⚠️ 以下所有内容总结都来自于 大语言模型的能力,如有错误,仅供参考,谨慎使用
🔴 请注意:千万不要用于严肃的学术场景,只能用于论文阅读前的初筛!
💗 如果您觉得我们的项目对您有帮助 ChatPaperFree ,还请您给我们一些鼓励!⭐️ HuggingFace免费体验
2025-02-11 更新
Overcoming Fake Solutions in Semi-Dual Neural Optimal Transport: A Smoothing Approach for Learning the Optimal Transport Plan
Authors:Jaemoo Choi, Jaewoong Choi, Dohyun Kwon
We address the convergence problem in learning the Optimal Transport (OT) map, where the OT Map refers to a map from one distribution to another while minimizing the transport cost. Semi-dual Neural OT, a widely used approach for learning OT Maps with neural networks, often generates fake solutions that fail to transfer one distribution to another accurately. We identify a sufficient condition under which the max-min solution of Semi-dual Neural OT recovers the true OT Map. Moreover, to address cases when this sufficient condition is not satisfied, we propose a novel method, OTP, which learns both the OT Map and the Optimal Transport Plan, representing the optimal coupling between two distributions. Under sharp assumptions on the distributions, we prove that our model eliminates the fake solution issue and correctly solves the OT problem. Our experiments show that the OTP model recovers the optimal transport map where existing methods fail and outperforms current OT-based models in image-to-image translation tasks. Notably, the OTP model can learn stochastic transport maps when deterministic OT Maps do not exist, such as one-to-many tasks like colorization.
我们解决了学习最佳传输(OT)地图时的收敛性问题,其中OT地图是指从一个分布到另一个分布的最小化传输成本的映射。半双神经网络OT是一种广泛用于学习神经网络OT地图的方法,但经常产生假解,无法准确地将一个分布转移到另一个分布。我们确定了半双神经网络OT的最大最小解恢复真实OT地图的充分条件。