⚠️ 以下所有内容总结都来自于 大语言模型的能力,如有错误,仅供参考,谨慎使用
🔴 请注意:千万不要用于严肃的学术场景,只能用于论文阅读前的初筛!
💗 如果您觉得我们的项目对您有帮助 ChatPaperFree ,还请您给我们一些鼓励!⭐️ HuggingFace免费体验
2025-02-08 更新
ReactEmbed: A Cross-Domain Framework for Protein-Molecule Representation Learning via Biochemical Reaction Networks
Authors:Amitay Sicherman, Kira Radinsky
The challenge in computational biology and drug discovery lies in creating comprehensive representations of proteins and molecules that capture their intrinsic properties and interactions. Traditional methods often focus on unimodal data, such as protein sequences or molecular structures, limiting their ability to capture complex biochemical relationships. This work enhances these representations by integrating biochemical reactions encompassing interactions between molecules and proteins. By leveraging reaction data alongside pre-trained embeddings from state-of-the-art protein and molecule models, we develop ReactEmbed, a novel method that creates a unified embedding space through contrastive learning. We evaluate ReactEmbed across diverse tasks, including drug-target interaction, protein-protein interaction, protein property prediction, and molecular property prediction, consistently surpassing all current state-of-the-art models. Notably, we showcase ReactEmbed’s practical utility through successful implementation in lipid nanoparticle-based drug delivery, enabling zero-shot prediction of blood-brain barrier permeability for protein-nanoparticle complexes. The code and comprehensive database of reaction pairs are available for open use at \href{https://github.com/amitaysicherman/ReactEmbed}{GitHub}.
