⚠️ 以下所有内容总结都来自于 大语言模型的能力,如有错误,仅供参考,谨慎使用
🔴 请注意:千万不要用于严肃的学术场景,只能用于论文阅读前的初筛!
💗 如果您觉得我们的项目对您有帮助 ChatPaperFree ,还请您给我们一些鼓励!⭐️ HuggingFace免费体验
2025-02-06 更新
OmniHuman-1: Rethinking the Scaling-Up of One-Stage Conditioned Human Animation Models
Authors:Gaojie Lin, Jianwen Jiang, Jiaqi Yang, Zerong Zheng, Chao Liang
End-to-end human animation, such as audio-driven talking human generation, has undergone notable advancements in the recent few years. However, existing methods still struggle to scale up as large general video generation models, limiting their potential in real applications. In this paper, we propose OmniHuman, a Diffusion Transformer-based framework that scales up data by mixing motion-related conditions into the training phase. To this end, we introduce two training principles for these mixed conditions, along with the corresponding model architecture and inference strategy. These designs enable OmniHuman to fully leverage data-driven motion generation, ultimately achieving highly realistic human video generation. More importantly, OmniHuman supports various portrait contents (face close-up, portrait, half-body, full-body), supports both talking and singing, handles human-object interactions and challenging body poses, and accommodates different image styles. Compared to existing end-to-end audio-driven methods, OmniHuman not only produces more realistic videos, but also offers greater flexibility in inputs. It also supports multiple driving modalities (audio-driven, video-driven and combined driving signals). Video samples are provided on the ttfamily project page (https://omnihuman-lab.github.io)
近年来,端到端的人脸动画,如音频驱动的人脸生成,已经取得了显著的进步。然而,现有方法仍然难以扩展为大型通用视频生成模型,从而限制了它们在真实应用中的潜力。在本文中,我们提出了OmniHuman,这是一个基于Diffusion Transformer的框架,通过混合运动相关条件来扩大训练阶段的数据规模。为此,我们介绍了两种针对这些混合条件的训练原则,以及相应的模型架构和推理策略。这些设计使OmniHuman能够充分利用数据驱动的运动生成,最终实现高度逼真的人类视频生成。更重要的是,OmniHuman支持各种肖像内容(特写、肖像、半身、全身)、支持说话和唱歌、处理人与物体的互动以及具有挑战性的身体姿势,并适应不同的图像风格。