⚠️ 以下所有内容总结都来自于 大语言模型的能力,如有错误,仅供参考,谨慎使用
🔴 请注意:千万不要用于严肃的学术场景,只能用于论文阅读前的初筛!
💗 如果您觉得我们的项目对您有帮助 ChatPaperFree ,还请您给我们一些鼓励!⭐️ HuggingFace免费体验
2025-02-07 更新
CAPE: Covariate-Adjusted Pre-Training for Epidemic Time Series Forecasting
Authors:Zewen Liu, Juntong Ni, Max S. Y. Lau, Wei Jin
Accurate forecasting of epidemic infection trajectories is crucial for safeguarding public health. However, limited data availability during emerging outbreaks and the complex interaction between environmental factors and disease dynamics present significant challenges for effective forecasting. In response, we introduce CAPE, a novel epidemic pre-training framework designed to harness extensive disease datasets from diverse regions and integrate environmental factors directly into the modeling process for more informed decision-making on downstream diseases. Based on a covariate adjustment framework, CAPE utilizes pre-training combined with hierarchical environment contrasting to identify universal patterns across diseases while estimating latent environmental influences. We have compiled a diverse collection of epidemic time series datasets and validated the effectiveness of CAPE under various evaluation scenarios, including full-shot, few-shot, zero-shot, cross-location, and cross-disease settings, where it outperforms the leading baseline by an average of 9.9% in full-shot and 14.3% in zero-shot settings. The code will be released upon acceptance.
流行病感染轨迹的准确预测对于保障公共卫生至关重要。然而,新兴疫情期间的有限数据以及环境因素与疾病动态之间的复杂相互作用为有效预测带来了重大挑战。为了应对这些挑战,我们引入了CAPE,这是一个新型的流行病预训练框架,旨在利用来自不同地区的广泛疾病数据集,并将环境因素直接融入建模过程,以为下游疾病的决策提供更有依据的决策支持。基于协变量调整框架,CAPE利用预训练结合分层环境对比,在估计潜在环境影响的同时,识别疾病之间的通用模式。我们收集了各种各样的流行病时间序列数据集,并在各种评估场景下验证了CAPE的有效性,包括全数据、小数据、零数据、跨地点和跨疾病设置。CAPE在全数据场景下较领先基线高出平均9.9%,在零数据场景下高出平均14.3%。代码将在接受后发布。
论文及项目相关链接
Summary
CAPE是一个新型传染病预训练框架,利用广泛的疾病数据集和环境因素信息来预测传染病的轨迹,提高了决策的可靠性。通过采用协变量调整框架、层次环境对比和预训练技术,CAPE能够在各种疾病背景下识别通用模式并估算潜在环境影响。在多种评估场景下,CAPE的表现优于现有基线模型。
Key Takeaways
- CAPE是一个针对传染病预测的预训练框架,用于利用广泛的疾病数据集和环境因素进行建模。
- CAPE采用协变量调整框架来整合环境数据,提高预测准确性。
- 通过层次环境对比技术,CAPE能够识别不同疾病间的通用模式。
- CAPE通过预训练技术来优化模型性能。
- 在多种评估场景中,CAPE表现优于现有基线模型,特别是在少样本和无样本场景下表现更突出。
- CAPE能够处理有限数据下的传染病预测挑战,为公共卫生决策提供更可靠的信息支持。
点此查看论文截图
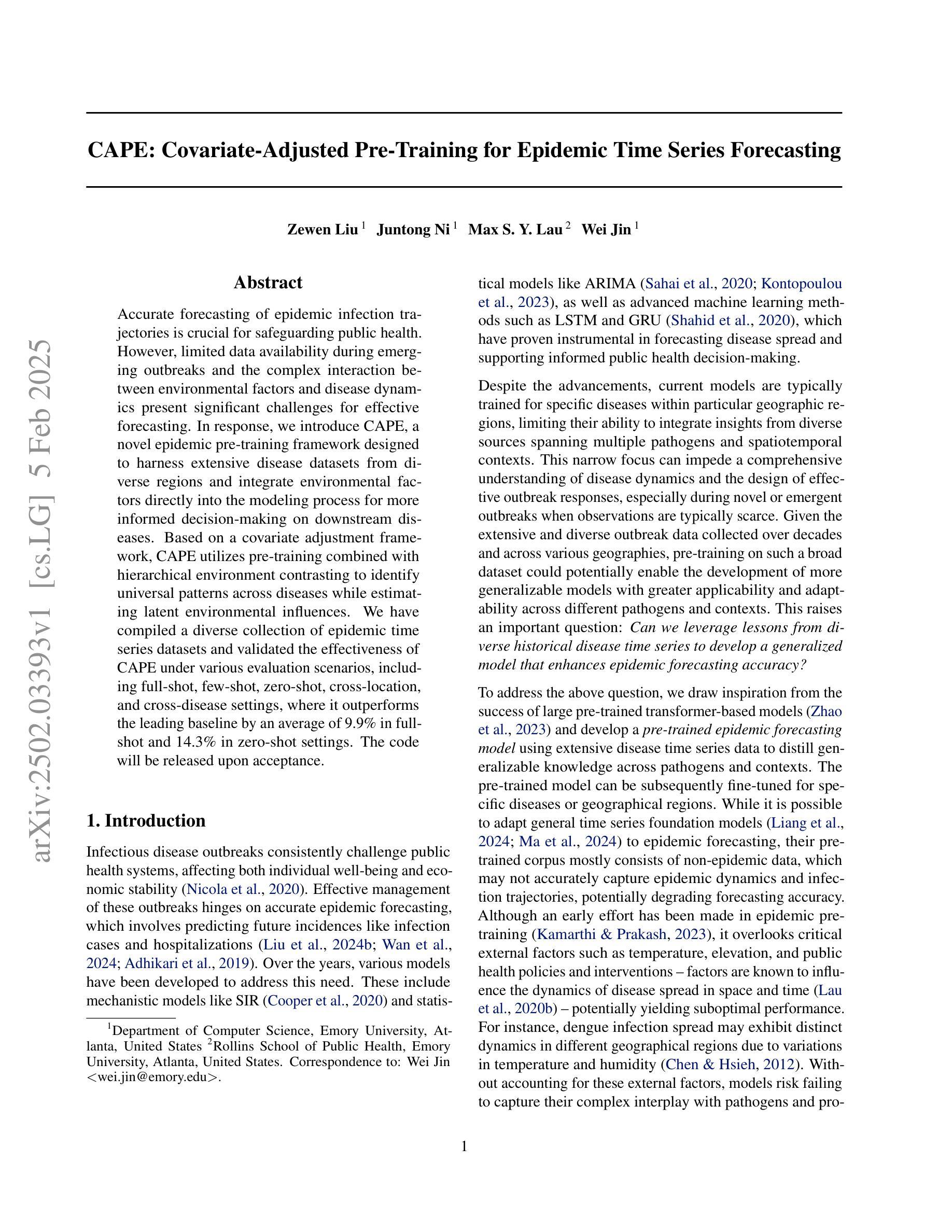
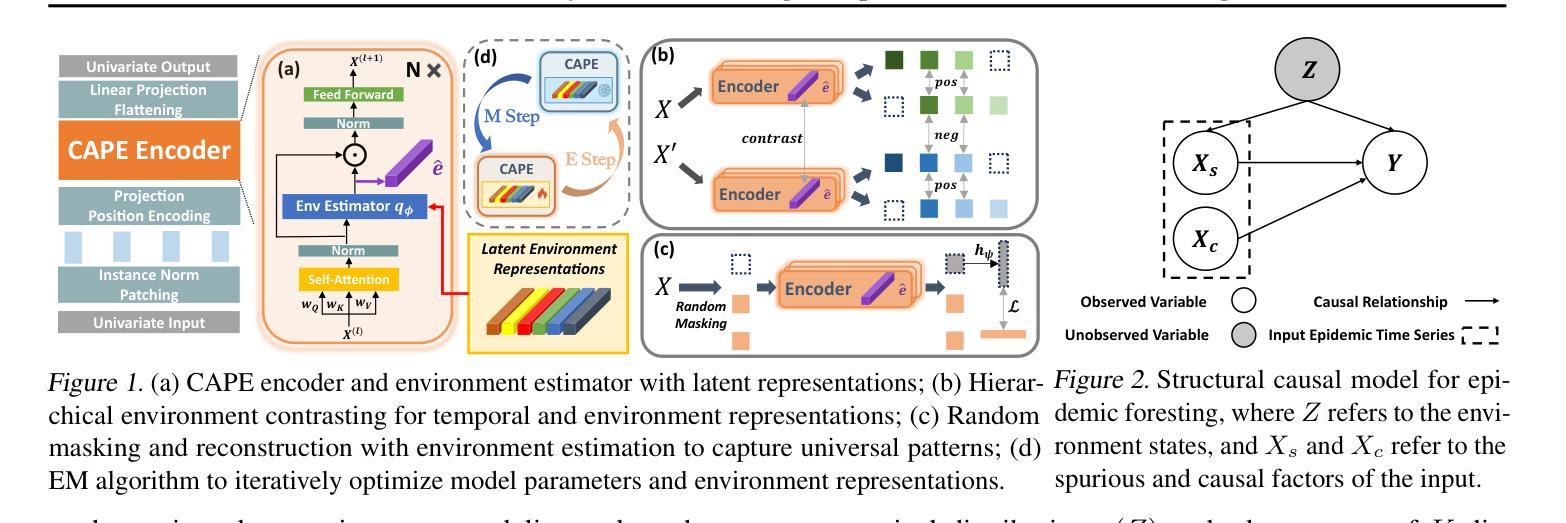
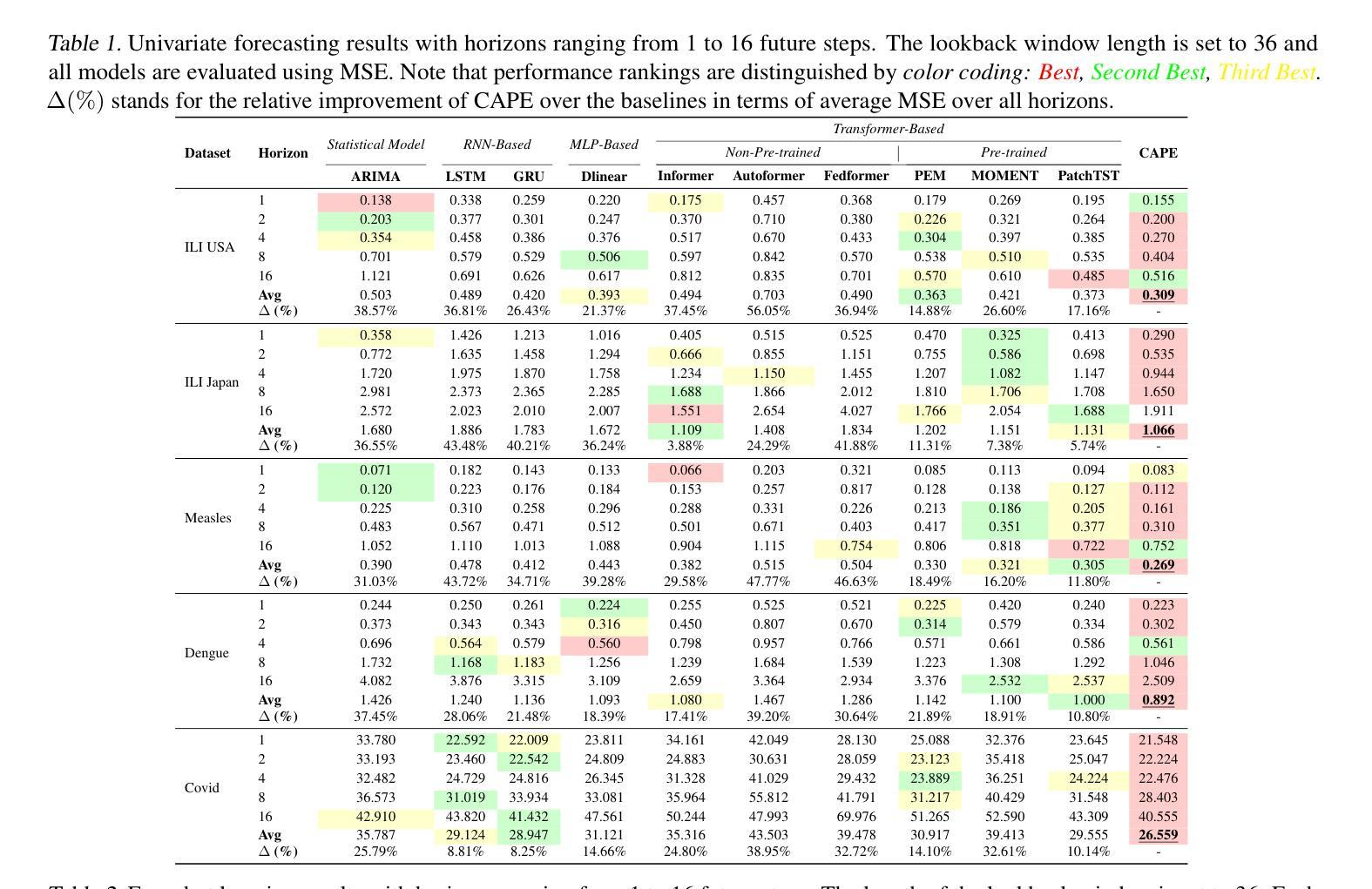
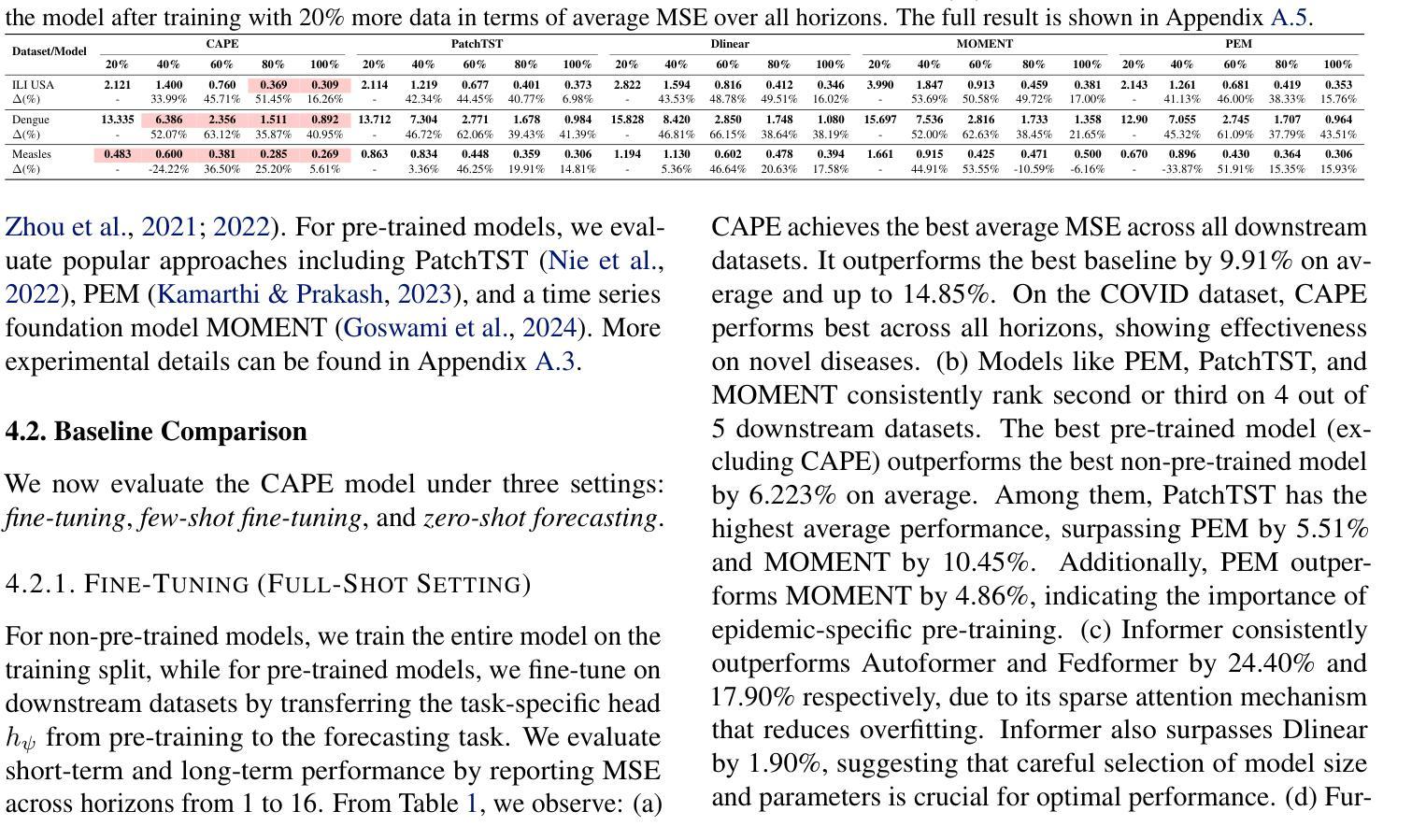
ALPET: Active Few-shot Learning for Citation Worthiness Detection in Low-Resource Wikipedia Languages
Authors:Aida Halitaj, Arkaitz Zubiaga
Citation Worthiness Detection (CWD) consists in determining which sentences, within an article or collection, should be backed up with a citation to validate the information it provides. This study, introduces ALPET, a framework combining Active Learning (AL) and Pattern-Exploiting Training (PET), to enhance CWD for languages with limited data resources. Applied to Catalan, Basque, and Albanian Wikipedia datasets, ALPET outperforms the existing CCW baseline while reducing the amount of labeled data in some cases above 80%. ALPET’s performance plateaus after 300 labeled samples, showing it suitability for low-resource scenarios where large, labeled datasets are not common. While specific active learning query strategies, like those employing K-Means clustering, can offer advantages, their effectiveness is not universal and often yields marginal gains over random sampling, particularly with smaller datasets. This suggests that random sampling, despite its simplicity, remains a strong baseline for CWD in constraint resource environments. Overall, ALPET’s ability to achieve high performance with fewer labeled samples makes it a promising tool for enhancing the verifiability of online content in low-resource language settings.
