⚠️ 以下所有内容总结都来自于 大语言模型的能力,如有错误,仅供参考,谨慎使用
🔴 请注意:千万不要用于严肃的学术场景,只能用于论文阅读前的初筛!
💗 如果您觉得我们的项目对您有帮助 ChatPaperFree ,还请您给我们一些鼓励!⭐️ HuggingFace免费体验
2025-02-11 更新
Augmented Conditioning Is Enough For Effective Training Image Generation
Authors:Jiahui Chen, Amy Zhang, Adriana Romero-Soriano
Image generation abilities of text-to-image diffusion models have significantly advanced, yielding highly photo-realistic images from descriptive text and increasing the viability of leveraging synthetic images to train computer vision models. To serve as effective training data, generated images must be highly realistic while also sufficiently diverse within the support of the target data distribution. Yet, state-of-the-art conditional image generation models have been primarily optimized for creative applications, prioritizing image realism and prompt adherence over conditional diversity. In this paper, we investigate how to improve the diversity of generated images with the goal of increasing their effectiveness to train downstream image classification models, without fine-tuning the image generation model. We find that conditioning the generation process on an augmented real image and text prompt produces generations that serve as effective synthetic datasets for downstream training. Conditioning on real training images contextualizes the generation process to produce images that are in-domain with the real image distribution, while data augmentations introduce visual diversity that improves the performance of the downstream classifier. We validate augmentation-conditioning on a total of five established long-tail and few-shot image