与现有的端到端音频驱动方法相比,OmniHuman不仅生成更逼真的视频,而且在输入方面提供了更大的灵活性。它还支持多种驱动模式(音频驱动、视频驱动和组合驱动信号)。视频样本可以在ttfamily项目页面(https://omnihuman-lab.github.io)上找到。
论文及项目相关链接
PDF https://omnihuman-lab.github.io/
Summary
奥悯人类动画技术取得了显著进展,但仍存在规模上的挑战。本文提出OmniHuman框架,基于扩散Transformer技术,通过混合运动条件进行训练,实现高度真实感的人类视频生成。OmniHuman支持多种肖像内容、说话和唱歌、人机交互和复杂动作姿态,同时兼容不同图像风格。相较于现有端到端音频驱动方法,OmniHuman更具真实感和灵活性。
Key Takeaways
- 奥悯人类动画技术近年来取得显著进展,但仍面临规模化挑战。
- OmniHuman框架基于扩散Transformer技术,通过混合运动条件进行训练。
- OmniHuman能实现高度真实感的人类视频生成。
- OmniHuman支持多种肖像内容、说话和唱歌、人机交互和复杂动作姿态。
- OmniHuman兼容不同图像风格。
- 与现有端到端音频驱动方法相比,OmniHuman更具真实感和灵活性。
点此查看论文截图
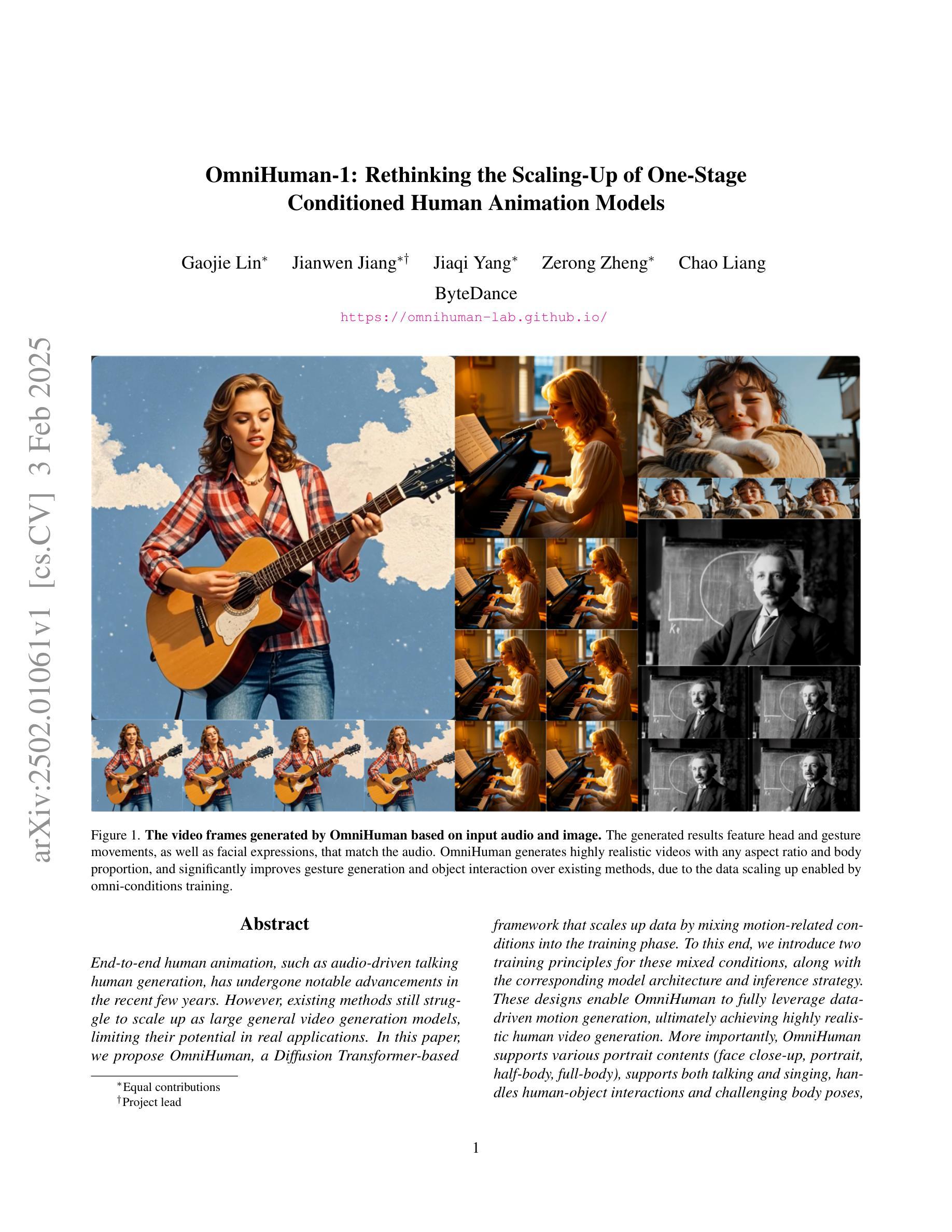
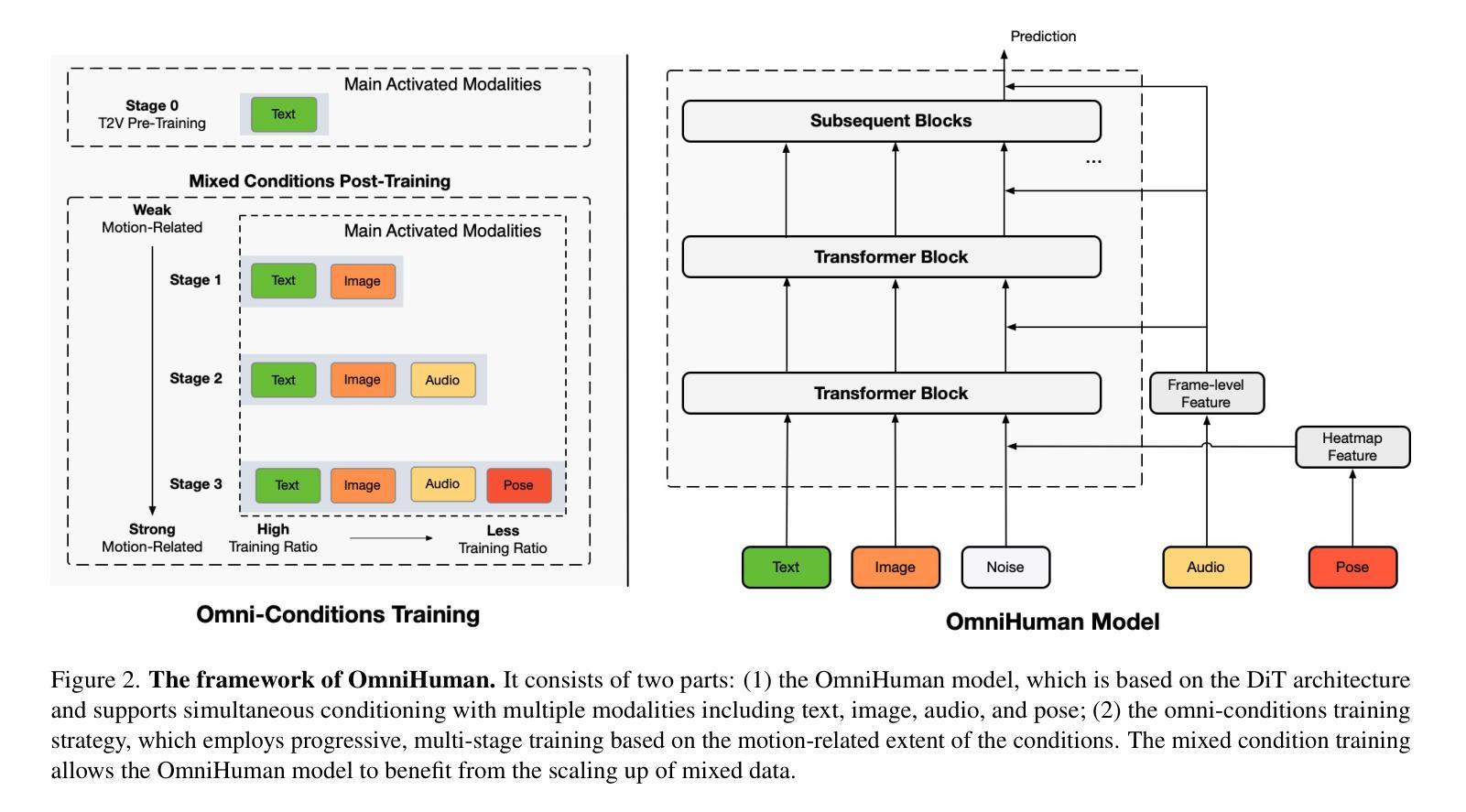
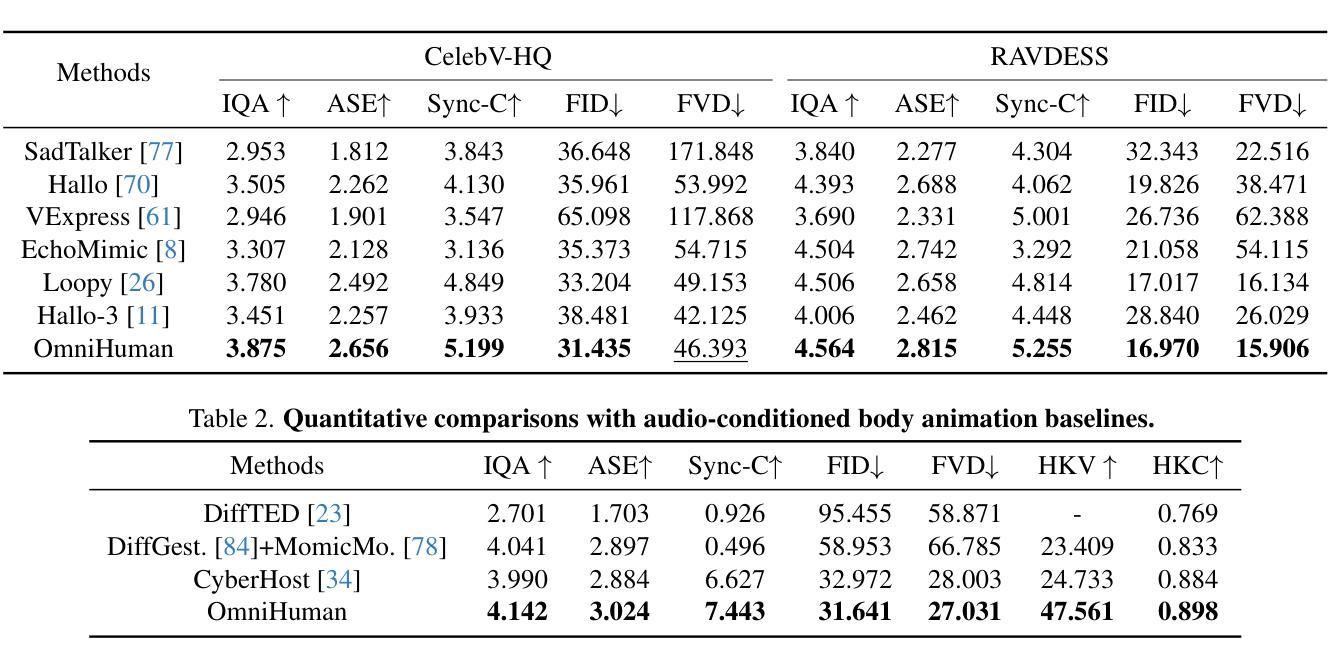

Emotional Face-to-Speech
Authors:Jiaxin Ye, Boyuan Cao, Hongming Shan
How much can we infer about an emotional voice solely from an expressive face? This intriguing question holds great potential for applications such as virtual