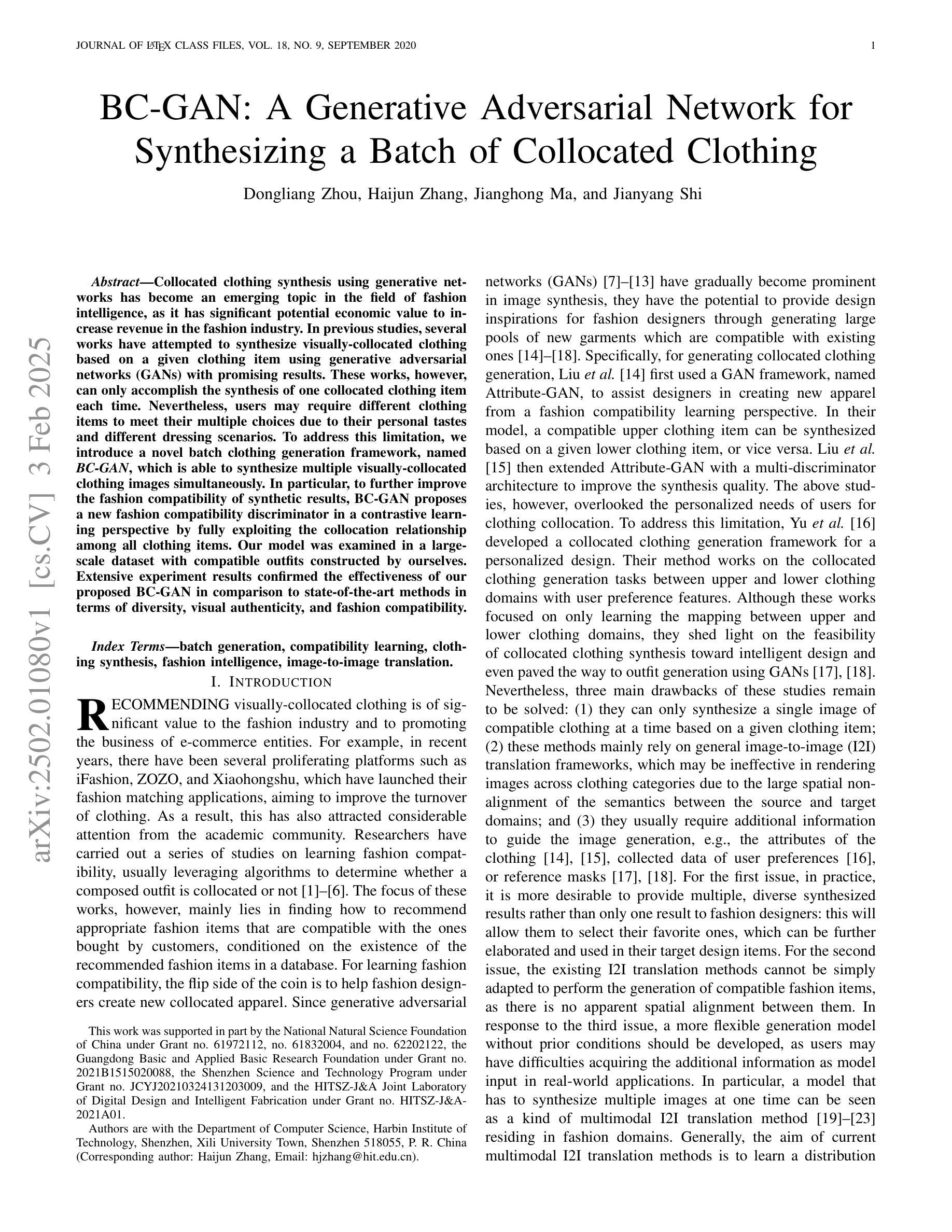
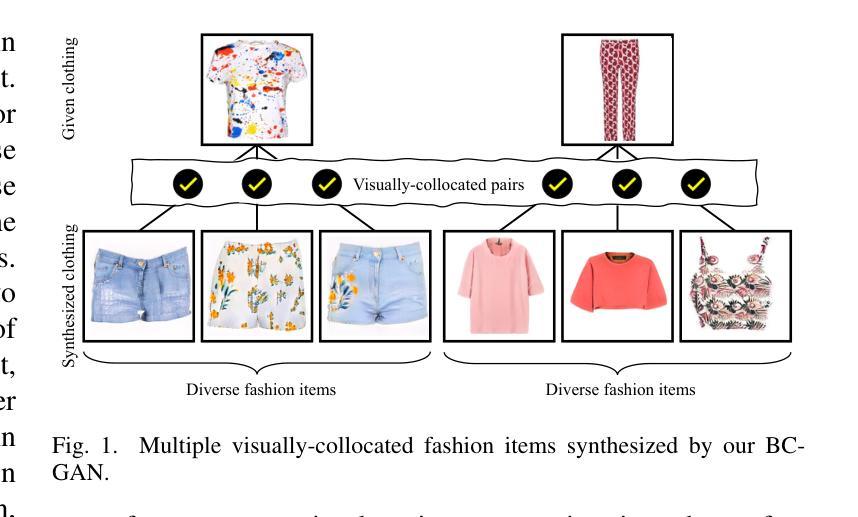
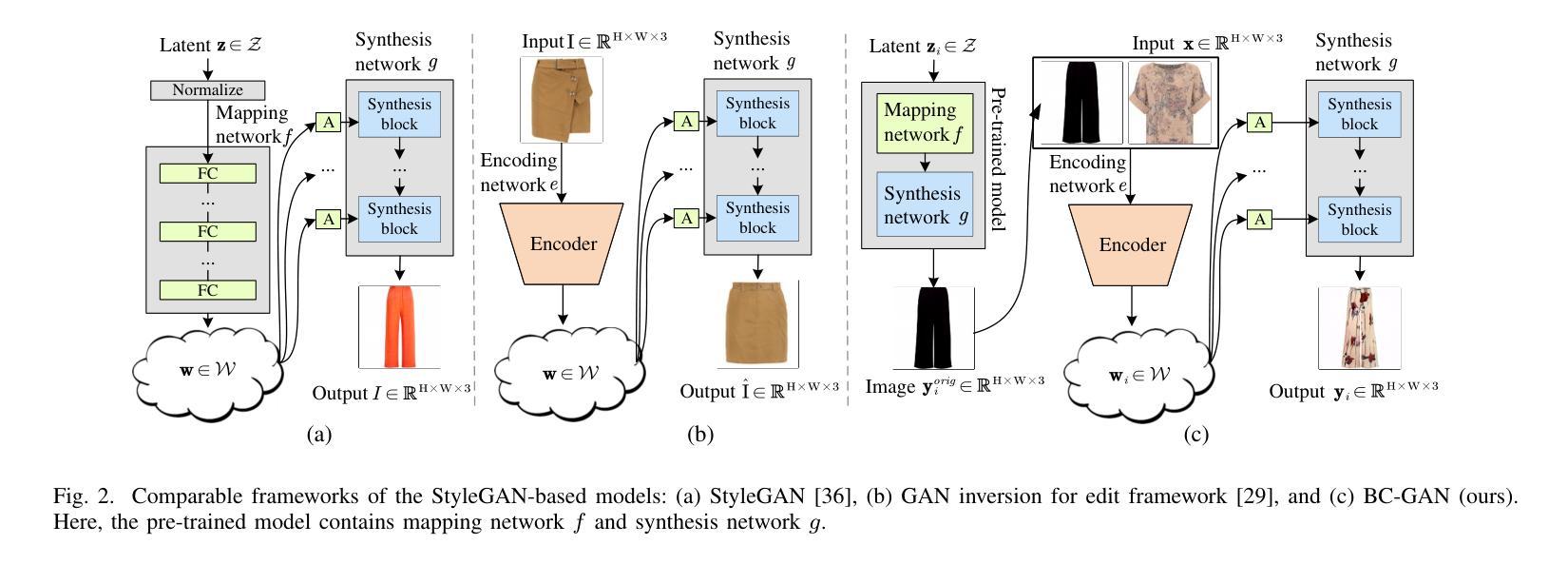
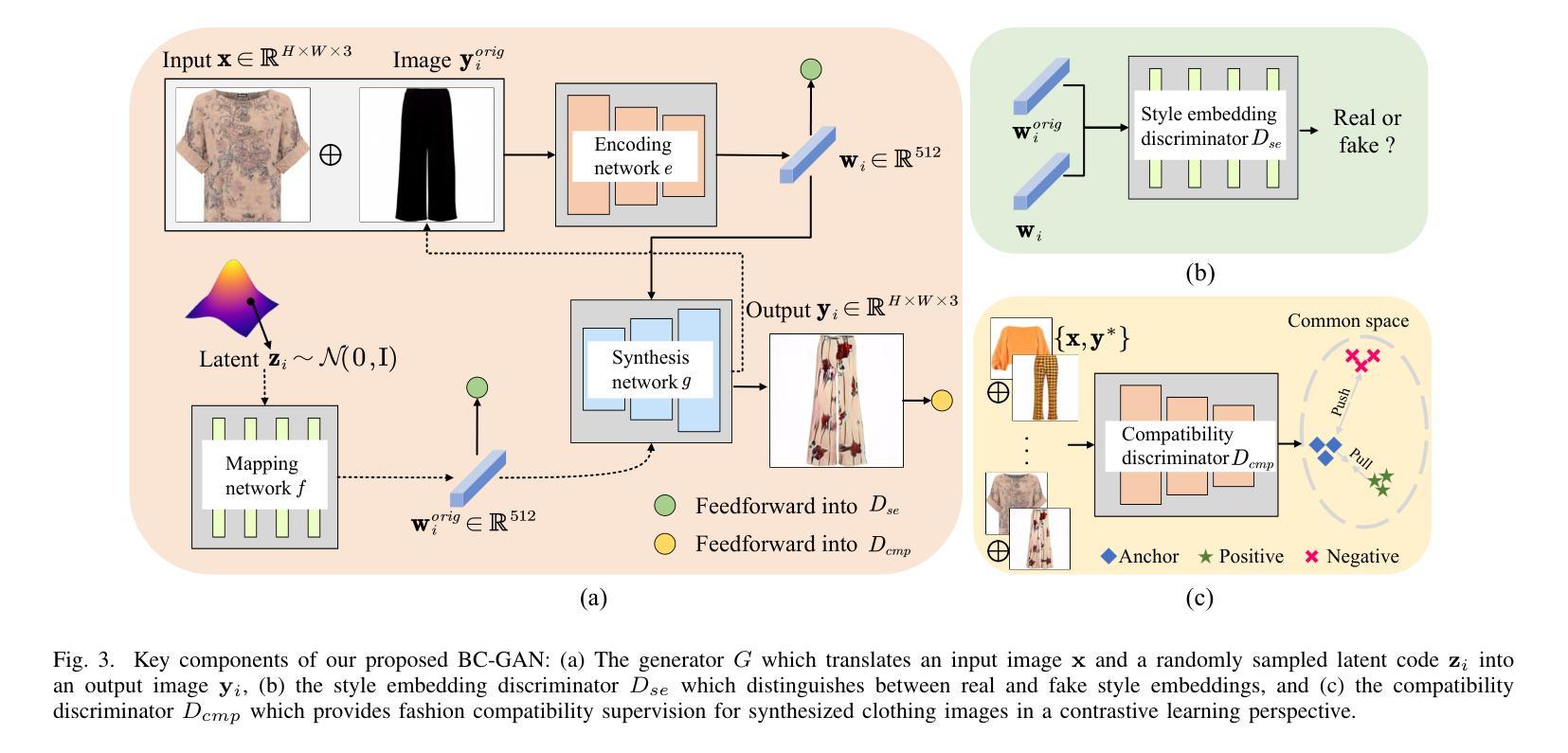
Weak Supervision Dynamic KL-Weighted Diffusion Models Guided by Large Language Models
Authors:Julian Perry, Frank Sanders, Carter Scott
In this paper, we presents a novel method for improving text-to-image generation by combining Large Language Models (LLMs) with diffusion models, a hybrid approach aimed at achieving both higher quality and efficiency in image synthesis from text descriptions. Our approach introduces a new dynamic KL-weighting strategy to optimize the diffusion process, along with incorporating semantic understanding from pre-trained LLMs to guide the generation process. The proposed method significantly improves both the visual quality and alignment of generated images with text descriptions, addressing challenges such as computational inefficiency, instability in training, and robustness to textual variability. We evaluate our method on the COCO dataset and demonstrate its superior performance over traditional GAN-based models, both quantitatively and qualitatively. Extensive experiments, including ablation studies and human evaluations, confirm that our method outperforms existing approaches in terms of image realism, relevance to the input text, and overall aesthetic quality. Our approach also shows promise in scalability to other multimodal tasks, making it a versatile solution for a wide range of generative applications.