classification benchmarks and show that leveraging augmentations to condition the generation process results in consistent improvements over the state-of-the-art on the long-tailed benchmark and remarkable gains in extreme few-shot regimes of the remaining four benchmarks. These results constitute an important step towards effectively leveraging synthetic data for downstream training.
文本到图像的扩散模型的图像生成能力已经显著增强,能够从描述性的文本中产生高度逼真的图像,并且提高了利用合成图像来训练计算机视觉模型的可行性。为了作为有效的训练数据,生成的图像必须在目标数据分布的支持下既高度逼真又足够多样化。然而,最先进的条件图像生成模型主要经过了针对创意应用的优化,优先考虑图像的真实性和对提示的遵循,而不是条件多样性。在本文中,我们研究了如何提高生成图像的多样性,旨在提高其作为下游图像分类模型训练数据的效力,而无需对图像生成模型进行微调。我们发现,通过在生成过程中加入增强现实图像和文本提示作为条件,可以产生有效的合成数据集用于下游训练。以真实训练图像作为条件使生成过程具有上下文相关性,从而生成与真实图像分布同域的图像,而数据增强则引入了视觉多样性,提高了下游分类器的性能。我们在五个公认的长尾和少镜头图像分类基准测试上验证了增强现实条件下的效果,并表明利用增强现实条件来指导生成过程,在长尾基准测试上实现了对最新技术的持续改进,并在其余四个基准测试的极端少镜头情况下取得了显著的收益。这些结果构成有效利用合成数据进行下游训练的重要一步。
论文及项目相关链接
Summary
本文探讨了如何改进生成图像多样性以提高其作为下游图像分类模型训练数据的效率。研究发现,通过对生成过程进行基于真实图像和文本提示的增强条件化处理,能有效提高合成图像的质量。这种处理方式使得生成的图像更符合真实图像分布,同时数据增强技术引入的视觉多样性也提高了下游分类器的性能。在五个长尾和少量样本的图像分类基准测试中验证了这一方法的有效性,其在长尾基准测试上表现出一致的优势,并在其余四个基准的极端小样本情况下取得了显著的提升。这标志着有效使用合成数据进行下游训练的重要一步。
Key Takeaways
- 文本到图像的扩散模型已经取得了显著的进展,可以生成高度逼真的图像。
- 生成图像作为下游计算机视觉模型的训练数据需要同时具备高度真实性和足够的多样性。
- 当前优化主要侧重于创意应用,优先考虑图像的真实性和对提示的遵循,而忽视了条件多样性。
- 通过在生成过程中引入基于真实图像和文本提示的条件化,可以提高生成图像的有效性作为合成数据集。
- 条件化生成过程使得图像更符合真实图像分布,同时数据增强技术提高了下游分类器的性能。
- 在多个图像分类基准测试中验证了增强条件化的有效性,包括长尾和少量样本场景。
点此查看论文截图
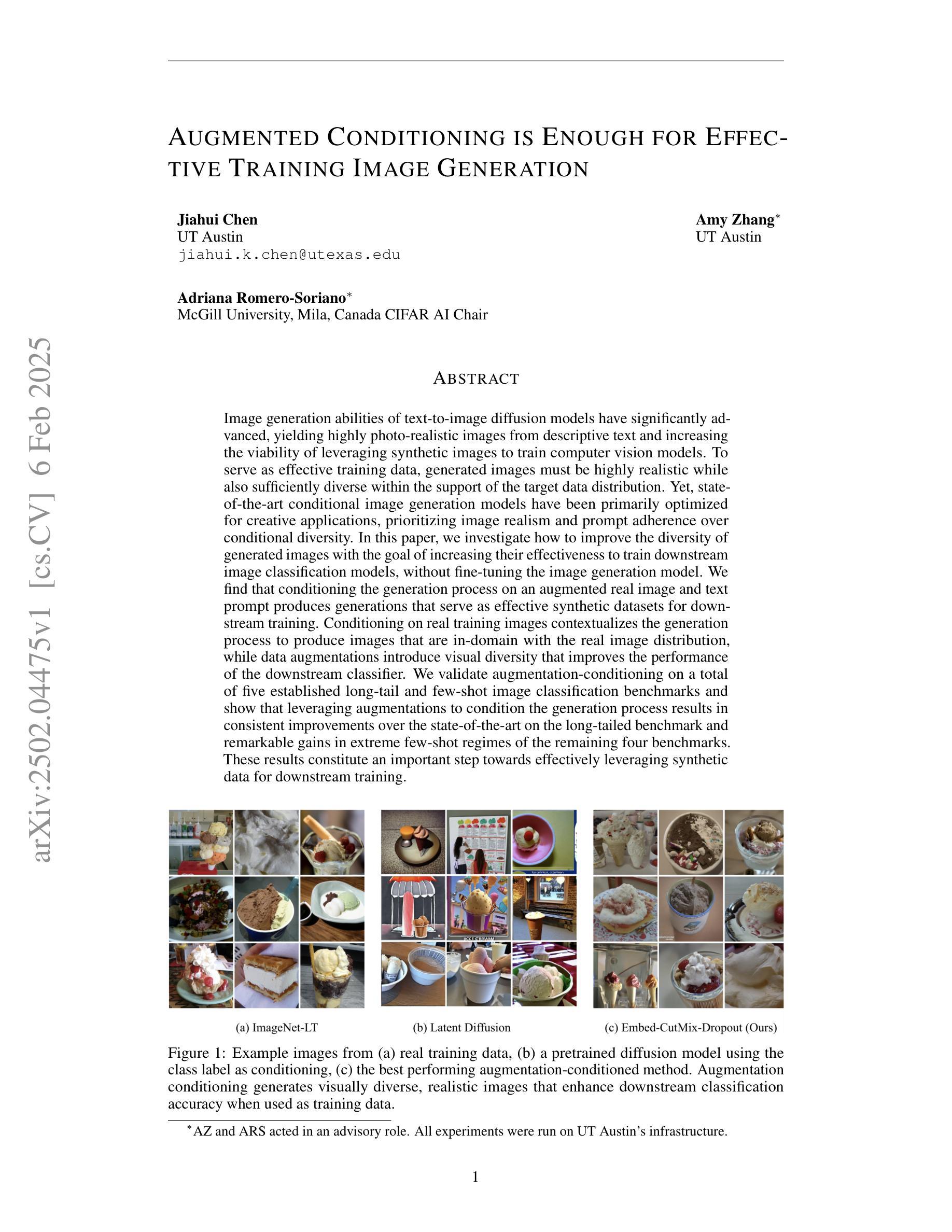
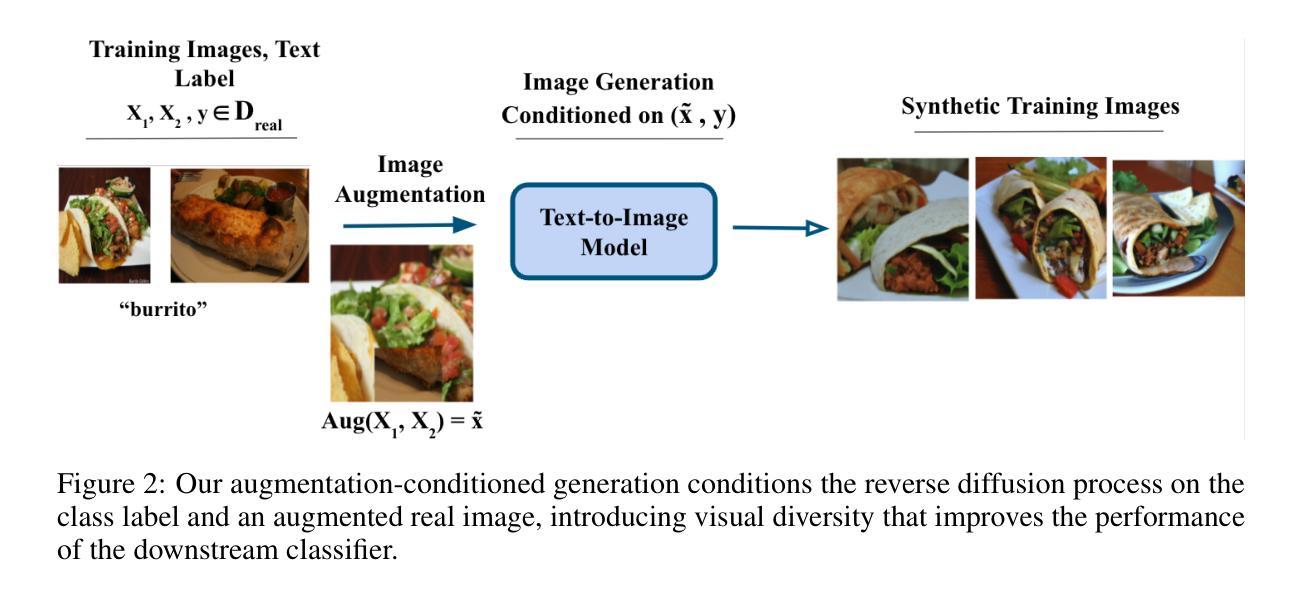
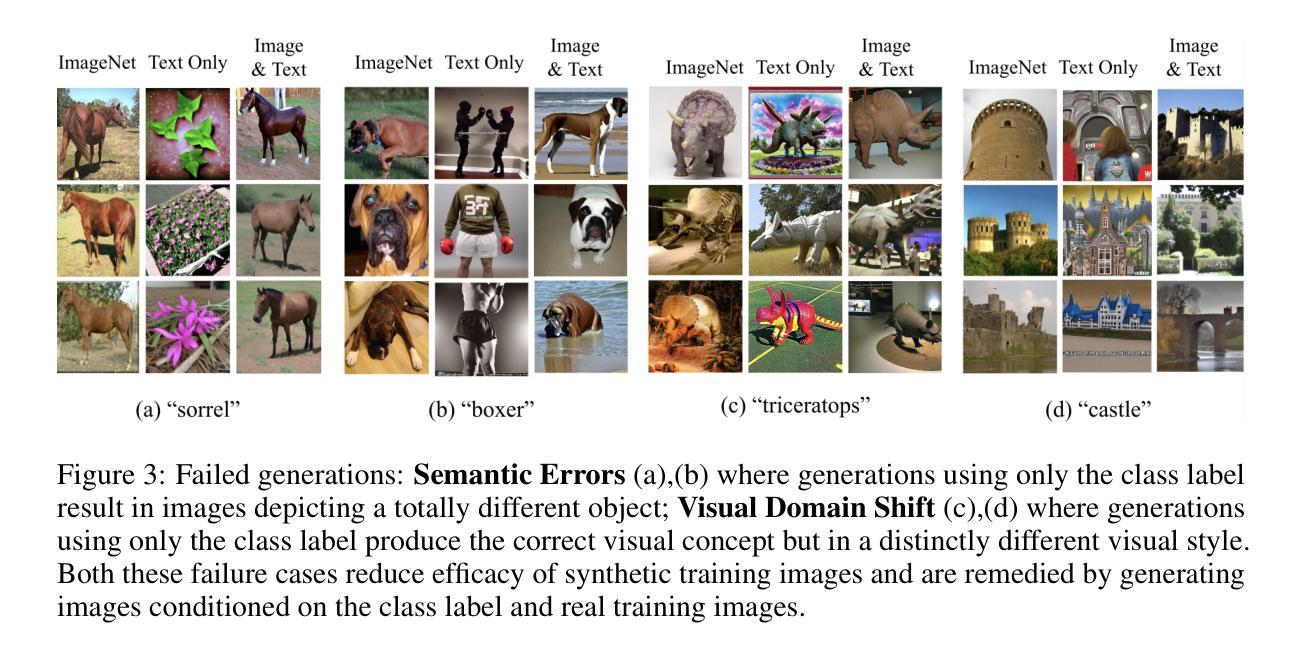
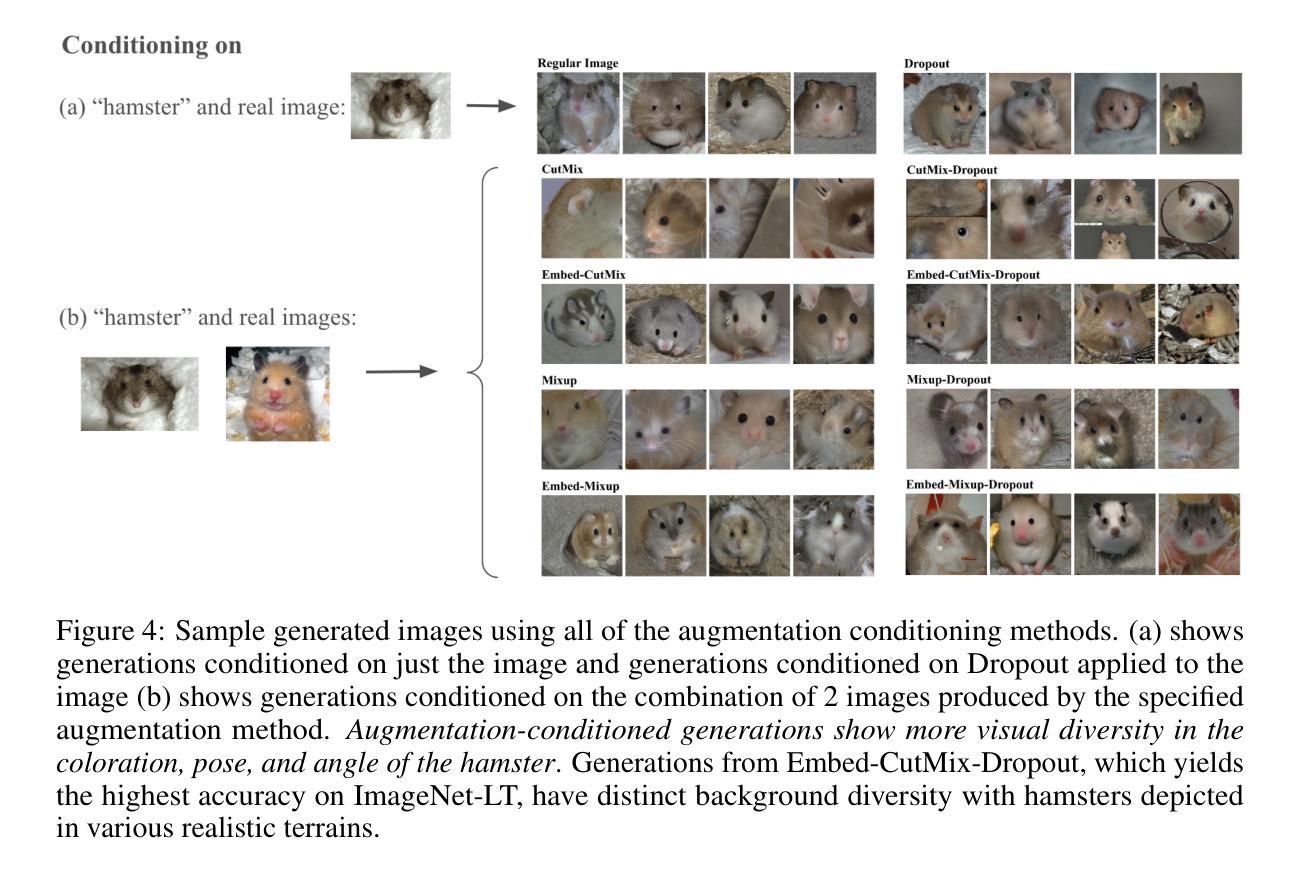
Transforming Multimodal Models into Action Models for Radiotherapy