character dubbing and aiding individuals with expressive language disorders. Existing face-to-speech methods offer great promise in capturing identity characteristics but struggle to generate diverse vocal styles with emotional expression. In this paper, we explore a new task, termed emotional face-to-speech, aiming to synthesize emotional speech directly from expressive facial cues. To that end, we introduce DEmoFace, a novel generative framework that leverages a discrete diffusion transformer (DiT) with curriculum learning, built upon a multi-level neural audio codec. Specifically, we propose multimodal DiT blocks to dynamically align text and speech while tailoring vocal styles based on facial emotion and identity. To enhance training efficiency and generation quality, we further introduce a coarse-to-fine curriculum learning algorithm for multi-level token processing. In addition, we develop an enhanced predictor-free guidance to handle diverse conditioning scenarios, enabling multi-conditional generation and disentangling complex attributes effectively. Extensive experimental results demonstrate that DEmoFace generates more natural and consistent speech compared to baselines, even surpassing speech-driven methods. Demos are shown at https://demoface-ai.github.io/.
仅凭一张富有表现力的脸,我们能对带有情感的嗓音做出多少推断?这个引人入胜的问题在虚拟角色配音和辅助有表达性语言障碍的个体等应用中具有巨大潜力。现有的面部到语音的方法在捕捉身份特征方面表现出巨大的前景,但在生成具有情感表达的多样化语音风格方面存在困难。在本文中,我们探索了一项新任务,称为情感面部到语音,旨在直接从富有表现力的面部线索中合成情感语音。为此,我们引入了DEmoFace,这是一个新的生成框架,它利用带有课程学习的离散扩散变压器(DiT),建立在多级神经音频编解码器上。具体来说,我们提出了多模态DiT块,以动态对齐文本和语音,同时根据面部情感和身份定制语音风格。为了提高训练效率和生成质量,我们还为多级令牌处理引入了一种从粗到细的课程教学算法。此外,我们开发了一种增强的无预测器指导方法,以处理各种条件场景,实现多条件生成并有效地解开复杂属性。大量的实验结果证明,与基线相比,DEmoFace生成的语音更加自然和一致,甚至超越了语音驱动的方法。演示请访问:https://demoface-ai.github.io/。
论文及项目相关链接
Summary
面孔驱动的情感语音合成研究有了新的进展。在本文中,提出了一种新型生成框架DEmoFace,结合离散扩散变压器(DiT)和分级神经网络音频编解码器,实现了基于表情面孔的语音合成。该方法能够根据不同面孔情感和身份定制语音风格,并采用从粗到细的分级学习算法提高训练效率和生成质量。实验结果表明,DEmoFace生成的语音更加自然和一致,超越了基线方法。演示网站为:https://demoface-ai.github.io/。
