⚠️ 以下所有内容总结都来自于 大语言模型的能力,如有错误,仅供参考,谨慎使用
🔴 请注意:千万不要用于严肃的学术场景,只能用于论文阅读前的初筛!
💗 如果您觉得我们的项目对您有帮助 ChatPaperFree ,还请您给我们一些鼓励!⭐️ HuggingFace免费体验
2025-02-08 更新
SLCGC: A lightweight Self-supervised Low-pass Contrastive Graph Clustering Network for Hyperspectral Images
Authors:Yao Ding, Zhili Zhang, Aitao Yang, Yaoming Cai, Xiongwu Xiao, Danfeng Hong, Junsong Yuan
Self-supervised hyperspectral image (HSI) clustering remains a fundamental yet challenging task due to the absence of labeled data and the inherent complexity of spatial-spectral interactions. While recent advancements have explored innovative approaches, existing methods face critical limitations in clustering accuracy, feature discriminability, computational efficiency, and robustness to noise, hindering their practical deployment. In this paper, a self-supervised efficient low-pass contrastive graph clustering (SLCGC) is introduced for HSIs. Our approach begins with homogeneous region generation, which aggregates pixels into spectrally consistent regions to preserve local spatial-spectral coherence while drastically reducing graph complexity. We then construct a structural graph using an adjacency matrix A and introduce a low-pass graph denoising mechanism to suppress