Authors:Matteo Ferrante, Alessandra Carosi, Rolando Maria D Angelillo, Nicola Toschi
Radiotherapy is a crucial cancer treatment that demands precise planning to balance tumor eradication and preservation of healthy tissue. Traditional treatment planning (TP) is iterative, time-consuming, and reliant on human expertise, which can potentially introduce variability and inefficiency. We propose a novel framework to transform a large multimodal foundation model (MLM) into an action model for TP using a few-shot reinforcement learning (RL) approach. Our method leverages the MLM’s extensive pre-existing knowledge of physics, radiation, and anatomy, enhancing it through a few-shot learning process. This allows the model to iteratively improve treatment plans using a Monte Carlo simulator. Our results demonstrate that this method outperforms conventional RL-based approaches in both quality and efficiency, achieving higher reward scores and more optimal dose distributions in simulations on prostate cancer data. This proof-of-concept suggests a promising direction for integrating advanced AI models into clinical workflows, potentially enhancing the speed, quality, and standardization of radiotherapy treatment planning.
放射治疗是癌症治疗的关键手段,需要精确的计划来平衡肿瘤消除和保留健康组织。传统的治疗计划(TP)是迭代的、耗时的,并依赖于人类专家,这可能会引入变数和低效率。我们提出了一种新型框架,使用少量强化学习(RL)方法将多模态基础模型(MLM)转化为治疗行动模型。我们的方法利用MLM对物理、辐射和解剖学的现有丰富知识,并通过少量学习过程加以增强。这允许模型使用蒙特卡洛模拟器迭代改进治疗计划。我们的结果表明,该方法在质量和效率方面均优于传统基于RL的方法,在前列腺癌数据模拟中实现了更高的奖励分数和更优化的剂量分布。这一概念验证表明,将先进的AI模型整合到临床工作流程中的方向充满希望,可能会提高放射治疗计划的速度、质量和标准化水平。
论文及项目相关链接
Summary
本文提出了一种新型框架,将大型多模态基础模型转化为用于放射治疗计划的动作模型。该模型采用小样本强化学习技术,利用基础模型已有的物理学、放射学和解剖学知识,提高治疗效果的预测精度。研究结果表明,该方法的优化效果显著优于传统基于强化学习的方法,展现了整合先进人工智能模型至临床治疗流程的可行性及提高治疗规划的速度和标准化水平的潜力。
