⚠️ 以下所有内容总结都来自于 大语言模型的能力,如有错误,仅供参考,谨慎使用
🔴 请注意:千万不要用于严肃的学术场景,只能用于论文阅读前的初筛!
💗 如果您觉得我们的项目对您有帮助 ChatPaperFree ,还请您给我们一些鼓励!⭐️ HuggingFace免费体验
2025-02-06 更新
Design and Simulation of the Adaptive Continuous Entanglement Generation Protocol
Authors:Caitao Zhan, Joaquin Chung, Allen Zang, Alexander Kolar, Rajkumar Kettimuthu
Generating and distributing remote entangled pairs (EPs) is the most important responsibility of quantum networks, because entanglement serves as the fundamental resource for important quantum networks applications. A key performance metric for quantum networks is the time-to-serve (TTS) for users’ EP requests, which is the time to distribute EPs between the requesting users. Reducing the TTS is critically important given the limited qubit coherence time. In this paper, we study the Adaptive Continuous entanglement generation Protocol (ACP), which enables quantum network nodes to continuously generate EPs with their neighbors, while adaptively selecting the neighbors to reduce the TTS. Meanwhile, entanglement purification is used to mitigate the idling decoherence of the EPs generated by the ACP prior to the arrival user requests. We extend the capability of the SeQUeNCe simulator to allow the implementation of the ACP with