引用价值检测(CWD)的目的是确定文章或集合中的哪些句子应该通过引用验证其提供的信息。本研究介绍了ALPET,这是一个结合主动学习(AL)和模式挖掘训练(PET)的框架,以提高对数据资源有限的语言的CWD能力。在加泰罗尼亚语、巴斯克语和阿尔巴尼亚语维基百科数据集上应用时,ALPET的表现超过了现有的CCW基线,在某些情况下减少了超过80%的标注数据。ALPET的性能在300个标注样本后达到平稳,这显示出它在资源匮乏的场景下的适用性,在那里大型标注数据集并不常见。虽然特定的主动学习查询策略,如使用K-Means聚类等,可能会带来优势,但其有效性并非普遍适用,并且往往只带来相对于随机采样的微小收益,特别是在较小的数据集上。这表明,尽管随机采样方法简单,但在受限资源环境中仍然是CWD的强基线。总体而言,ALPET能够在较少的标注样本上实现高性能,使其成为提高低资源语言环境中在线内容可验证性的有前途的工具。
论文及项目相关链接
PDF 24 pages, 8 figures, 4 tables
Summary
本文介绍了Citation Worthiness Detection(CWD)的概念,并提出了一种结合主动学习和模式挖掘训练(ALPET)的新框架,以提高在低资源语言环境中的CWD性能。研究表明,ALPET在加泰罗尼亚语、巴斯克语和阿尔巴尼亚语Wikipedia数据集上的表现优于现有CCW基线,并且在某些情况下可以减少80%以上的标注数据量。此外,ALPET在300个标注样本后性能趋于稳定,适合资源匮乏的场景。虽然特定的主动学习和查询策略如K-Means聚类在某些情况下具有优势,但其效果并非普遍有效,且通常仅在大型数据集上获得微小优势。因此,随机采样在资源受限环境中仍是CWD的强基线。总体而言,ALPET在减少标注样本的同时实现高性能,是增强低资源语言环境中在线内容可验证性的有前途的工具。
Key Takeaways
- Citation Worthiness Detection (CWD)旨在确定文章中哪些句子应该通过引用验证其提供的信息。
- ALPET框架结合了主动学习和模式挖掘训练,旨在提高低资源语言环境中的CWD性能。
- ALPET在加泰罗尼亚语、巴斯克语和阿尔巴尼亚语Wikipedia数据集上的表现优于现有基线。
- ALPET能够在减少标注数据量的同时保持高性能,有时减少量超过80%。
- 在低资源环境下,随机采样作为CWD的基线策略仍然有效。
- 特定主动学习和查询策略的效果并非普遍显著,通常在大型数据集上才能获得微小优势。
点此查看论文截图
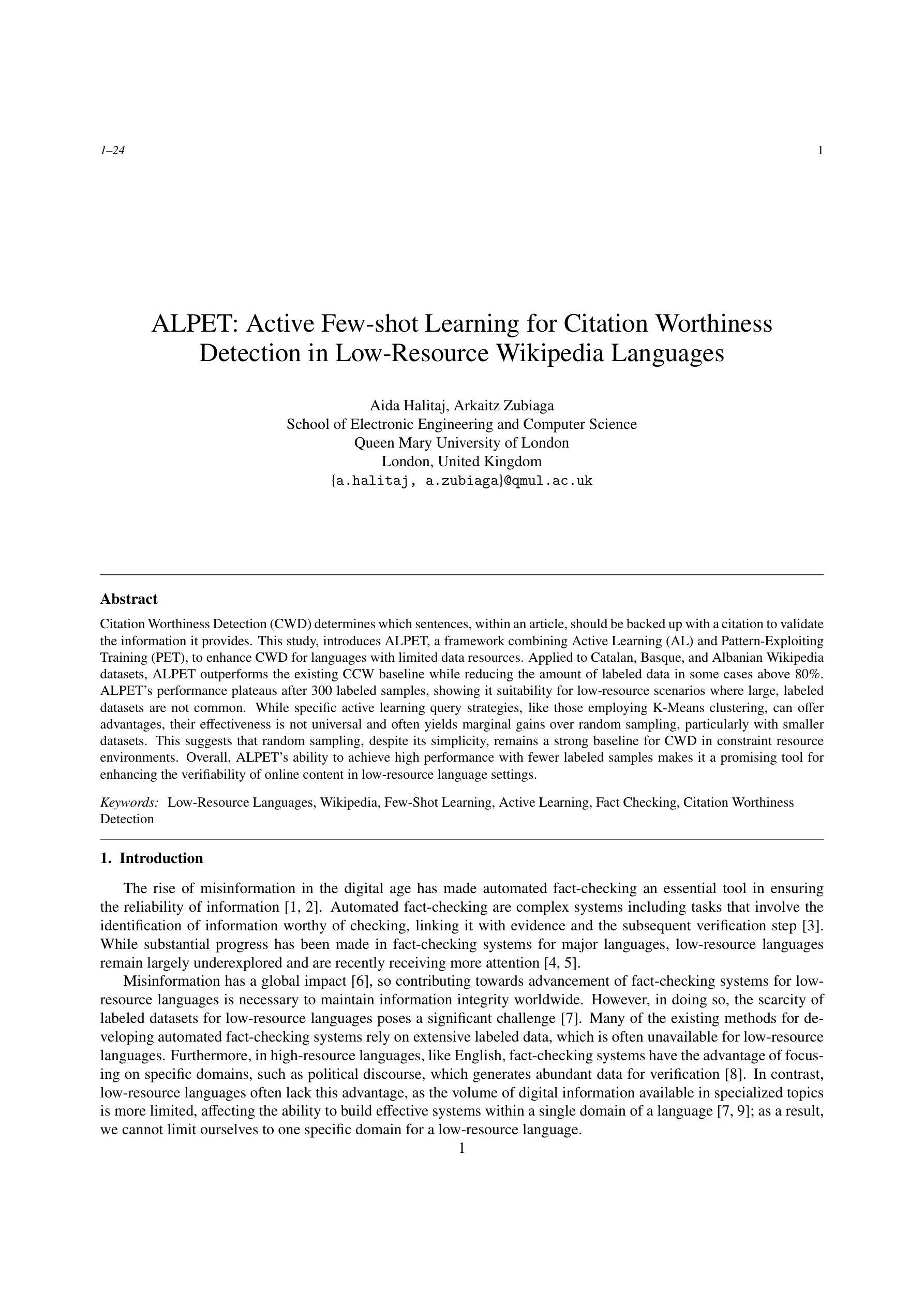
Scalable In-Context Learning on Tabular Data via Retrieval-Augmented Large Language Models
Authors:Xumeng Wen, Shun Zheng, Zhen Xu, Yiming Sun, Jiang Bian
Recent studies have shown that large language models (LLMs), when customized with post-training on tabular data, can acquire general tabular in-context learning (TabICL) capabilities. These models are able to transfer effectively across diverse data schemas and different task domains. However, existing LLM-based TabICL approaches are constrained to few-shot scenarios due to the sequence length limitations of LLMs, as tabular instances represented in plain text consume substantial tokens. To address this limitation and enable scalable TabICL for any data size, we propose retrieval-augmented LLMs tailored to tabular data. Our approach incorporates a customized retrieval module, combined with retrieval-guided instruction-tuning for LLMs. This enables LLMs to effectively leverage larger datasets, achieving significantly improved performance across 69 widely recognized datasets and demonstrating promising scaling behavior. Extensive comparisons with state-of-the-art tabular models reveal that, while LLM-based TabICL still lags behind well-tuned numeric models in overall performance, it uncovers powerful algorithms under limited contexts, enhances ensemble diversity, and excels on specific datasets. These unique properties underscore the potential of language as a universal and accessible interface for scalable tabular data learning.