本文提出了一种结合大型语言模型(LLMs)与扩散模型改进文本到图像生成的新方法。这是一种混合方法,旨在实现文本描述生成图像的高质量和高效率。我们的方法引入了一种新的动态KL加权策略来优化扩散过程,同时结合预训练LLMs的语义理解来指导生成过程。所提出的方法显著提高了生成图像与文本描述之间的视觉质量和对齐程度,解决了计算效率低下、训练不稳定以及对文本变化的不鲁棒等挑战。我们在COCO数据集上评估了我们的方法,与传统基于GAN的模型相比,从定量和定性两个方面都证明了其优越性。大量实验,包括消融研究和人类评估,证实我们的方法在图像真实性、与输入文本的关联性和整体美学质量方面优于现有方法。我们的方法在其他多模式任务中的可扩展性也显示出前景,使其成为广泛生成应用的通用解决方案。
论文及项目相关链接
Summary
本文提出了一种将大型语言模型(LLMs)与扩散模型相结合的新方法,旨在提高文本到图像的生成质量及效率。该方法引入了一种新的动态KL权重策略来优化扩散过程,并结合预训练LLMs的语义理解来引导生成过程。该方法显著提高了生成图像与文本描述之间的视觉质量和一致性,解决了计算效率低下、训练不稳定和对文本变化的不稳健等挑战。在COCO数据集上的评估表明,与传统基于GAN的模型相比,该方法在图像逼真度、与输入文本的关联性和整体美学质量方面表现出卓越性能。此外,该方法在其他多模式任务中显示出可扩展性,成为广泛生成应用的通用解决方案。
Key Takeaways
- 提出了结合大型语言模型(LLMs)和扩散模型的新方法,用于改进文本到图像的生成。
- 引入动态KL权重策略优化扩散过程。
- 通过预训练LLMs的语义理解引导生成过程。
- 在COCO数据集上表现出卓越性能,提高了图像与文本描述之间的视觉质量和一致性。
- 解决了计算效率低下、训练不稳定和对文本变化的不稳健等挑战。
- 方法在其他多模式任务中显示出可扩展性。
点此查看论文截图
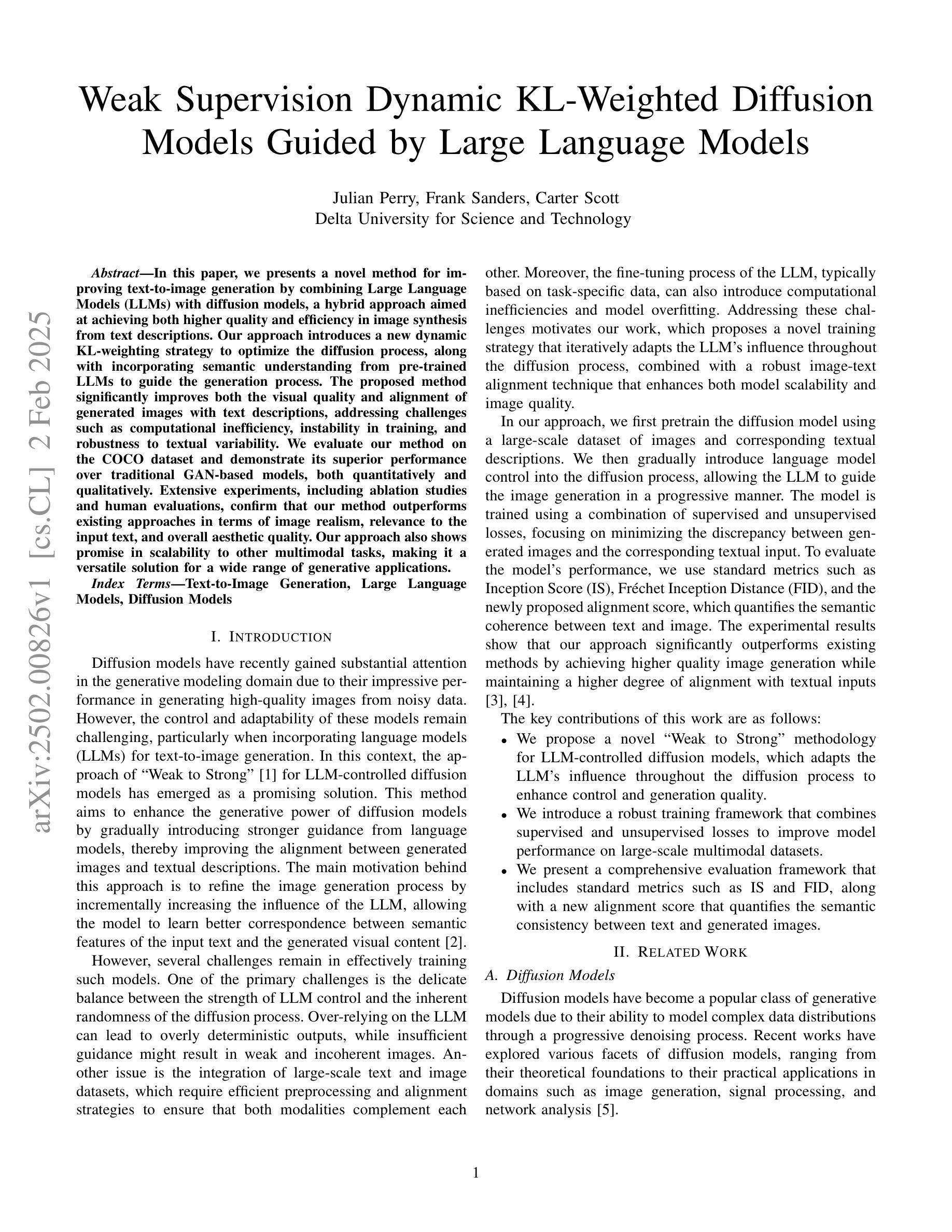
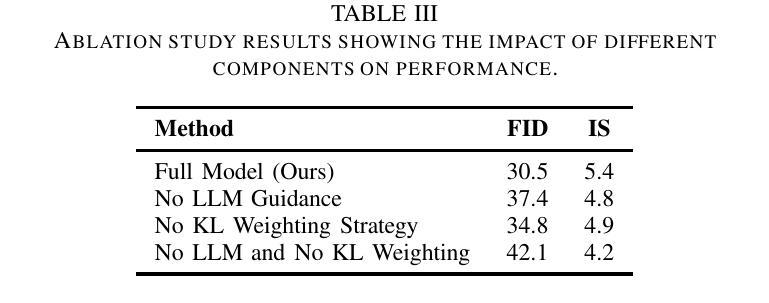
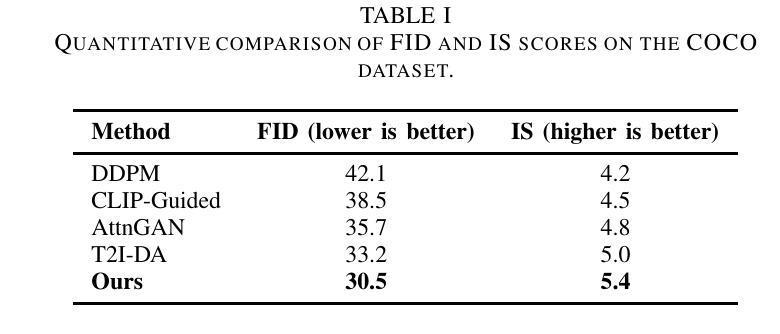
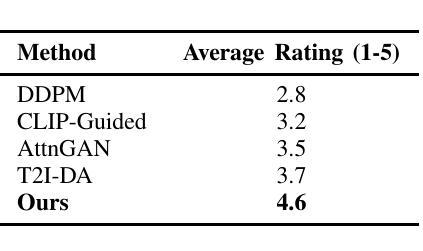
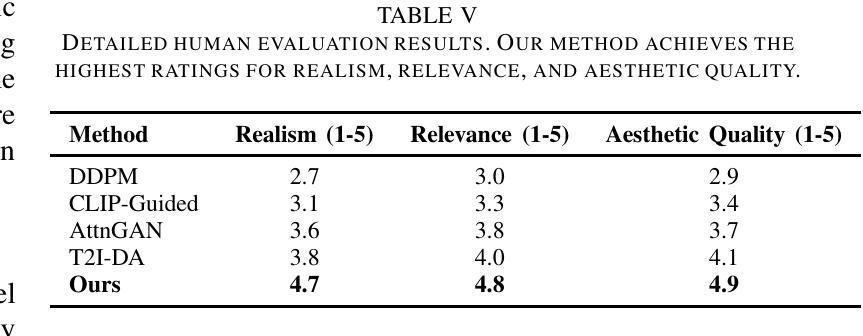
GAN-Based Architecture for Low-dose Computed Tomography Imaging Denoising
Authors:Yunuo Wang, Ningning Yang, Jialin Li
Generative Adversarial Networks (GANs) have surfaced as a revolutionary element within the domain of low-dose computed tomography (LDCT) imaging, providing an advanced resolution to the enduring issue of reconciling radiation exposure with image quality. This comprehensive review synthesizes the rapid advancements in GAN-based LDCT denoising techniques, examining the evolution from foundational architectures to state-of-the-art models incorporating advanced features such as anatomical priors, perceptual loss functions, and innovative regularization strategies. We critically analyze various GAN architectures, including conditional GANs (cGANs), CycleGANs, and Super-Resolution GANs (SRGANs), elucidating their unique strengths and limitations in the context of LDCT denoising. The evaluation provides both qualitative and quantitative results related to the improvements in performance in benchmark and clinical datasets with metrics such as PSNR, SSIM, and LPIPS. After highlighting the positive results, we discuss some of the challenges preventing a wider clinical use, including the interpretability of the images generated by GANs, synthetic artifacts, and the need for clinically relevant metrics. The review concludes by highlighting the essential significance of GAN-based methodologies in the progression of precision medicine via tailored LDCT denoising models, underlining the transformative possibilities presented by artificial intelligence within contemporary radiological practice.