Key Takeaways
以下是该文本的关键见解:
- 放射治疗是一种需要精确规划的治疗方式,旨在平衡肿瘤消除与健康组织的保护。传统治疗规划过程繁琐且依赖专家经验,存在潜在的不确定性。
- 提出了一种新型框架,将大型多模态基础模型转化为用于放射治疗计划的动作模型,利用小样本强化学习技术。
- 该方法利用了基础模型已有的物理学、放射学和解剖学知识,并通过小样本学习过程进行增强。
- 该方法能够在模拟过程中优化治疗计划,并展现出较高的奖励分数和更优的剂量分布。在前列腺癌数据上的模拟结果显示了此方法相对于传统方法的优越性。
点此查看论文截图
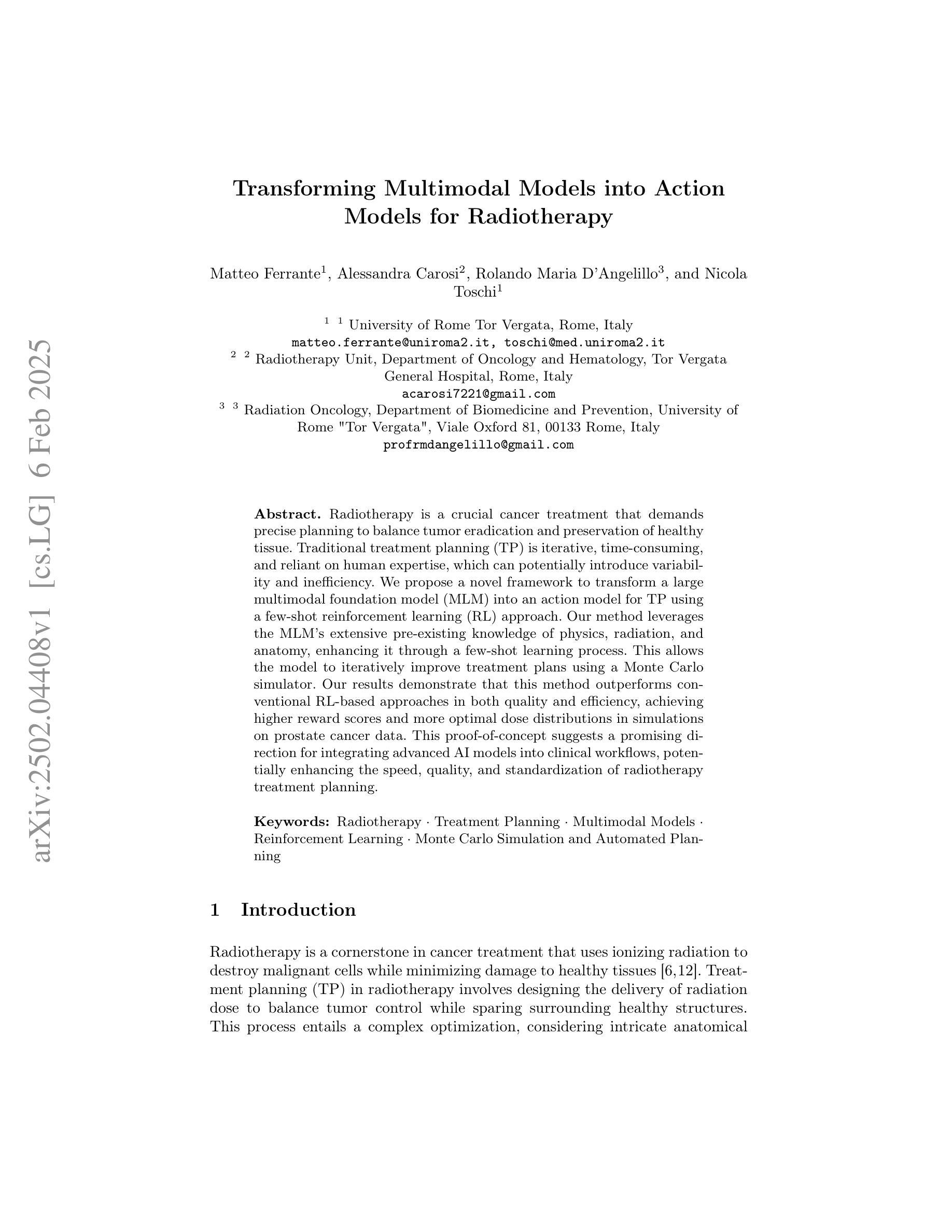
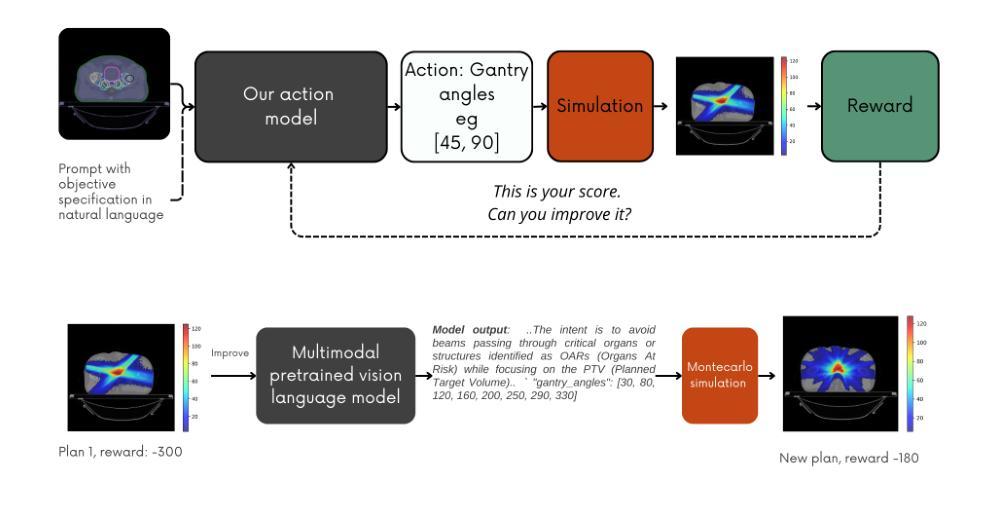
NER4all or Context is All You Need: Using LLMs for low-effort, high-performance NER on historical texts. A humanities informed approach
Authors:Torsten Hiltmann, Martin Dröge, Nicole Dresselhaus, Till Grallert, Melanie Althage, Paul Bayer, Sophie Eckenstaler, Koray Mendi, Jascha Marijn Schmitz, Philipp Schneider, Wiebke Sczeponik, Anica Skibba
Named entity recognition (NER) is a core task for historical research in automatically establishing all references to people, places, events and the like. Yet, do to the high linguistic and genre diversity of sources, only limited canonisation of spellings, the level of required historical domain knowledge, and the scarcity of annotated training data, established approaches to natural language processing (NLP) have been both extremely expensive and yielded only unsatisfactory results in terms of recall and precision. Our paper introduces a new approach. We demonstrate how readily-available, state-of-the-art LLMs significantly outperform two leading NLP frameworks, spaCy and flair, for NER in historical documents by seven to twentytwo percent higher F1-Scores. Our ablation study shows how providing historical context to the task and a bit of persona modelling