最近的研究表明,大型语言模型(LLM)在针对表格数据进行后训练定制后,可以获得通用的上下文内表格学习(TabICL)能力。这些模型能够跨不同的数据架构和任务领域进行有效迁移。然而,由于LLM的序列长度限制,现有的基于LLM的TabICL方法仅限于小样本场景,以纯文本形式表示的表格实例会消耗大量令牌。为了解决这一限制,实现任何数据规模的可扩展TabICL,我们提出了针对表格数据定制的检索增强LLM。我们的方法结合了一个定制的检索模块,并结合检索指导的LLM指令调整。这使得LLM能够有效利用更大的数据集,在69个广泛认可的数据集上实现了显著的性能提升,并表现出了有前景的可扩展性行为。与最先进表格模型的广泛比较表明,虽然基于LLM的TabICL在总体性能上仍落后于经过良好调整的数值模型,但它在有限上下文下揭示了强大的算法,增强了组合多样性,并在特定数据集上表现出色。这些独特的特点突显了语言作为通用和可访问界面用于可扩展表格数据学习的潜力。
论文及项目相关链接
PDF Preprint
Summary
大型语言模型(LLM)通过针对表格数据进行后训练,可以获取通用的表格上下文学习能力(TabICL)。然而,由于文本表示的表格实例消耗大量令牌,现有LLM-based TabICL方法受限于小样本文档。为应对这一限制并实现对任意数据规模的TabICL进行扩展,我们提出定制的检索增强型LLM以用于表格数据。我们的方法结合了定制检索模块和检索引导LLM指令调整,使得LLM能够有效利用大规模数据集。对比结果显示,尽管相较于调优的数字模型,LLM在总体性能上仍有不足,但在特定情境下揭示了强大的算法,增强了集成多样性并在特定数据集上表现出卓越性能。这表明语言作为通用和可访问的接口具有可扩展的表格数据学习潜力。
Key Takeaways
- LLM通过定制的后训练可以获得通用的表格上下文学习能力(TabICL)。
- 现有LLM-based TabICL方法受限于小样本文档场景,因为表格数据转换为文本消耗大量令牌。
- 为解决此限制,提出了检索增强型LLM方法,结合定制检索模块和检索引导指令调整。
- 该方法使LLM能够利用大规模数据集进行有效学习。
- 对比实验表明,LLM在总体性能上相较于调优的数字模型仍有不足,但在特定情境下展现出强大的算法性能。
- LLM增强了集成多样性并在特定数据集上表现出卓越性能。
点此查看论文截图
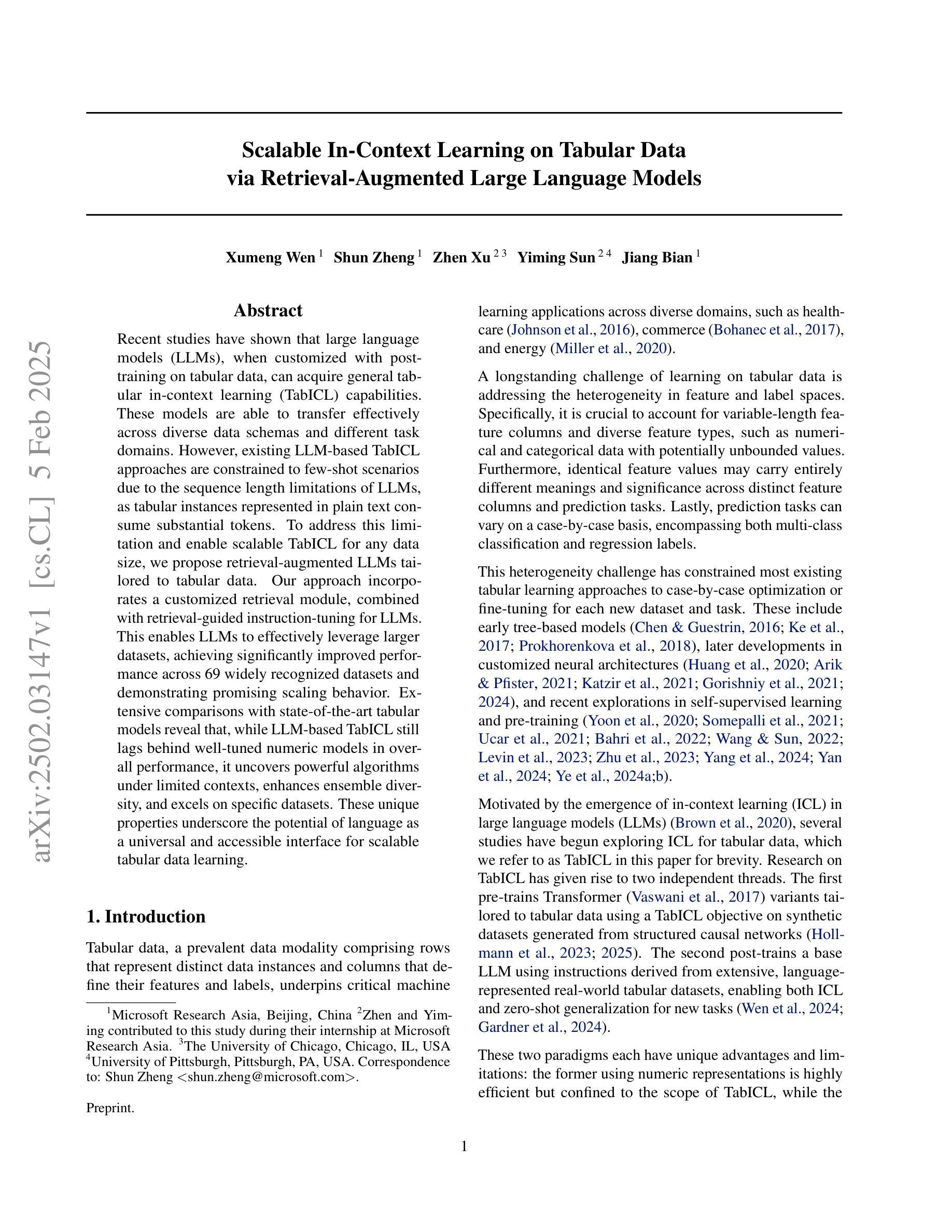
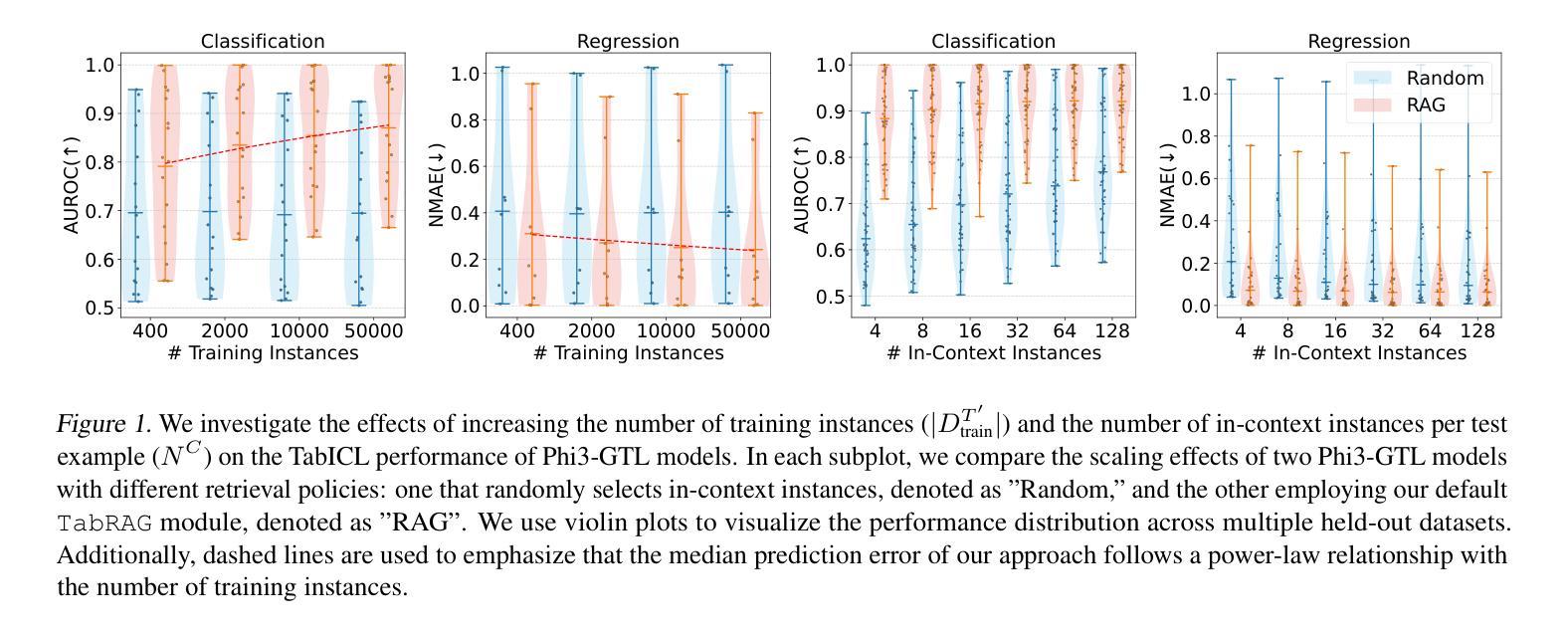
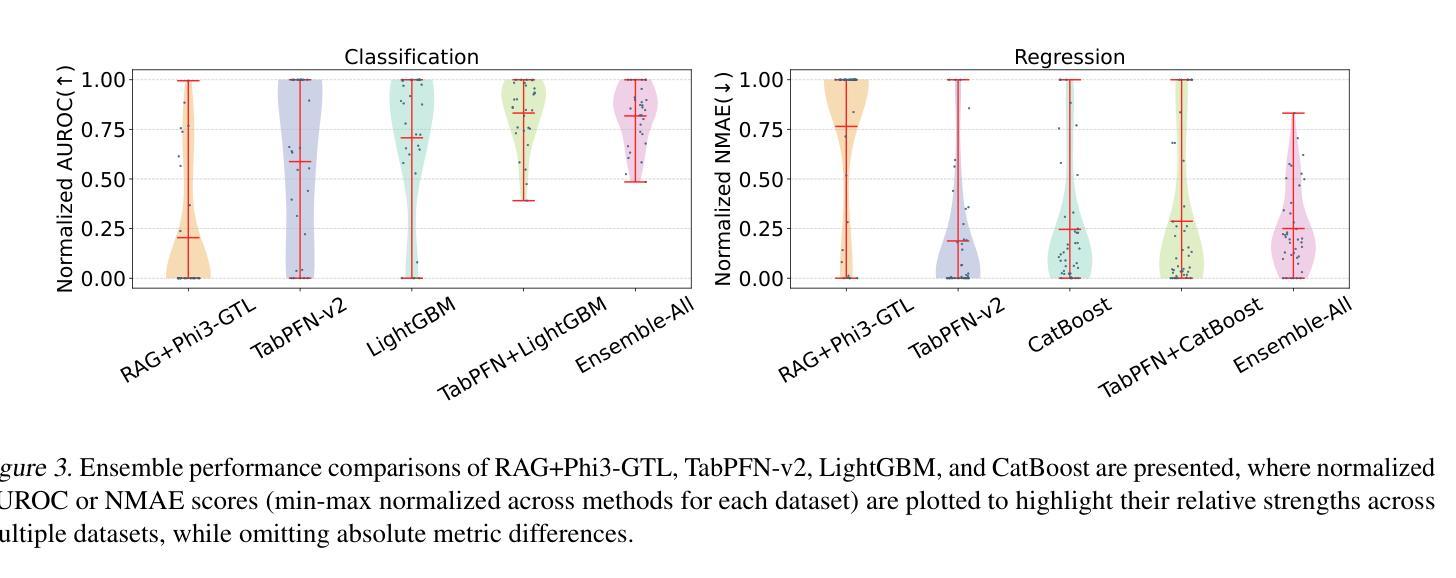
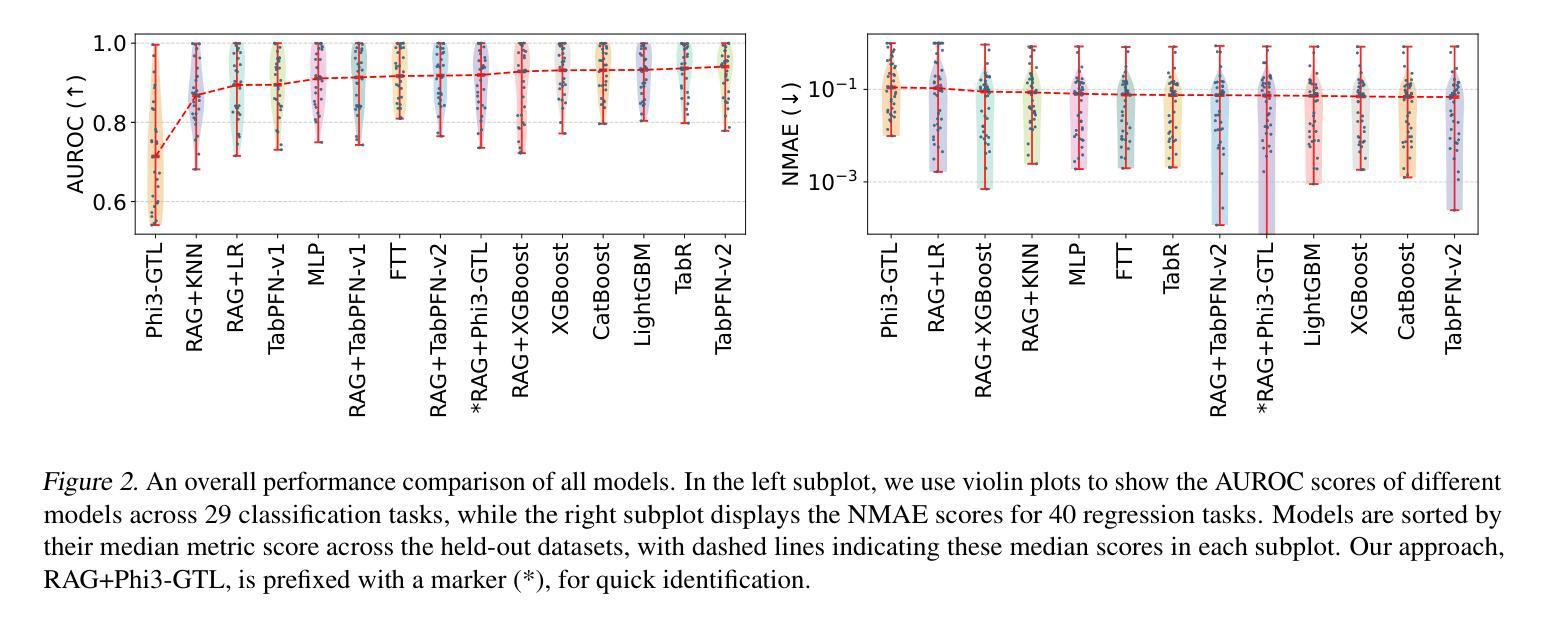
OmniRL: In-Context Reinforcement Learning by Large-Scale Meta-Training in Randomized Worlds
Authors:Fan Wang, Pengtao Shao, Yiming Zhang, Bo Yu, Shaoshan Liu, Ning Ding, Yang Cao, Yu Kang, Haifeng Wang
We introduce OmniRL, a highly generalizable in-context reinforcement learning (ICRL) model that is meta-trained on hundreds of thousands of diverse tasks. These tasks are procedurally generated by randomizing state transitions and rewards within Markov Decision Processes. To facilitate this extensive meta-training, we propose two key innovations: 1. An efficient data synthesis pipeline for ICRL, which leverages the interaction histories of diverse behavior policies; and 2. A novel modeling framework that integrates both imitation learning and reinforcement learning (RL) within the context, by incorporating prior knowledge. For the first time, we demonstrate that in-context learning (ICL) alone, without any gradient-based fine-tuning, can successfully tackle unseen Gymnasium tasks through imitation learning, online RL, or offline RL. Additionally, we show that achieving generalized ICRL capabilities-unlike task identification-oriented few-shot learning-critically depends on long trajectories generated by variant tasks and diverse behavior policies. By emphasizing the potential of ICL and departing from pre-training focused on acquiring specific skills, we further underscore the significance of meta-training aimed at cultivating the ability of ICL itself.