full support. Then through extensive simulations, we evaluate the ACP at different network scales, demonstrating significant improvements in both the TTS (up to 94% decrease) and the fidelity (up to 0.05 increase) of distributed entanglement.
生成和分发远程纠缠对(EPs)是量子网络最重要的职责之一,因为纠缠是重要量子网络应用的基本资源。量子网络的关键性能指标是用户EP请求的服务时间(TTS),即向请求用户分发EP的时间。鉴于有限的量子比特相干时间,减少TTS至关重要。本文研究了自适应连续纠缠生成协议(ACP),该协议使量子网络节点能够与其邻居持续生成EPs,同时自适应选择邻居以减少TTS。同时,使用纠缠净化来缓解由ACP生成的EPs在到达用户请求之前的空闲退相干。我们扩展了SeQUeNCe模拟器的功能,以允许完全支持实施ACP。然后通过大量模拟,我们在不同的网络规模上评估了ACP,显示出分布式纠缠的TTS(减少高达94%)和保真度(提高高达0.05)的显著改善。
论文及项目相关链接
PDF 8 pages, 10 figures, accepted at QCNC 2025
总结
本文研究了量子网络中自适应连续纠缠生成协议(ACP),该协议使量子网络节点能够与其邻居连续生成纠缠对,并自适应选择邻居以减少服务时间。该协议还采用纠缠纯化来缓解ACP生成的纠缠对的空闲消相干,直到用户请求到达。通过广泛的模拟,证明ACP在不同网络规模下的服务时间(TTS)和纠缠保真度均有显著提高,TTS最多可降低94%,保真度最多可提高0.05。
关键见解
- 量子网络中生成和分发远程纠缠对(EPs)是最重要的职责,因为纠缠是重要量子网络应用的基本资源。
- 服务时间(TTS)是量子网络的关键性能指标,它是用户纠缠对请求之间的分发时间。
- 由于有限的量子比特相干时间,减少TTS至关重要。
- 自适应连续纠缠生成协议(ACP)允许量子网络节点与其邻居连续生成EPs,并自适应选择邻居以减少TTS。
- ACP使用纠缠纯化来缓解生成的纠缠对的空闲消相干。
- 通过模拟证明ACP在减少TTS和提高纠缠分布质量方面效果显著。具体来说,最多可以减少高达94%的TTS并提高高达0.05的纠缠保真度。
点此查看论文截图
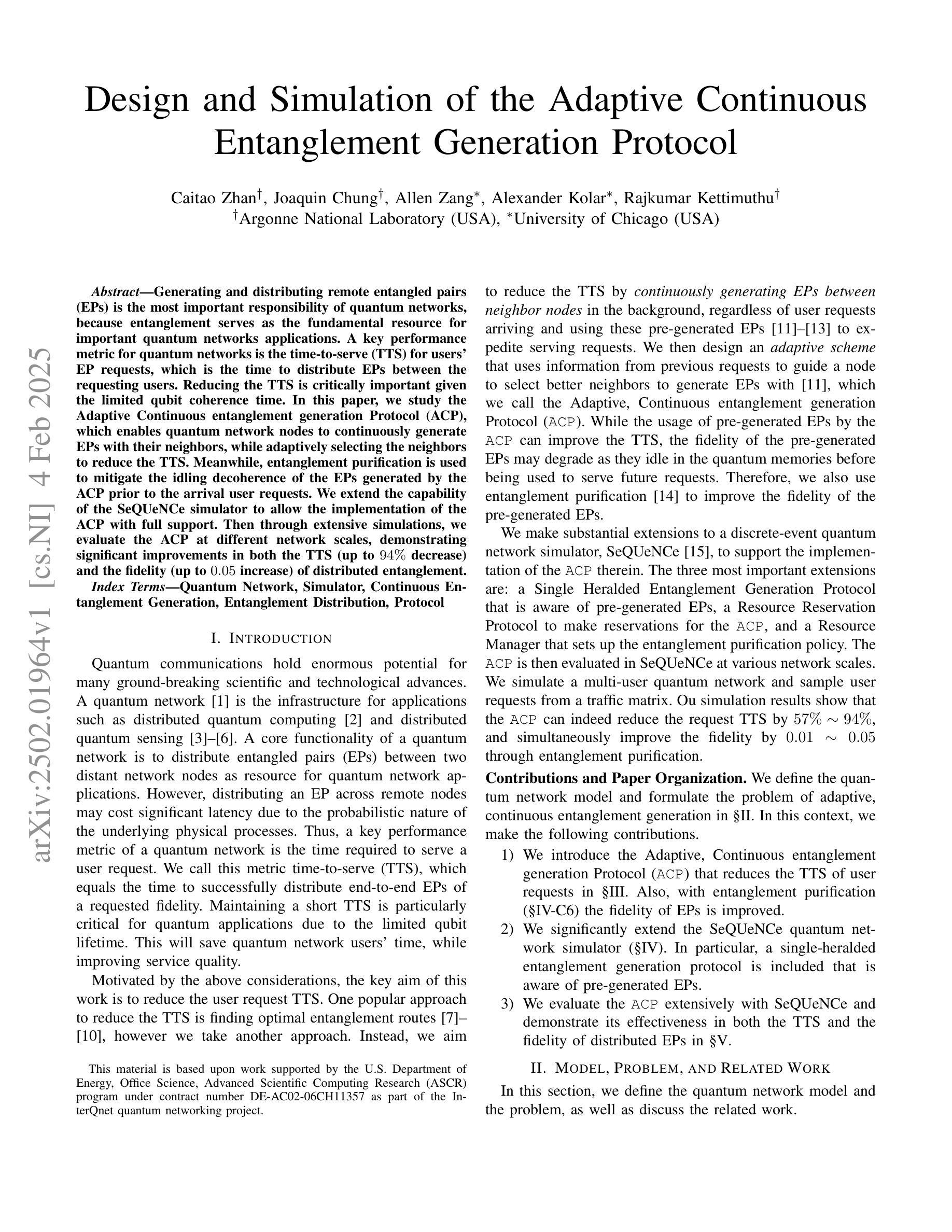
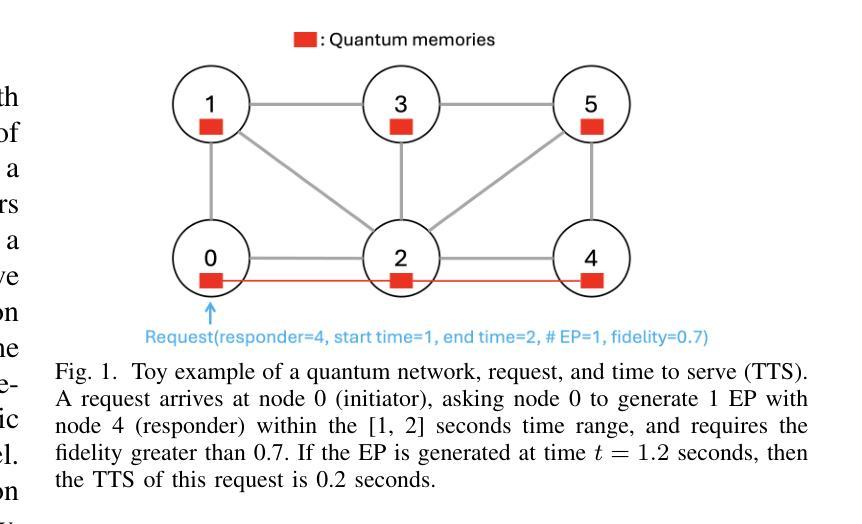
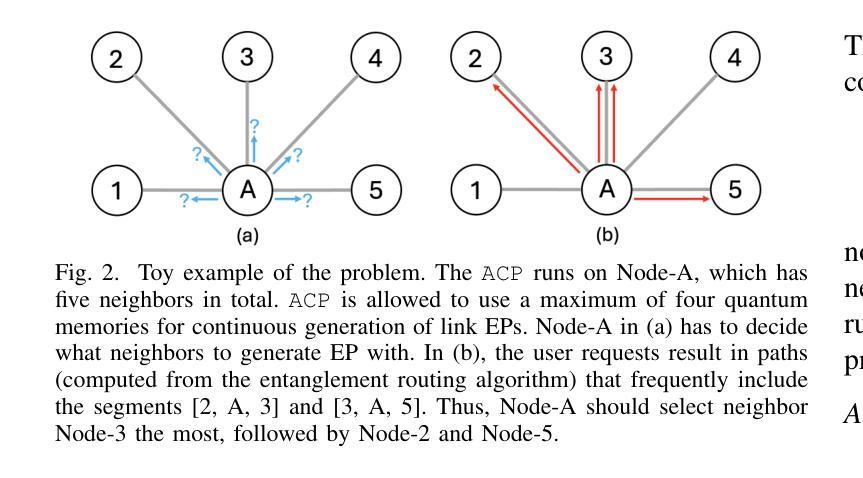
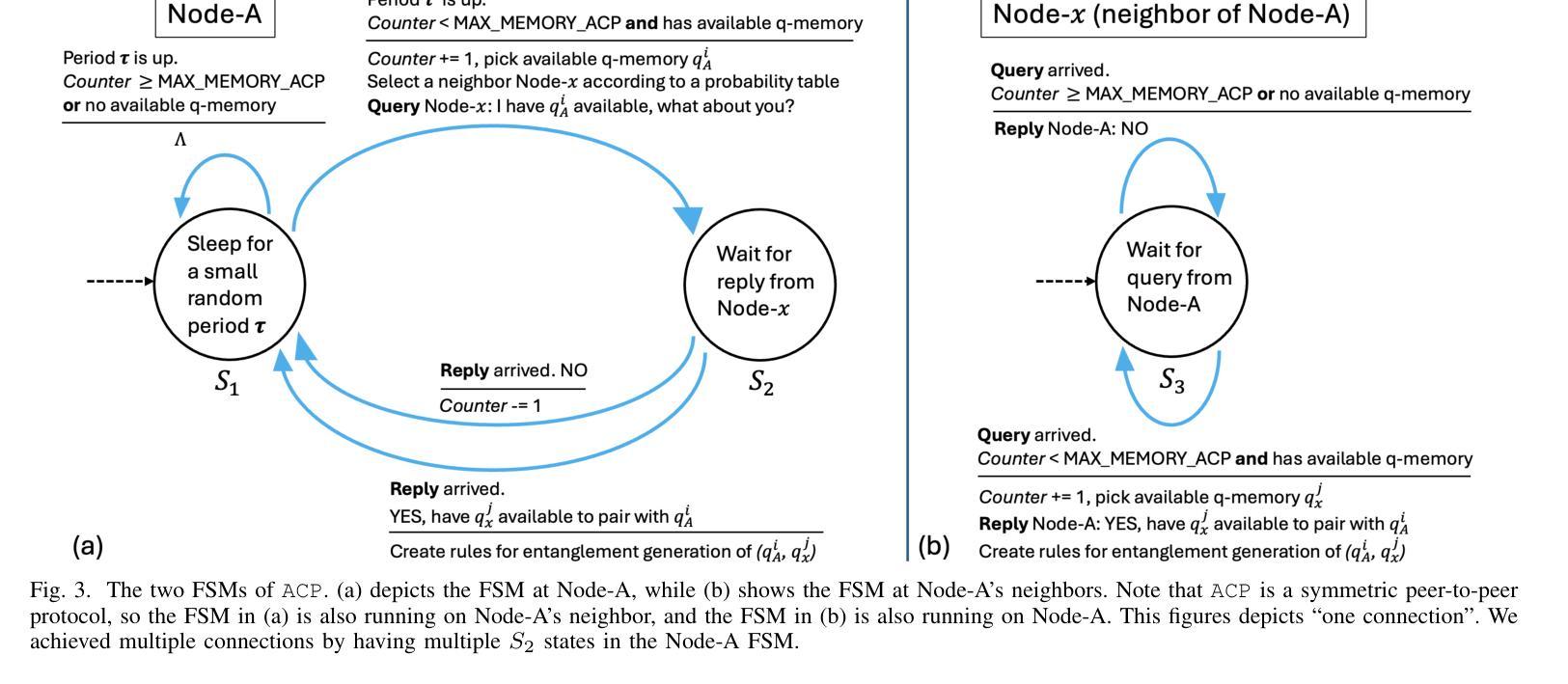
