⚠️ 以下所有内容总结都来自于 大语言模型的能力,如有错误,仅供参考,谨慎使用
🔴 请注意:千万不要用于严肃的学术场景,只能用于论文阅读前的初筛!
💗 如果您觉得我们的项目对您有帮助 ChatPaperFree ,还请您给我们一些鼓励!⭐️ HuggingFace免费体验
2025-02-07 更新
AAD-DCE: An Aggregated Multimodal Attention Mechanism for Early and Late Dynamic Contrast Enhanced Prostate MRI Synthesis
Authors:Divya Bharti, Sriprabha Ramanarayanan, Sadhana S, Kishore Kumar M, Keerthi Ram, Harsh Agarwal, Ramesh Venkatesan, Mohanasankar Sivaprakasam
Dynamic Contrast-Enhanced Magnetic Resonance Imaging (DCE-MRI) is a medical imaging technique that plays a crucial role in the detailed visualization and identification of tissue perfusion in abnormal lesions and radiological suggestions for biopsy. However, DCE-MRI involves the administration of a Gadolinium based (Gad) contrast agent, which is associated with a risk of toxicity in the body. Previous deep learning approaches that synthesize DCE-MR images employ unimodal non-contrast or low-dose contrast MRI images lacking focus on the local perfusion information within the anatomy of interest. We propose AAD-DCE, a generative adversarial network (GAN) with an aggregated attention discriminator module consisting of global and local discriminators. The discriminators provide a spatial embedded attention map to drive the generator to synthesize early and late response DCE-MRI images. Our