我们介绍了OmniRL,这是一个高度通用的上下文强化学习(ICRL)模型,它在数十万个不同的任务上进行元训练。这些任务是通过在马尔可夫决策过程中随机化状态转换和奖励来程序生成的。为了促进这种广泛的元训练,我们提出了两项关键创新:1.为ICRL设计的有效数据合成管道,它利用了各种行为策略的交互历史;2.一种新型建模框架,通过融入先验知识,将模仿学习和强化学习(RL)结合在上下文之中。我们首次证明,仅通过上下文学习(ICL),无需任何基于梯度的微调,就可以通过模仿学习、在线强化学习或离线强化学习成功解决未见过的Gym任务。此外,我们表明,与面向任务识别的少样本学习不同,实现通用的ICRL功能严重依赖于由不同任务和多样行为策略产生的长轨迹。我们强调ICL的潜力,并侧重于摆脱以获取特定技能为重点的预训练,进一步突显以培养ICL能力为目标的元训练的重要性。
论文及项目相关链接
PDF Preprint
Summary
奥咪RL模型是一种高度通用的上下文强化学习(ICRL)模型,它通过百万级别的多样化任务进行元训练。该模型利用马尔可夫决策过程中的状态转换和奖励的随机性来程序化生成这些任务。为了实现广泛的元训练,该论文提出了两个关键的创新点:一是为ICRL设计的有效数据合成管道,它利用各种行为策略的交互历史;二是整合模仿学习和强化学习(RL)的新型建模框架。该论文首次证明,仅通过上下文学习(ICL),无需基于梯度的微调,就能成功解决未见的Gymnasium任务,包括模仿学习、在线RL或离线RL。实现广义ICRL能力关键在于长轨迹的生成依赖于多样化任务和多种行为策略,而不是面向任务识别的少样本学习。该论文强调了ICL的潜力,并强调偏离以获取特定技能为重点的预训练,进一步突出了以培养ICL能力为目标的元训练的重要性。
Key Takeaways
- OmniRL是一个高度通用的上下文强化学习(ICRL)模型,通过大量多样化任务进行元训练。
- OmniRL利用马尔可夫决策过程中的状态转换和奖励的随机性程序化生成任务。
- OmniRL提出了两个关键创新点:一个高效的数据合成管道用于ICRL,以及一个整合模仿学习和强化学习的建模框架。
- 仅通过上下文学习(ICL)就能解决未见的Gymnasium任务,包括模仿学习、在线RL或离线RL。
- 实现广义ICRL能力需要依赖于多样化任务和多种行为策略生成的长轨迹,而不是面向任务识别的少样本学习。
- 该论文强调以获取ICL能力为目标的元训练的重要性,而非以获取特定技能为重点的预训练。
点此查看论文截图
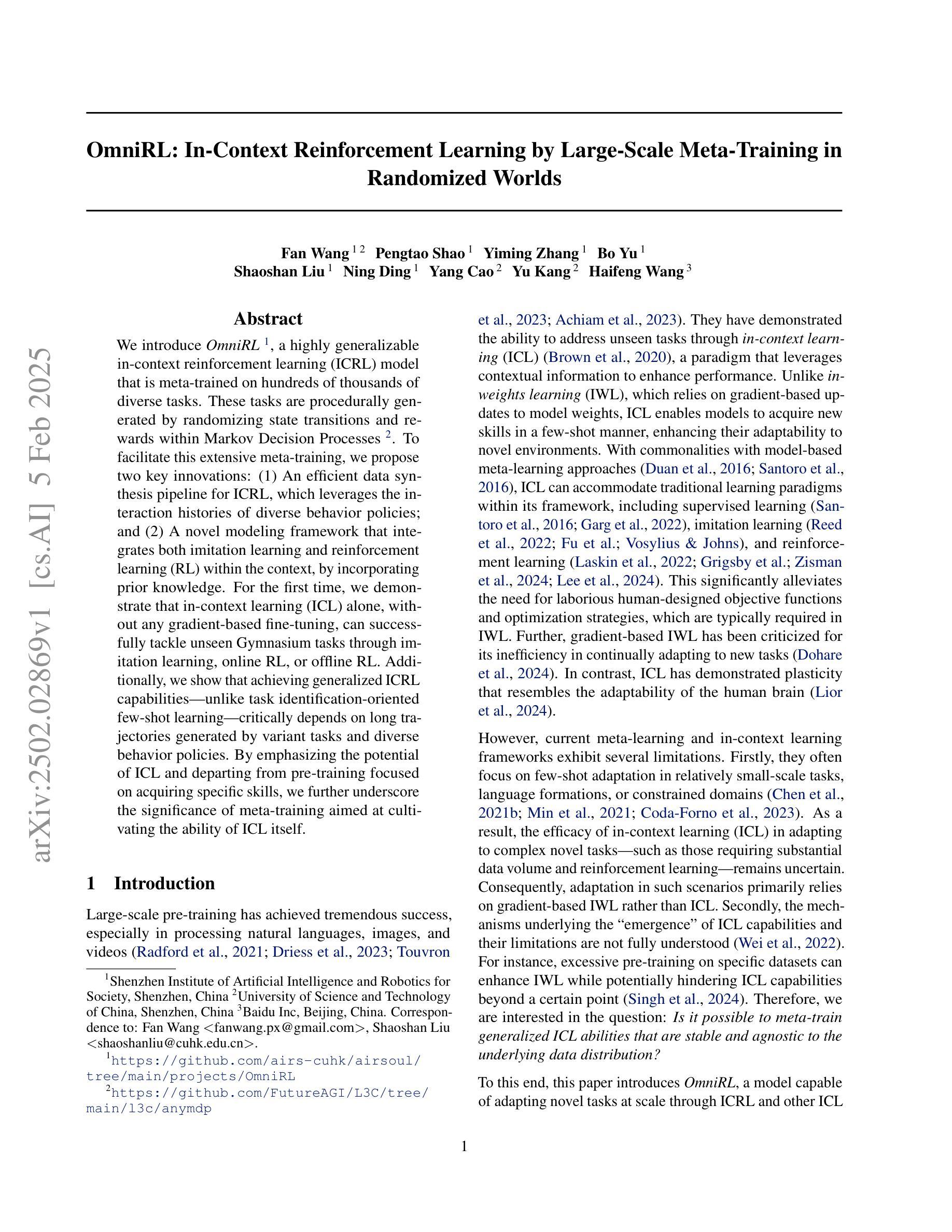
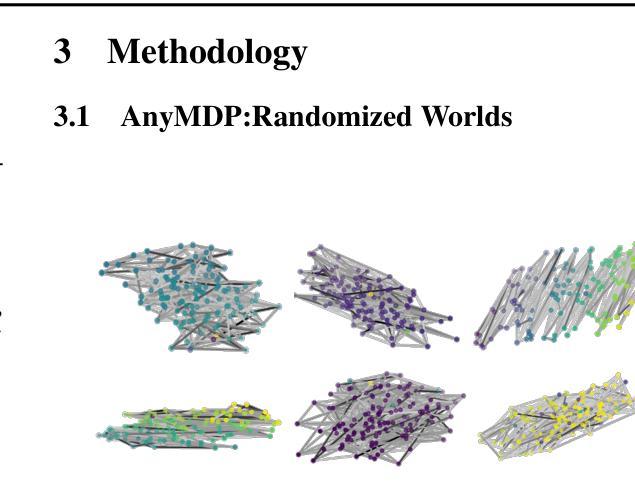
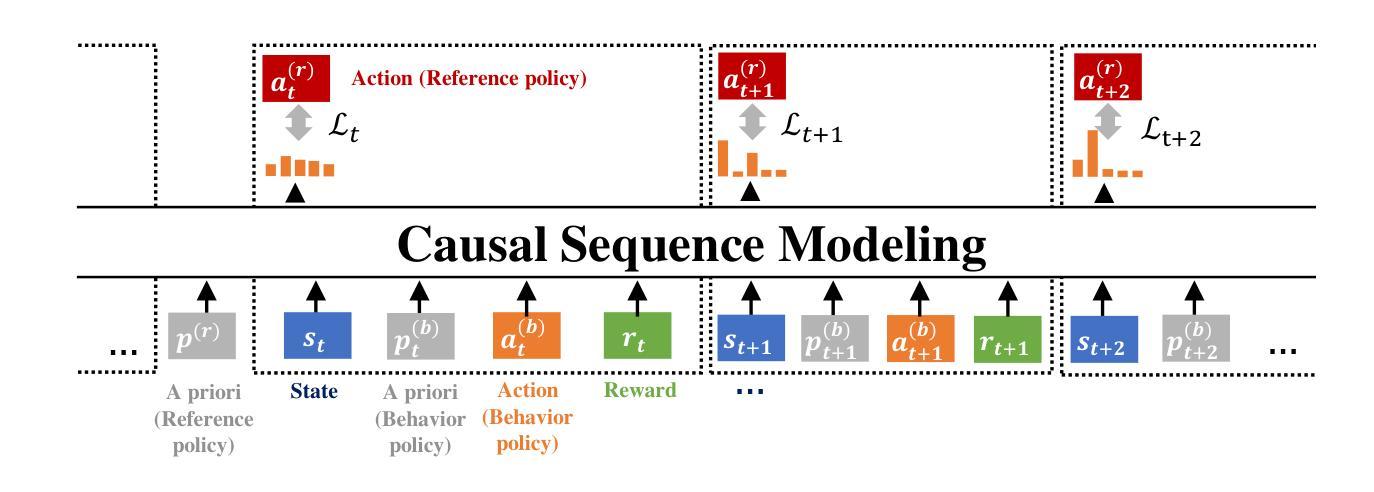
Transformers Boost the Performance of Decision Trees on Tabular Data across Sample Sizes
Authors:Mayuka Jayawardhana, Renbo Tu, Samuel Dooley, Valeriia Cherepanova, Andrew Gordon Wilson, Frank Hutter, Colin White, Tom Goldstein, Micah Goldblum
Large language models (LLMs) perform remarkably well on tabular datasets in zero- and few-shot settings, since they can extract meaning from natural language column headers that describe features and labels. Similarly, TabPFN, a recent non-LLM transformer pretrained on numerous tables for in-context learning, has demonstrated excellent performance for dataset sizes up to a thousand samples. In contrast, gradient-boosted decision trees (GBDTs) are typically trained from scratch on each dataset without benefiting from pretraining data and must learn the relationships between columns from their entries alone since they lack natural language understanding. LLMs and TabPFN excel on small tabular datasets where a strong prior is essential, yet they are not competitive with GBDTs on medium or large datasets, since their context lengths are limited. In this paper, we propose a simple and lightweight approach for fusing large language models and TabPFN with gradient-boosted decision trees, which allows scalable GBDTs to benefit from the natural language capabilities and pretraining of transformers. We name our fusion methods LLM-Boost and PFN-Boost, respectively. While matching or surpassing the performance of the transformer at sufficiently small dataset sizes and GBDTs at sufficiently large sizes, LLM-Boost and PFN-Boost outperform both standalone components on a wide range of dataset sizes in between. We demonstrate state-of-the-art performance against numerous baselines and ensembling algorithms. We find that PFN-Boost achieves the best average performance among all methods we test for all but very small dataset sizes. We release our code at http://github.com/MayukaJ/LLM-Boost .