that turns focus away from a purely linguistic approach are core to a successful prompting strategy. We also demonstrate that, contrary to our expectations, providing increasing numbers of examples in few-shot approaches does not improve recall or precision below a threshold of 16-shot. In consequence, our approach democratises access to NER for all historians by removing the barrier of scripting languages and computational skills required for established NLP tools and instead leveraging natural language prompts and consumer-grade tools and frontends.
命名实体识别(NER)是历史研究中的一项核心任务,可自动建立对所有涉及人员、地点、事件等的引用。然而,由于源头的语言和体裁多样性、拼写规范有限、所需的历史领域知识程度以及注释训练数据的稀缺性,自然语言处理(NLP)的既定方法既极为昂贵,在召回率和精确率方面也仅获得了令人不满意的结果。我们的论文介绍了一种新方法。我们展示了如何现成使用最先进的大型语言模型,以F1分数高出7至22个百分点的方式,显著优于用于历史文献NER的两个领先NLP框架spaCy和flair。我们的消融研究表明,为任务提供历史背景和一定程度的个性化建模,远离纯粹的语言方法,是成功的提示策略的核心。我们还证明,与我们预期相反的是,在少于几次尝试的方法中提供越来越多的例子,在达到16次提示的阈值后,并不会提高召回率或精确率。因此,我们的方法通过消除使用既定NLP工具所需的脚本语言和计算技能障碍,转而利用自然语言提示、消费级工具和前端,使所有历史学家都能民主地使用NER。
论文及项目相关链接
Summary
在历史文献中的命名实体识别(NER)任务面临诸多挑战,如高语言多样性、拼写规范化程度有限、所需历史领域知识程度以及标注训练数据的稀缺性等。本文引入了一种新方法,展示如何借助先进的大型语言模型(LLMs)显著提升现有自然语言处理(NLP)框架的性能,例如spaCy和flair等。本研究显示LLMs在F1得分上高出7至22个百分点,并通过消融研究证明了为任务提供历史背景和个性化建模是有效策略的关键。此外,本研究还表明,在少数镜头方法中,提供不断增加的例子数量并不一定能提高召回率和精确度,存在一个不超过16次的阈值。因此,我们的方法通过利用自然语言提示和消费者级工具及前端,为历史学家提供了民主化的NER访问方式,消除了编写语言和计算技能方面的障碍。
Key Takeaways
- 命名实体识别(NER)在历史研究中有重要应用,但面临诸多挑战。
- 现有自然语言处理(NLP)方法在召回和精确度方面表现不佳。
- 先进的大型语言模型(LLMs)显著提高了在历史文献NER任务上的性能。
- 为任务提供历史背景和个性化建模是提升性能的关键策略。
- 在少数镜头方法中,提供不断增加的例子数量并不一定能提高召回率和精确度,存在一个阈值。
- 本研究通过利用自然语言提示和消费者级工具及前端,简化了NER任务的访问门槛。
点此查看论文截图
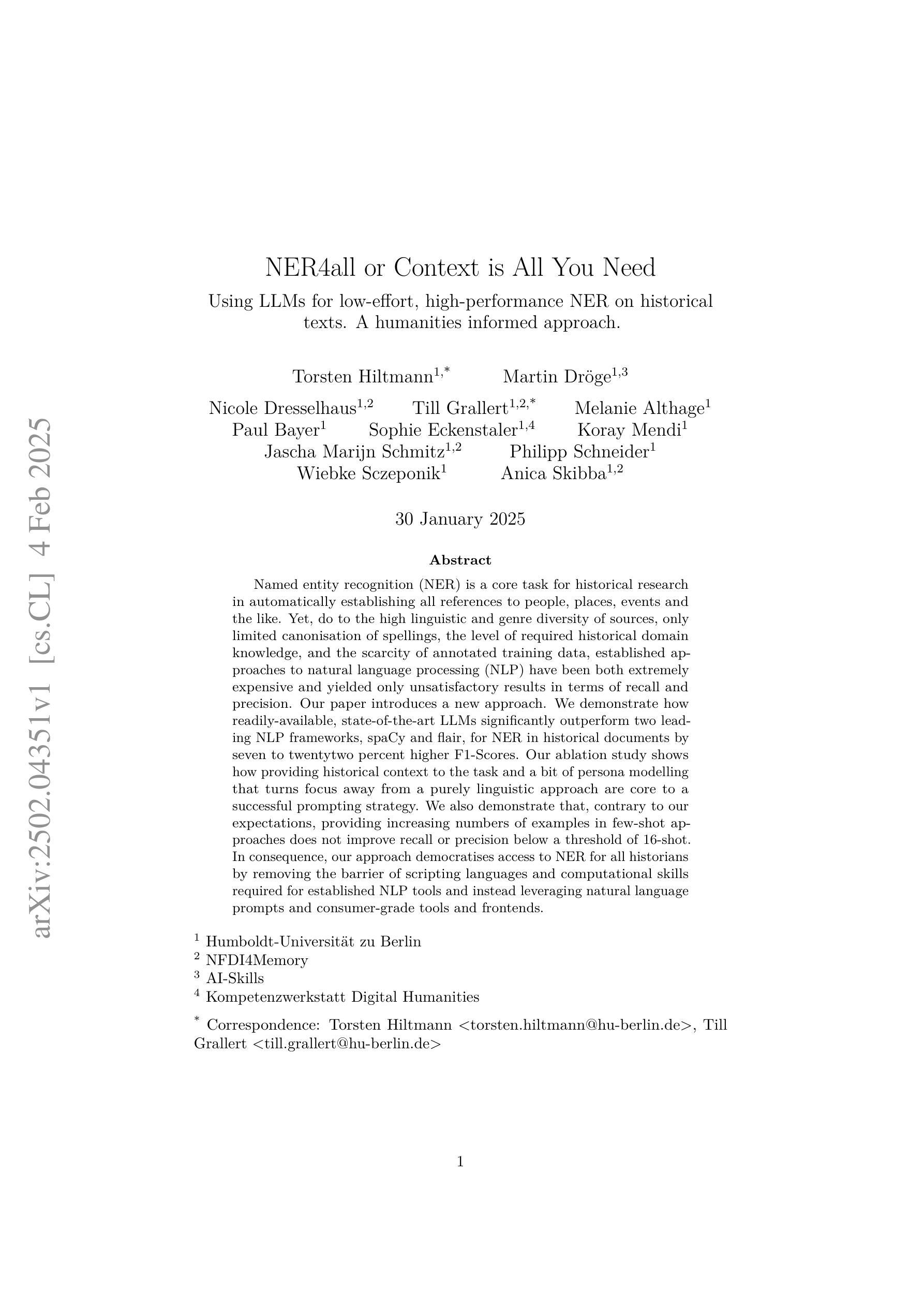
LLM4WM: Adapting LLM for Wireless Multi-Tasking