high-frequency noise in the graph topology, ensuring stable feature propagation. A dual-branch graph contrastive learning module is developed, where Gaussian noise perturbations generate augmented views through two multilayer perceptrons (MLPs), and a cross-view contrastive loss enforces structural consistency between views to learn noise-invariant representations. Finally, latent embeddings optimized by this process are clustered via K-means. Extensive experiments and repeated comparative analysis have verified that our SLCGC contains high clustering accuracy, low computational complexity, and strong robustness. The code source will be available at https://github.com/DY-HYX.
自监督型高光谱图像(HSI)聚类仍然是一项基本而具有挑战性的任务,因为缺乏标记数据和高光谱数据空间光谱交互的固有复杂性。尽管最近有进展探索了创新方法,但现有方法在聚类准确性、特征辨别力、计算效率和抗噪声鲁棒性方面存在关键局限性,阻碍了其实践部署。本文介绍了一种用于HSI的自监督高效低通对比图聚类(SLCGC)。我们的方法首先从同质区域生成开始,将像素聚集成光谱一致的区域,以保留局部空间光谱一致性,同时大大降低图的复杂性。然后,我们使用邻接矩阵A构建结构图,并引入低通图去噪机制来抑制图拓扑中的高频噪声,以确保特征稳定传播。开发了一个双分支图对比学习模块,其中高斯噪声扰动通过两个多层感知器(MLP)生成增强视图,跨视图对比损失强制执行视图之间的结构一致性,以学习噪声不变表示。最后,通过此过程优化的潜在嵌入通过K-means进行聚类。广泛的实验和重复对比分析证实,我们的SLCGC具有高聚类准确性、低计算复杂度和强鲁棒性。代码源将在https://github.com/DY-HYX上提供。
论文及项目相关链接
PDF 12 pages, 9 figures
Summary
本文提出一种自监督高效低通对比图聚类(SLCGC)方法用于处理高光谱图像聚类任务。方法通过生成同质区域、构建结构图并引入低通图去噪机制以及双分支图对比学习模块,实现了高聚类准确性、低计算复杂度和强鲁棒性。
