⚠️ 以下所有内容总结都来自于 大语言模型的能力,如有错误,仅供参考,谨慎使用
🔴 请注意:千万不要用于严肃的学术场景,只能用于论文阅读前的初筛!
💗 如果您觉得我们的项目对您有帮助 ChatPaperFree ,还请您给我们一些鼓励!⭐️ HuggingFace免费体验
2025-02-06 更新
CASIM: Composite Aware Semantic Injection for Text to Motion Generation
Authors:Che-Jui Chang, Qingze Tony Liu, Honglu Zhou, Vladimir Pavlovic, Mubbasir Kapadia
Recent advances in generative modeling and tokenization have driven significant progress in text-to-motion generation, leading to enhanced quality and realism in generated motions. However, effectively leveraging textual information for conditional motion generation remains an open challenge. We observe that current approaches, primarily relying on fixed-length text embeddings (e.g., CLIP) for global semantic injection, struggle to capture the composite nature of human motion, resulting in suboptimal motion quality and controllability. To address this limitation, we propose the Composite Aware Semantic Injection Mechanism (CASIM), comprising a composite-aware semantic encoder and a text-motion aligner that learns the dynamic correspondence between text and motion tokens. Notably, CASIM is model and representation-agnostic, readily integrating with both autoregressive and diffusion-based methods. Experiments on HumanML3D and KIT benchmarks demonstrate that CASIM consistently improves motion quality, text-motion alignment, and retrieval scores across state-of-the-art methods. Qualitative analyses further highlight the superiority of our composite-aware approach over fixed-length semantic injection, enabling precise motion control from text prompts and stronger generalization to unseen text inputs.