method employs multimodal inputs - T2 weighted (T2W), Apparent Diffusion Coefficient (ADC), and T1 pre-contrast for image synthesis. Extensive comparative and ablation studies on the ProstateX dataset show that our model (i) is agnostic to various generator benchmarks and (ii) outperforms other DCE-MRI synthesis approaches with improvement margins of +0.64 dB PSNR, +0.0518 SSIM, -0.015 MAE for early response and +0.1 dB PSNR, +0.0424 SSIM, -0.021 MAE for late response, and (ii) emphasize the importance of attention ensembling. Our code is available at https://github.com/bhartidivya/AAD-DCE.
动态对比增强磁共振成像(DCE-MRI)是一种医学成像技术,对于异常病变中的组织灌注的详细可视化和识别以及放射学活检建议起到关键作用。然而,DCE-MRI涉及使用基于钆(Gad)的造影剂,这带来了体内毒性的风险。先前用于合成DCE-MR图像的深度学习技术主要使用非对比或低剂量对比MRI图像的单模态,缺乏关注解剖结构内局部灌注信息。我们提出了AAD-DCE,这是一个包含全局和局部鉴别器的生成对抗网络(GAN)聚合注意力鉴别器模块。鉴别器提供了空间嵌入注意力图,以驱动生成器合成早期和晚期响应DCE-MRI图像。我们的方法采用多模态输入——T2加权(T2W)、表观扩散系数(ADC)和T1预对比图像用于图像合成。在ProstateX数据集上的广泛比较和消融研究表明,我们的模型(i)不受各种生成器基准测试的影响;(ii)与其他DCE-MRI合成方法相比,早期响应的峰值信噪比(PSNR)提高了+0.64 dB、结构相似性(SSIM)提高了+0.0518、平均绝对误差(MAE)降低了-0.015,晚期响应的PSNR提高了+0.1 dB、SSIM提高了+0.0424、MAE降低了-0.021;(iii)强调了注意力集成的重要性。我们的代码可在https://github.com/bhartidivya/AAD-DCE处获取。
论文及项目相关链接
PDF Accepted at ICASSP 2025
Summary
本研究提出了AAD-DCE,一种基于生成对抗网络(GAN)的生成模型,用于合成动态对比增强磁共振成像(DCE-MRI)。该模型采用聚集注意力鉴别器模块,包含全局和局部鉴别器,可为合成图像提供空间嵌入注意力图。