大型语言模型(LLM)在零样本和少样本设置下的表格数据集上表现非常出色,因为它们可以从描述特征和标签的自然语言列标题中提取意义。类似地,TabPFN作为一个最近的非LLM转换器,在大量表格上进行预训练以进行上下文学习,已显示出在多达一千个样本的数据集上表现出色。相比之下,梯度提升决策树(GBDT)通常会在每个数据集上进行从头训练,而无法受益于预训练数据,并且它们仅能从条目本身学习列之间的关系,因为它们缺乏自然语言理解能力。LLM和TabPFN在小型表格数据集中表现出色,其中强大的先验知识至关重要,但在中等或大型数据集上,它们无法与GBDT竞争,因为它们的上下文长度有限。在本文中,我们提出了一种简单而轻量级的方法,用于将大型语言模型和TabPFN与梯度提升决策树融合,这使得可扩展的GBDT可以受益于转换器的自然语言能力和预训练。我们将我们的融合方法分别命名为LLM-Boost和PFN-Boost。尽管在小数据集上LLM-Boost和PFN-Boost的性能与转换器相匹配或超过它,并且在足够大的数据集上GBDT的性能相匹配或超过它,但它们在各种中间数据集大小上的表现均优于这两个独立组件。我们与众多基准线和集成算法相比,展现了最佳性能。我们发现,除了非常小的数据集外,PFN-Boost在所有测试方法中取得了最佳的平均性能。我们在http://github.com/MayukaJ/LLM-Boost上发布了我们的代码。
论文及项目相关链接
PDF 12 pages, 6 figures
Summary
大型语言模型(LLMs)在零样本和少样本环境下处理表格数据集时表现出色,因为它们可以从描述特征和标签的自然语言列标题中提取意义。相比之下,梯度提升决策树(GBDTs)通常针对每个数据集从头开始训练,无法从预训练数据中受益,并且必须仅从条目本身中学习列之间的关系。LLMs和TabPFN在小型表格数据集上表现出色,其中强先验知识至关重要,但在中等或大型数据集上无法与GBDTs竞争。本文提出了一种简单而轻量级的方法,将大型语言模型和TabPFN与梯度提升决策树融合,使可扩展的GBDTs受益于变换器的自然语言能力和预训练。我们分别将融合方法命名为LLM-Boost和PFN-Boost。当数据集大小足够小和足够大时,LLM-Boost和PFN-Boost分别匹配或超越变换器和GBDTs的性能,但在两者之间的一系列数据集大小上表现出优于两者的性能。我们在众多基准测试和集成算法上展示了卓越的性能。我们发现,除非常小的数据集大小外,PFN-Boost在所有方法中实现了最佳的平均性能。我们的代码已发布在http://github.com/MayukaJ/LLM-Boost。
Key Takeaways
- LLMs在零样本和少样本环境下处理表格数据集时具有优势,得益于它们对自然语言的深刻理解。
- TabPFN预训练的表格理解能力在非大型数据集上表现优秀。
- 梯度提升决策树(GBDTs)通常不依赖预训练数据,但在处理中等或大型数据集时表现良好。
- LLM-Boost和PFN-Boost方法融合了LLMs和TabPFN的优点,提升了GBDTs的性能。
- LLM-Boost和PFN-Boost在不同数据集大小上均表现出卓越性能,超越了单纯的LLMs和GBDTs。
- PFN-Boost在大多数数据集大小上实现了最佳的平均性能。
点此查看论文截图
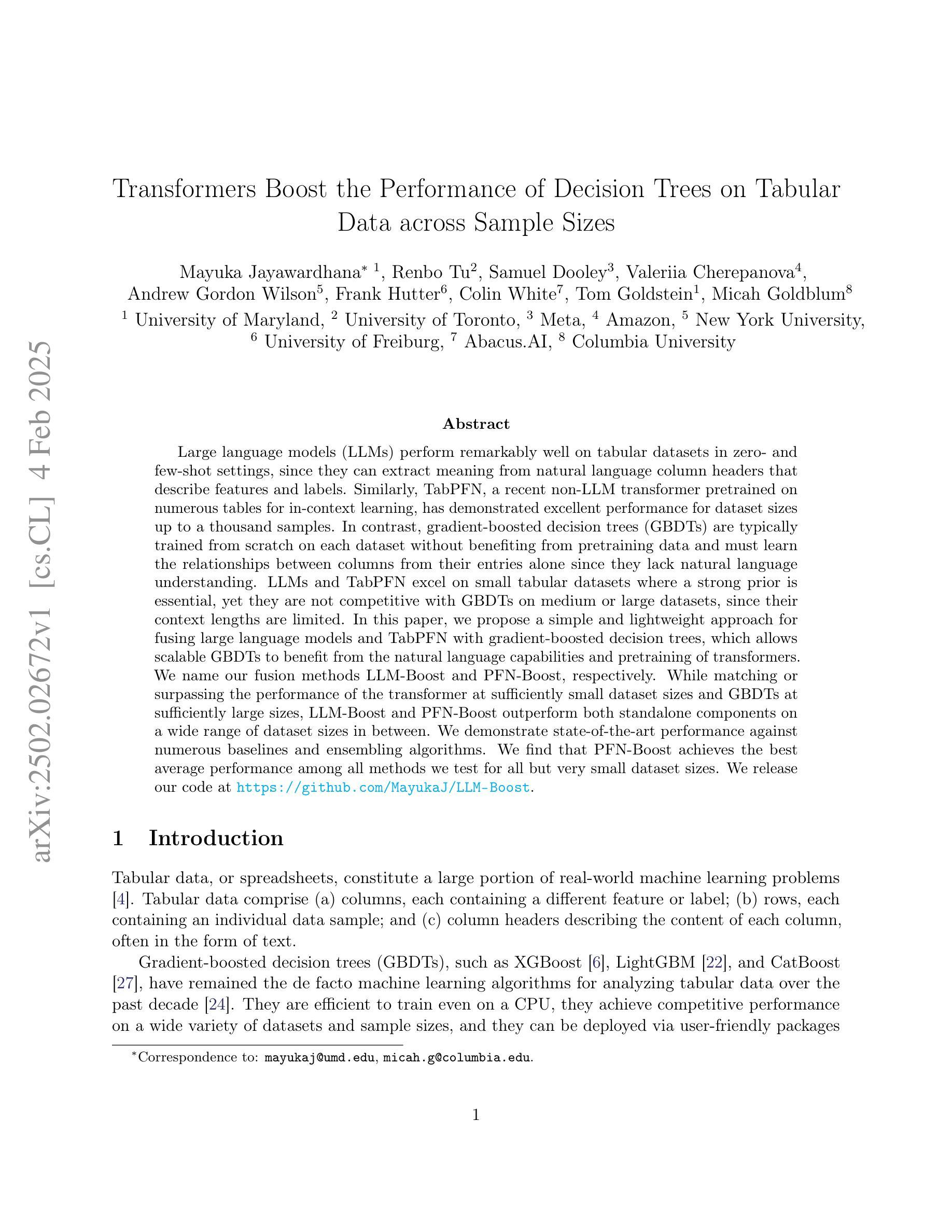
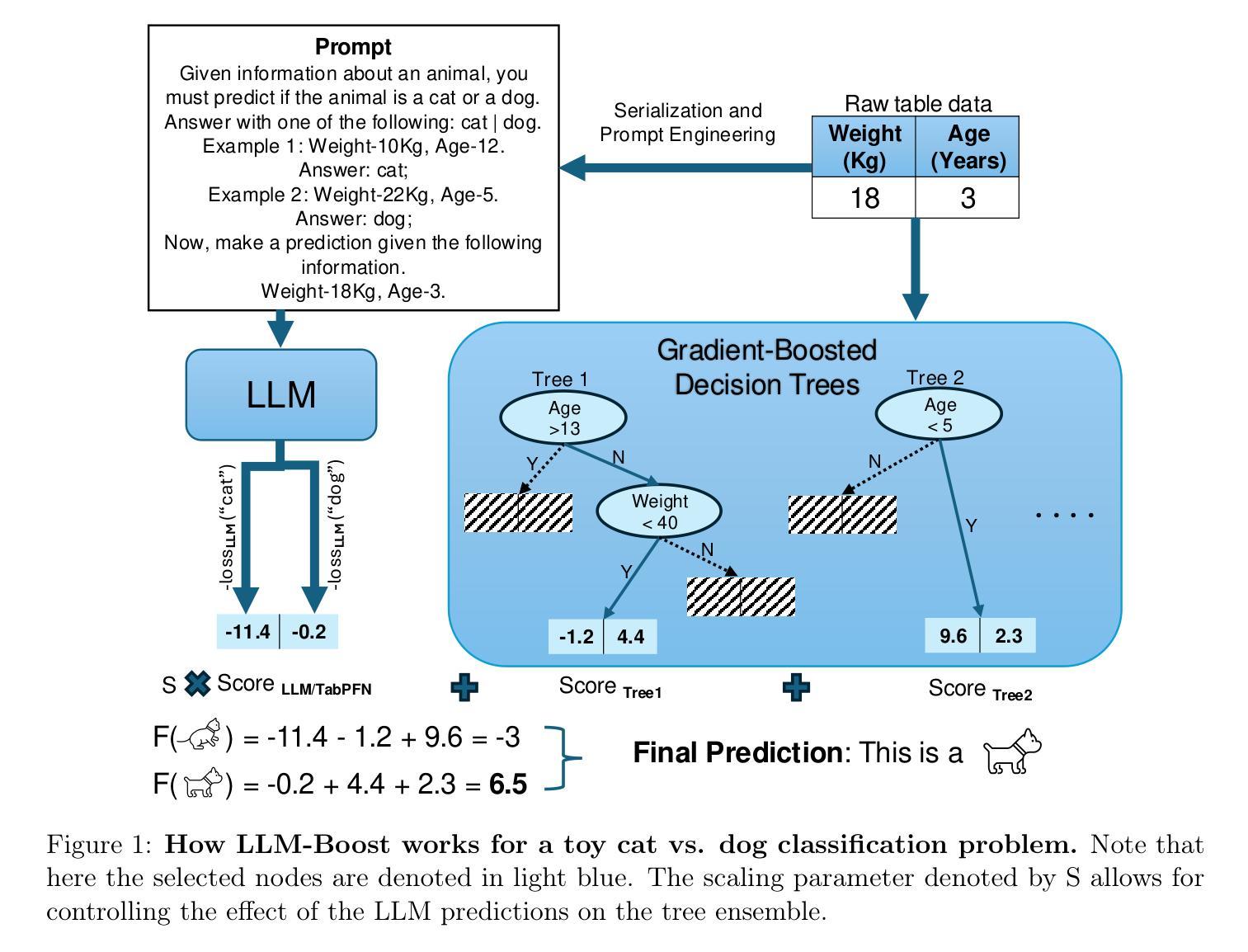
BARE: Combining Base and Instruction-Tuned Language Models for Better Synthetic Data Generation
Authors:Alan Zhu, Parth Asawa, Jared Quincy Davis, Lingjiao Chen, Boris Hanin, Ion Stoica, Joseph E. Gonzalez, Matei Zaharia
As the demand for high-quality data in model training grows, researchers and developers are increasingly generating synthetic data to tune and train LLMs. A common assumption about synthetic data is that sampling from instruct-tuned models is sufficient; however, these models struggle to produce diverse outputs-a key requirement for generalization. Despite various prompting methods, in this work we show that achieving meaningful diversity from instruct-tuned models remains challenging. In contrast, we find base models without post-training exhibit greater diversity, but are less capable at instruction following and hence of lower quality. Leveraging this insight, we propose Base-Refine (BARE), a synthetic data generation method that combines the diversity of base models with the quality of instruct-tuned models through a two-stage process. With minimal few-shot examples and curation, BARE generates diverse and high-quality datasets, improving downstream task performance. We show that fine-tuning with as few as 1,000 BARE-generated samples can reach performance comparable to the best similarly sized models on LiveCodeBench tasks. Furthermore, fine-tuning with BARE-generated data achieves a 101% improvement over instruct-only data on GSM8K and a 18.4% improvement over SOTA methods on RAFT.