近期生成建模和分词技术的进展推动了文本到运动生成的显著进步,提高了生成运动的品质和真实感。然而,如何有效利用文本信息进行有条件的运动生成仍然是一个开放性的挑战。我们发现,当前的方法主要依赖于固定长度的文本嵌入(例如CLIP)进行全局语义注入,很难捕捉人类运动的复合性质,导致运动质量和可控性不佳。为了解决这一局限性,我们提出了复合感知语义注入机制(CASIM),包括一个复合感知语义编码器和文本运动对齐器,学习文本和运动令牌之间的动态对应关系。值得注意的是,CASIM是模型和无表示特定的,很容易与自回归和基于扩散的方法集成。在人类ML3D和KIT基准测试上的实验表明,CASIM持续提高了运动质量、文本运动对齐和最新方法的检索得分。定性分析进一步突出了我们复合感知方法相较于固定长度语义注入的优势,能够实现从文本提示的精确运动控制,并增强对未见文本输入的泛化能力。
论文及项目相关链接
Summary
文本中介绍了近期生成建模和标记化的进展推动了文本到运动生成领域的显著进步,但如何利用文本信息进行条件运动生成仍存在挑战。当前方法主要依赖固定长度的文本嵌入(如CLIP)进行全局语义注入,难以捕捉人类运动的复合性质,导致运动质量和可控性不佳。