Authors:Xuanyu Liu, Shijian Gao, Boxun Liu, Xiang Cheng, Liuqing Yang
The wireless channel is fundamental to communication, encompassing numerous tasks collectively referred to as channel-associated tasks. These tasks can leverage joint learning based on channel characteristics to share representations and enhance system design. To capitalize on this advantage, LLM4WM is proposed–a large language model (LLM) multi-task fine-tuning framework specifically tailored for channel-associated tasks. This framework utilizes a Mixture of Experts with Low-Rank Adaptation (MoE-LoRA) approach for multi-task fine-tuning, enabling the transfer of the pre-trained LLM’s general knowledge to these tasks. Given the unique characteristics of wireless channel data, preprocessing modules, adapter modules, and multi-task output layers are designed to align the channel data with the LLM’s semantic feature space. Experiments on a channel-associated multi-task dataset demonstrate that LLM4WM outperforms existing methodologies in both full-sample and few-shot evaluations, owing to its robust multi-task joint modeling and transfer learning capabilities.
无线信道是通信的基础,包含许多统称为信道相关任务(channel-associated tasks)的工作。这些任务可以利用基于信道特性的联合学习来共享表示并增强系统设计。为了充分利用这一优势,提出了LLM4WM——一种针对信道相关任务的大型语言模型(LLM)多任务微调框架。该框架采用低秩适应(LoRA)的混合专家(MoE)方法进行多任务微调,能够实现预训练LLM通用知识向这些任务的迁移。考虑到无线信道数据的独特特点,设计了预处理模块、适配器模块和多任务输出层,以使信道数据与LLM的语义特征空间对齐。在信道相关多任务数据集上的实验表明,LLM4WM在全样本和少量样本评估中都优于现有方法,这得益于其强大的多任务联合建模和迁移学习能力。
论文及项目相关链接
Summary