Key Takeaways
- 情感面孔可作为情感语音合成的驱动因素,具有广泛的应用前景,如虚拟角色配音和辅助表达性语言障碍人士。
- DEmoFace是一种新型生成框架,利用离散扩散变压器(DiT)和分级神经网络音频编解码器实现情感语音合成。
- 多模态DiT块可以动态对齐文本和语音,同时根据面部情感和身份定制语音风格。
- 采用从粗到细的分级学习算法提高训练效率和生成质量。
- 引入预测器免费指导来处理各种条件场景,实现多条件生成并有效分离复杂属性。
- 实验结果表明,DEmoFace生成的语音更加自然和一致,超越了基线方法。
点此查看论文截图
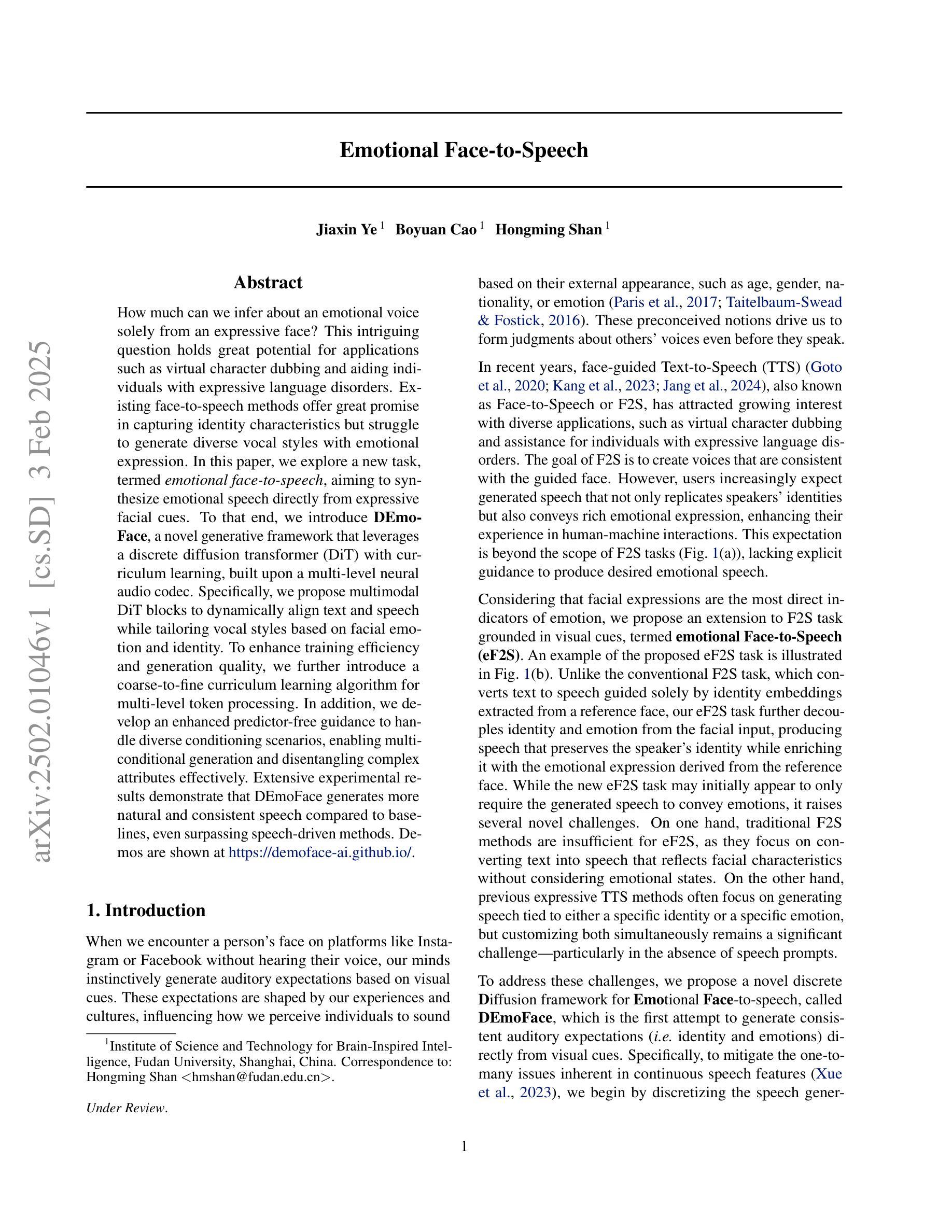
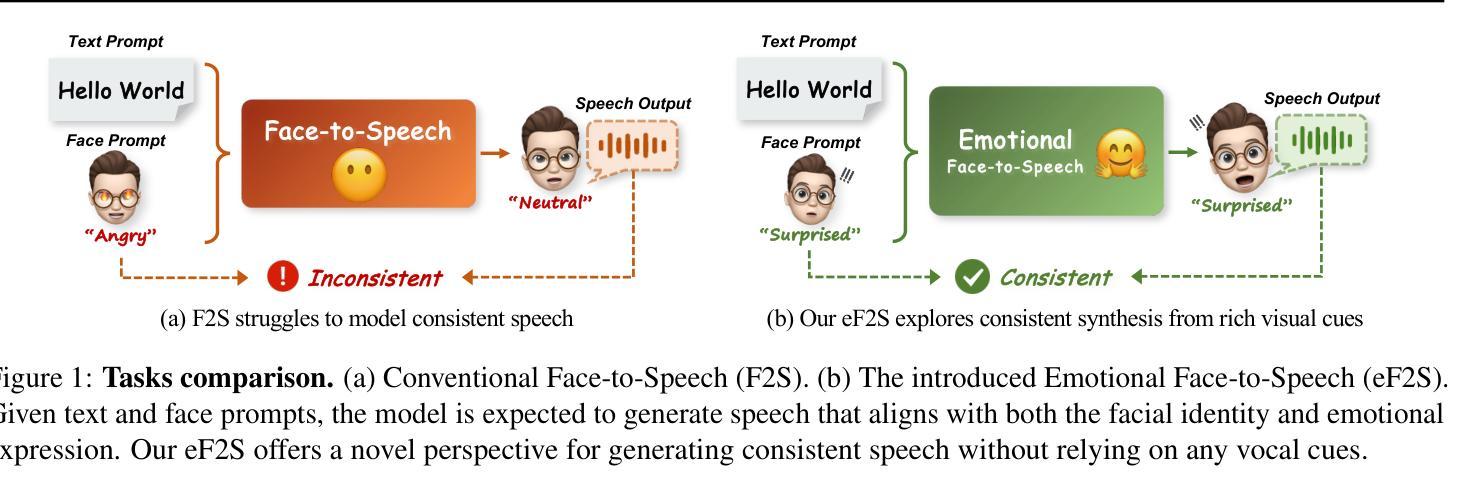
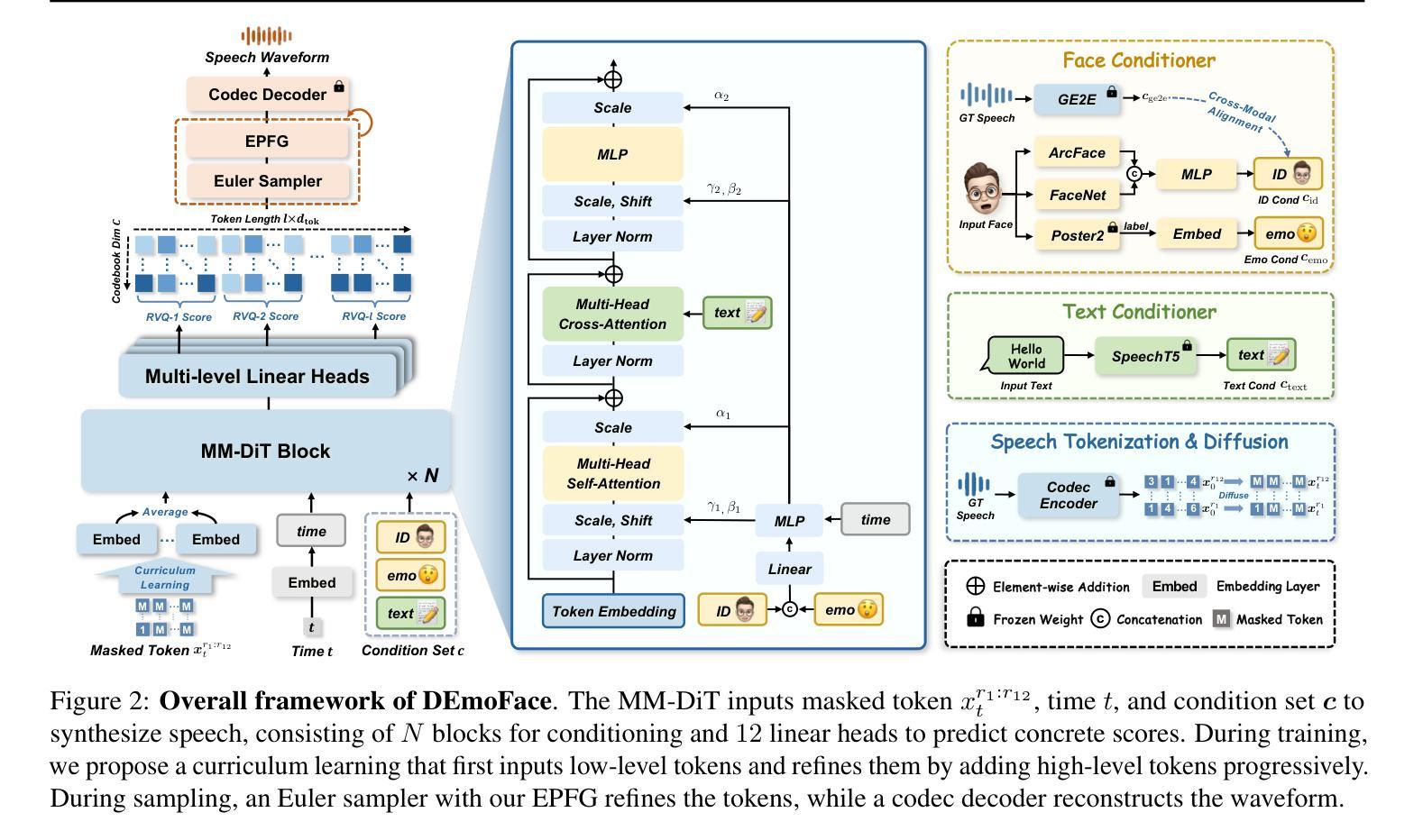
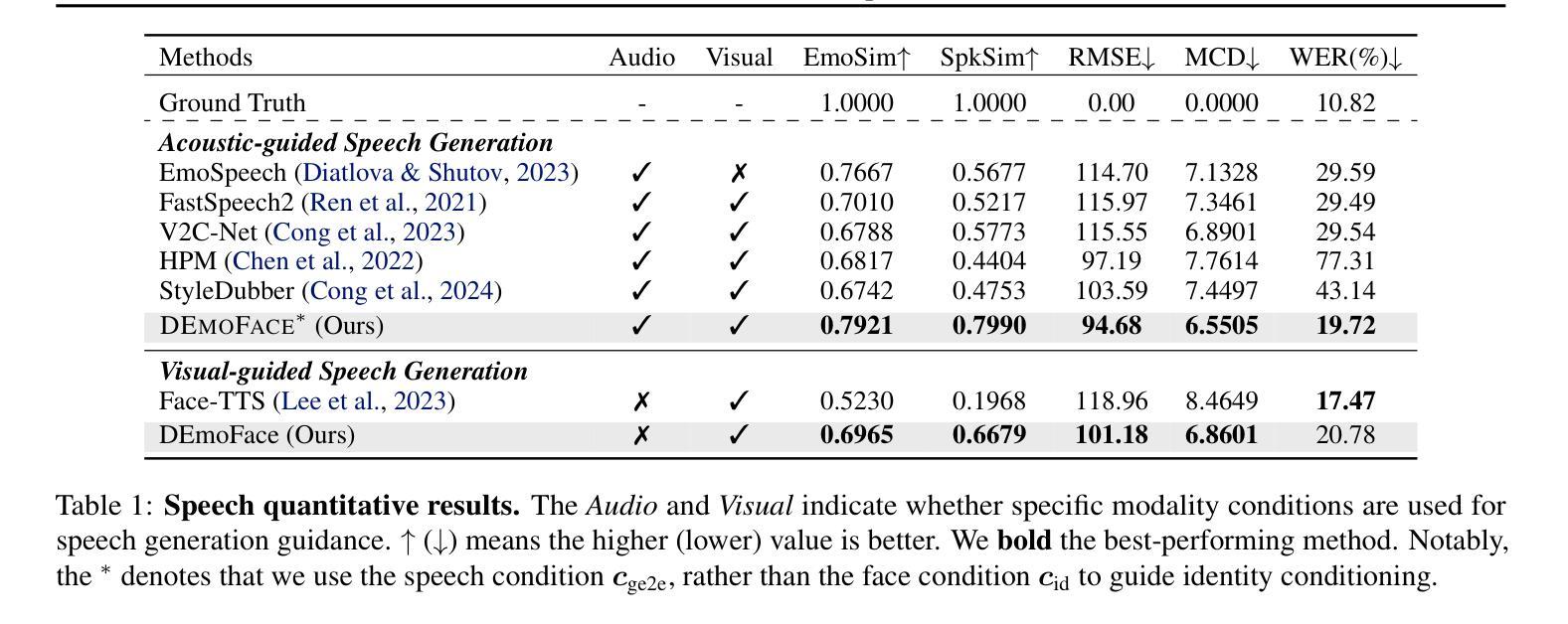
EmoTalkingGaussian: Continuous Emotion-conditioned Talking Head Synthesis
Authors:Junuk Cha, Seongro Yoon, Valeriya Strizhkova, Francois Bremond, Seungryul Baek
3D Gaussian splatting-based talking head synthesis has recently gained attention for its ability to render high-fidelity images with real-time inference speed. However, since it is typically trained on only a short video that lacks the diversity in facial emotions, the resultant talking heads struggle