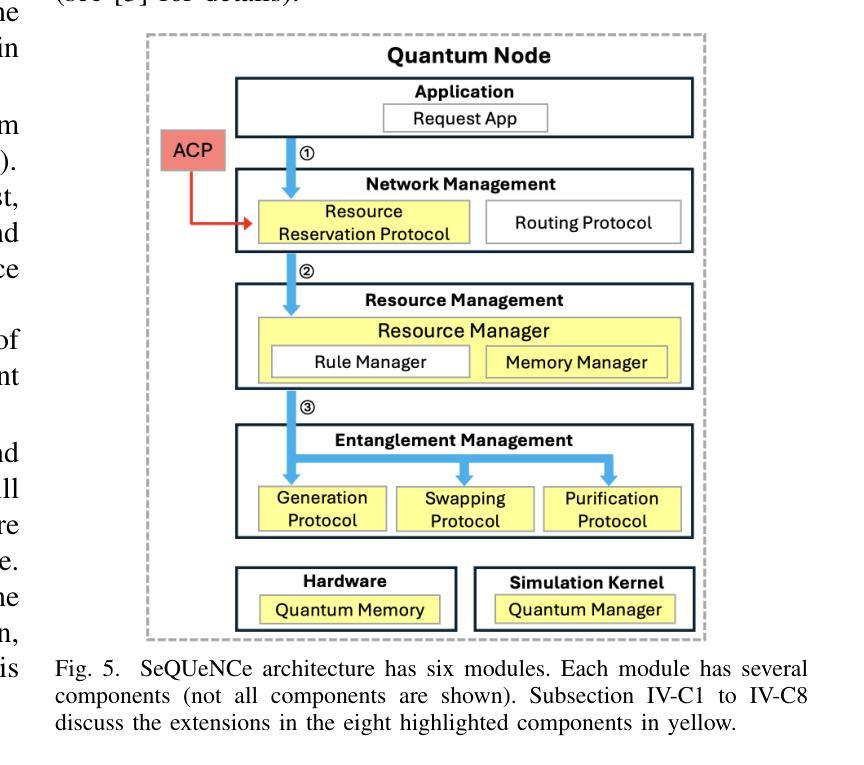
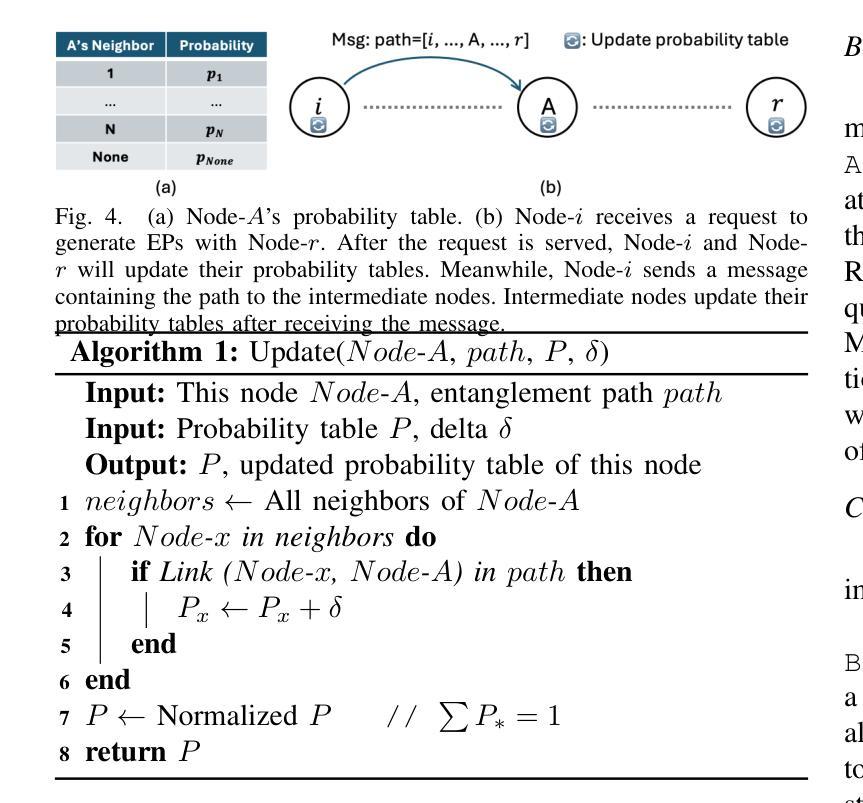
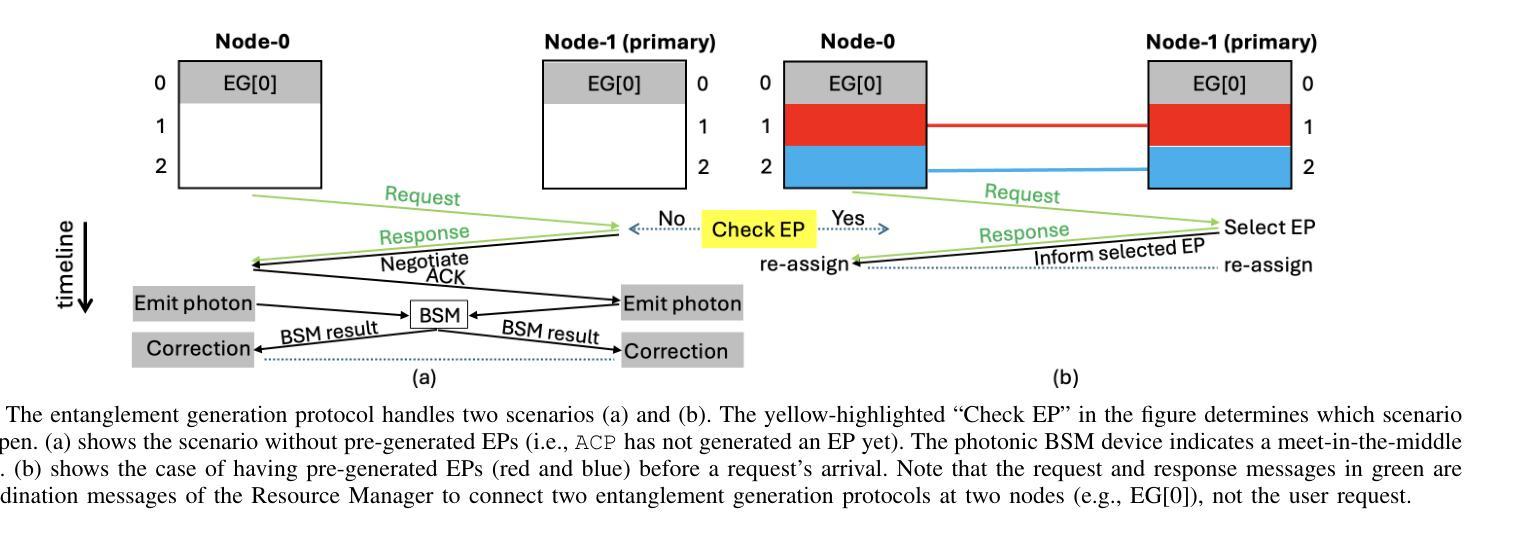
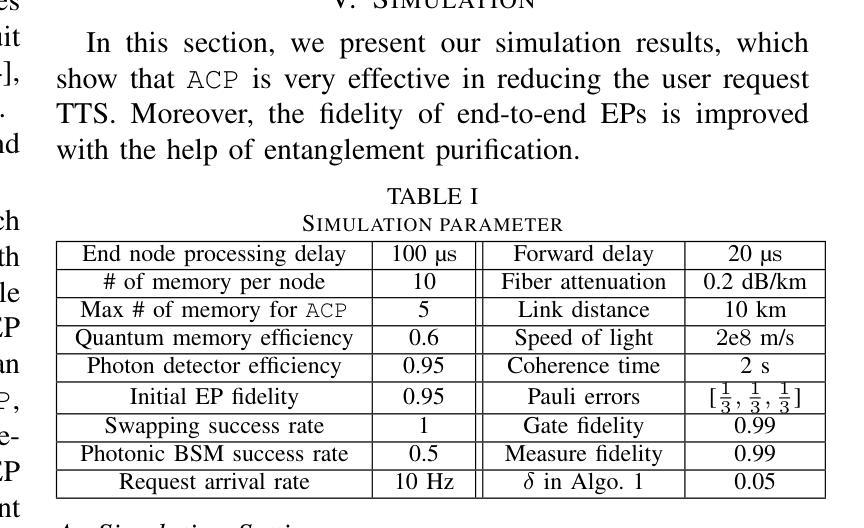
Continuous Autoregressive Modeling with Stochastic Monotonic Alignment for Speech Synthesis
Authors:Weiwei Lin, Chenghan He
We propose a novel autoregressive modeling approach for speech synthesis, combining a variational autoencoder (VAE) with a multi-modal latent space and an autoregressive model that uses Gaussian Mixture Models (GMM) as the conditional probability distribution. Unlike previous methods that rely on residual vector quantization, our model leverages continuous speech representations from the VAE’s latent space, greatly simplifying the training and inference pipelines. We also introduce a stochastic monotonic alignment mechanism to enforce strict monotonic alignments. Our approach significantly outperforms the state-of-the-art autoregressive model VALL-E in both subjective and objective evaluations, achieving these results with only 10.3% of VALL-E’s parameters. This demonstrates the potential of continuous speech language models as a more efficient alternative to existing quantization-based speech language models. Sample audio can be found at https://tinyurl.com/gmm-lm-tts.