为解决此问题,提出了复合感知语义注入机制(CASIM),包括复合感知语义编码器和文本运动对齐器,学习文本和运动标记之间的动态对应关系。CASIM可融入自回归和扩散方法,并在HumanML3D和KIT基准测试中表现出改进效果。
Key Takeaways
- 文本到运动生成领域取得显著进步,得益于生成建模和标记化技术的最新发展。
- 利用文本信息进行条件运动生成仍存在挑战。
- 当前方法主要依赖固定长度的文本嵌入,难以捕捉人类运动的复合性质。
- CASIM机制旨在解决这一问题,通过复合感知语义编码器和文本运动对齐器有效捕捉文本与运动的动态关系。
- CASIM具有模型和无表示偏好性,可融入自回归和扩散方法。
- 在HumanML3D和KIT基准测试上,CASIM提高了运动质量、文本与运动对齐以及检索得分。
点此查看论文截图
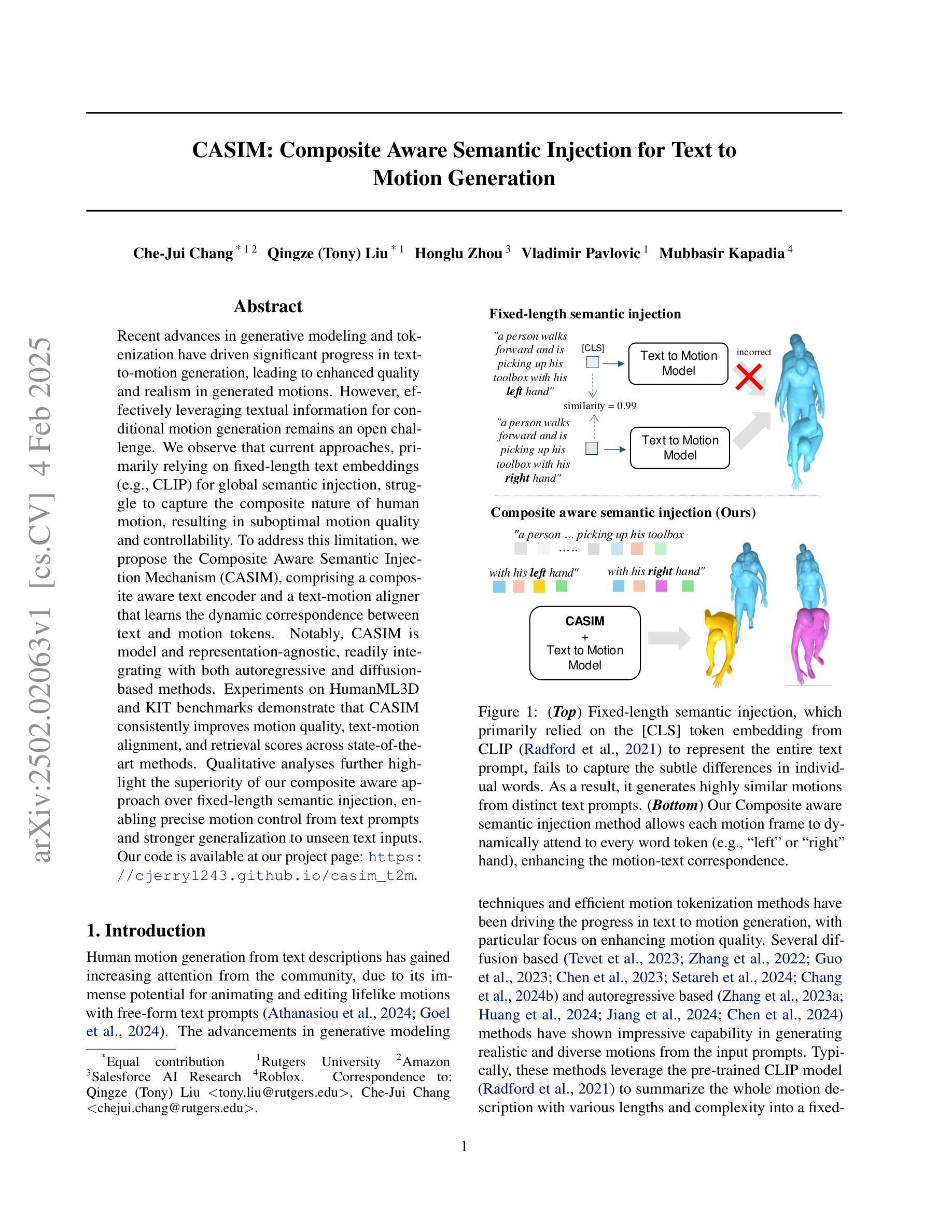
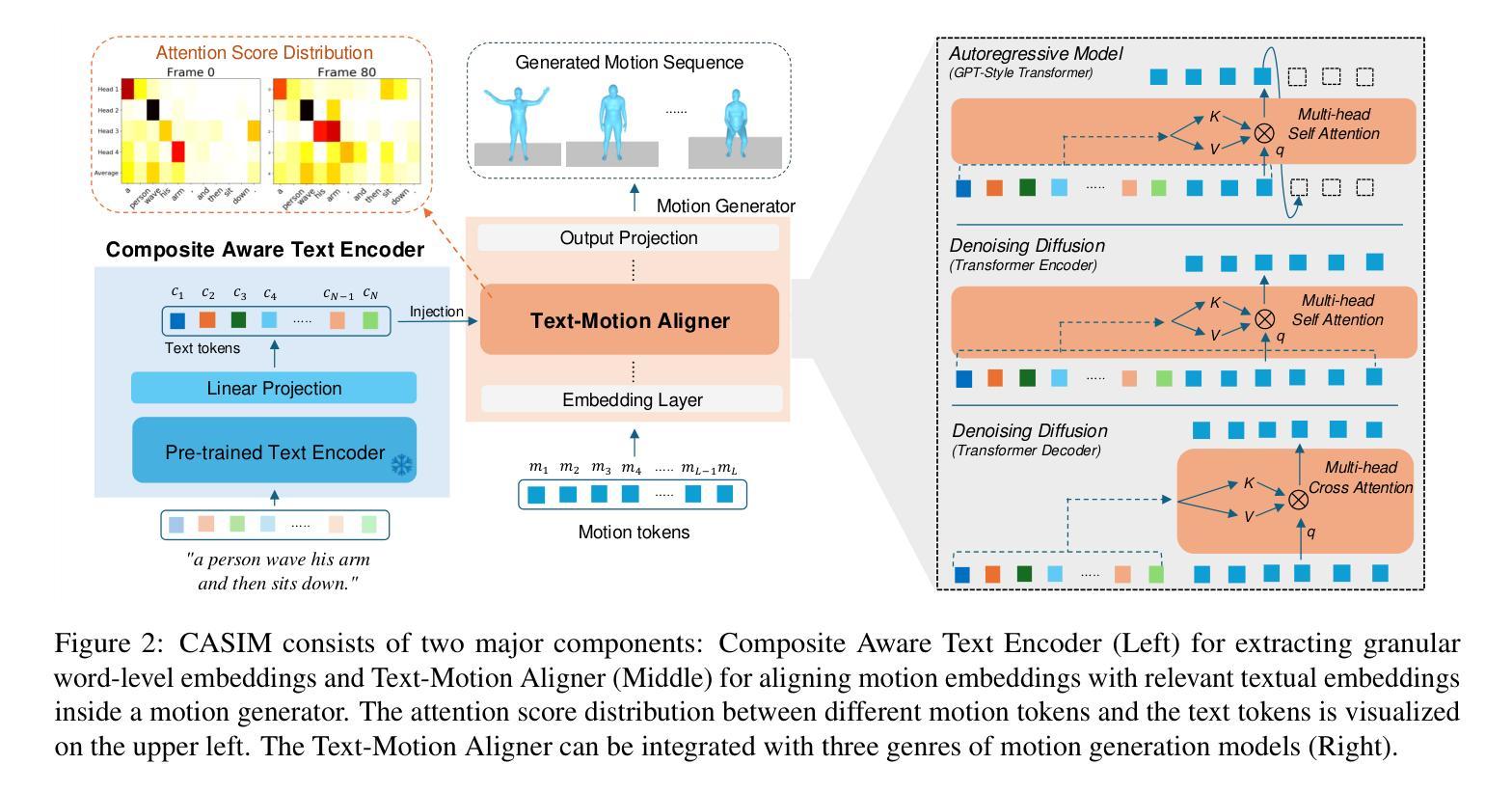
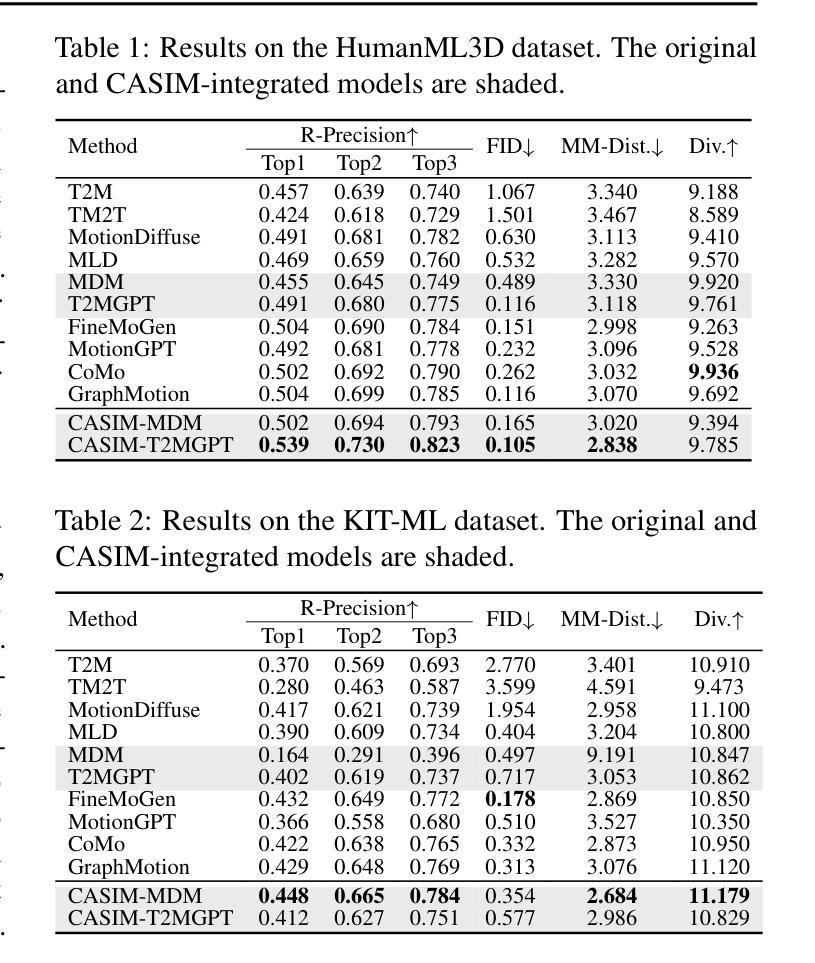
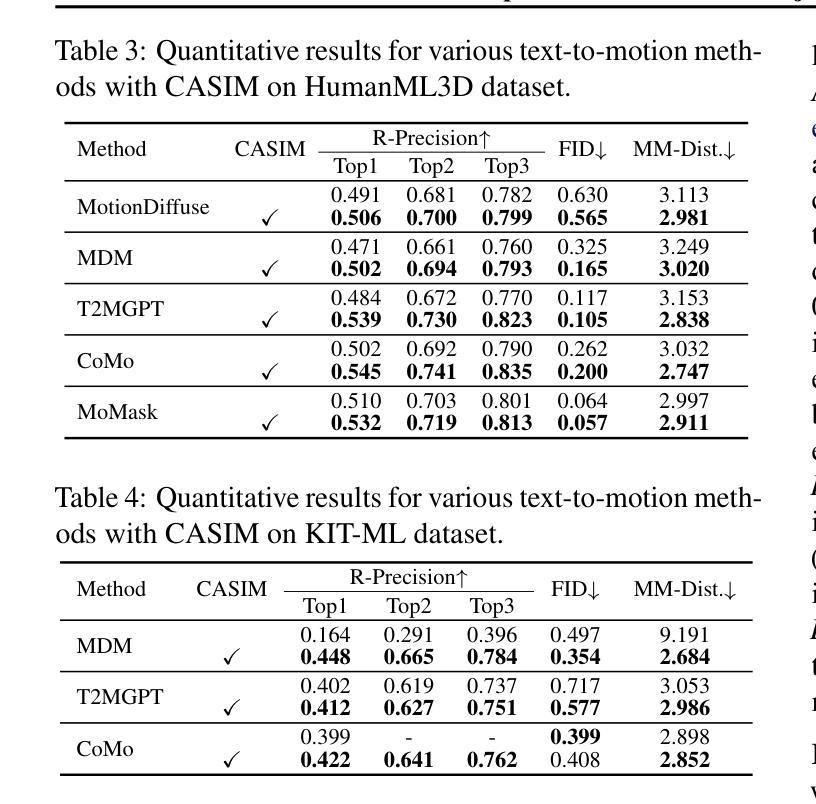
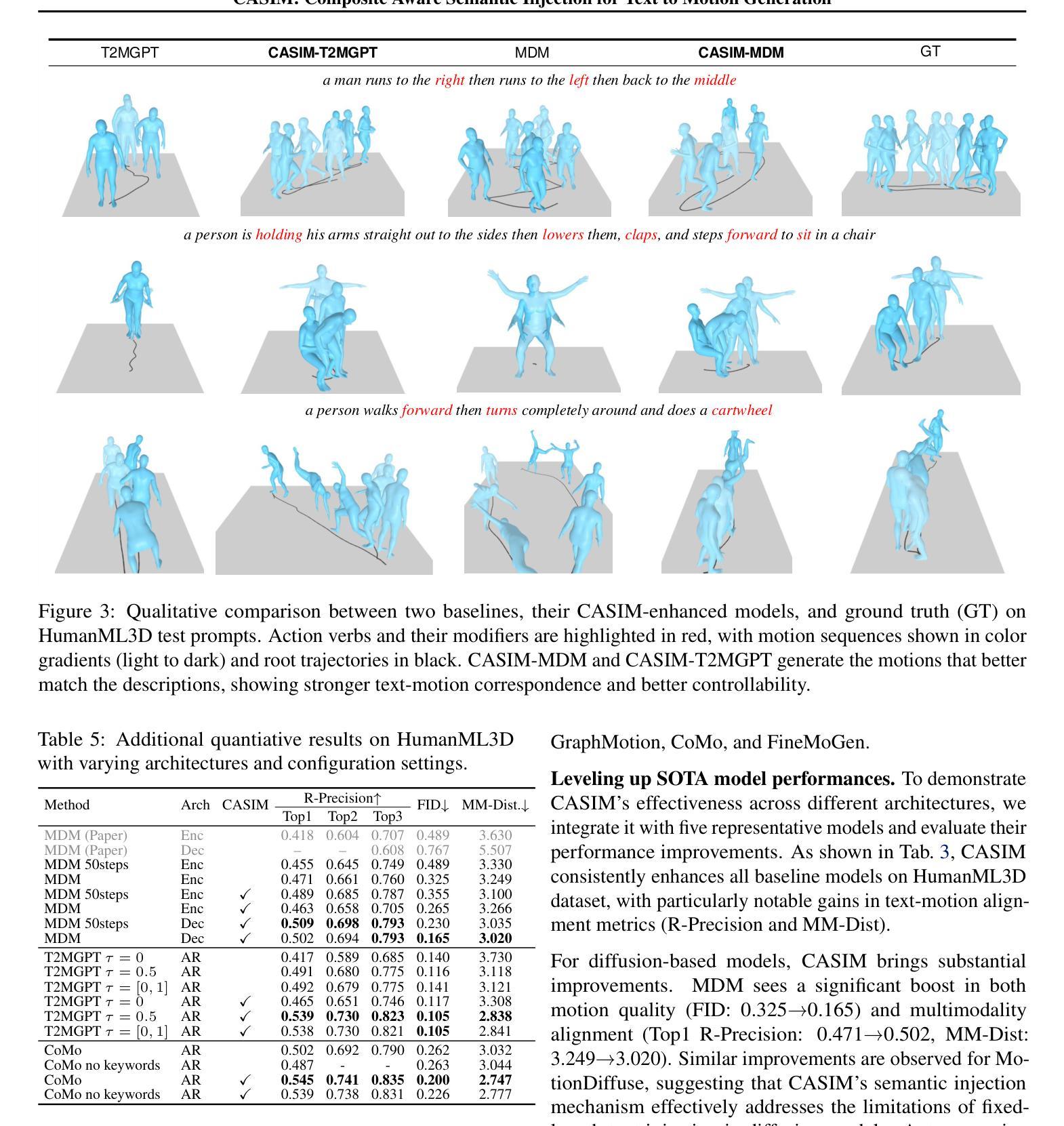