随着模型训练中对高质量数据的需求不断增长,研究者和开发者们正在越来越多地生成合成数据来调整和优化大型语言模型(LLMs)。关于合成数据的常见假设是,从指令优化模型中采样就足够了;然而,这些模型在产生多样化输出方面存在困难,这是泛化的一个关键要求。尽管有各种提示方法,但在这项工作中,我们表明从指令优化模型中实现有意义的多样性仍然具有挑战性。相比之下,我们发现没有经过后训练的基准模型表现出更大的多样性,但在遵循指令方面的能力较差,因此质量较低。我们利用这一见解,提出了Base-Refine(BARE)方法,这是一种合成数据生成方法,它通过两阶段过程结合基准模型的多样性和指令优化模型的质量。通过少量的示例和筛选,BARE可以生成多样且高质量的数据集,提高下游任务性能。我们展示使用仅1000个BARE生成样本进行微调时,在LiveCodeBench任务上的性能可与类似大小的最佳模型相当。此外,使用BARE生成的数据进行微调在GSM8K上实现了比仅使用指令数据提高101%的性能,并在RAFT上实现了比最先进方法提高18.4%的效果。
论文及项目相关链接
Summary
生成高质量数据对于模型训练至关重要,研究者利用合成数据来调整和优化大型语言模型(LLMs)。尽管存在各种提示方法,但发现从指令调整模型中实现有意义的多样性仍然具有挑战性。相反,研究发现未经训练的基准模型展现出更大的多样性,但在指令遵循方面能力较低。基于此,提出了结合基准模型的多样性和指令调整模型的质量的合成数据生成方法——Base-Refine(BARE)。通过最少的几次示例和筛选,BARE生成了多样且高质量的数据集,提高了下游任务性能。在LiveCodeBench任务上,使用BARE生成的样本进行微调达到了出色的性能表现。相较于指令仅涉及的数据,使用BARE生成的微调数据在GSM8K上实现了101%的提升,并在RAFT任务上比最先进的处理方法提升了约达至有特定的表达目的的智能和思维方式已经明确的文化准则相近的个人所处的当下所面临的政治环境等要素都使得翻译变得更为复杂和困难。翻译不仅需要语言层面的转换,还需要对文化语境、社会背景、历史背景等进行深入理解。因此,翻译是一项需要高度专业知识和技能的工作。随着全球化的不断发展,翻译行业的前景广阔,对于具备专业技能和经验的翻译人才的需求也在不断增加。同时,随着人工智能技术的不断进步,机器翻译也在不断发展,但机器翻译仍然无法完全取代人工翻译的地位。未来翻译行业将朝着更加专业化和精细化的方向发展,对于翻译人才的要求也将不断提高。在此基础上推动行业的发展,不断挑战自我技能与适应环境变化成为未来译者职业发展的重要方向之一。此次对话表明了一个共同的认识:机器虽然强大但仍无法取代人的智能与创造力这是机器翻译未来发展中需要面对的重要挑战之一。,还取得了显著的提升效果。
Key Takeaways
- 生成合成数据对于训练LLMs至关重要。
- 指令调整模型在生成多样输出方面存在挑战。
- 未经训练的基准模型展现出更大的多样性但在指令遵循方面能力较低。
- BARE方法结合了基准模型的多样性和指令调整模型的质量。
- 使用BARE生成的少量样本进行微调可达到出色的性能表现。
- BARE方法在多个任务上实现了显著的性能提升。
点此查看论文截图
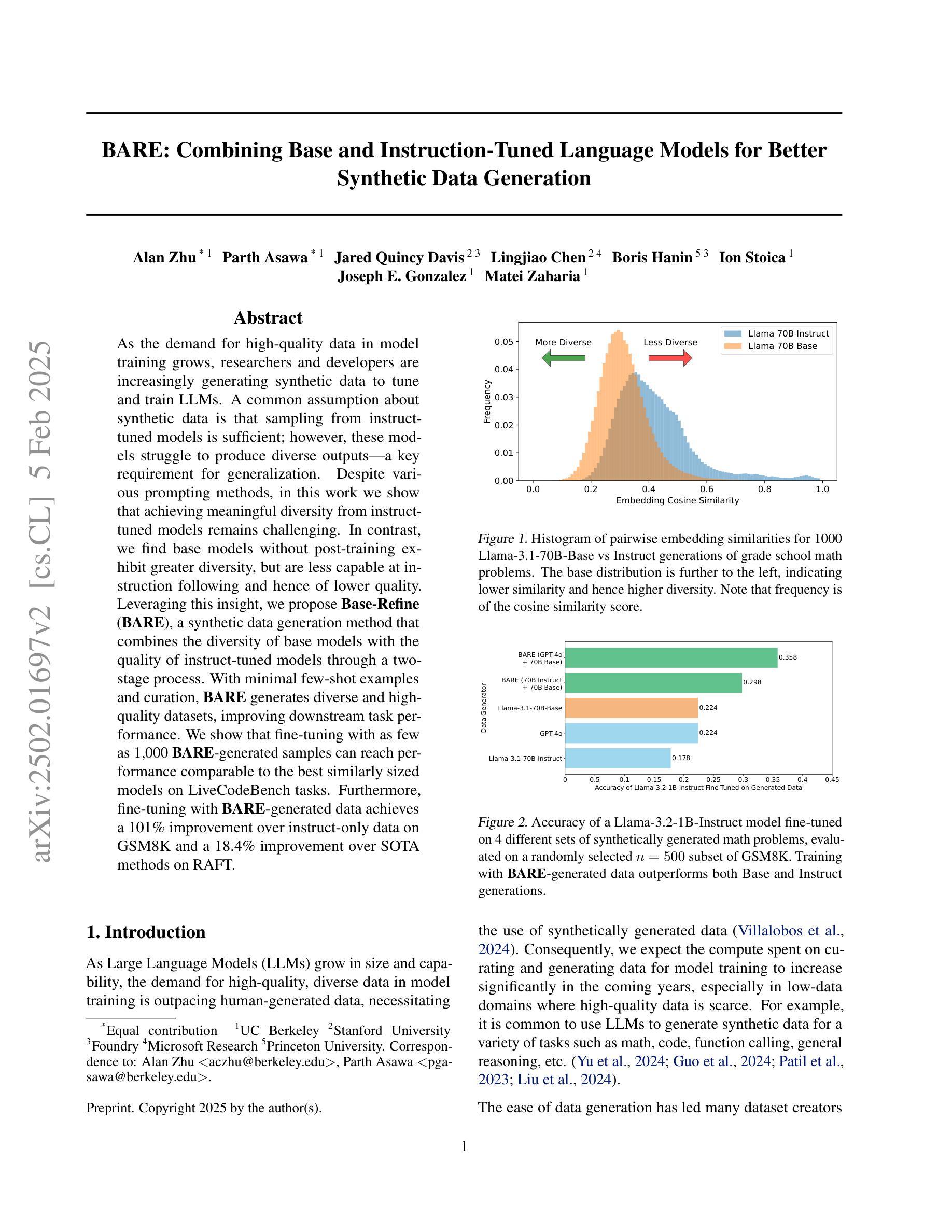
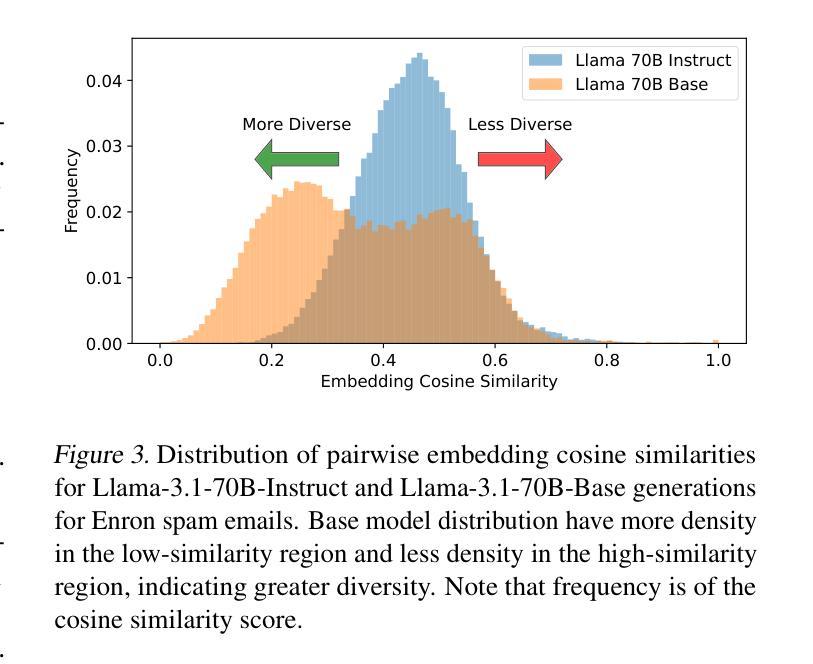
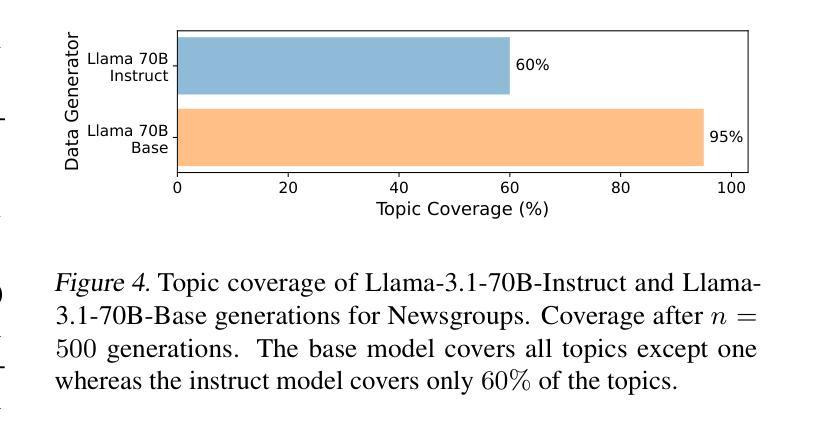
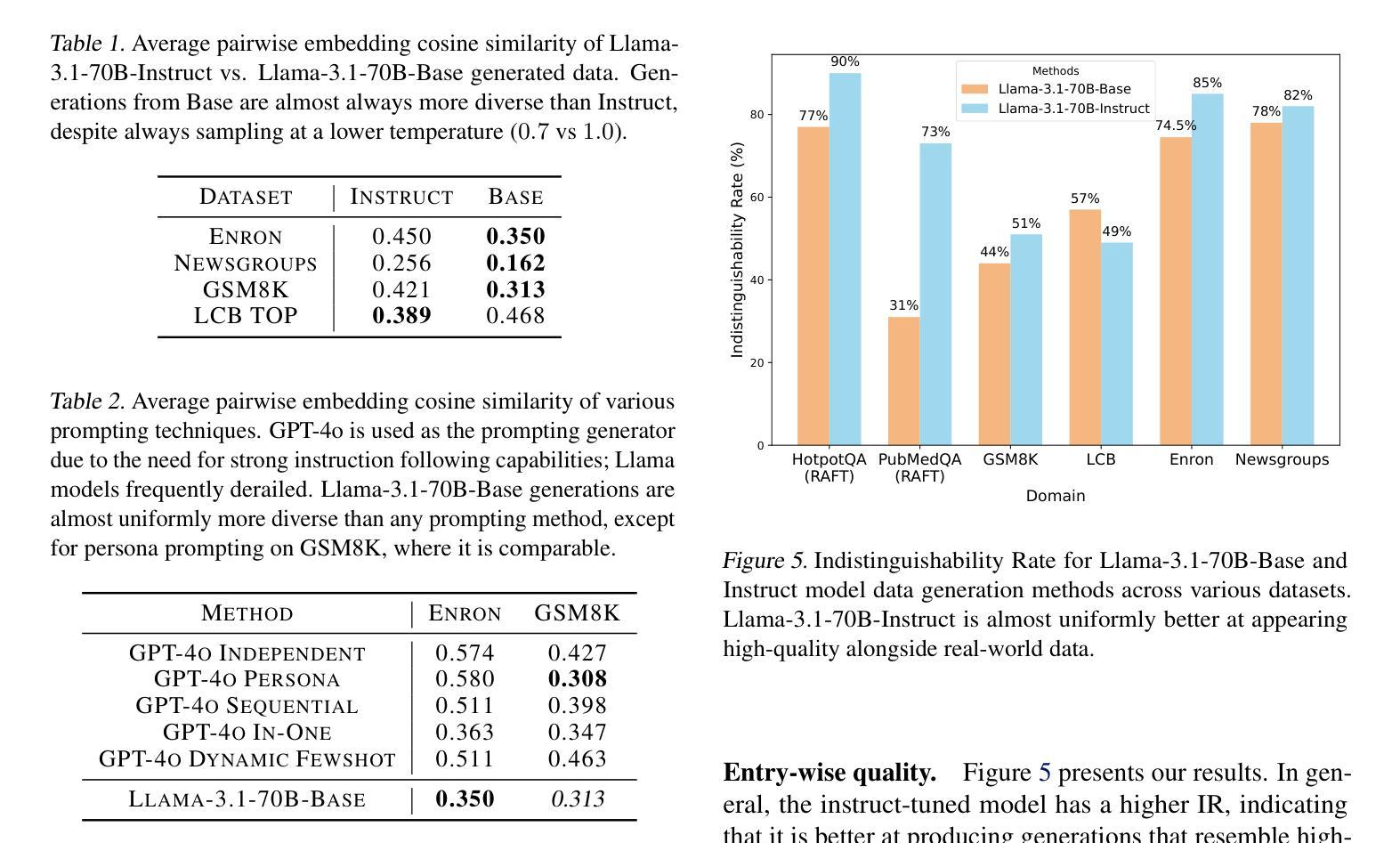
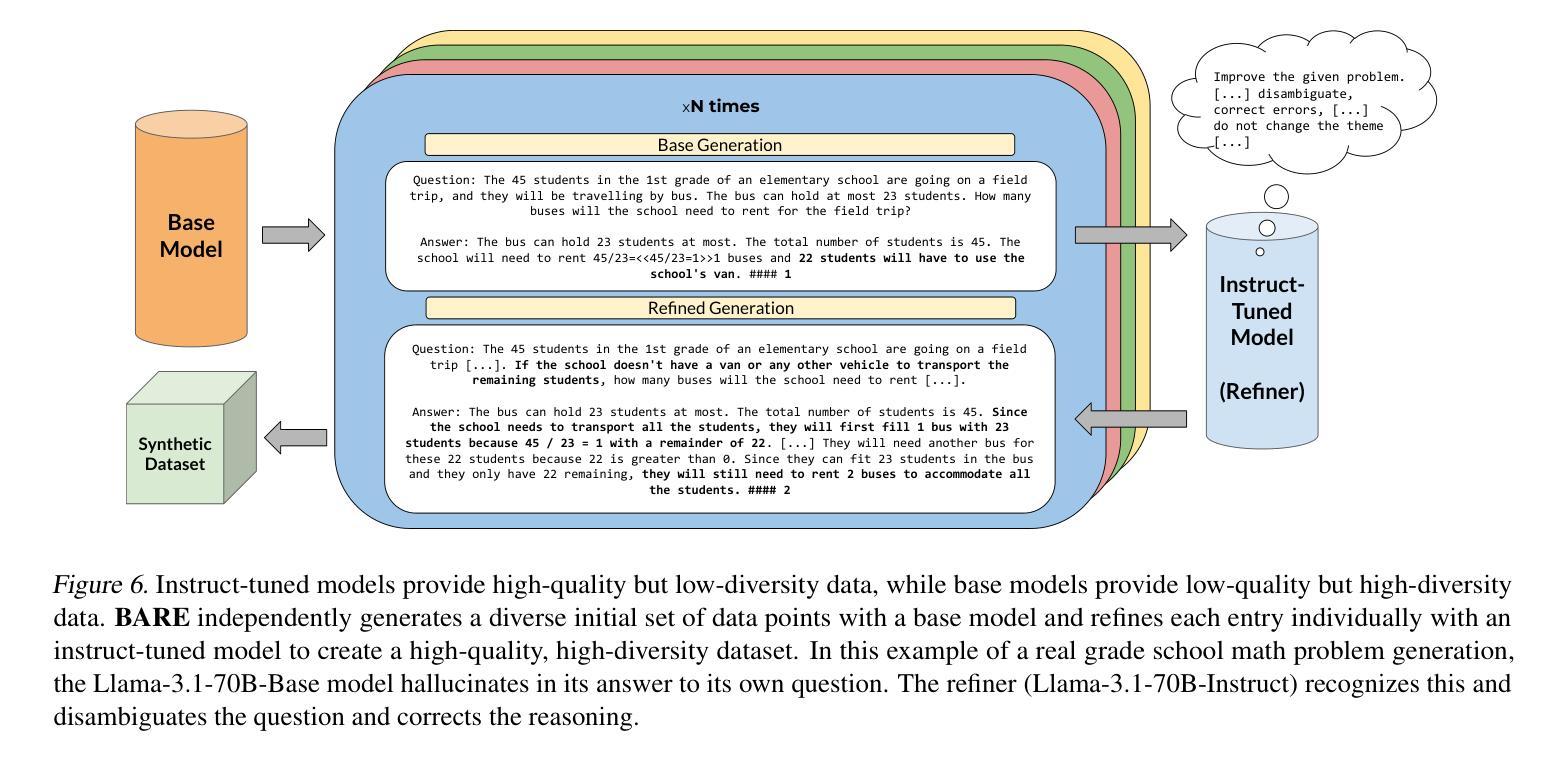
Aggregation Artifacts in Subjective Tasks Collapse Large Language Models’ Posteriors
Authors:Georgios Chochlakis, Alexandros Potamianos, Kristina Lerman, Shrikanth Narayanan
In-context Learning (ICL) has become the primary method for performing natural language tasks with Large Language Models (LLMs). The knowledge acquired during pre-training is crucial for this few-shot capability, providing the model with task priors. However, recent studies have shown that ICL predominantly relies on retrieving task priors rather than “learning” to perform tasks. This limitation is particularly evident in complex subjective domains such as emotion and morality, where priors significantly influence posterior predictions. In this work, we examine whether this is the result of the aggregation used in corresponding datasets, where trying to combine low-agreement, disparate annotations might lead to annotation artifacts that create detrimental noise in the prompt. Moreover, we evaluate the posterior bias towards certain annotators by grounding our study in appropriate, quantitative measures of LLM priors. Our results indicate that aggregation is a confounding factor in the modeling of subjective tasks, and advocate focusing on modeling individuals instead. However, aggregation does not explain the entire gap between ICL and the state of the art, meaning other factors in such tasks also account for the observed phenomena. Finally, by rigorously studying annotator-level labels, we find that it is possible for minority annotators to both better align with LLMs and have their perspectives further amplified.