计算生物学和药物发现中的挑战在于创建能够捕捉蛋白质和分子内在属性及其相互作用的全面表示。传统方法通常关注单模态数据,如蛋白质序列或分子结构,这限制了它们捕捉复杂生化关系的能力。这项工作通过整合包含分子和蛋白质之间互动的生化反应来增强这些表示。通过利用反应数据和最前沿的蛋白质和分子模型的预训练嵌入,我们开发了一种通过对比学习创建统一嵌入空间的新方法,名为ReactEmbed。我们在多种任务上评估了ReactEmbed,包括药物-靶点相互作用、蛋白质-蛋白质相互作用、蛋白质属性预测和分子属性预测,它始终超越了所有当前的最先进模型。值得注意的是,我们通过成功实施基于脂质纳米粒子的药物输送展示了ReactEmbed的实际效用,能够实现蛋白质-纳米粒子复合物的血脑屏障通透性零样本预测。反应对的代码和综合数据库可在GitHub上免费使用:https://github.com/amitaysicherman/ReactEmbed。
论文及项目相关链接
Summary
本文介绍了计算生物学和药物发现领域中的一大挑战,即创建能够捕捉蛋白质和分子内在属性和相互作用的全面表示。传统方法常常局限于单模态数据,如蛋白质序列或分子结构,无法捕捉复杂的生物化学关系。本文通过整合包含分子和蛋白质之间互动的化学反应数据,并结合最先进蛋白质和分子模型的预训练嵌入技术,提出了ReactEmbed这一新方法,创建了一个统一嵌入空间。ReactEmbed在不同任务上的表现均超越了当前所有最先进模型,包括药物靶点交互、蛋白质-蛋白质交互、蛋白质属性预测和分子属性预测等。