Key Takeaways
- 自监督高效低通对比图聚类(SLCGC)被介绍用于处理高光谱图像(HSI)聚类任务。
- 方法通过生成同质区域来减少图的复杂性,同时保留局部的空间光谱一致性。
- 结构图的构建使用邻接矩阵A,并引入低通图去噪机制以抑制图拓扑中的高频噪声。
- 开发了双分支图对比学习模块,通过高斯噪声扰动生成两个视图,并通过跨视图对比损失学习噪声不变表示。
- 优化后的潜在嵌入通过K-means进行聚类。
- 实验和比较分析验证了SLCGC的高聚类准确性、低计算复杂度和强鲁棒性。
点此查看论文截图
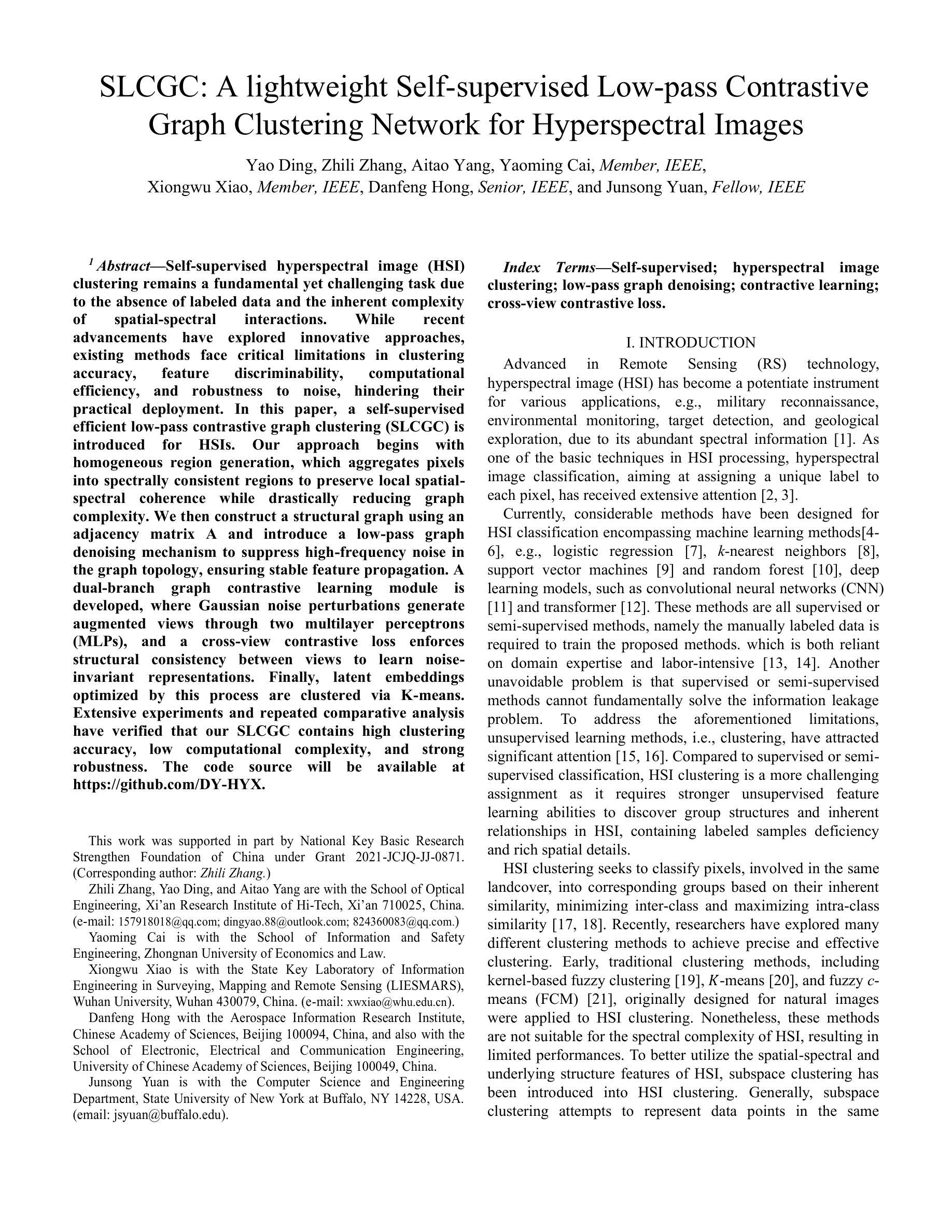
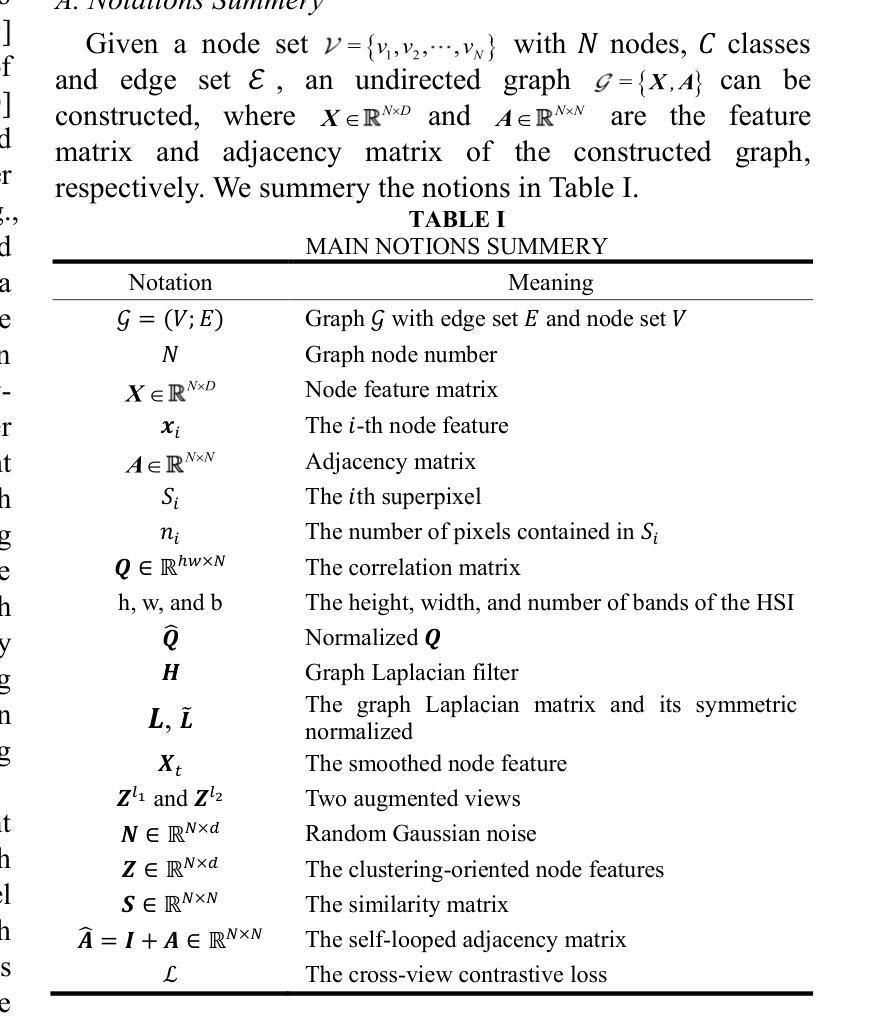
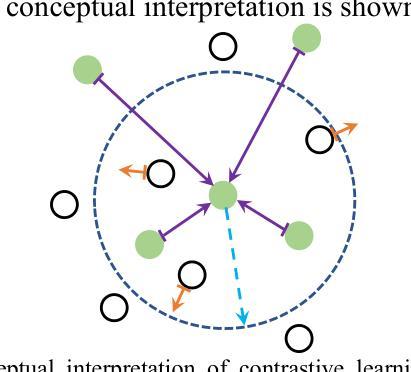
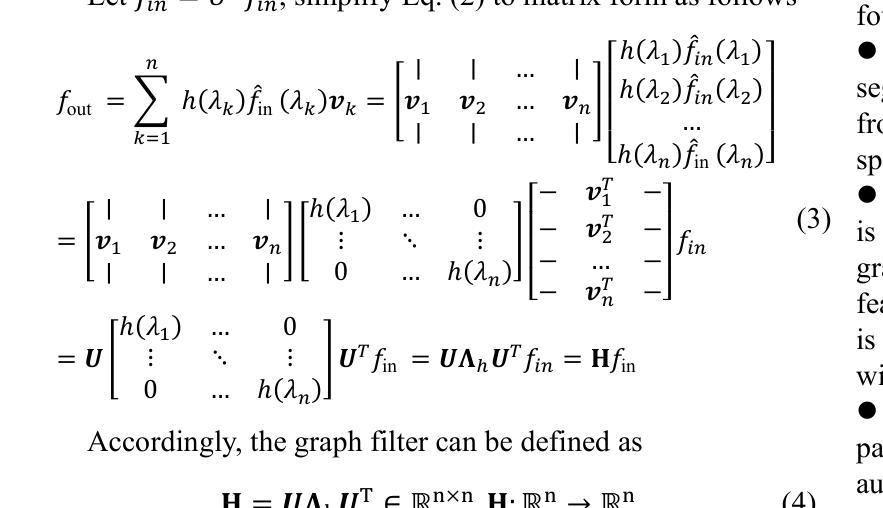
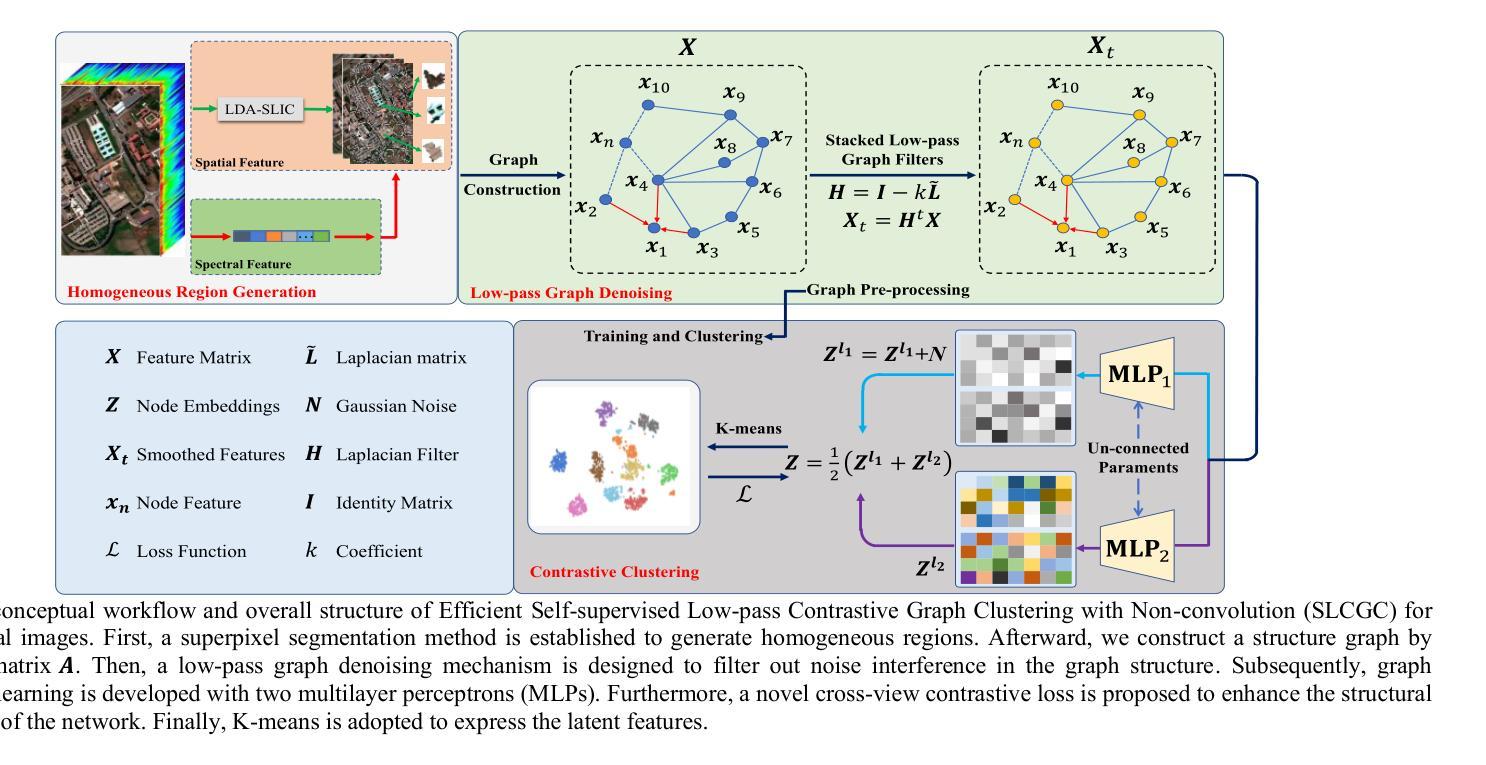
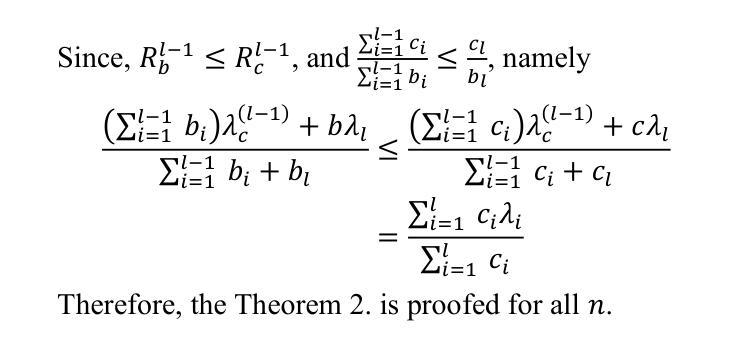
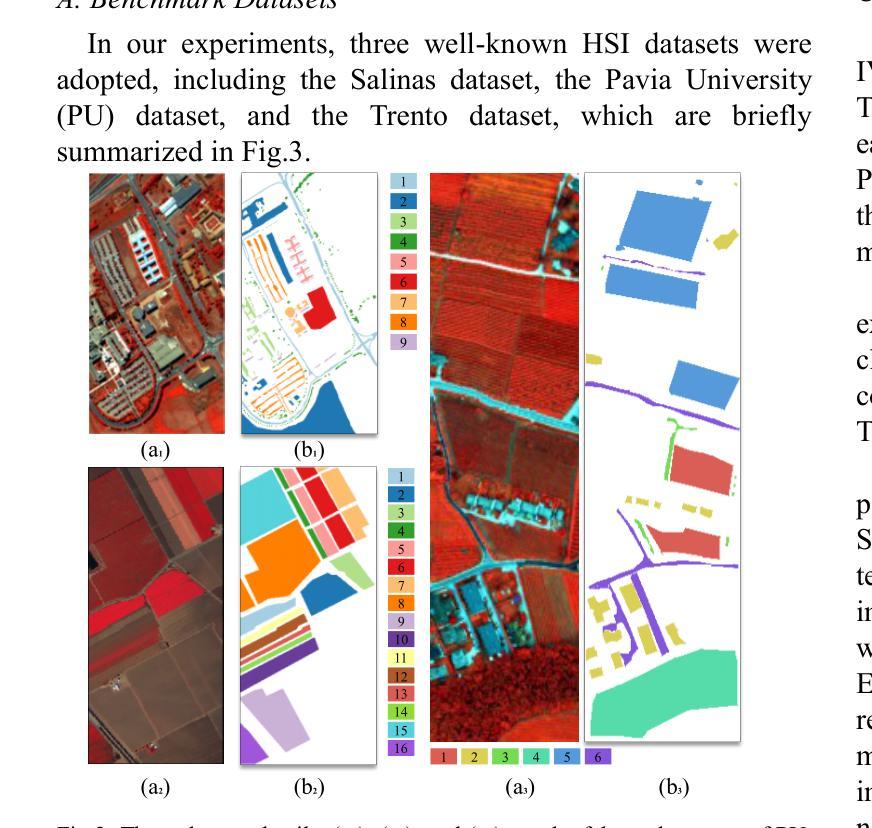
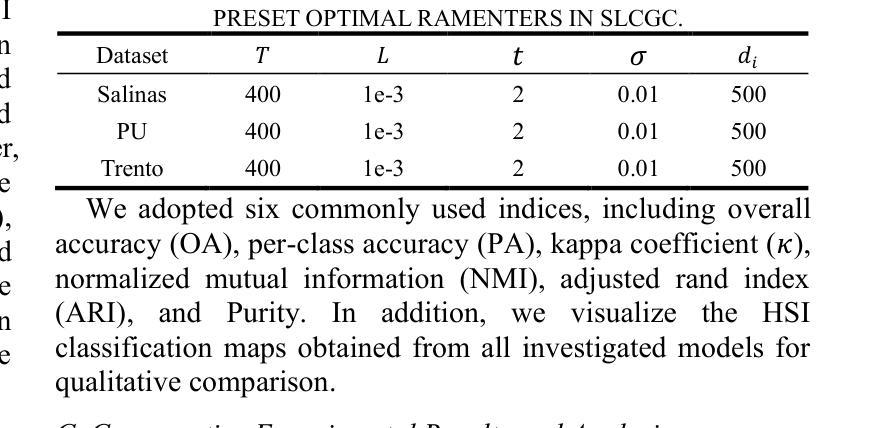