上下文学习(ICL)已成为使用大型语言模型(LLM)执行自然语言任务的主要方法。在预训练期间获得的知识对于这种小样本能力至关重要,为模型提供任务先验。然而,最近的研究表明,ICL主要依赖于检索任务先验,而不是“学习”执行任务。在情感、道德等复杂的主观领域,这种局限性尤为明显,先验知识对后验预测有重大影响。在这项工作中,我们调查这是否是由于相应数据集中使用的聚合方法造成的。尝试将低同意度、分散的注释结合起来可能会导致注释伪像,在提示中产生有害的噪音。此外,我们通过基于定量的大型语言模型先验知识衡量方法,评估了对某些注释器的后验偏见。我们的结果表明,聚合是主观任务建模中的混淆因素,并主张重点对个体进行建模。然而,聚合并不能完全解释ICL与最新技术之间的差距,这意味着此类任务中的其他因素也导致了观察到的现象。最后,通过对注释器级别的标签进行严格研究,我们发现少数注释器与大型语言模型更加吻合,并且他们的观点得到了进一步的放大。
论文及项目相关链接
PDF 16 pages, 12 figures, 3 tables
Summary
大型语言模型(LLM)进行自然语言任务时主要依赖上下文学习(ICL),其关键在于预训练阶段获取的知识,为模型提供了任务先验信息。但研究表明,ICL主要依赖检索任务先验信息而非“学习”执行任务。在情感、道德等复杂主观领域,这种局限性尤为明显。本文探讨了这一现象是否由数据集使用的聚合方法导致,尝试将低一致性、分散的注释结合起来可能产生注释伪影,给提示带来有害噪声。同时评估了模型对特定注释者的后验偏见。研究结果表明,聚合是模拟主观任务中的混淆因素,并主张将重点放在模拟个人上。然而,聚合并不能完全解释ICL与当前技术水平之间的差距,意味着还有其他因素也影响了观察到的现象。通过深入研究注释器级别的标签,发现少数注释器与LLM更匹配,他们的观点也得到了进一步的放大。
Key Takeaways
- ICL成为LLM执行自然语言任务的主要方法,依赖于预训练知识提供的任务先验信息。
- ICL在复杂主观任务中主要依赖检索任务先验而非学习过程。
- 数据集的聚合方法是影响模型表现的因素之一,尝试结合低一致性、分散的注释可能产生注释伪影。
- 聚合方法对模拟主观任务有混淆作用,应关注模拟个人层面的方法。
- 后验偏向特定注释者可能影响模型表现,需要对此进行深入研究。
- 聚合并不能完全解释ICL与当前技术水平的差距,其他因素也影响任务表现。
点此查看论文截图
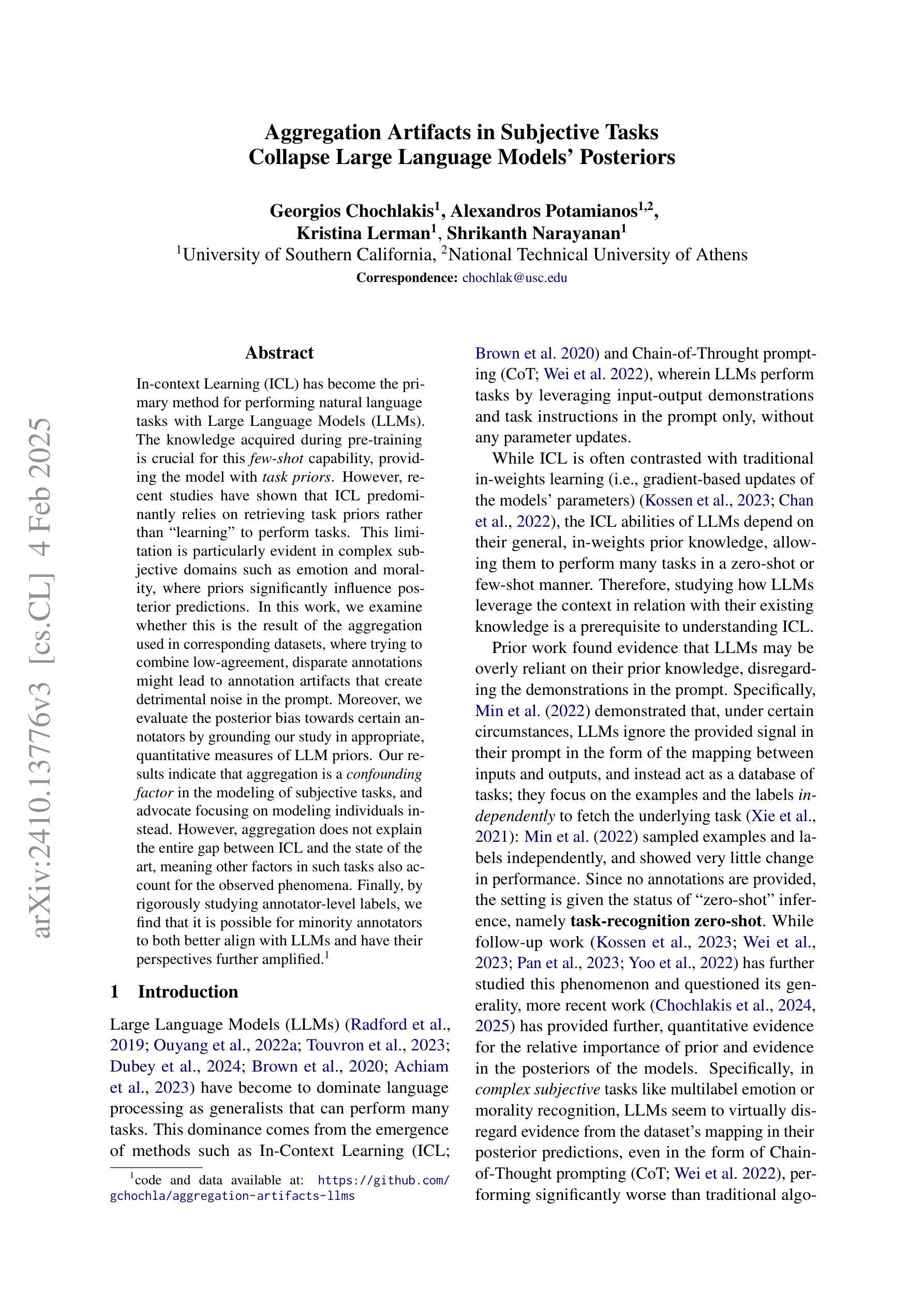
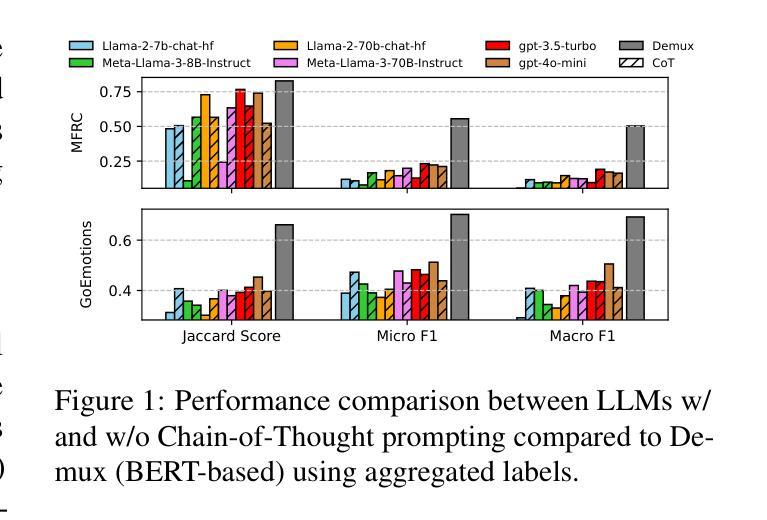
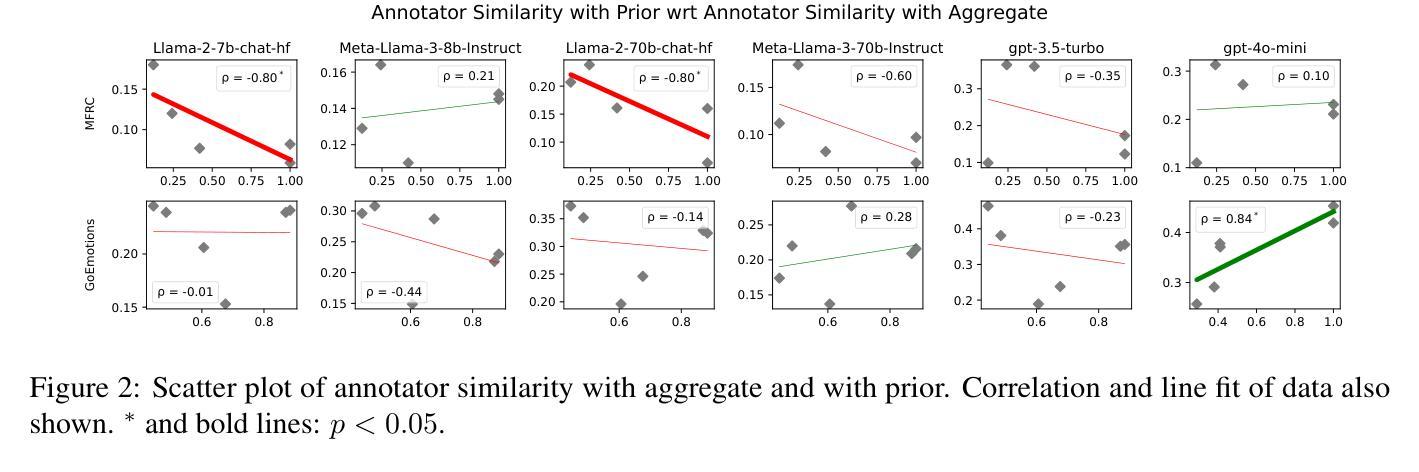
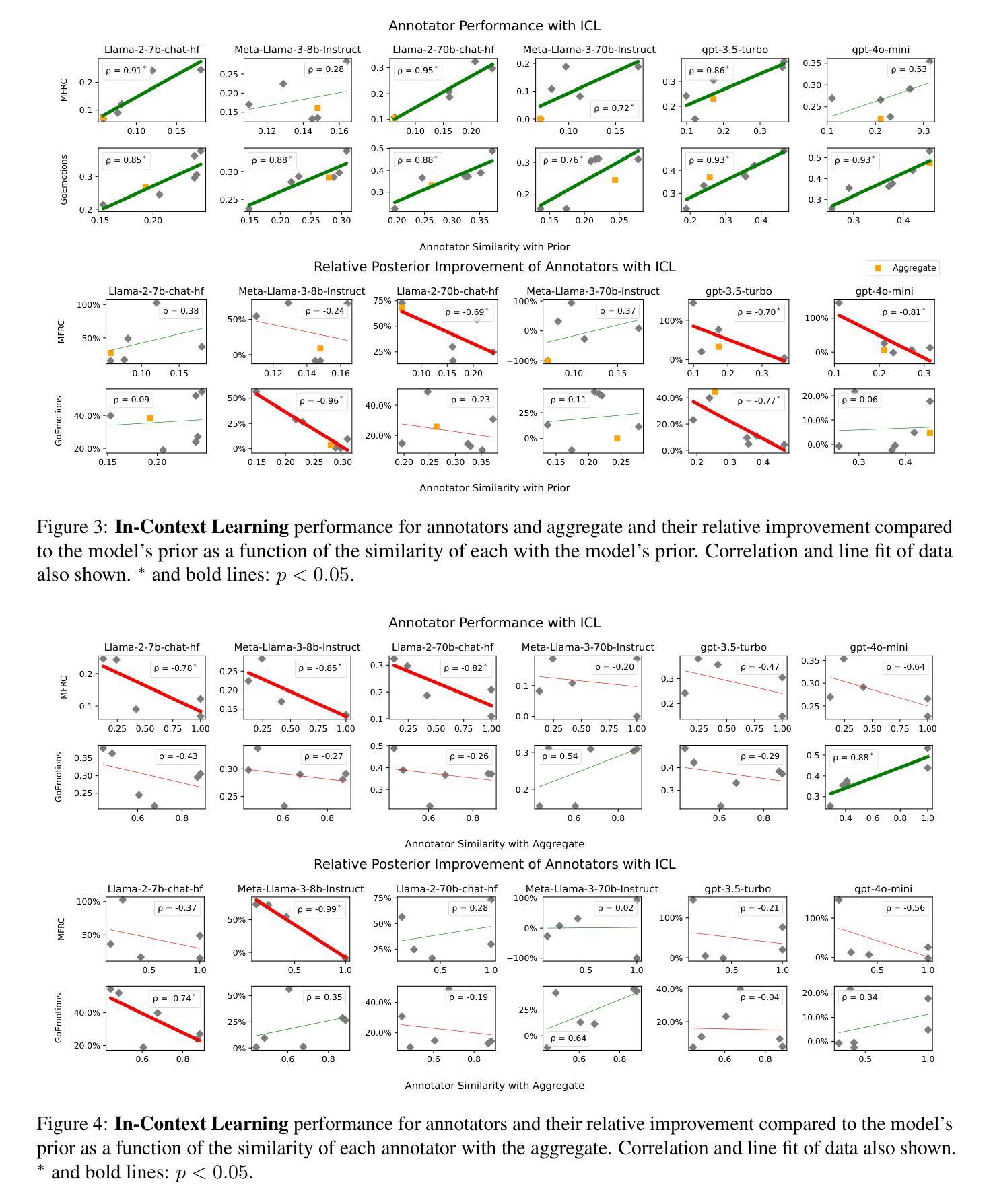
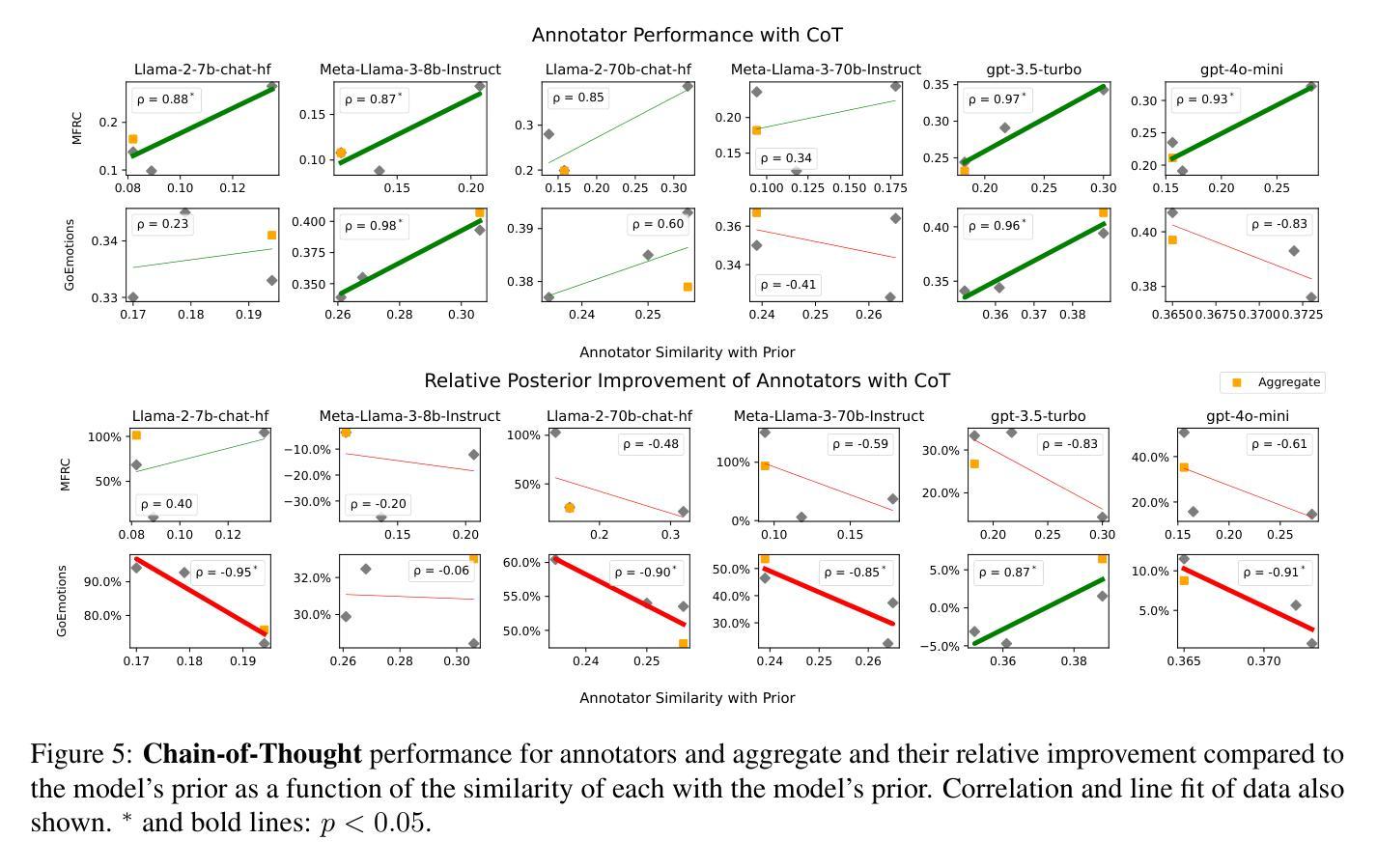
Agent-OM: Leveraging LLM Agents for Ontology Matching
Authors:Zhangcheng Qiang, Weiqing Wang, Kerry Taylor
Ontology matching (OM) enables semantic interoperability between different ontologies and resolves their conceptual heterogeneity by aligning related entities. OM systems currently have two prevailing design paradigms: conventional knowledge-based expert systems and newer machine learning-based predictive systems. While large language models (LLMs) and LLM agents have revolutionised data engineering and have been applied creatively in many domains, their potential for OM remains underexplored. This study introduces a novel agent-powered LLM-based design paradigm for OM systems. With consideration of several specific challenges in leveraging LLM agents for OM, we propose a generic framework, namely Agent-OM (Agent for Ontology Matching), consisting of two Siamese agents for retrieval and matching, with a set of OM tools. Our framework is implemented in a proof-of-concept system. Evaluations of three Ontology Alignment Evaluation Initiative (OAEI) tracks over state-of-the-art OM systems show that our system can achieve results very close to the long-standing best performance on simple OM tasks and can significantly improve the performance on complex and few-shot OM tasks.