to represent a wide range of emotions. To address this issue, we propose a lip-aligned emotional face generator and leverage it to train our EmoTalkingGaussian model. It is able to manipulate facial emotions conditioned on continuous emotion values (i.e., valence and arousal); while retaining synchronization of lip movements with input audio. Additionally, to achieve the accurate lip synchronization for in-the-wild audio, we introduce a self-supervised learning method that leverages a text-to-speech network and a visual-audio synchronization network. We experiment our EmoTalkingGaussian on publicly available videos and have obtained better results than state-of-the-arts in terms of image quality (measured in PSNR, SSIM, LPIPS), emotion expression (measured in V-RMSE, A-RMSE, V-SA, A-SA, Emotion Accuracy), and lip synchronization (measured in LMD, Sync-E, Sync-C), respectively.
基于3D高斯投影技术的谈话头部合成技术因其能够实时生成高保真图像而备受关注。然而,由于其通常仅在缺乏面部表情多样性的短视频上进行训练,因此生成的谈话头部在表达广泛情绪方面存在困难。为了解决这一问题,我们提出了一种唇形对齐情绪面部生成器,并利用它训练了我们的EmoTalkingGaussian模型。该模型能够在连续的情绪值(即效价和激活)条件下操作面部表情,同时保持与输入音频的唇部运动同步。此外,为了实现野外音频的精确唇形同步,我们引入了一种自我监督学习方法,该方法利用文本到语音网络和视觉-音频同步网络。我们在公开视频上测试了EmoTalkingGaussian,在图像质量(以PSNR、SSIM、LPIPS衡量)、情感表达(以V-RMSE、A-RMSE、V-SA、A-SA、情感准确度衡量)和唇形同步(以LMD、Sync-E、Sync-C衡量)等方面获得了比当前最佳水平更好的结果。
论文及项目相关链接
PDF 22 pages
Summary:基于高斯球投影技术的三维头部合成方法受到关注,其能够实时生成高质量图像。为解决训练视频缺乏情感多样性问题,提出情感面部生成器并用其训练EmoTalkingGaussian模型,该模型可根据连续情感值操控面部表情并同步唇部动作。引入文本转语音网络和视觉音频同步网络实现自我监督学习,提升唇音同步准确性。在公开视频上的实验结果表明其在图像质量、情感表达和唇音同步方面优于其他最新方法。
Key Takeaways:
- 基于高斯球投影技术的三维头部合成方法能实时生成高质量图像。
- 训练视频缺乏情感多样性导致生成的头部难以表达多种情感。
- 提出情感面部生成器并用其训练EmoTalkingGaussian模型,可根据连续情感值操控面部表情。
- 模型能同步唇部动作与输入音频。
- 为提高唇音同步准确性,引入文本转语音网络和视觉音频同步网络的自我监督学习方法。
- EmoTalkingGaussian模型在图像质量、情感表达和唇音同步方面的表现优于其他最新方法。
点此查看论文截图
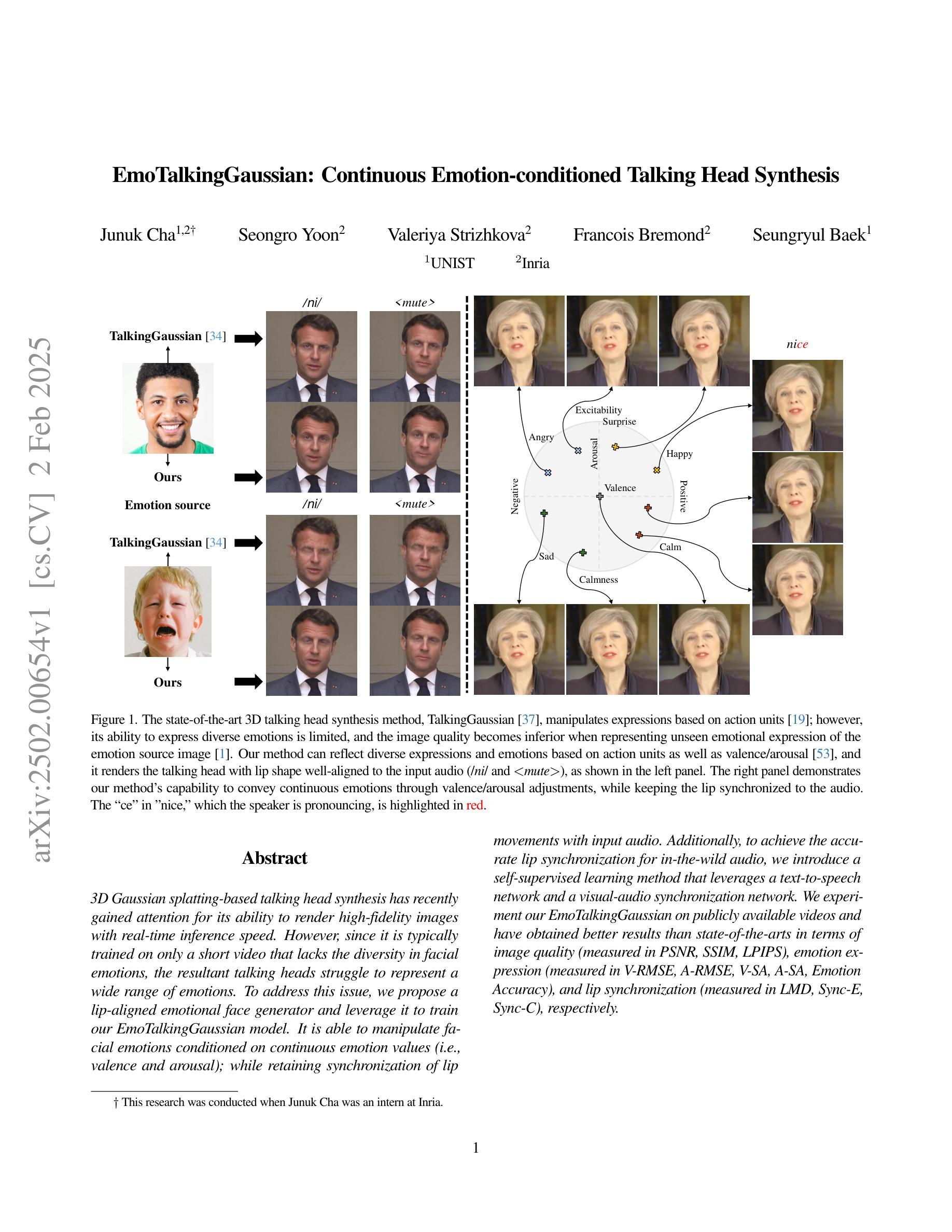
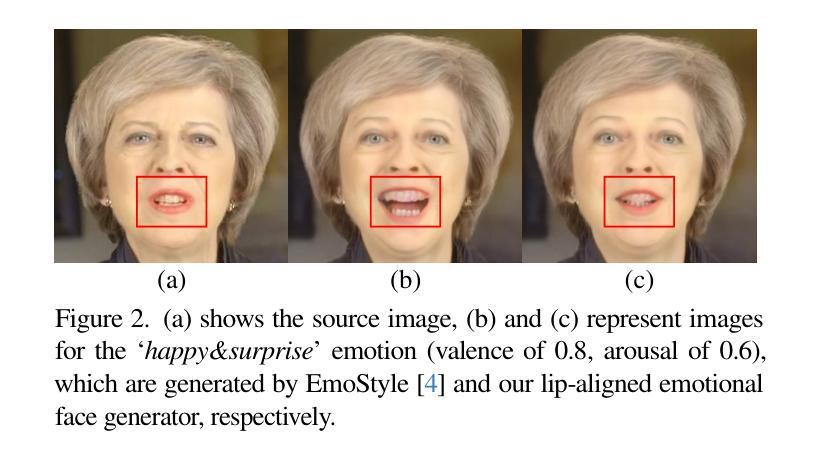
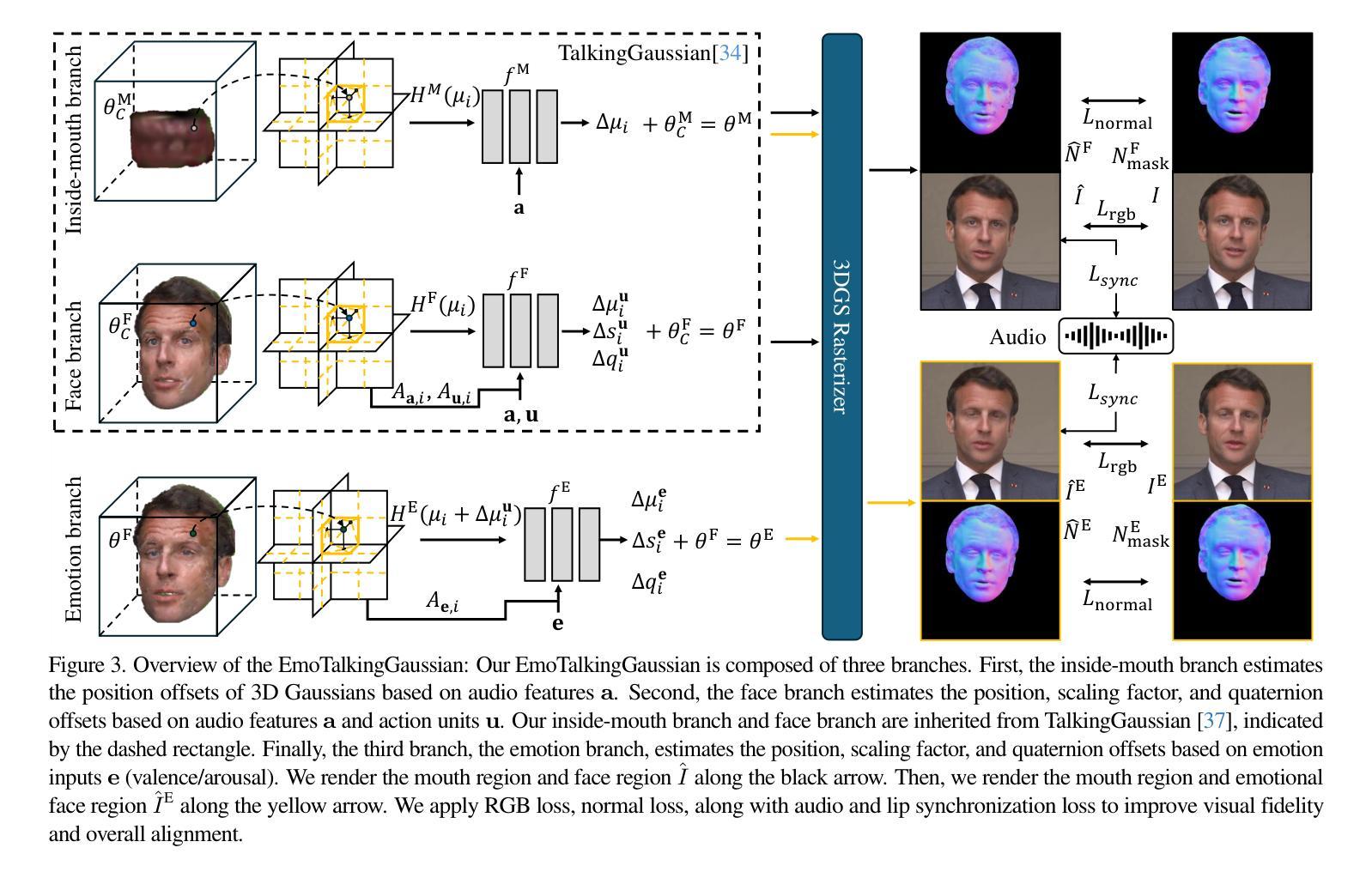
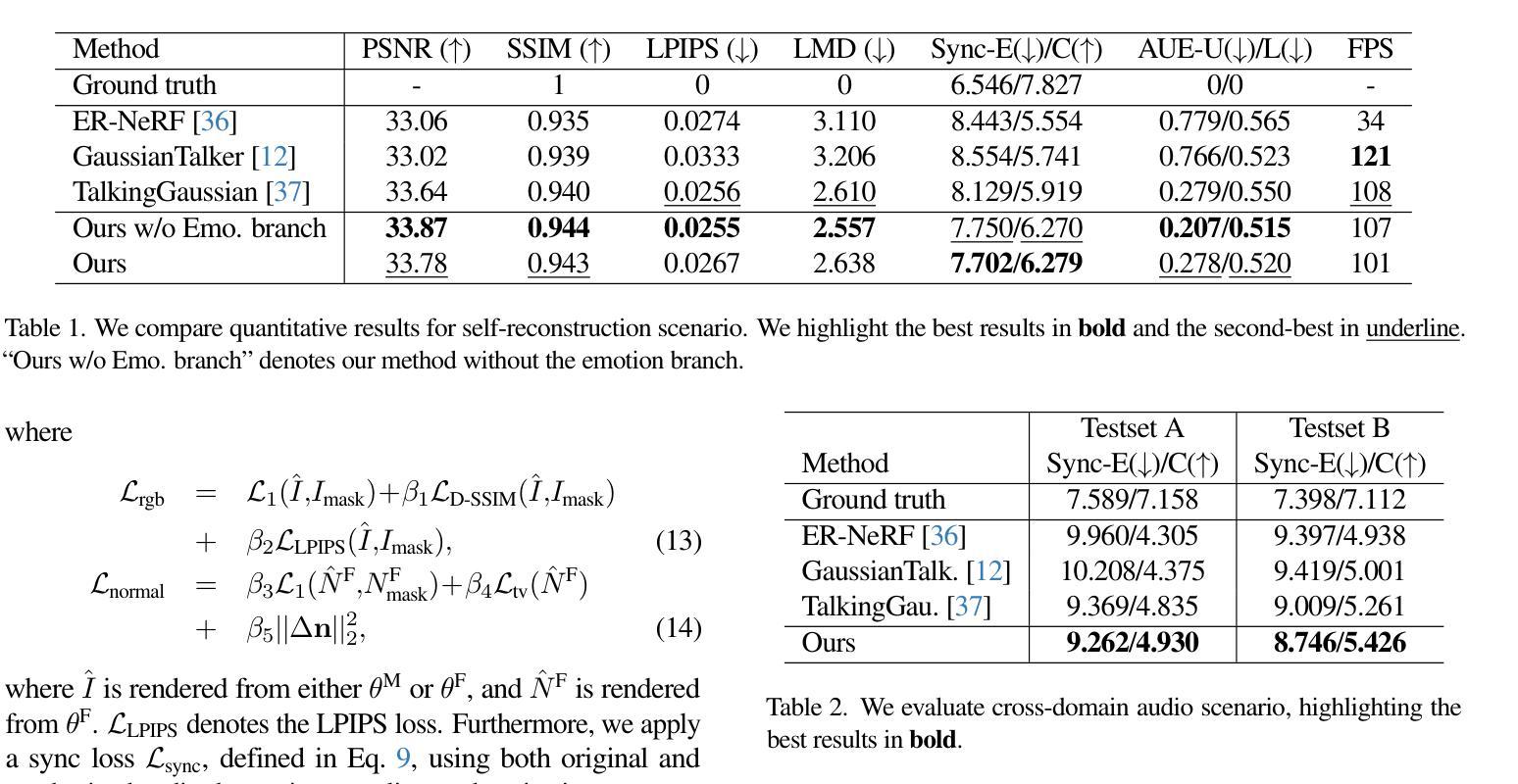