我们提出了一种用于语音合成的新型自回归建模方法,该方法结合了变分自编码器(VAE)的多模态潜在空间和一个使用高斯混合模型(GMM)作为条件概率分布的自回归模型。与之前依赖于残差向量量化的方法不同,我们的模型利用VAE潜在空间中的连续语音表示,从而极大地简化了训练和推理流程。我们还引入了一种随机单调对齐机制来执行严格的单调对齐。我们的方法在主观和客观评估上都显著优于最先进的自回归模型VALL-E,并且仅使用VALL-E的1.03%参数就能达到这些结果。这证明了连续语音语言模型作为现有基于量化的语音语言模型的更高效替代方案的潜力。示例音频可在https://tinyurl.com/gmm-lm-tts找到。
论文及项目相关链接
PDF ICLR 2025
Summary
本文提出了一种结合变分自编码器(VAE)和多模态潜在空间的自回归建模方法,用于语音合成。该方法使用高斯混合模型(GMM)作为条件概率分布,并引入随机单调对齐机制来强制执行严格的对齐。该方法在主观和客观评估上都显著优于现有的自回归模型VALL-E,并且使用的参数仅为VALL-E的10.3%,展现了连续语音语言模型作为现有量化语音语言模型的更高效替代方案的潜力。
Key Takeaways
- 本文提出了一种新颖的自回归建模方法,结合了变分自编码器(VAE)和多模态潜在空间用于语音合成。
- 该方法使用高斯混合模型(GMM)作为条件概率分布。
- 引入连续语音表示,简化了训练和推理流程。
- 采用了随机单调对齐机制以确保严格的对齐。
- 在主观和客观评估中,该方法显著优于当前先进的自回归模型VALL-E。
- 该方法使用的参数仅为VALL-E的10.3%。
- 研究展示了连续语音语言模型的潜力,可作为量化语音语言模型的更高效替代方案。
点此查看论文截图
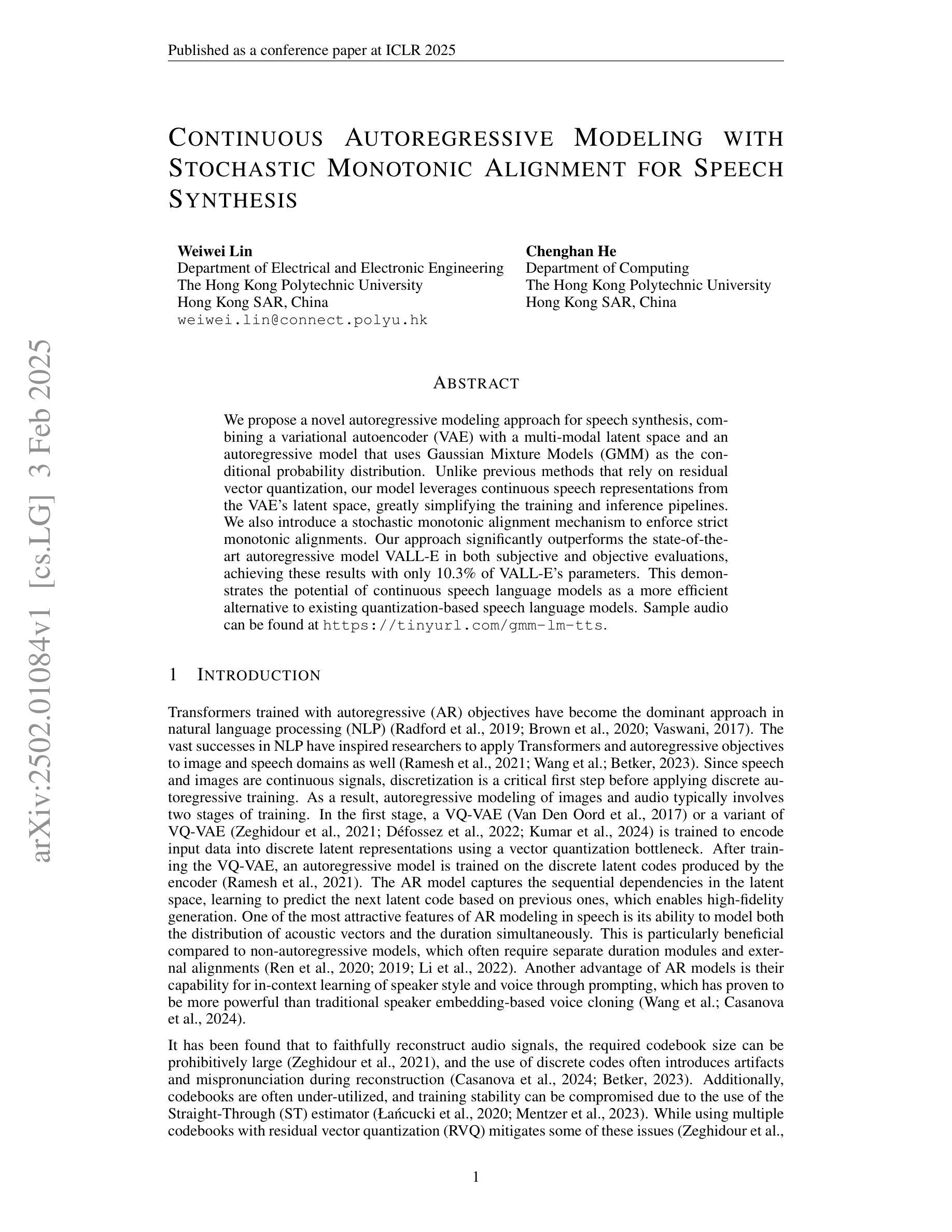
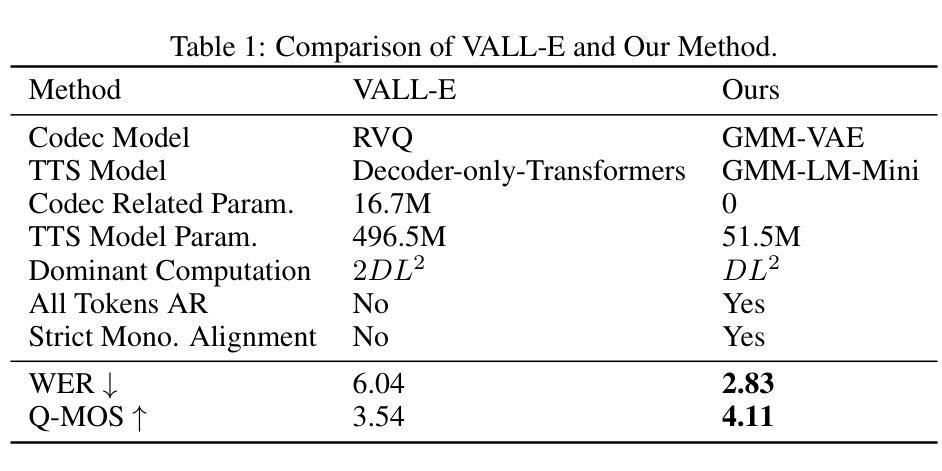
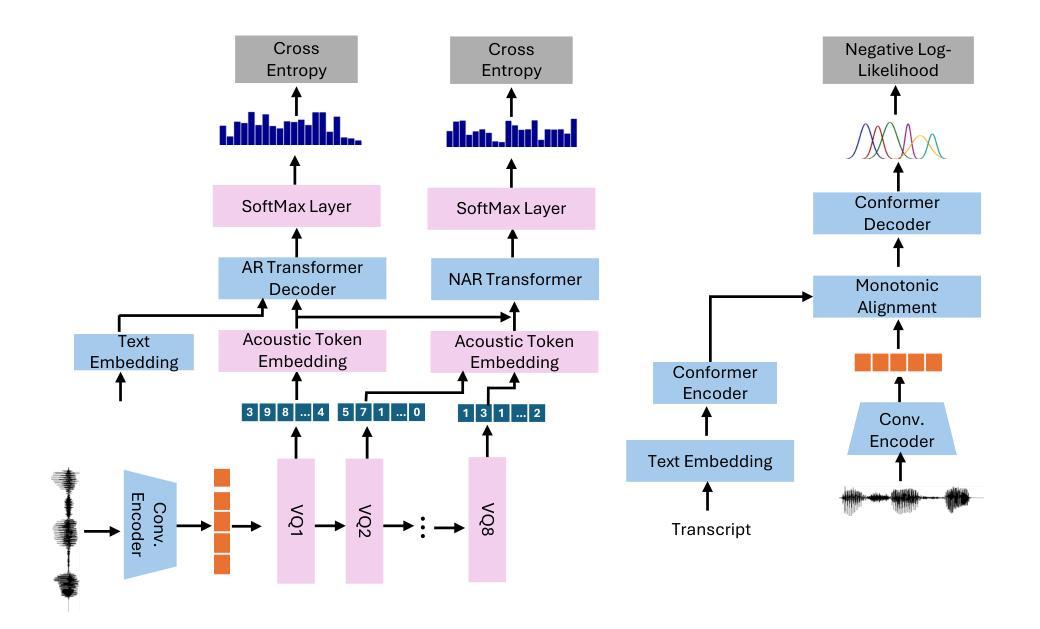
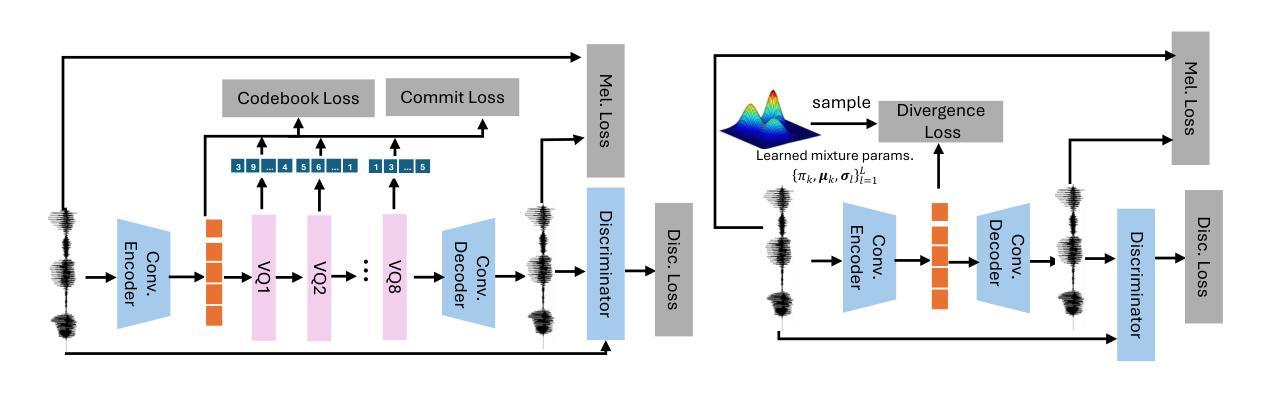
EmoTalkingGaussian: Continuous Emotion-conditioned Talking Head Synthesis
Authors:Junuk Cha, Seongro Yoon, Valeriya Strizhkova, Francois Bremond, Seungryul Baek
3D Gaussian splatting-based talking head synthesis has recently gained attention for its ability to render high-fidelity images with real-time inference speed. However, since it is typically trained on only a short video that lacks the diversity in facial emotions, the resultant talking heads struggle to represent a wide range of emotions. To address this issue, we