特别是ReactEmbed在实际应用中表现出了实用价值,如在脂质体纳米颗粒药物输送中实现零样本预测血脑屏障通透性对于蛋白质-纳米颗粒复合物的表现。相关代码和反应对数据库已公开发布在GitHub上。
Key Takeaways
- 计算生物学和药物发现的挑战在于创建全面、准确的蛋白质和分子表示。
- 传统方法主要关注单模态数据,难以捕捉复杂的生物化学关系。
- ReactEmbed通过整合化学反应数据,结合预训练嵌入技术,创建了一个统一的嵌入空间。
- ReactEmbed在不同任务上的表现均超越现有最先进模型。
- ReactEmbed可成功应用于脂质体纳米颗粒药物输送中的血脑屏障通透性预测。
- ReactEmbed方法和反应对数据库已公开发布在GitHub上供开放使用。
点此查看论文截图
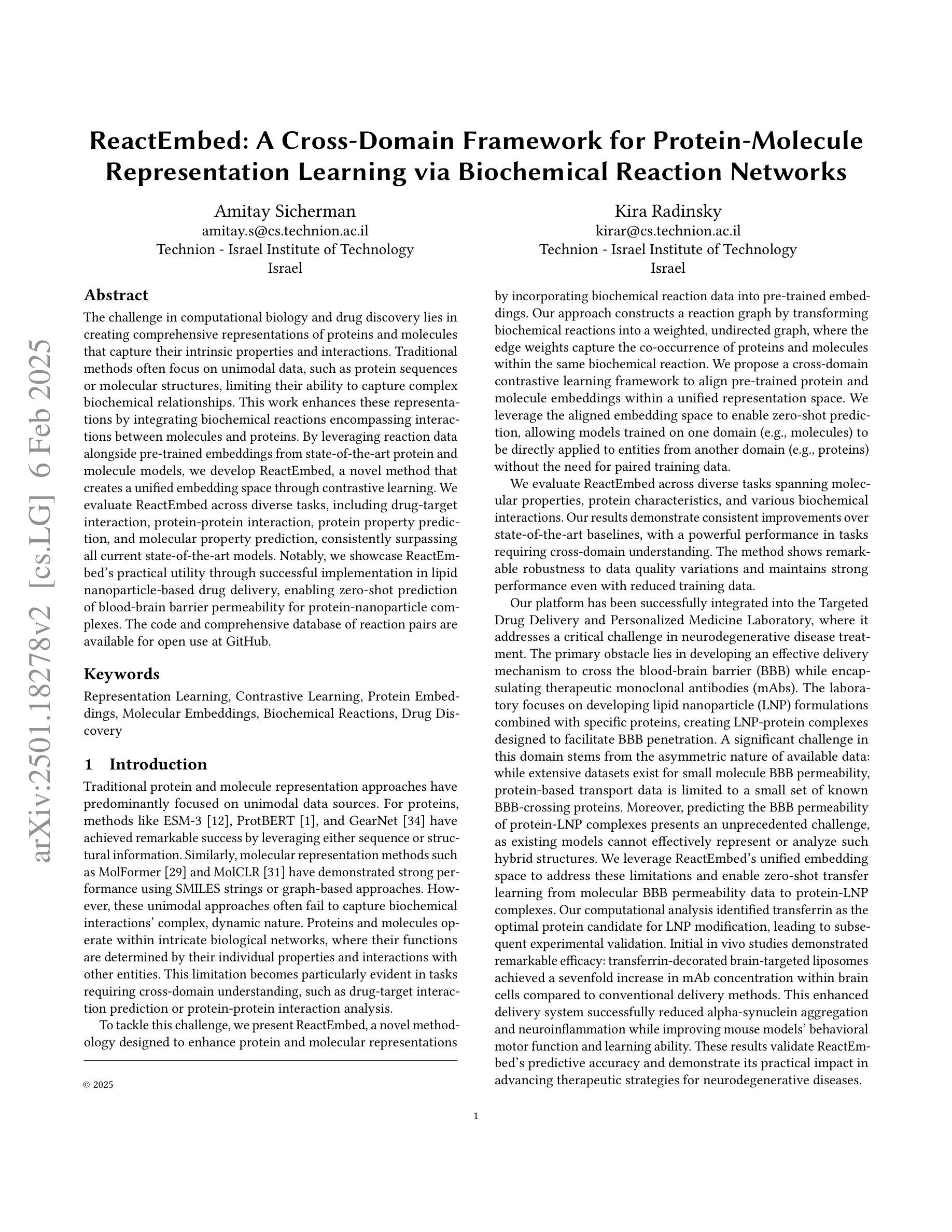
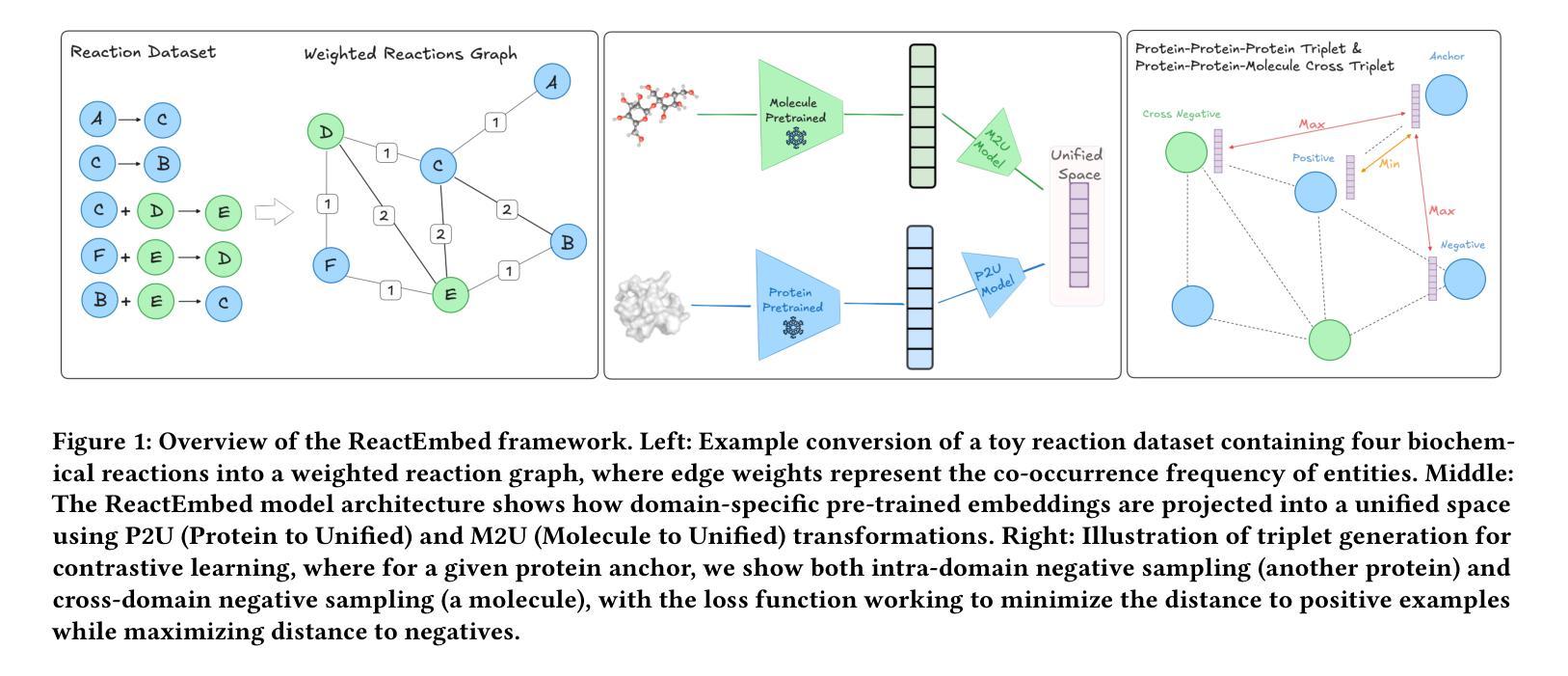
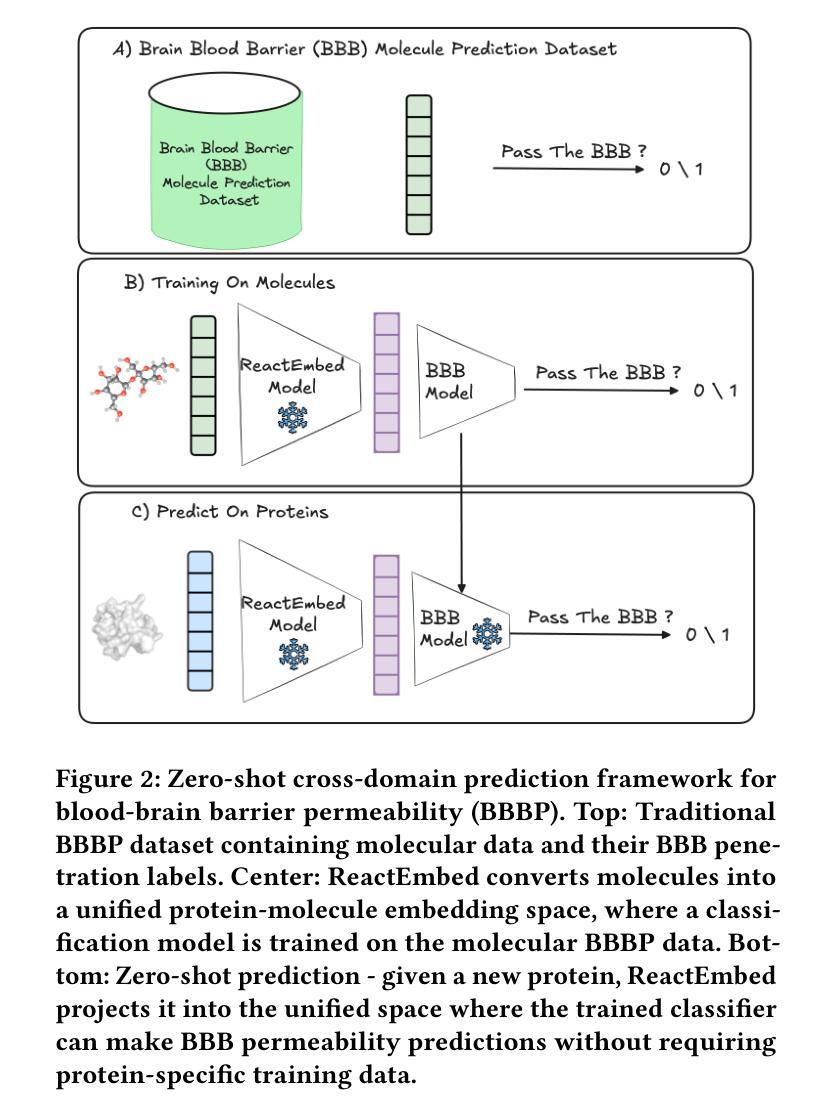
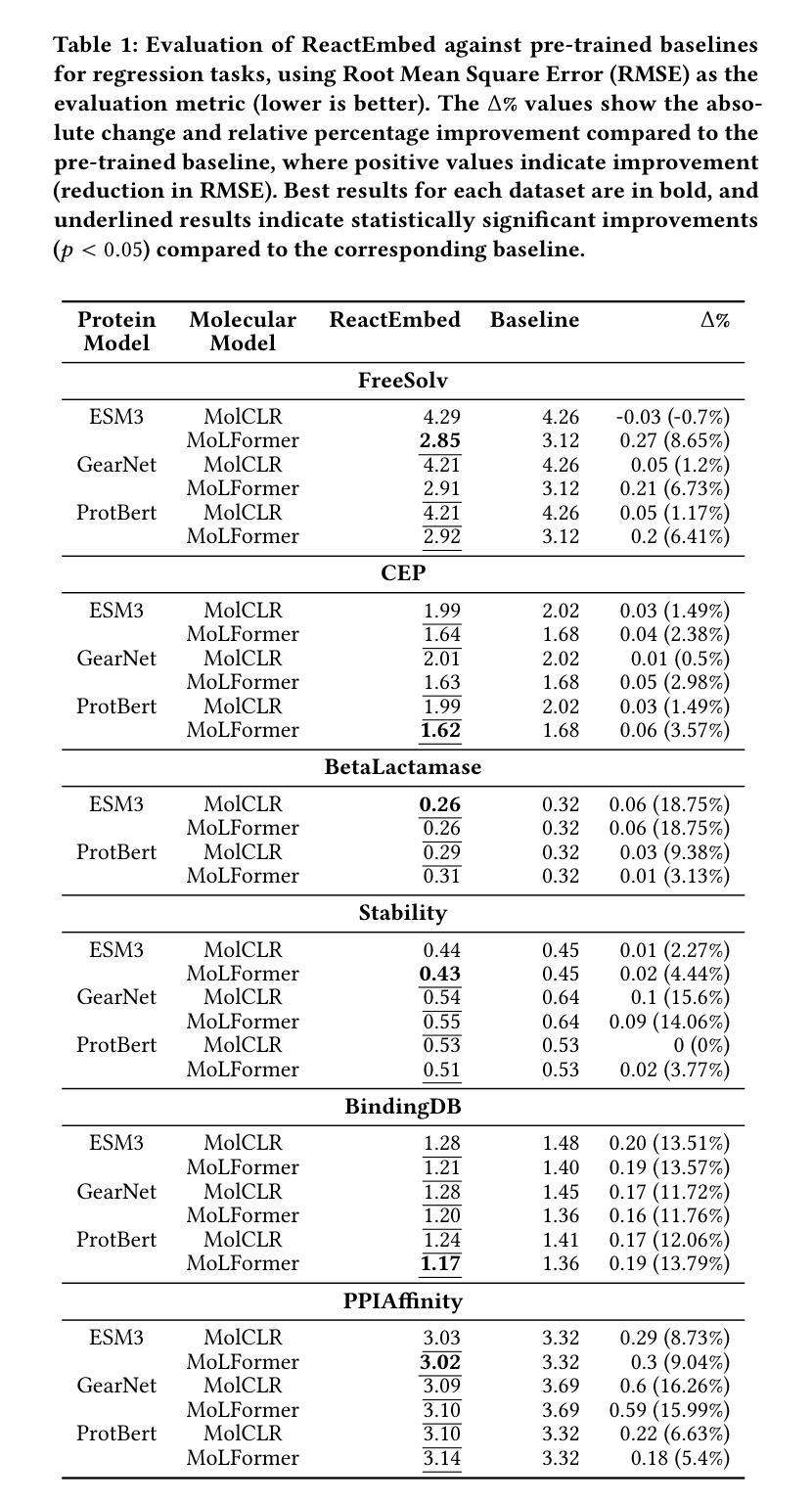
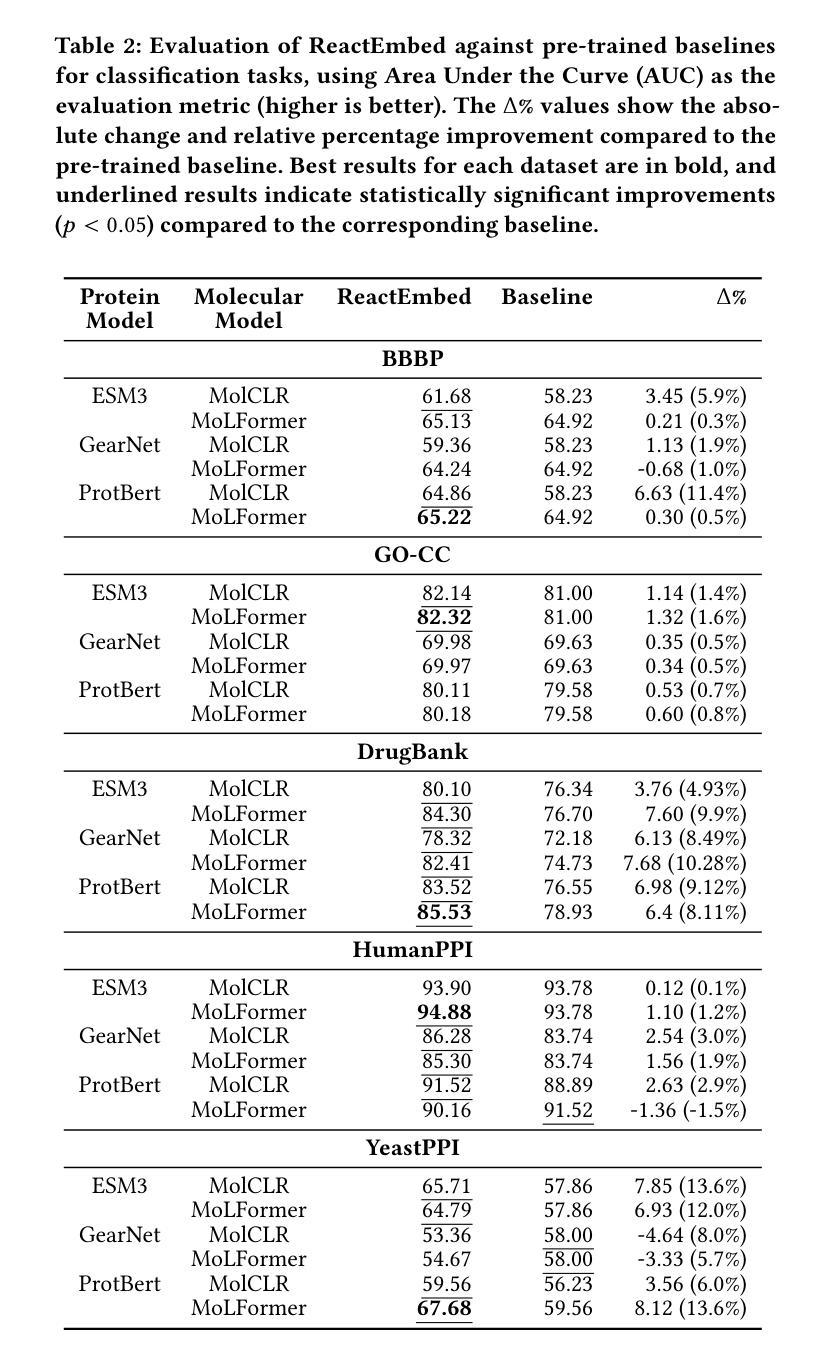