无线信道通信中的核心组成部分是多种集体被称为信道相关任务的类别。利用信道特性实现联合学习可共享表达并提升系统设计效率。针对此特点提出LLM4WM框架,它是一款大型语言模型(LLM)多任务微调框架,专门用于处理信道相关任务。该框架采用基于低秩适应的专家混合(MoE-LoRA)方法进行多任务微调,可将预训练的大型语言模型的通用知识转移到这些任务上。针对无线信道数据的独特特点,设计了预处理模块、适配器模块和多任务输出层,以使信道数据与大型语言模型的语义特征空间对齐。实验表明,LLM4WM在全样本和少量样本评估中都优于现有方法,得益于其强大的多任务联合建模和迁移学习能力。
Key Takeaways
- 无线信道通信中包含多种被称为信道相关任务的类别。
- 联合学习利用信道特性可以提高系统设计的效率。
- LLM4WM框架专门用于处理信道相关任务,基于大型语言模型进行多任务微调。
- LLM4WM使用MoE-LoRA方法进行多任务微调,能够转移预训练模型的通用知识。
- 针对无线信道数据的特性,设计了预处理模块、适配器模块和多任务输出层。
- LLM4WM在全样本和少量样本评估中表现优异。
点此查看论文截图
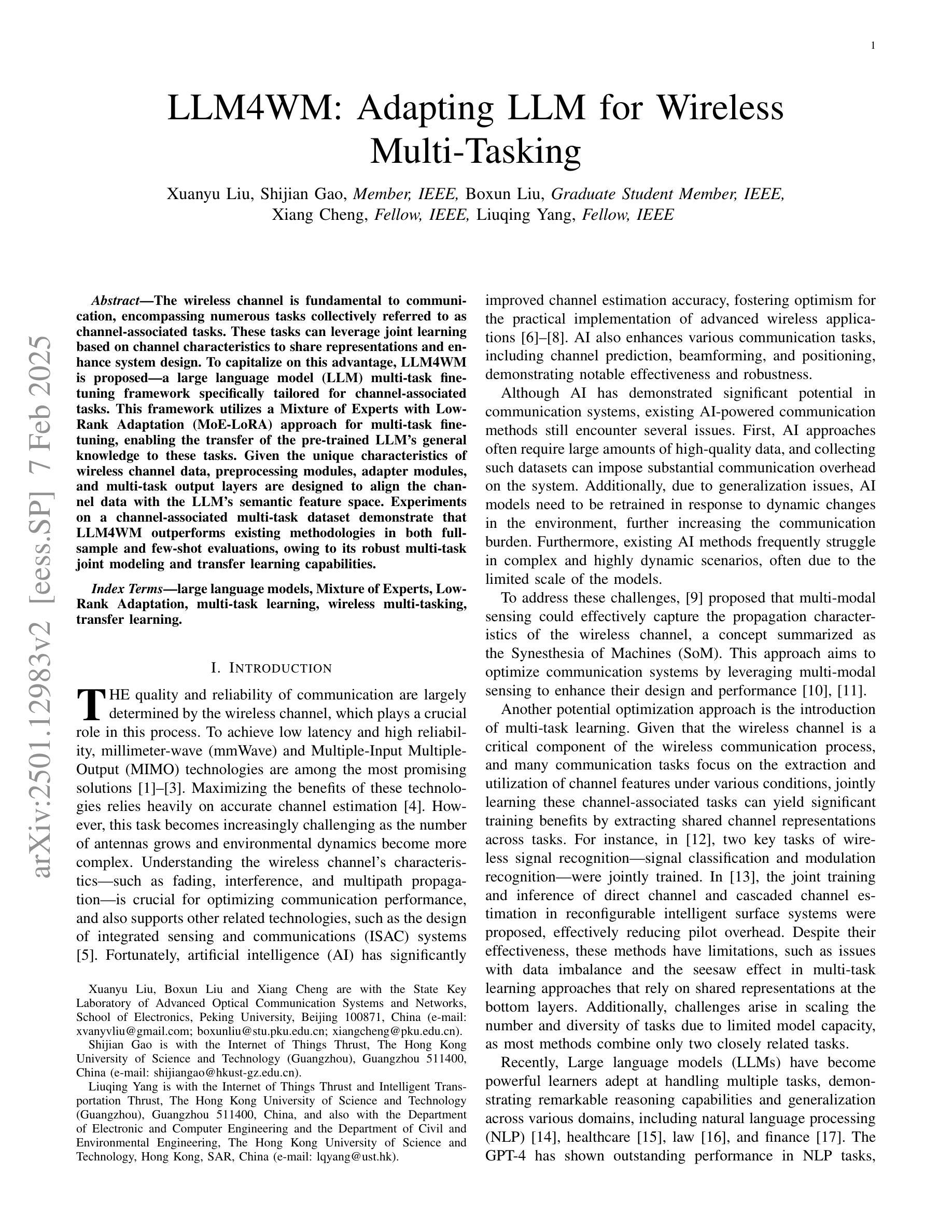
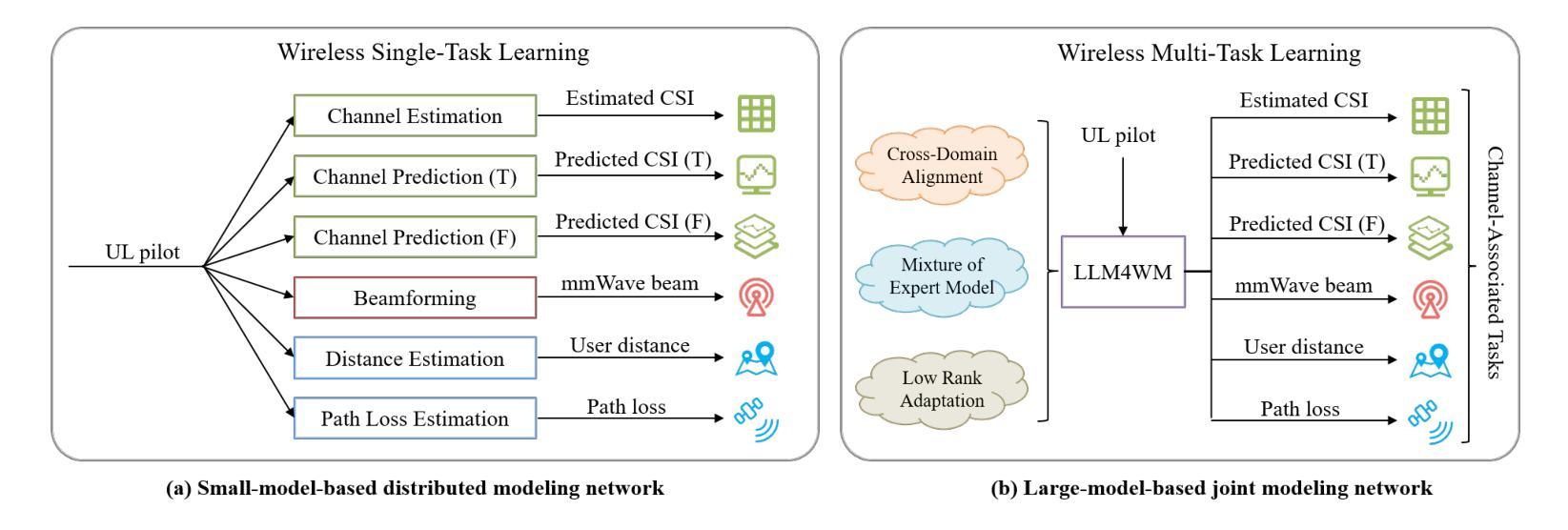
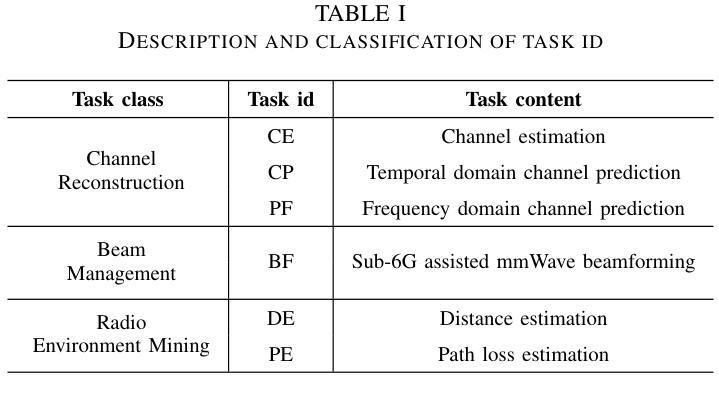
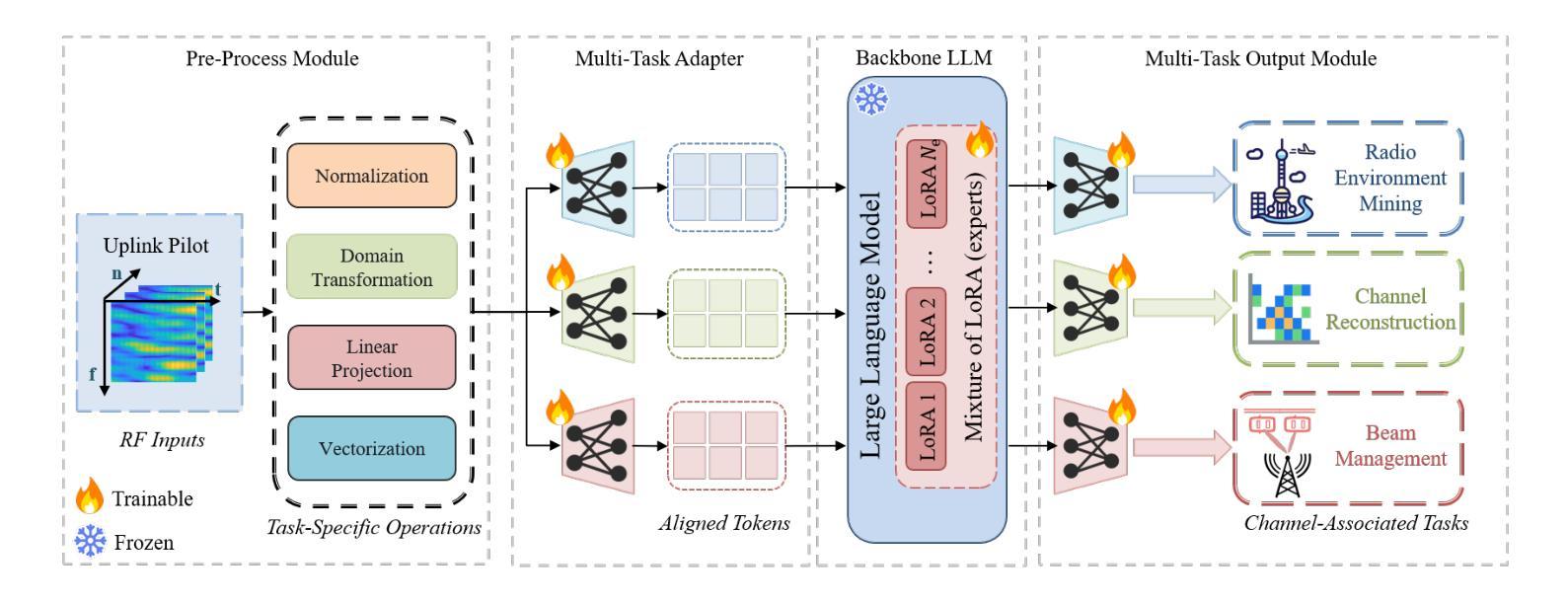
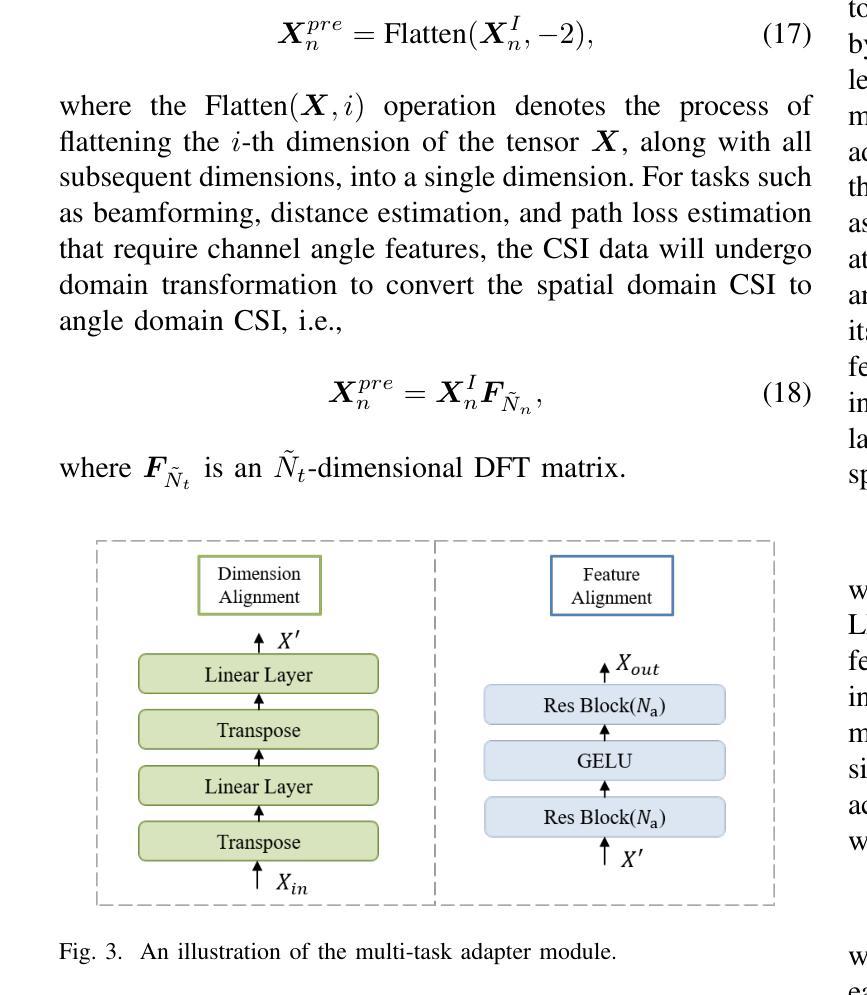
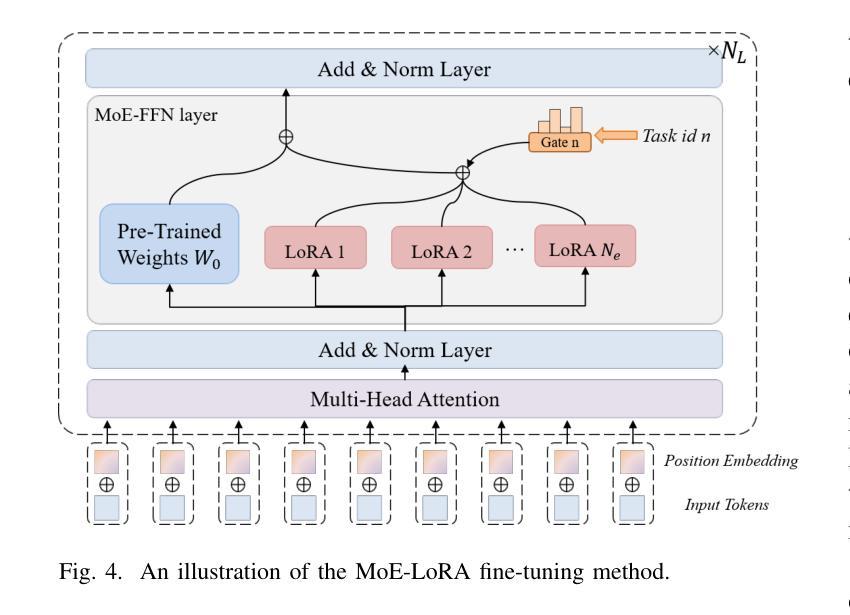
A Strong Baseline for Molecular Few-Shot Learning
Authors:Philippe Formont, Hugo Jeannin, Pablo Piantanida, Ismail Ben Ayed
Few-shot learning has recently attracted significant interest in drug discovery, with a recent, fast-growing literature mostly involving convoluted meta-learning strategies. We revisit the more straightforward fine-tuning approach for molecular data, and propose a regularized quadratic-probe loss based on the the Mahalanobis distance. We design a dedicated block-coordinate descent optimizer, which avoid the degenerate solutions of our loss. Interestingly, our simple fine-tuning approach achieves highly competitive performances in comparison to state-of-the-art methods, while being applicable to black-box settings and removing the need for specific episodic pre-training strategies. Furthermore, we introduce a new benchmark to assess the robustness of the competing methods to domain shifts. In this setting, our fine-tuning baseline obtains consistently better results than meta-learning methods.