propose a lip-aligned emotional face generator and leverage it to train our EmoTalkingGaussian model. It is able to manipulate facial emotions conditioned on continuous emotion values (i.e., valence and arousal); while retaining synchronization of lip movements with input audio. Additionally, to achieve the accurate lip synchronization for in-the-wild audio, we introduce a self-supervised learning method that leverages a text-to-speech network and a visual-audio synchronization network. We experiment our EmoTalkingGaussian on publicly available videos and have obtained better results than state-of-the-arts in terms of image quality (measured in PSNR, SSIM, LPIPS), emotion expression (measured in V-RMSE, A-RMSE, V-SA, A-SA, Emotion Accuracy), and lip synchronization (measured in LMD, Sync-E, Sync-C), respectively.
基于3D高斯涂抹技术的说话人头部合成近期因其能够实时生成高保真图像而受到关注。然而,由于其通常仅在缺乏面部情绪多样性的短视频上进行训练,所生成的说话人头部在表达广泛情绪方面存在困难。为了解决这个问题,我们提出了一种唇形对齐的情感面部生成器,并利用它来训练我们的EmoTalkingGaussian模型。该模型能够在连续的情绪值(即效价和激活)条件下操作面部情绪,同时保持与输入音频的唇部运动同步。此外,为了实现野外音频的精确唇部同步,我们引入了一种自我监督的学习方法,该方法利用文本到语音网络和视觉-音频同步网络。我们在公开视频上测试了EmoTalkingGaussian,在图像质量(以PSNR、SSIM、LPIPS衡量)、情感表达(以V-RMSE、A-RMSE、V-SA、A-SA、情感准确率衡量)和唇部同步(以LMD、Sync-E、Sync-C衡量)等方面获得了比现有技术更好的结果。
论文及项目相关链接
PDF 22 pages
Summary
三维高斯描点技术的说话人头合成法因其实时推理速度和高保真图像渲染能力而受到关注。但由于其通常在缺乏面部表情多样性的短视频上训练,生成的说话头难以表达多种情绪。为解决此问题,我们提出了唇对齐情感面部生成器并训练了EmoTalkingGaussian模型,该模型可以根据连续的情感值(如效价和激活)操纵面部表情,同时保持与输入音频的唇部运动同步。此外,我们还引入了一种自我监督学习方法,通过文字转语音网络和视听同步网络来实现对野生音频的精确唇部同步。在公开视频上的实验表明,EmoTalkingGaussian的图像质量、情感表达和唇部同步性能均优于现有技术。