生成对抗网络(GANs)在低剂量计算机断层扫描(LDCT)成像领域掀起了一场革命,为解决辐射暴露与图像质量之间的持久问题提供了高级解决方案。这篇综述综合了基于GAN的LDCT去噪技术的快速发展,从基础架构的演变到结合先进特性的最新模型,例如解剖学先验、感知损失函数和创新正则化策略。我们深入分析了各种GAN架构,包括条件GAN(cGANs)、CycleGANs和超分辨率GAN(SRGANs),阐明了它们在LDCT去噪背景下的独特优势和局限性。评估结果提供与基准测试和临床数据集性能提升相关的定性和定量结果,包括峰值信噪比、结构相似性度量指标和LPIPS等。在突出积极结果之后,我们讨论了一些阻碍更广泛临床应用的挑战,包括GAN生成图像的解读性、合成伪影以及需要临床相关指标等。最后,通过强调基于GAN的方法在通过定制LDCT去噪模型推动精准医学发展中的关键作用,本文总结了人工智能在当代放射实践中的变革性潜力。
论文及项目相关链接
Summary
GAN在网络领域掀起革命性变革,特别是在低剂量计算机断层扫描(LDCT)成像方面,为解决辐射暴露与图像质量之间的平衡问题提供了先进解决方案。本文综述了基于GAN的LDCT去噪技术的快速发展,从基础架构到融合先进特性的最新模型,如解剖先验、感知损失函数和创新正则化策略等。本文详细分析了各种GAN架构,包括条件GAN(cGAN)、CycleGAN和超分辨率GAN(SRGAN),阐述了它们在LDCT去噪方面的独特优势和局限性。在评估和对比中,提供了与基准测试数据集和临床数据集相关的性能改进定量和定性结果。本文强调了一些挑战,包括GAN生成的图像的可解释性、合成伪影以及需要临床相关指标等,阻碍了其更广泛的临床应用。最后,本文强调了基于GAN的方法在精准医学发展中的重要作用,特别是在定制LDCT去噪模型方面,突显人工智能在当代放射实践中的变革性潜力。
Key Takeaways
- GANs在低剂量计算机断层扫描(LDCT)成像领域具有革命性作用,解决了辐射暴露与图像质量之间的平衡问题。
- 基于GAN的LDCT去噪技术发展迅速,涵盖从基础架构到融合先进特性的最新模型。
- 条件GAN(cGAN)、CycleGAN和超分辨率GAN(SRGAN)等架构在LDCT去噪方面各有优势和局限性。
- GAN生成的图像存在可解释性、合成伪影等挑战,需要临床相关指标进行评估。
- 基于GAN的方法在精准医学发展中具有重要作用,特别是在定制LDCT去噪模型方面。
- GANs的应用展现了人工智能在当代放射实践中的变革性潜力。
点此查看论文截图
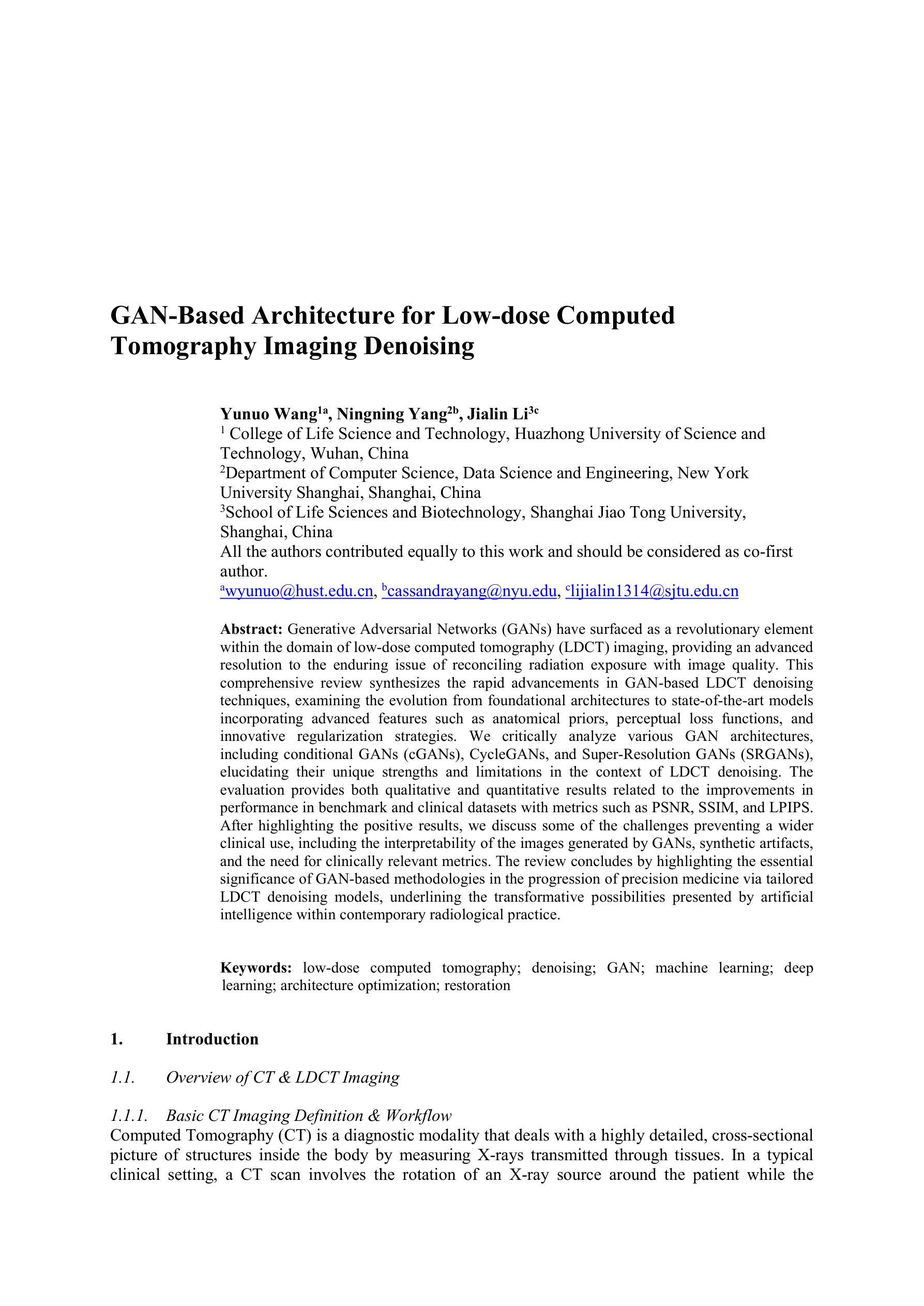
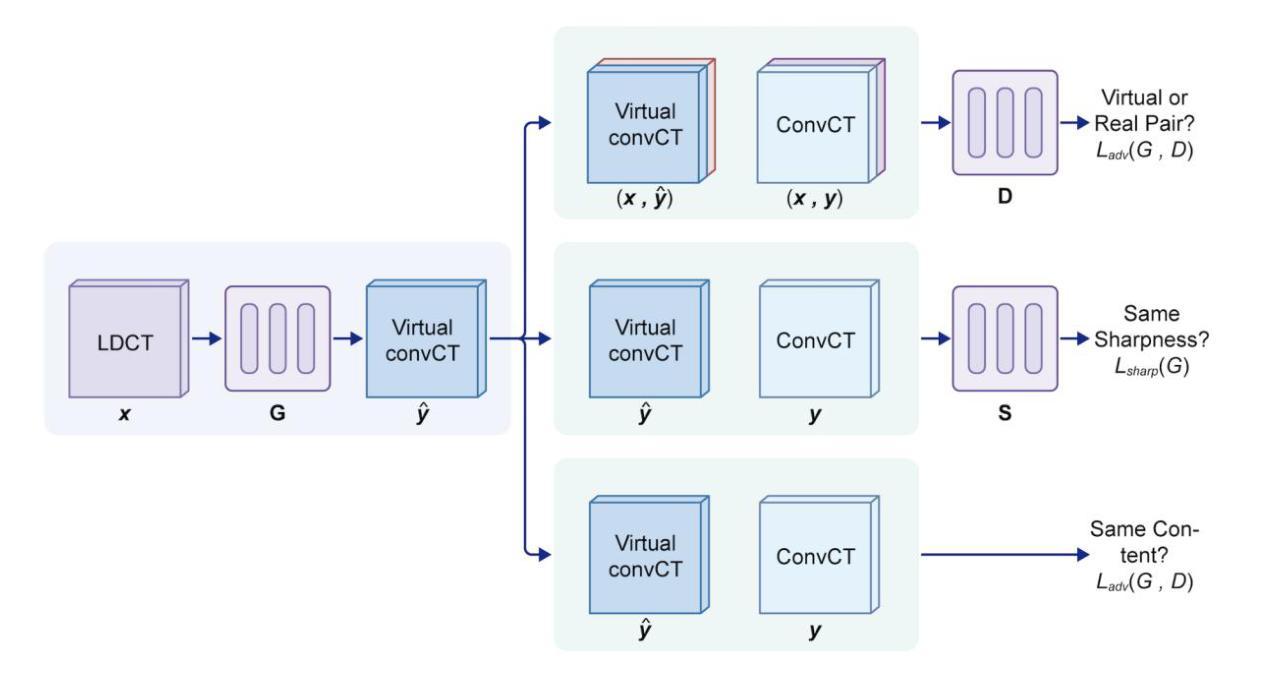
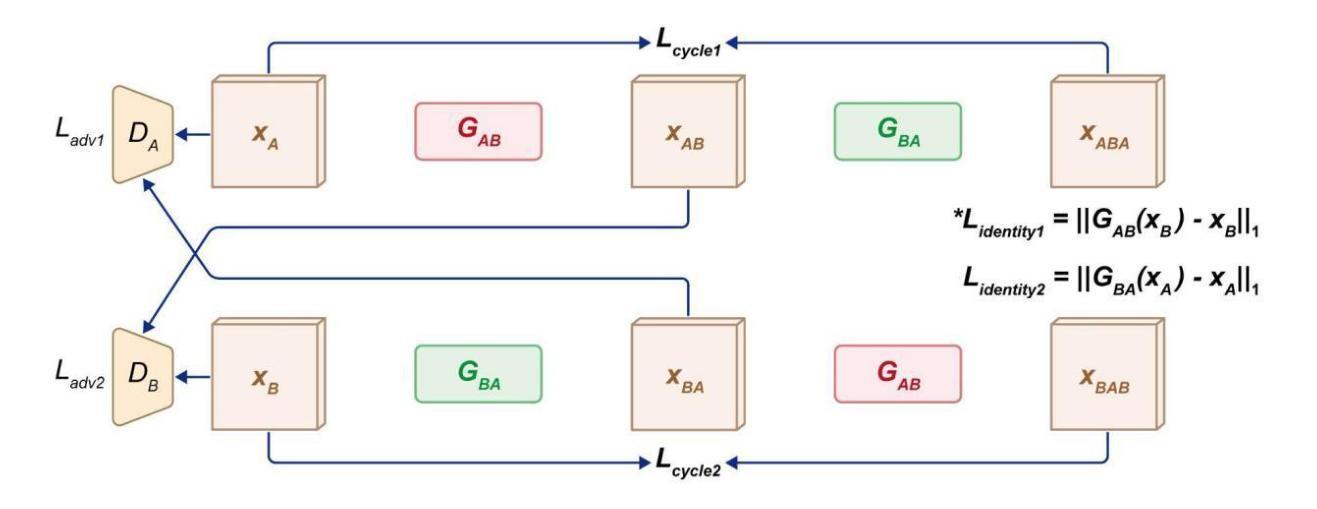
Posterior-Mean Rectified Flow: Towards Minimum MSE Photo-Realistic Image Restoration
Authors:Guy Ohayon, Tomer Michaeli, Michael Elad
Photo-realistic image restoration algorithms are typically evaluated by distortion measures (e.g., PSNR, SSIM) and by perceptual quality measures (e.g., FID, NIQE), where the desire is to attain the lowest possible distortion without compromising on perceptual quality. To achieve this goal, current methods commonly attempt to sample from the posterior distribution, or to optimize a weighted sum of a distortion loss (e.g., MSE) and a perceptual quality loss (e.g., GAN). Unlike previous works, this paper is concerned specifically with the optimal estimator that minimizes the MSE under a constraint of perfect perceptual index, namely where the distribution of the reconstructed images is equal to that of the ground-truth ones. A recent theoretical result shows that such an estimator can be constructed by optimally transporting the posterior mean prediction (MMSE estimate) to the distribution of the ground-truth images. Inspired by this result, we introduce Posterior-Mean Rectified Flow (PMRF), a simple yet highly effective algorithm that approximates this optimal estimator. In particular, PMRF first predicts the posterior mean, and then transports the result to a high-quality image using a rectified flow model that approximates the desired optimal transport map. We investigate the theoretical utility of PMRF and demonstrate that it consistently outperforms previous methods on a variety of image restoration tasks.