使用多模态输入,包括T2加权(T2W)、表观扩散系数(ADC)和T1预对比图像进行图像合成。在ProstateX数据集上的广泛比较和消融研究表明,该模型在早反应和晚反应方面都优于其他DCE-MRI合成方法。
Key Takeaways
- AAD-DCE是基于生成对抗网络(GAN)的模型,用于合成动态对比增强磁共振成像(DCE-MRI)。
- 该模型采用聚集注意力鉴别器模块,能关注图像局部灌注信息。
- 使用多模态输入包括T2加权、表观扩散系数和T1预对比图像进行图像合成。
- 在ProstateX数据集上的研究结果显示,AAD-DCE模型在早反应和晚反应方面都表现出优异性能。
- 该模型对各种生成器指标具有不敏感性。
- 与其他DCE-MRI合成方法相比,AAD-DCE在PSNR、SSIM和MAE等评价指标上均有显著提高。
点此查看论文截图
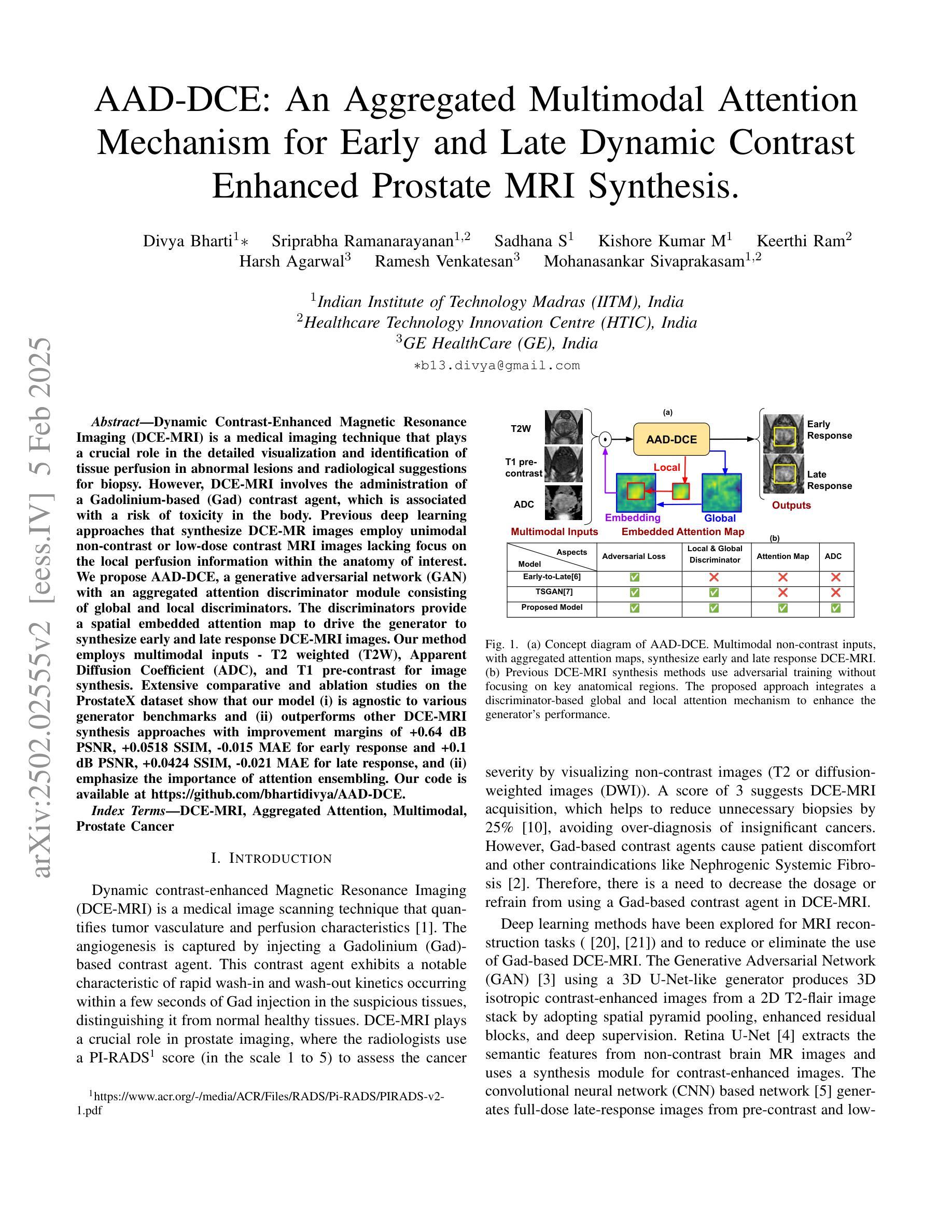
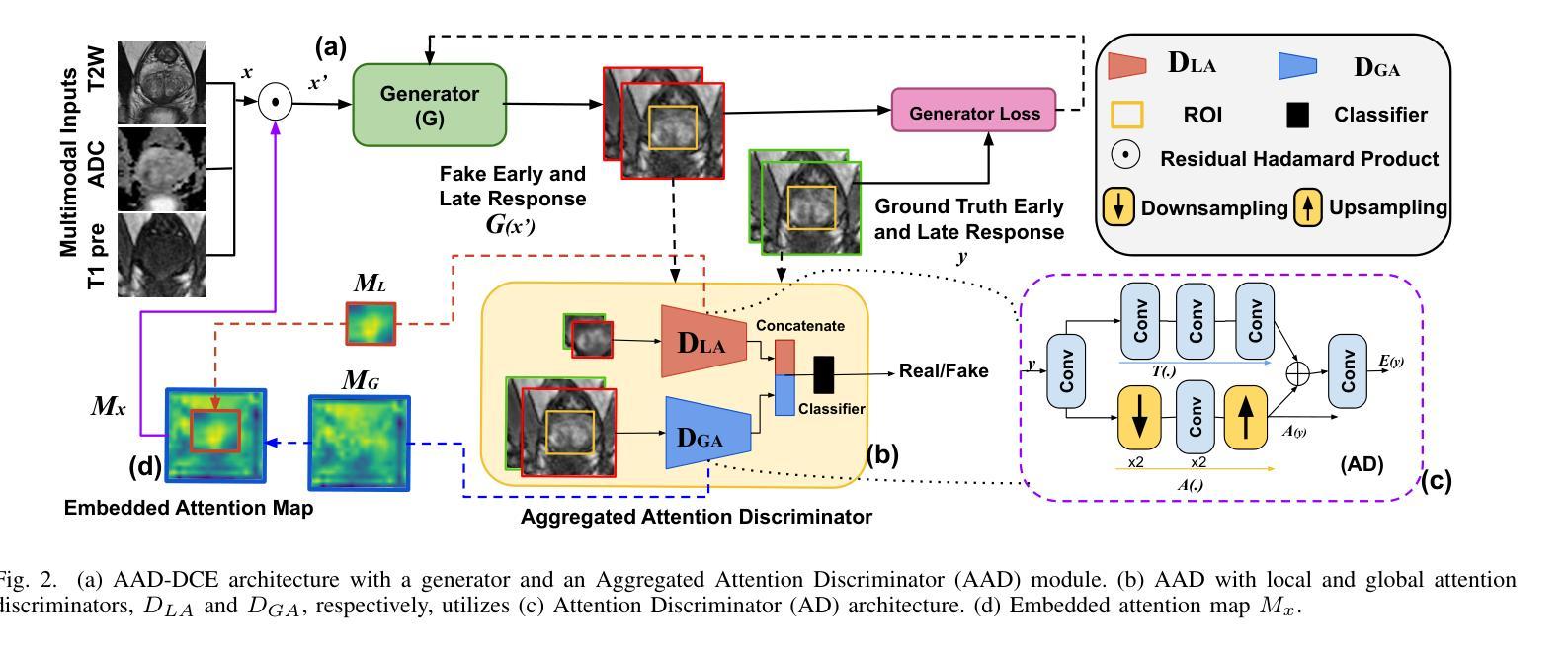
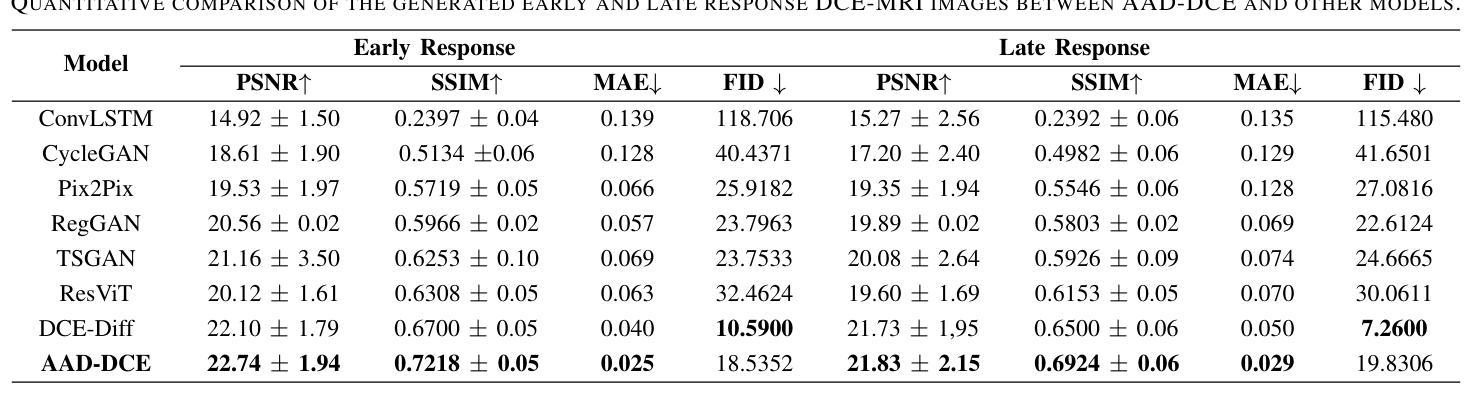
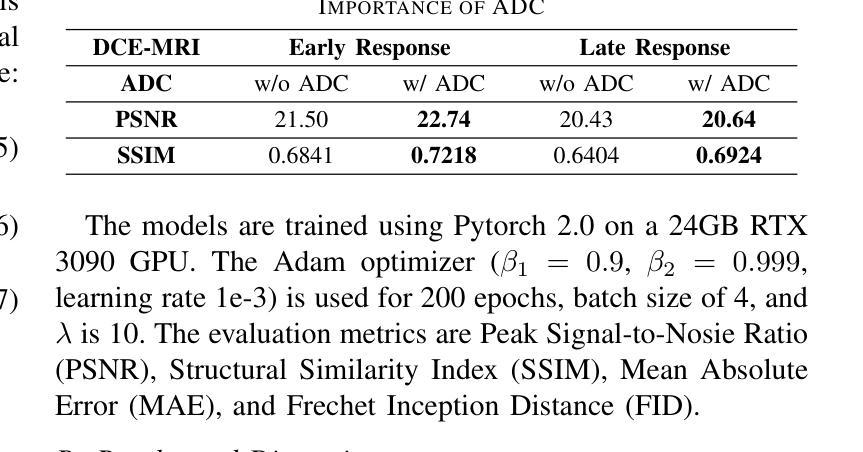
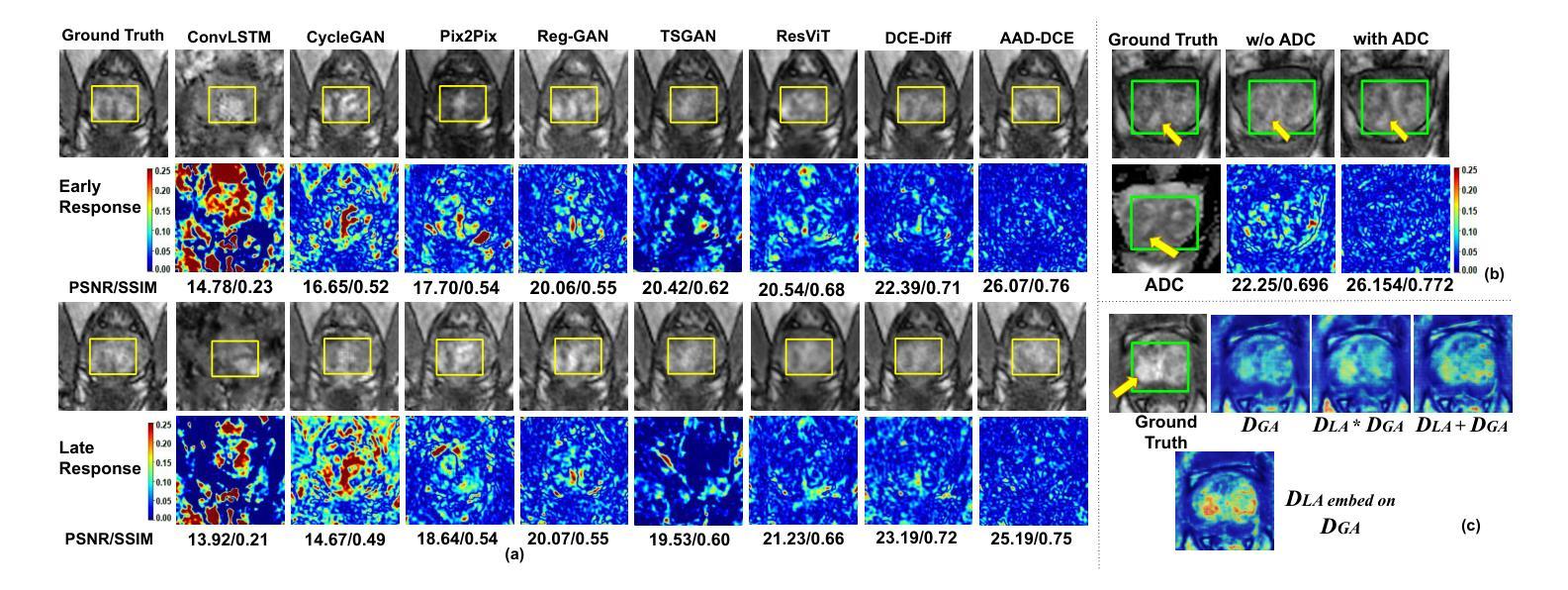
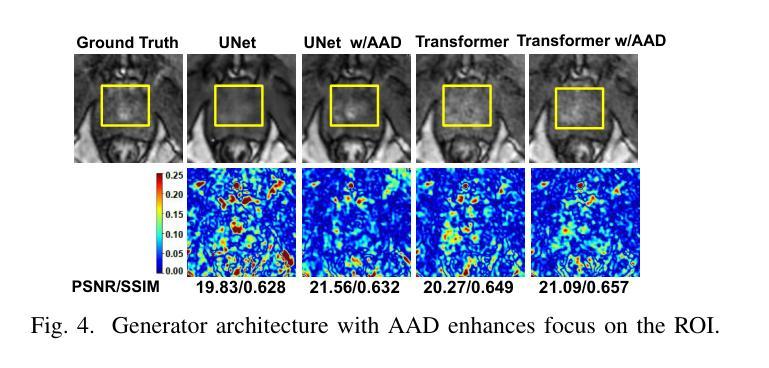
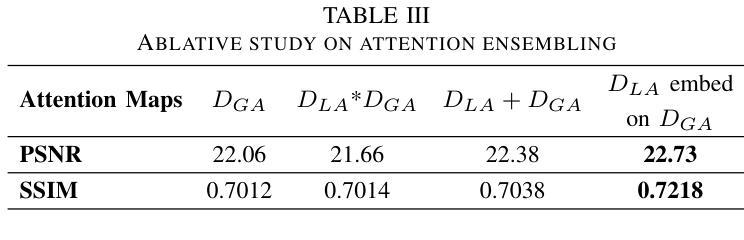