Key Takeaways
- 三维高斯描点技术用于说话人头合成,具备实时推理速度和高保真图像渲染能力。
- 现有方法因训练数据缺乏面部表情多样性,难以表达多种情绪。
- 提出唇对齐情感面部生成器,用以训练EmoTalkingGaussian模型。
- EmoTalkingGaussian模型可以根据连续的情感值操纵面部表情。
- EmoTalkingGaussian模型保持与输入音频的唇部运动同步。
- 引入自我监督学习方法,通过文字转语音网络和视听同步网络实现精确唇部同步。
点此查看论文截图
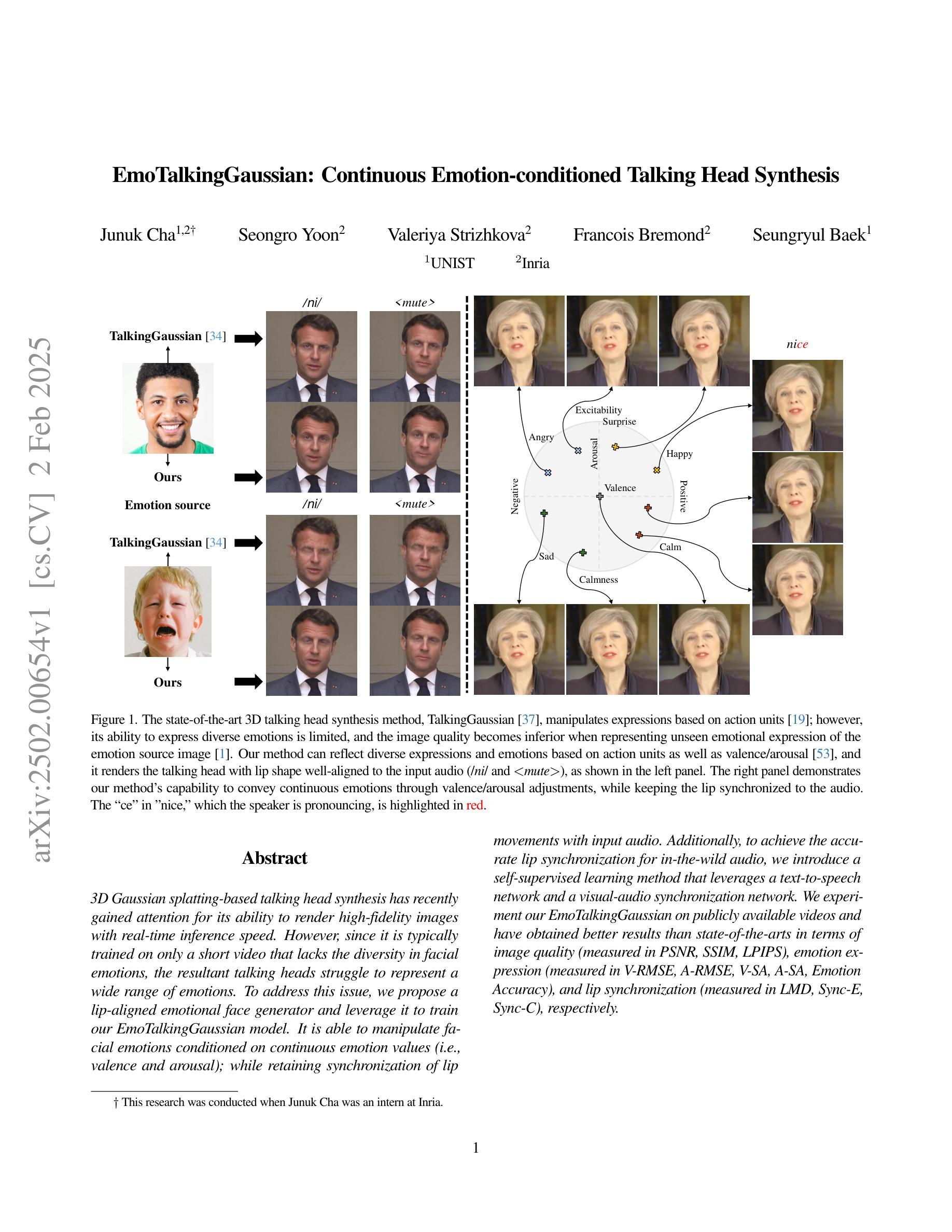
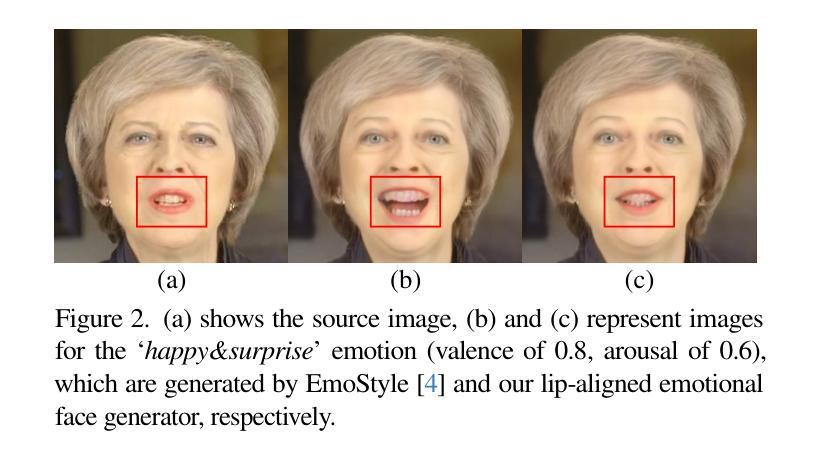
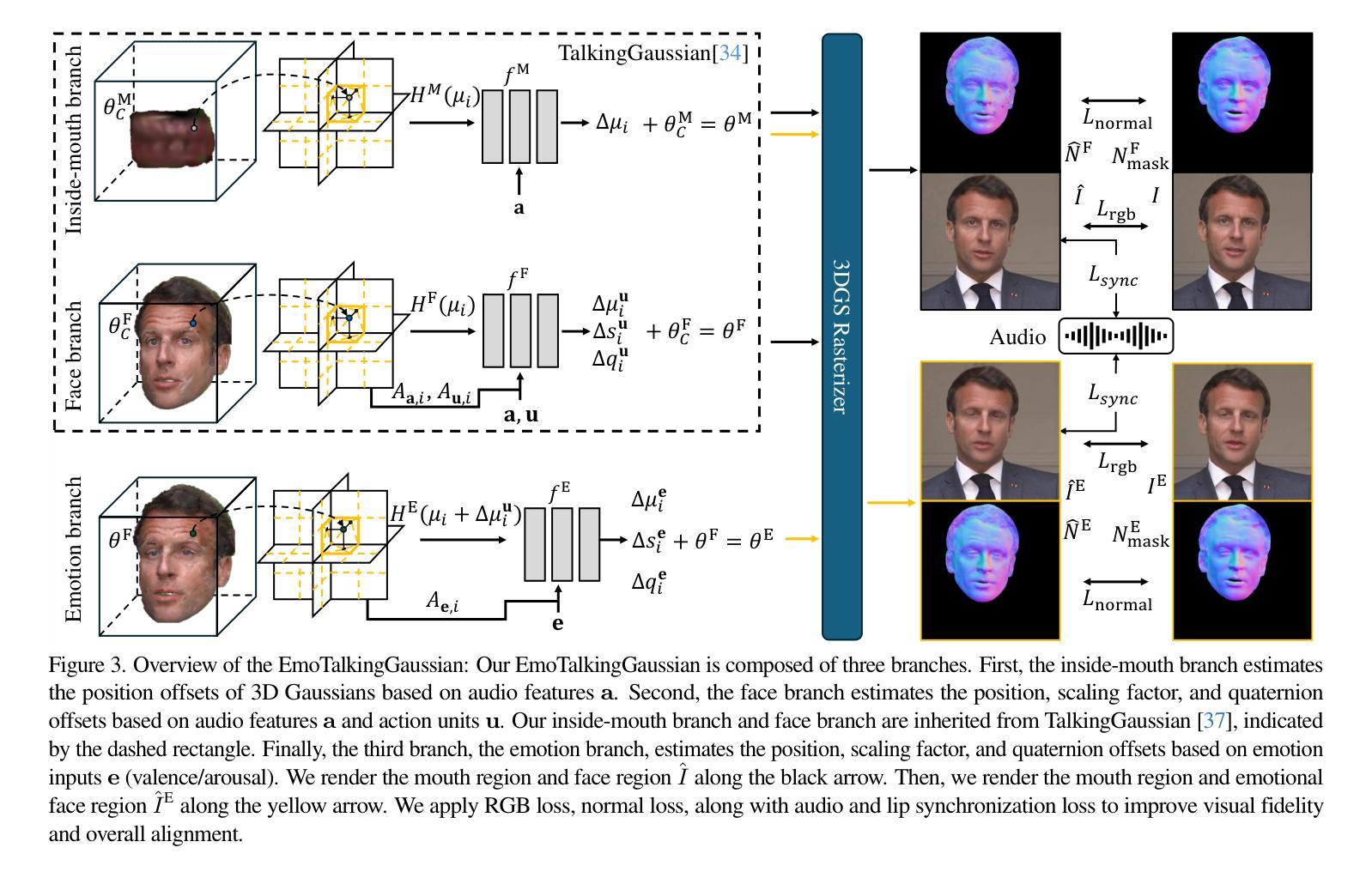
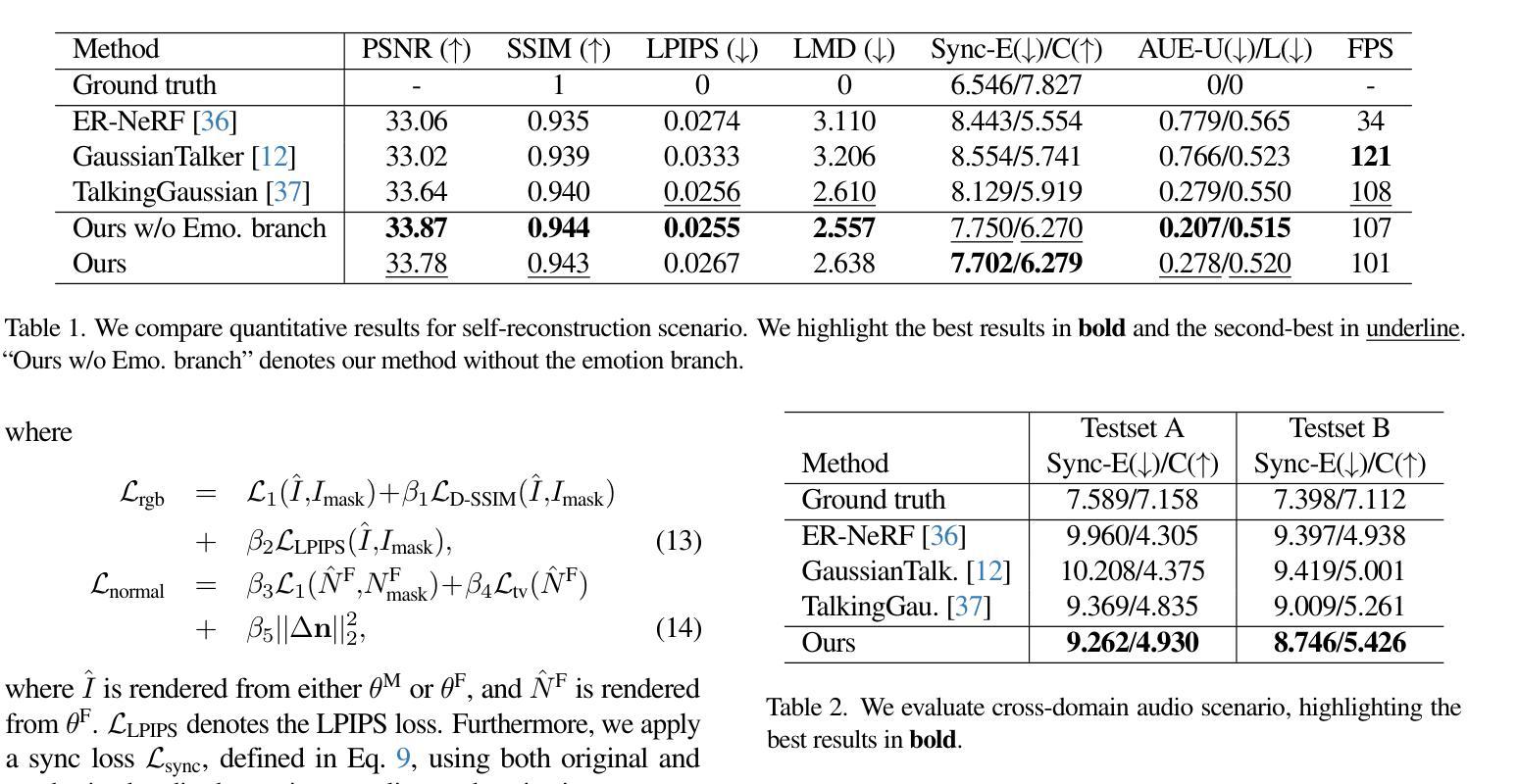
The Codec Language Model-based Zero-Shot Spontaneous Style TTS System for CoVoC Challenge 2024
Authors:Shuoyi Zhou, Yixuan Zhou, Weiqin Li, Jun Chen, Runchuan Ye, Weihao Wu, Zijian Lin, Shun Lei, Zhiyong Wu
This paper describes the zero-shot spontaneous style TTS system for the ISCSLP 2024 Conversational Voice Clone Challenge (CoVoC). We propose a LLaMA-based codec language model with a delay pattern to achieve spontaneous style voice cloning. To improve speech intelligibility, we introduce the Classifier-Free Guidance (CFG) strategy in the language model to strengthen conditional guidance on token prediction. To generate high-quality utterances, we adopt effective data preprocessing operations and fine-tune our model with selected high-quality spontaneous speech data. The official evaluations in the CoVoC constrained track show that our system achieves the best speech naturalness MOS of 3.80 and obtains considerable speech quality and speaker similarity results.