光栅图像恢复算法通常通过失真度量(例如PSNR、SSIM)和感知质量度量(例如FID、NIQE)进行评估。目标是尽可能达到最低的失真,同时不妥协感知质量。为了达到这个目标,当前的方法通常尝试从后验分布中进行采样,或优化失真损失(例如MSE)和感知质量损失的加权和(例如GAN)。不同于以前的工作,本文专注于在完美感知指数约束下最小化MSE的最优估计器,即在重建图像的分布与真实图像的分布相等的情况下。最近的理论结果表明,可以通过最优传输后验均值预测(MMSE估计)到真实图像的分布来构建这样的估计器。受此结果的启发,我们引入了后均值校正流(PMRF),这是一种简单而高效算法,可以近似这种最优估计器。特别是,PMRF首先预测后验均值,然后使用校正流模型将结果传输到高质量图像,该模型近似于所需的最佳传输映射。我们研究了PMRF的理论效用,并证明它在各种图像恢复任务上始终优于以前的方法。
论文及项目相关链接
PDF Accepted to ICLR 2025. Code and demo are available at https://pmrf-ml.github.io/
Summary
本文关注于在完美感知指数约束下最小化均方误差的最优估计器。在保持感知质量的同时实现最低的失真一直是图像修复算法的关键目标。为达到此目标,本文通过理论结果证明存在一个最优传输器可以将后验均值预测(MMSE估计)传输到真实图像分布。受这一结果的启发,本文提出了一种简单有效的算法——后验均值校正流(PMRF)。该算法首先预测后验均值,然后通过近似理想传输图的校正流模型将结果传送到高质量图像。研究显示,该方法在各种图像修复任务上的性能优于先前的方法。
Key Takeaways
- 图像修复算法的评价通常基于失真和感知质量两个标准,旨在实现最低失真并保持感知质量。
- 现有方法尝试从后验分布采样或优化失真损失和感知质量损失的加权和。
- 本文专注于在完美感知指数约束下最小化均方误差的最优估计器。
- 一个理论结果显示,存在一种最优传输器能将后验均值预测传输到真实图像分布。
- 受此理论结果的启发,提出了后验均值校正流(PMRF)算法。
- PMRF首先预测后验均值,然后通过校正流模型将结果优化为高质量图像。
点此查看论文截图
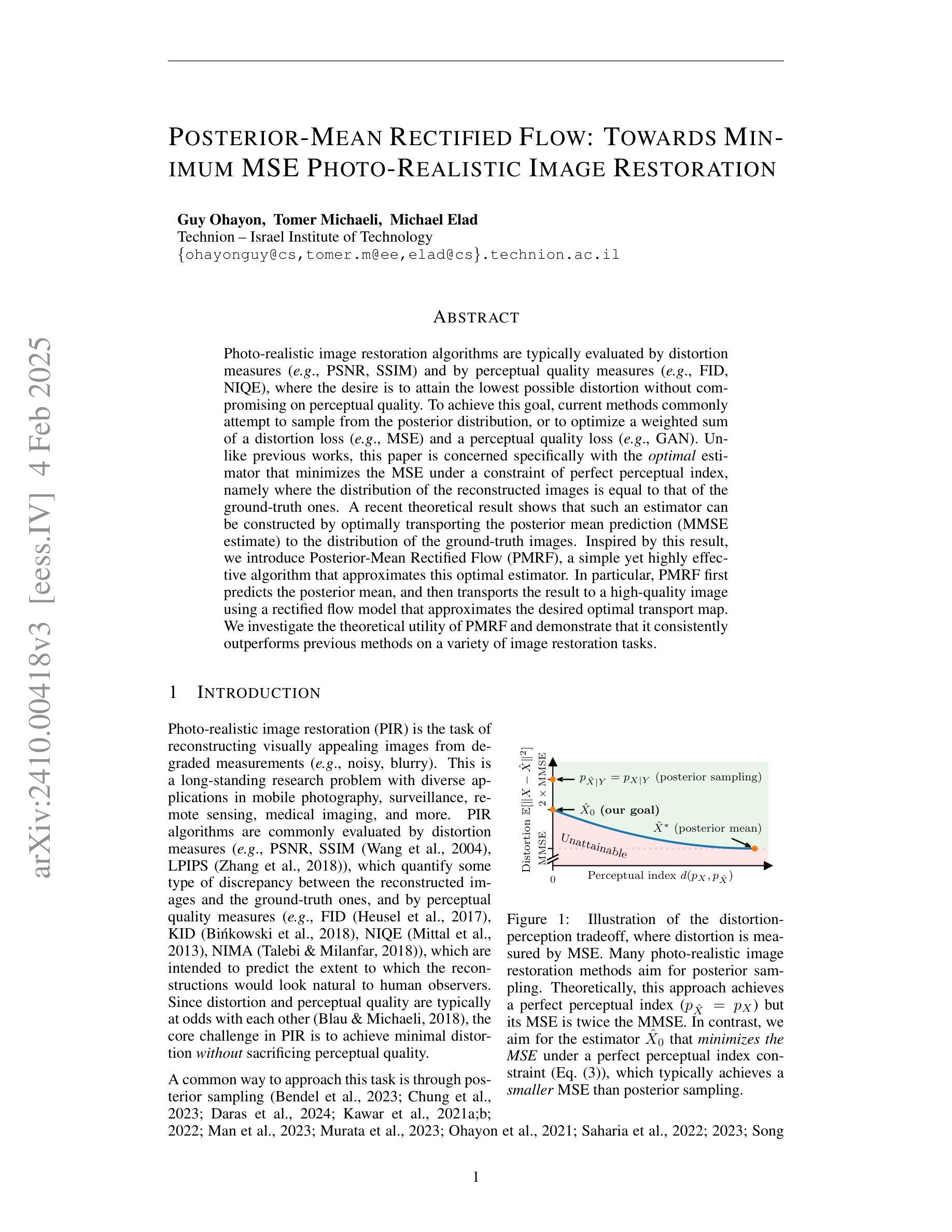
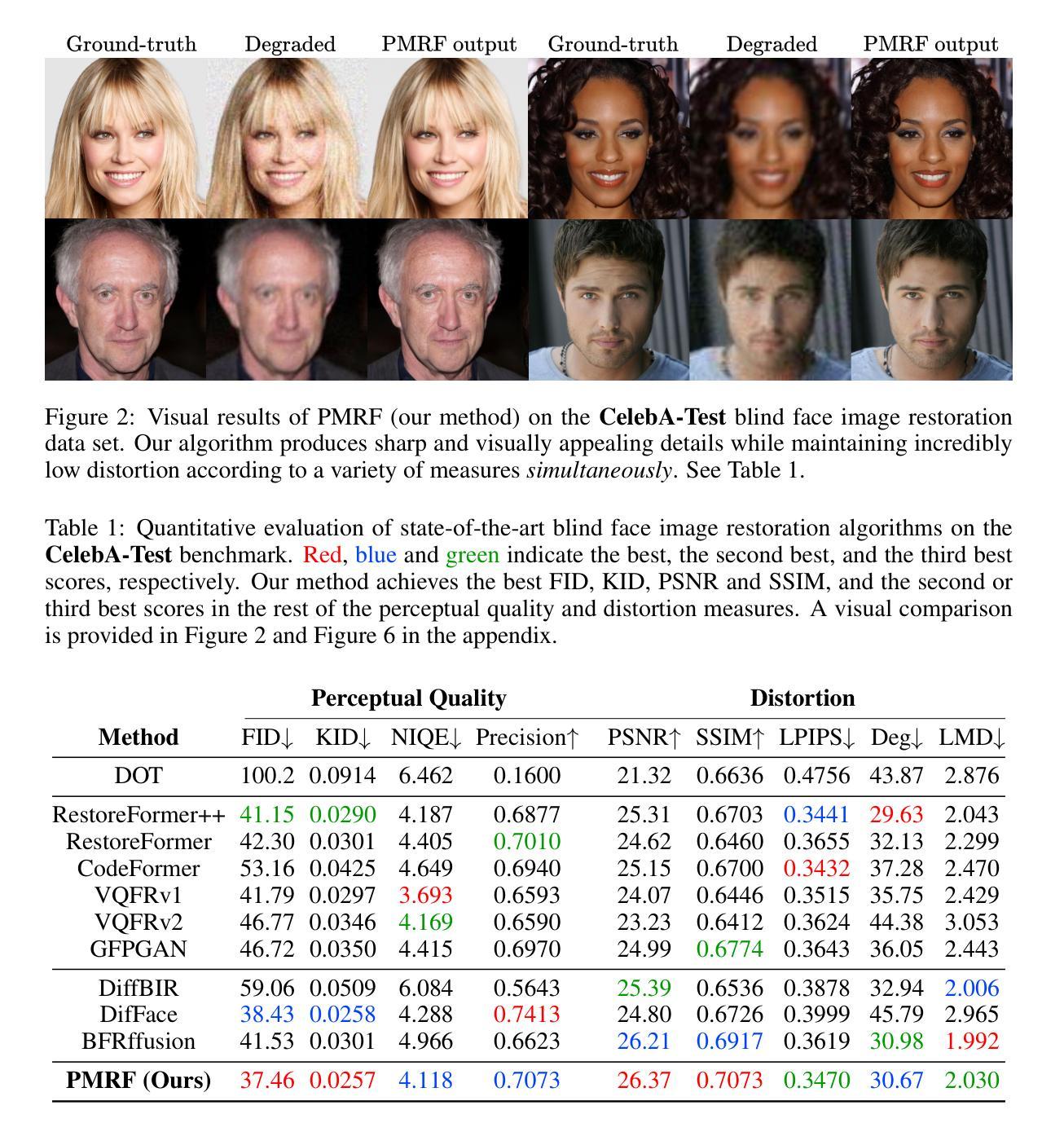