少量学习(Few-shot learning)最近在药物发现领域引起了广泛关注,相关的文献数量快速增加,主要涉及到复杂的元学习(meta-learning)策略。我们重新审视了针对分子数据的更直接的微调(fine-tuning)方法,并提出了一种基于马氏距离(Mahalanobis distance)的正则化二次探针损失(quadratic-probe loss)。我们设计了一个专用的块坐标下降优化器(block-coordinate descent optimizer),避免了我们的损失函数的退化解。有趣的是,我们简单的微调方法在与最新方法的比较中表现出了极具竞争力的性能,同时适用于黑盒设置,无需特定的情境预训练策略。此外,我们还引入了一个新的基准测试来评估各种方法对不同领域变化的稳健性。在这种情况下,我们的微调基线始终获得比元学习方法更好的结果。
论文及项目相关链接
PDF Published in Transactions on Machine Learning Research (02/2025)
Summary
简洁总结文本内容:研究团队重新审视了药物发现领域中的少样本学习技术,提出了一种基于马氏距离的正规化二次探针损失函数。设计了一种专用的块坐标下降优化器,避免了损失函数的退化解。简单的微调方法实现了与最新技术的高度竞争性能,适用于黑盒设置,无需特定的阶段性预训练策略。此外,研究团队还引入了一个新的基准测试来评估不同方法在不同领域转移中的稳健性,微调基线在此设置中表现优异。
Key Takeaways
以下是七个关键见解:
- 研究团队关注于少样本学习在药物发现领域的应用。
- 他们提出了一种基于马氏距离的正规化二次探针损失函数。
- 专用的块坐标下降优化器用于避免损失函数的退化解。
- 简单的微调方法实现了与最新技术的高度竞争性能。
- 该方法适用于黑盒设置,无需特定的阶段性预训练策略。
- 研究团队引入了一个新的基准测试来评估不同方法的稳健性。
点此查看论文截图
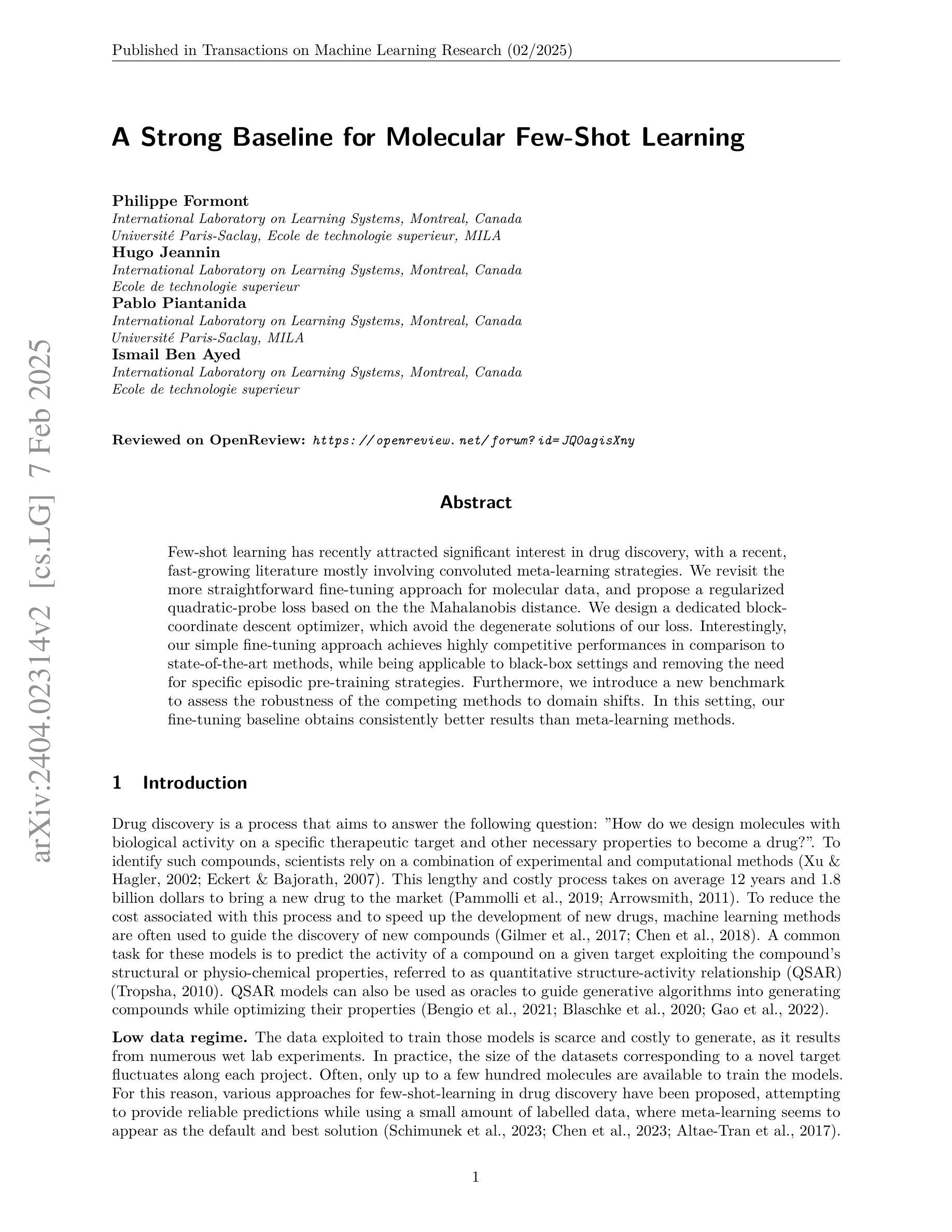
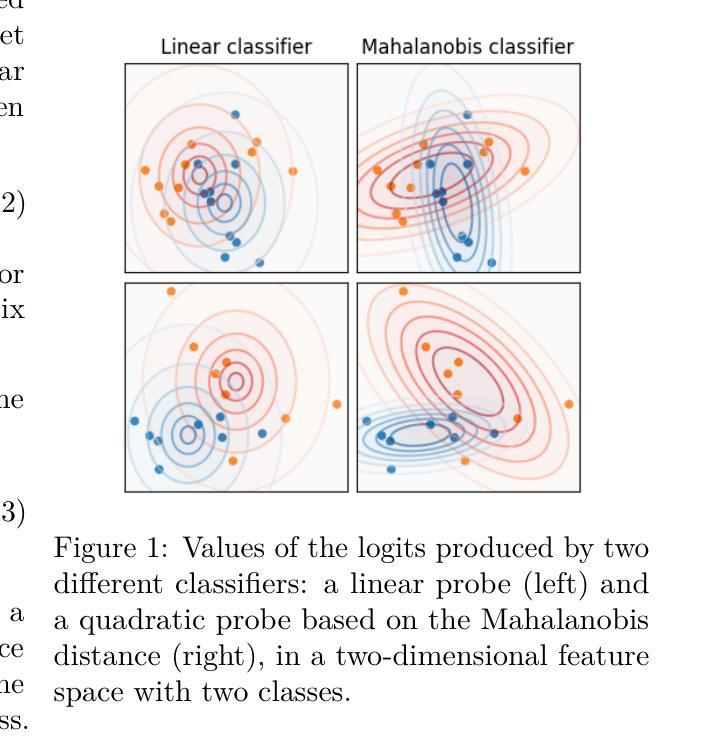