本文介绍了针对ISCSLP 2024对话式语音克隆挑战赛(CoVoC)的零样本自发风格TTS系统。我们提出了一种基于LLaMA的编码语言模型,采用延迟模式来实现自发风格语音克隆。为了提高语音清晰度,我们在语言模型中引入了无分类器引导(CFG)策略,以加强条件引导在令牌预测方面的作用。为了生成高质量的语句,我们采用了有效的数据预处理操作,并利用精选的高质量自发语音数据对模型进行了微调。在CoVoC约束轨道的官方评估中,我们的系统达到了最佳语音自然度MOS 3.80,并获得了显著的语音质量和说话人相似性结果。
论文及项目相关链接
PDF Accepted by ISCSLP 2024
Summary
本文介绍了针对ISCSLP 2024对话式语音克隆挑战(CoVoC)的零样本自发风格TTS系统。研究团队采用基于LLaMA的编码语言模型,通过延迟模式实现自发风格语音克隆。为提高语音清晰度,研究团队在模型中引入无分类器引导策略(CFG),强化标记预测的条件引导。通过有效数据预处理操作及高质量自发语音数据的微调,生成高质量话语。在CoVoC约束轨道的官方评估中,该系统获得最佳语音自然度MOS分数为3.80,在语音质量和说话人相似性方面也表现出优异结果。
Key Takeaways
- 采用LLaMA编码语言模型实现零样本自发风格TTS系统。
- 通过延迟模式实现自发风格语音克隆。
- 引入无分类器引导策略(CFG)提升语音清晰度及模型的预测准确性。
- 实施有效的数据预处理操作和采用高质量自发语音数据对模型进行微调。
- 系统在CoVoC约束轨道测试中展现出优秀的语音自然度表现,获得最高MOS分数。
- 系统的语音质量和说话人相似性评估表现显著。
点此查看论文截图
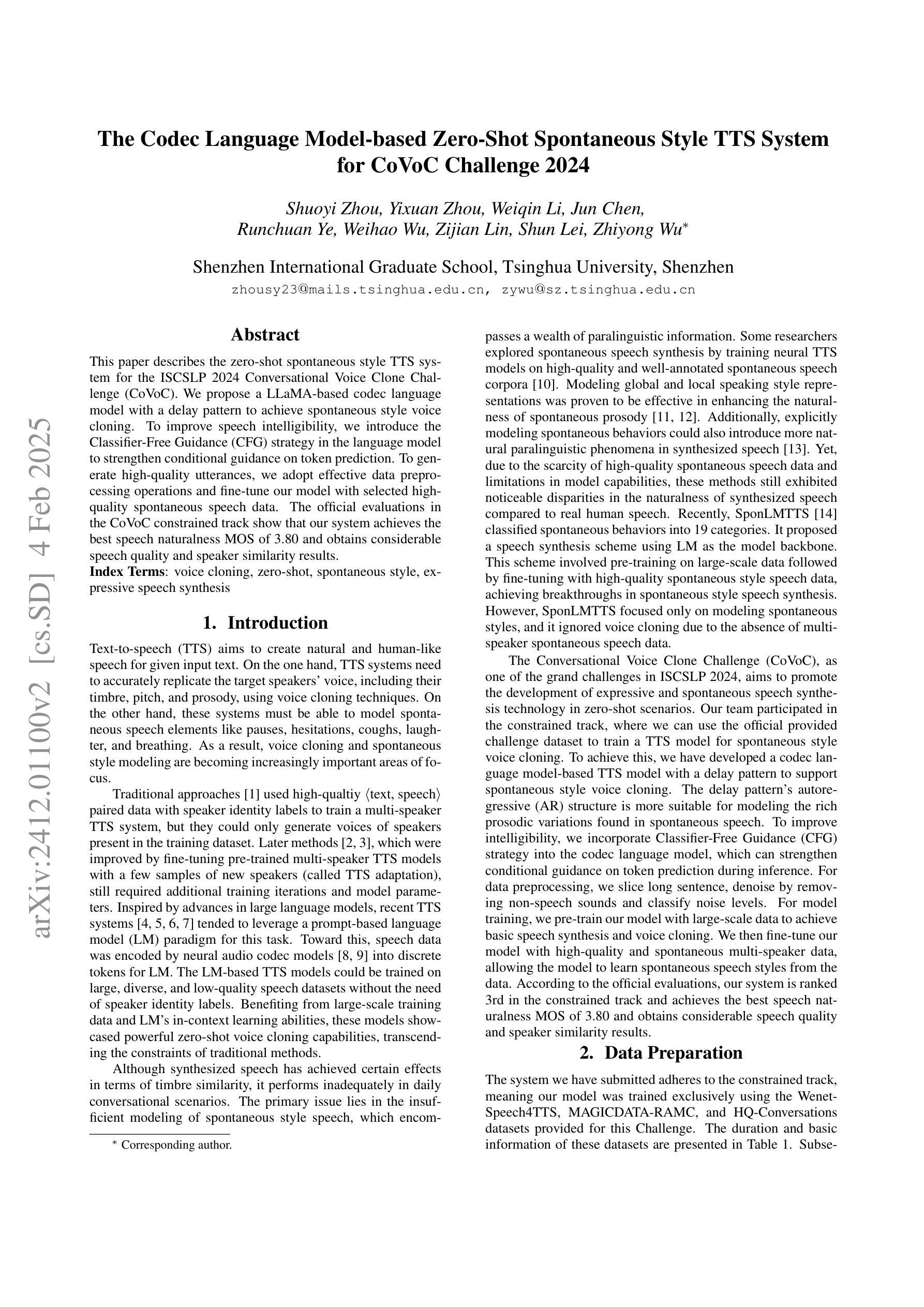
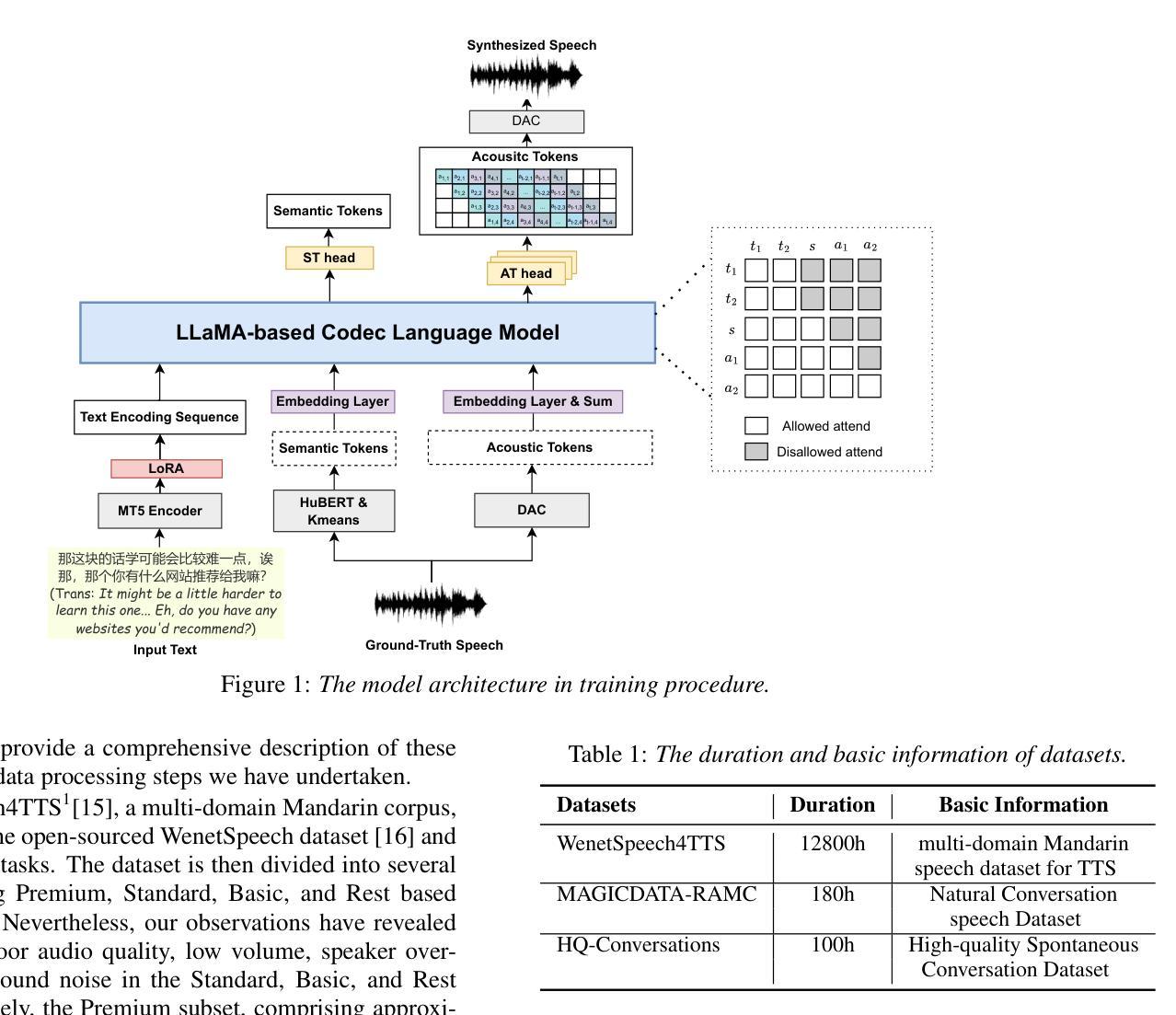
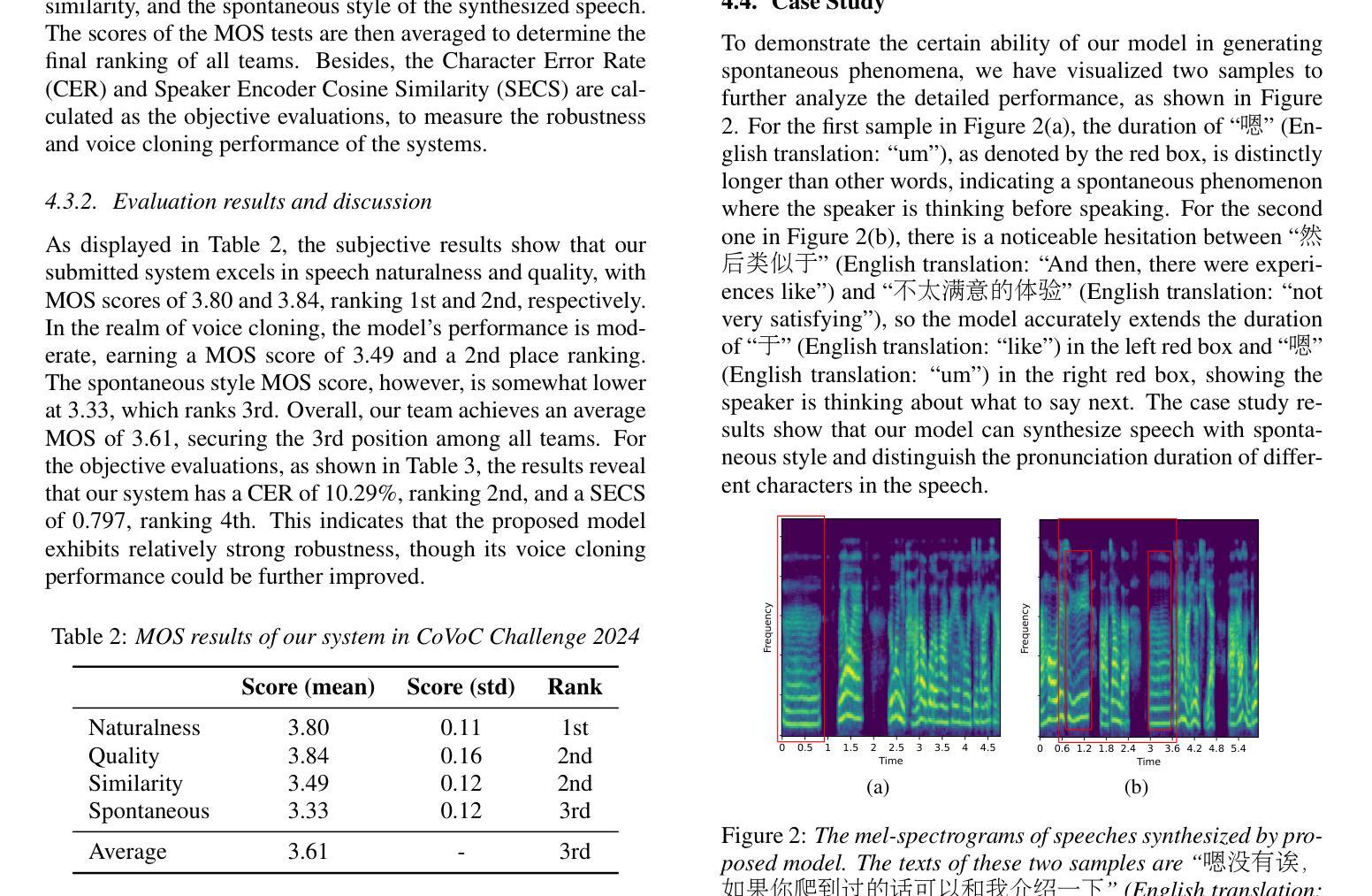
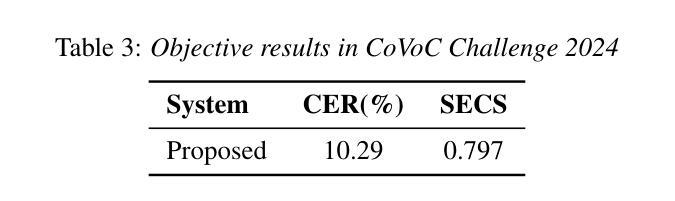