本体匹配(OM)通过使不同本体之间实现语义互操作性,并通过对齐相关实体解决其概念上的异质性。目前,OM系统主要有两种流行的设计范式:传统的基于知识的专家系统和较新的基于机器学习的预测系统。虽然大型语言模型(LLM)和LLM代理已经彻底改变了数据工程,并在许多领域得到了创造性的应用,但它们在OM中的潜力仍未得到充分探索。本研究引入了一种新型基于LLM的代理驱动OM系统设计范式。考虑到利用LLM代理进行OM面临的若干特定挑战,我们提出了一个通用框架,即Agent-OM(用于本体匹配的代理),它由两个用于检索和匹配的Siamese代理和一组OM工具组成。我们的框架在一个概念验证系统中实现。对三个本体对齐评估倡议(OAEI)赛道上的最新OM系统的评估表明,我们的系统在简单OM任务上的结果非常接近长期以来的最佳性能,并且在复杂和少量射击OM任务上可以显著提高性能。
论文及项目相关链接
PDF 19 pages, 12 figures, 3 tables
Summary
本研究提出一种基于新型智能体辅助的大型语言模型(LLM)设计范式,用于本体匹配(OM)系统。通过考虑利用LLM智能体进行OM所面临的特定挑战,研究团队提出了一个通用框架Agent-OM(用于本体匹配的智能体),包含两个用于检索和匹配的Siamese智能体以及一套OM工具。在概念验证系统中实现了该框架,评估结果显示,该系统在简单OM任务上的表现接近最佳,且在复杂和少量样本OM任务上能显著提高性能。
Key Takeaways
- 本研究提出了一种新的基于智能体的LLM设计范式用于OM系统。
- LLM和智能体在本体匹配中的潜力尚未得到充分探索。
- Agent-OM框架包含两个Siamese智能体,用于检索和匹配,以及一套OM工具。
- 该框架解决了利用LLM智能体进行OM的特定挑战。
- 系统在简单OM任务上的表现接近最佳。
- 系统在复杂和少量样本OM任务上能显著提高性能。
点此查看论文截图
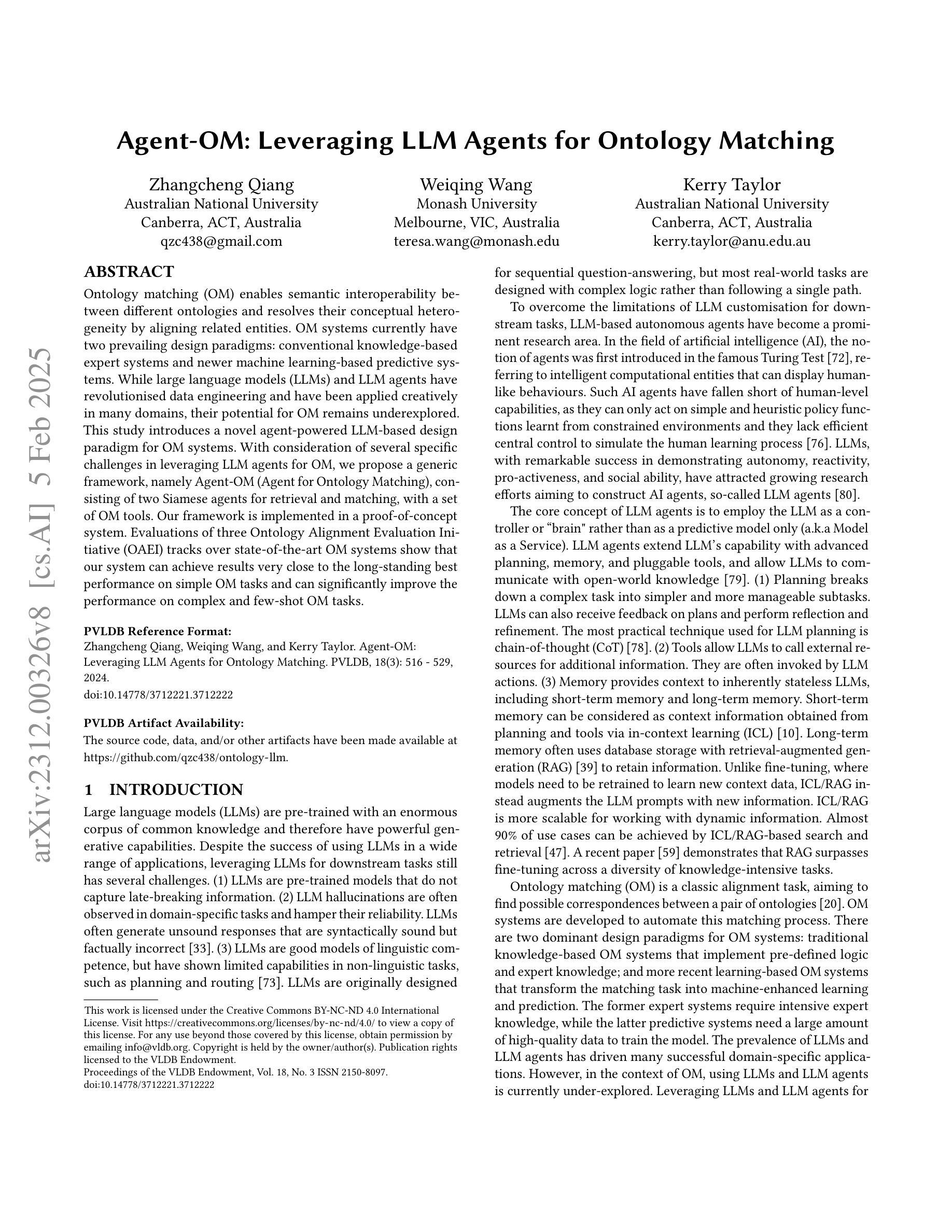
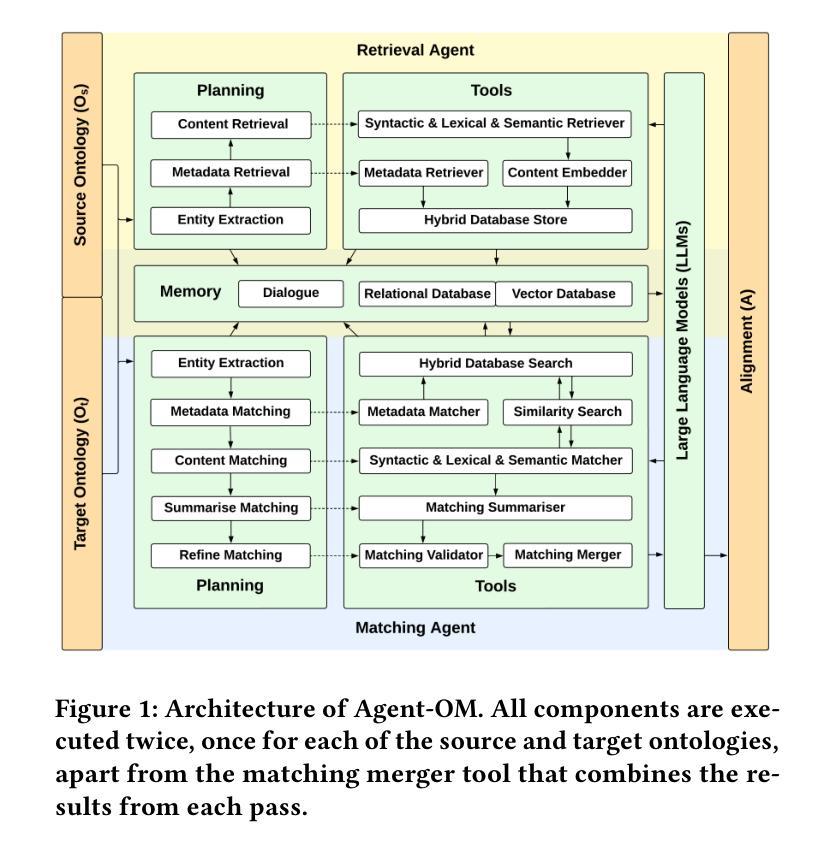
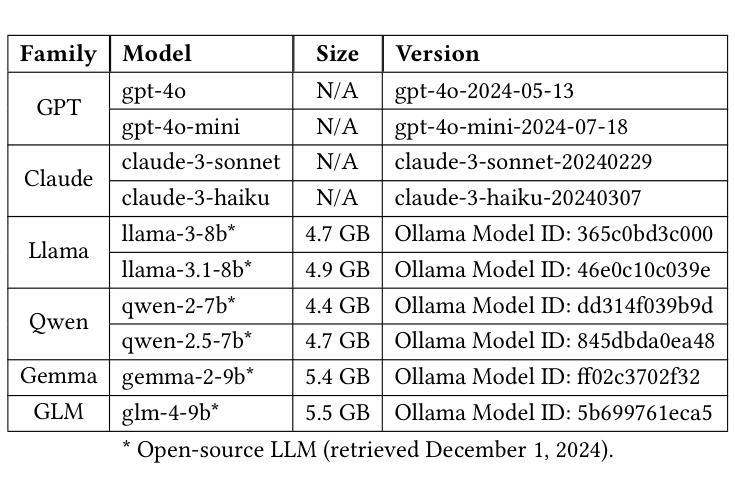
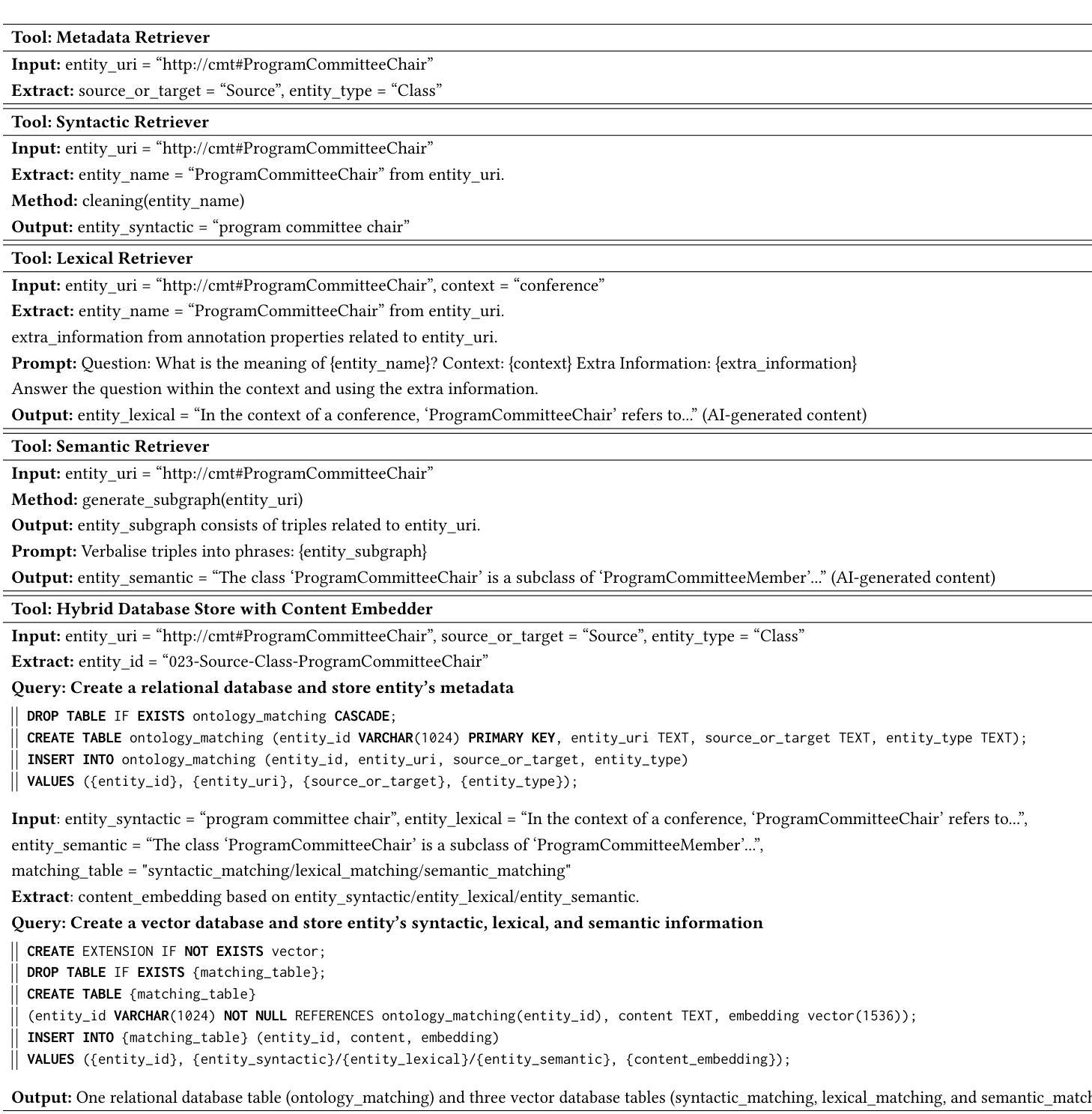