此外,为了处理不满足这个充分条件的情况,我们提出了一种新方法OTP,它同时学习OT地图和最佳传输计划,代表两个分布之间的最佳耦合。在关于分布的尖锐假设下,我们证明了我们的模型消除了假解问题并正确地解决了OT问题。我们的实验表明,在现有方法失败的情况下,OTP模型恢复了最佳传输地图,并在图像到图像的翻译任务中优于当前的基于OT的模型。值得注意的是,当不存在确定性OT地图时,如一对一任务(如颜色化)中的一对多任务,OTP模型可以学习随机传输地图。
论文及项目相关链接
PDF 18 pages, 10 figures
Summary
半对偶神经最优传输(OT)地图学习中的收敛问题得到解决。针对半对偶神经OT中经常出现的生成虚假解的问题,提出了一种新的方法OTP。该方法能学习OT地图和最优传输计划,代表两个分布之间的最优耦合。在锐利的假设条件下,证明了OTP模型能够消除虚假解并正确解决OT问题。实验表明,OTP模型在图像翻译任务中优于当前基于OT的模型,并能够在不存在确定性OT地图的情况下学习随机传输地图,如颜色化等一对一至多任务。
Key Takeaways
- 解决半对偶神经最优传输(OT)地图学习中的收敛问题。
- 半对偶神经OT方法存在生成虚假解的问题,可能影响分布的准确转换。
- 引入新方法OTP,能学习OT地图和最优传输计划,提供两个分布之间的最优耦合。
- 在特定假设下,OTP模型能消除虚假解,正确解决OT问题。
- OTP模型在图像翻译任务中表现优越,优于其他OT模型。
- OTP模型能在不存在确定性OT地图的情况下学习随机传输地图。
点此查看论文截图
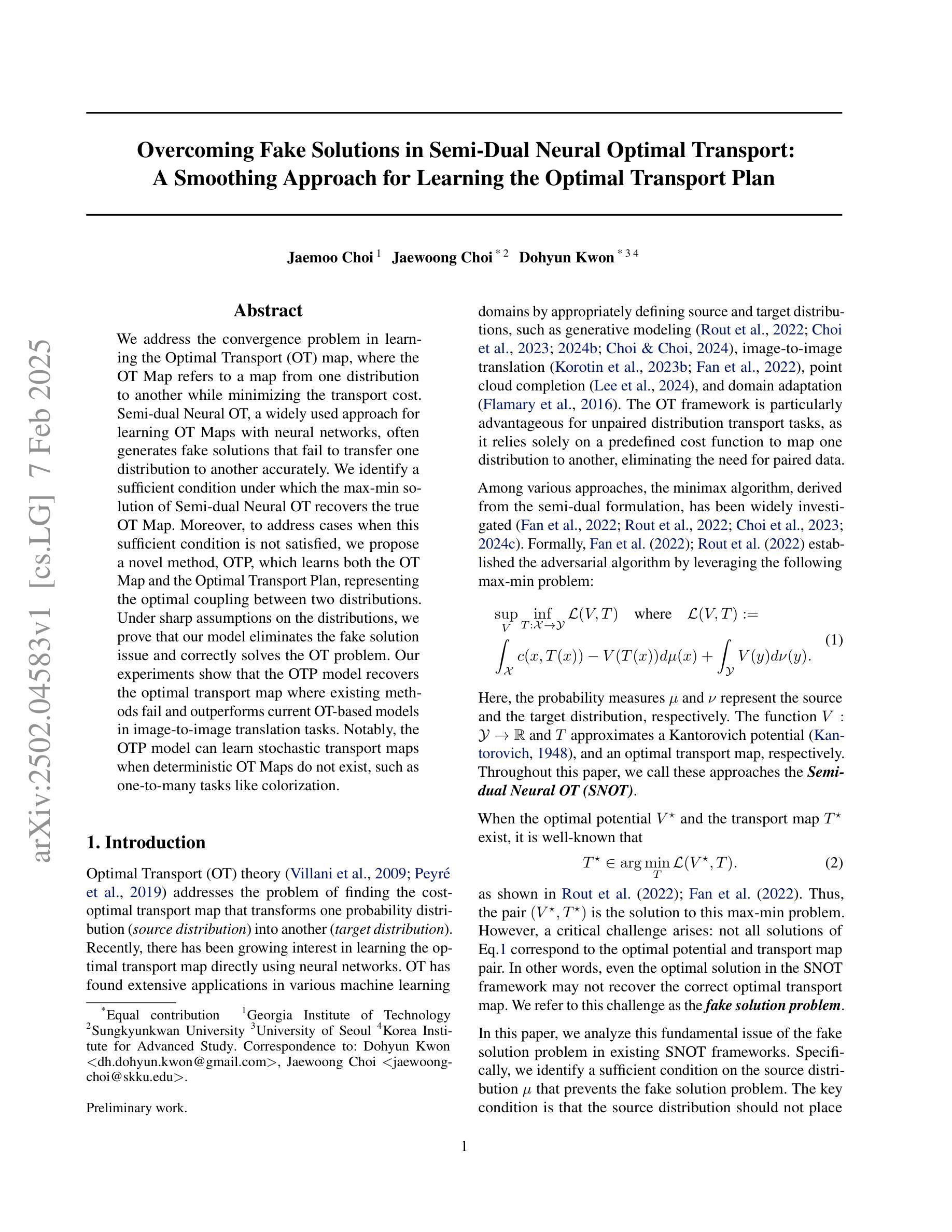
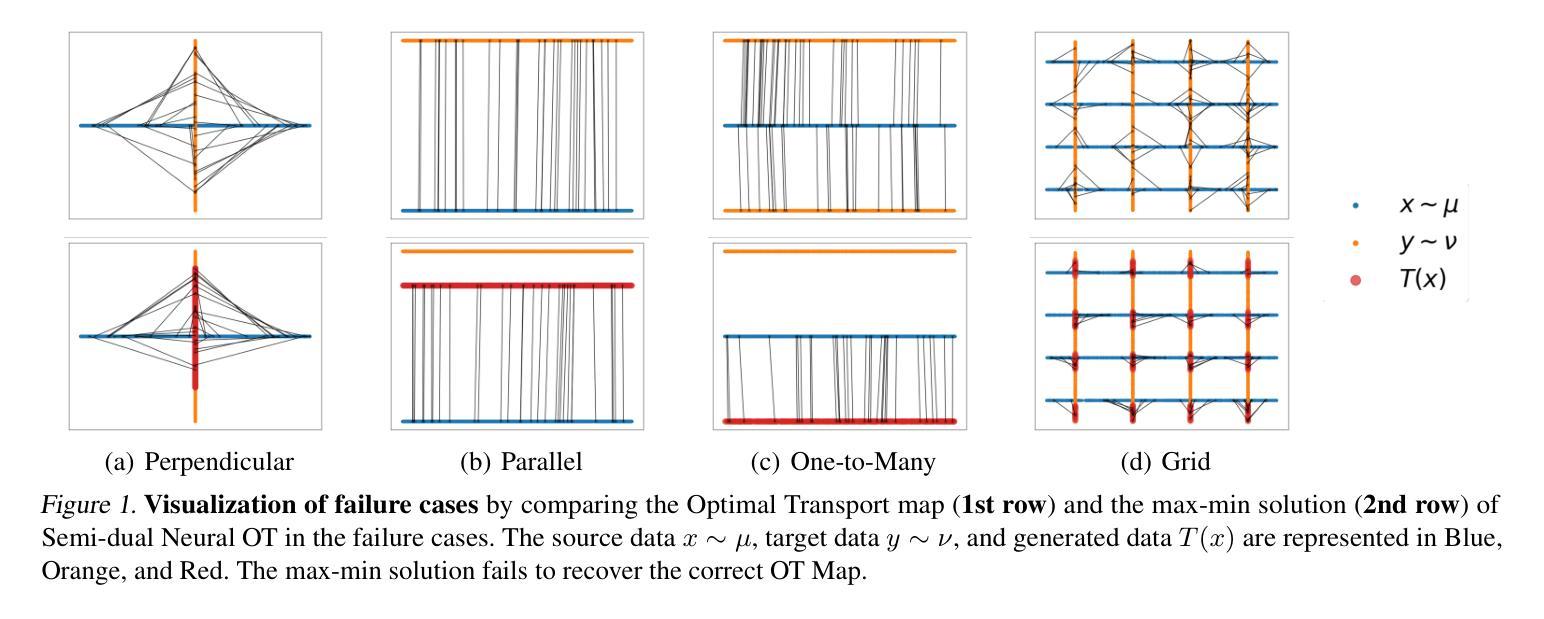
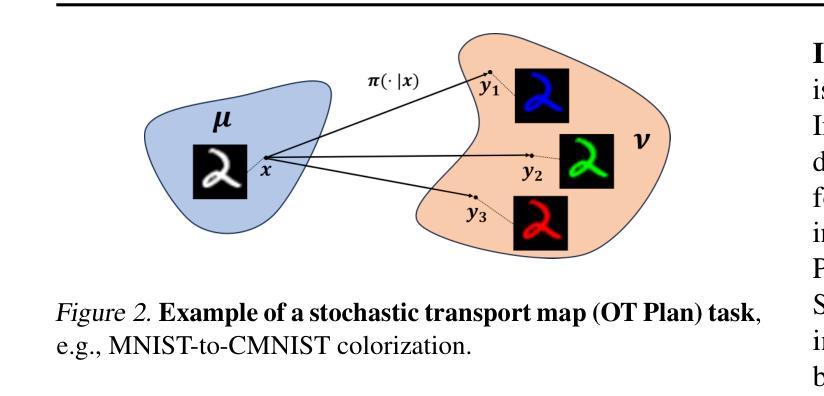