⚠️ 以下所有内容总结都来自于 大语言模型的能力,如有错误,仅供参考,谨慎使用
🔴 请注意:千万不要用于严肃的学术场景,只能用于论文阅读前的初筛!
💗 如果您觉得我们的项目对您有帮助 ChatPaperFree ,还请您给我们一些鼓励!⭐️ HuggingFace免费体验
2025-02-11 更新
PoI: Pixel of Interest for Novel View Synthesis Assisted Scene Coordinate Regression
Authors:Feifei Li, Qi Song, Chi Zhang, Hui Shuai, Rui Huang
The task of estimating camera poses can be enhanced through novel view synthesis techniques such as NeRF and Gaussian Splatting to increase the diversity and extension of training data. However, these techniques often produce rendered images with issues like blurring and ghosting, which compromise their reliability. These issues become particularly pronounced for Scene Coordinate Regression (SCR) methods, which estimate 3D coordinates at the pixel level. To mitigate the problems associated with unreliable rendered images, we introduce a novel filtering approach, which selectively extracts well-rendered pixels while discarding the inferior ones. This filter simultaneously measures the SCR model’s real-time reprojection loss and gradient during training. Building on this filtering technique, we also develop a new strategy to improve scene coordinate regression using sparse inputs, drawing on successful applications of sparse input techniques in novel view synthesis. Our experimental results validate the effectiveness of our method, demonstrating state-of-the-art performance on indoor and outdoor datasets.
通过NeRF和高斯蒙版等新型视图合成技术,可以估计相机姿态任务,从而提高训练数据的多样性和扩展性。然而,这些技术产生的渲染图像往往存在模糊和重影等问题,从而影响其可靠性。这些问题在场景坐标回归(SCR)方法中尤为突出,这些方法会估计像素级的3D坐标。为了解决与不可靠渲染图像相关的问题,我们引入了一种新型过滤方法,该方法能够有选择地提取渲染良好的像素,同时丢弃质量较差的像素。该过滤器在训练过程中实时测量SCR模型的再投影损失和梯度。基于这种过滤技术,我们还开发了一种利用稀疏输入改进场景坐标回归的新策略,借鉴了稀疏输入技术在新型视图合成中的成功应用。我们的实验结果验证了该方法的有效性,在室内和室外数据集上均表现出卓越的性能。
论文及项目相关链接
Summary
本文介绍了如何通过NeRF和Gaussian Splatting等新型视图合成技术提高相机姿态估计任务的性能,并增加训练数据的多样性和扩展性。然而,这些技术生成的渲染图像经常出现模糊和重影等问题,影响可靠性。针对场景坐标回归(SCR)方法,本文提出了一种新的过滤方法,该方法能够选择性地提取渲染良好的像素并丢弃质量较差的像素。此过滤器同时测量SCR模型的实时重投影损失和训练过程中的梯度。在此基础上,我们还开发了一种利用稀疏输入改进场景坐标回归的新策略,借鉴了稀疏输入在新型视图合成中的成功应用。实验结果验证了该方法的有效性,在室内和室外数据集上均表现出卓越的性能。
Key Takeaways
- 利用NeRF和Gaussian Splatting等新技术提高了相机姿态估计的效果和训练数据的多样性。
- 渲染图像存在模糊和重影问题,影响技术可靠性。
- 提出了一种新的过滤方法,能够选择性地提取高质量的渲染像素,同时测量场景坐标回归模型的实时重投影损失和梯度。
- 开发了利用稀疏输入改进场景坐标回归的策略,借鉴了稀疏输入在视图合成中的成功应用。
- 过滤技术和稀疏输入策略的结合提高了方法的有效性。
- 实验结果证明了该方法在室内和室外数据集上的卓越性能。
点此查看论文截图
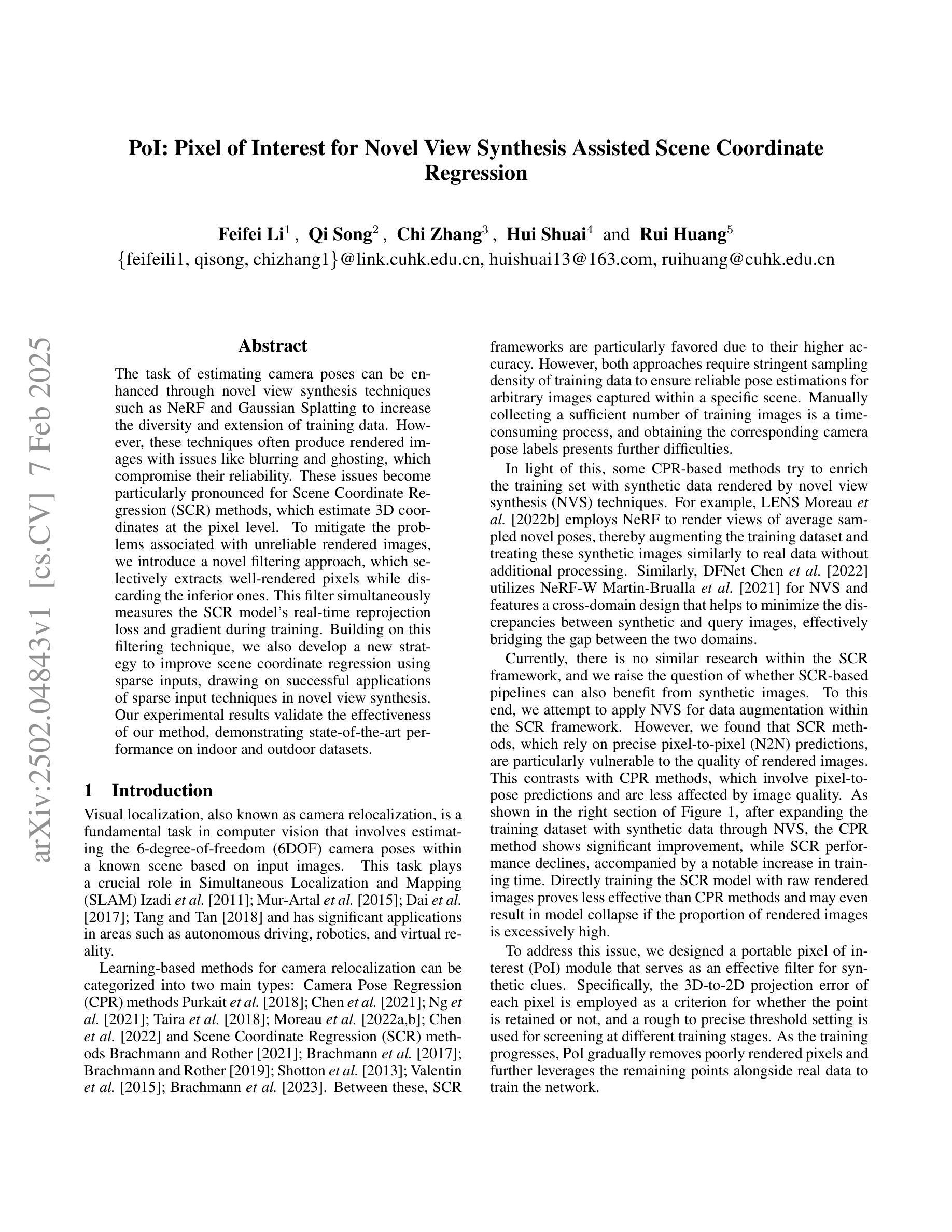
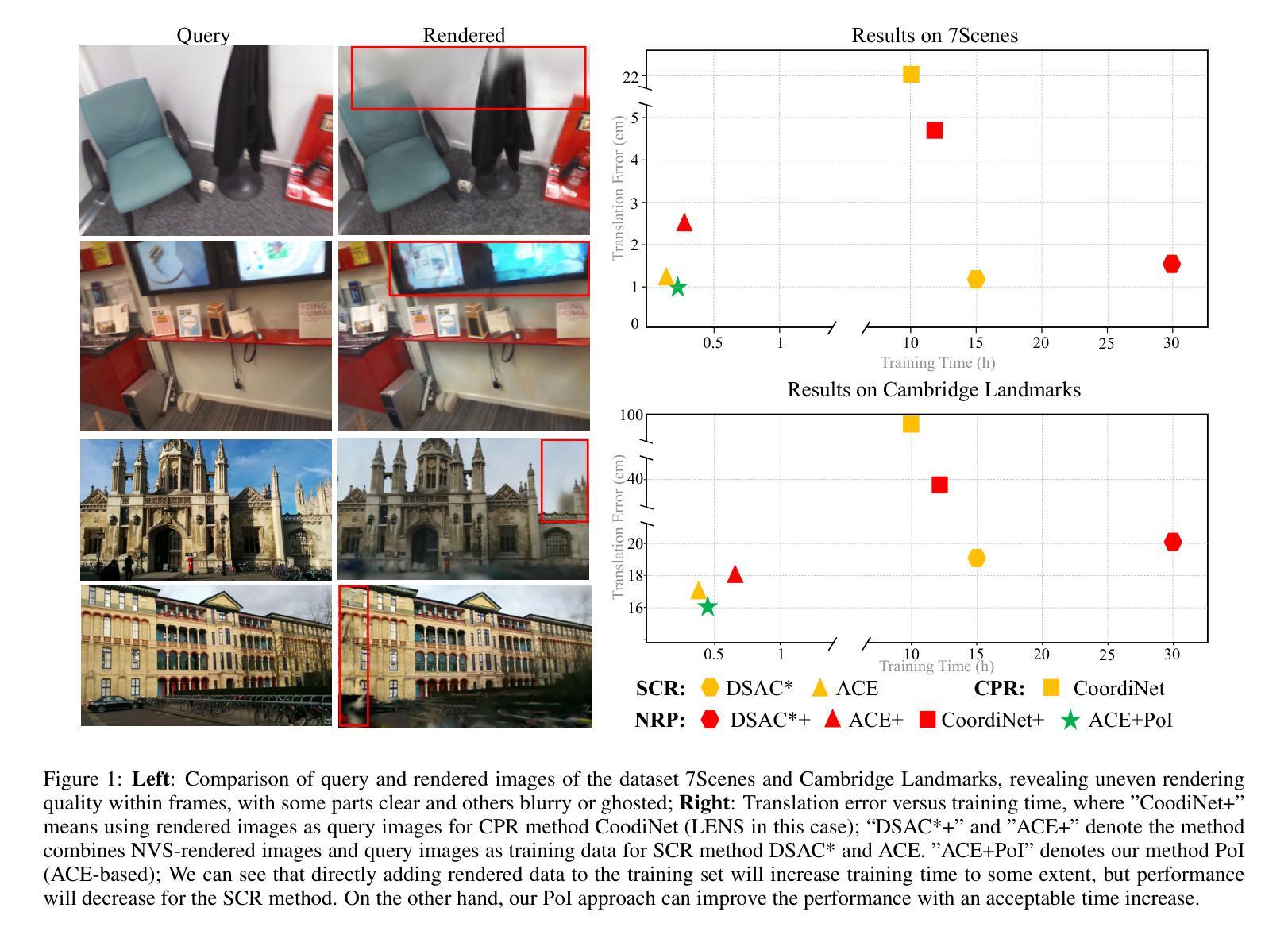
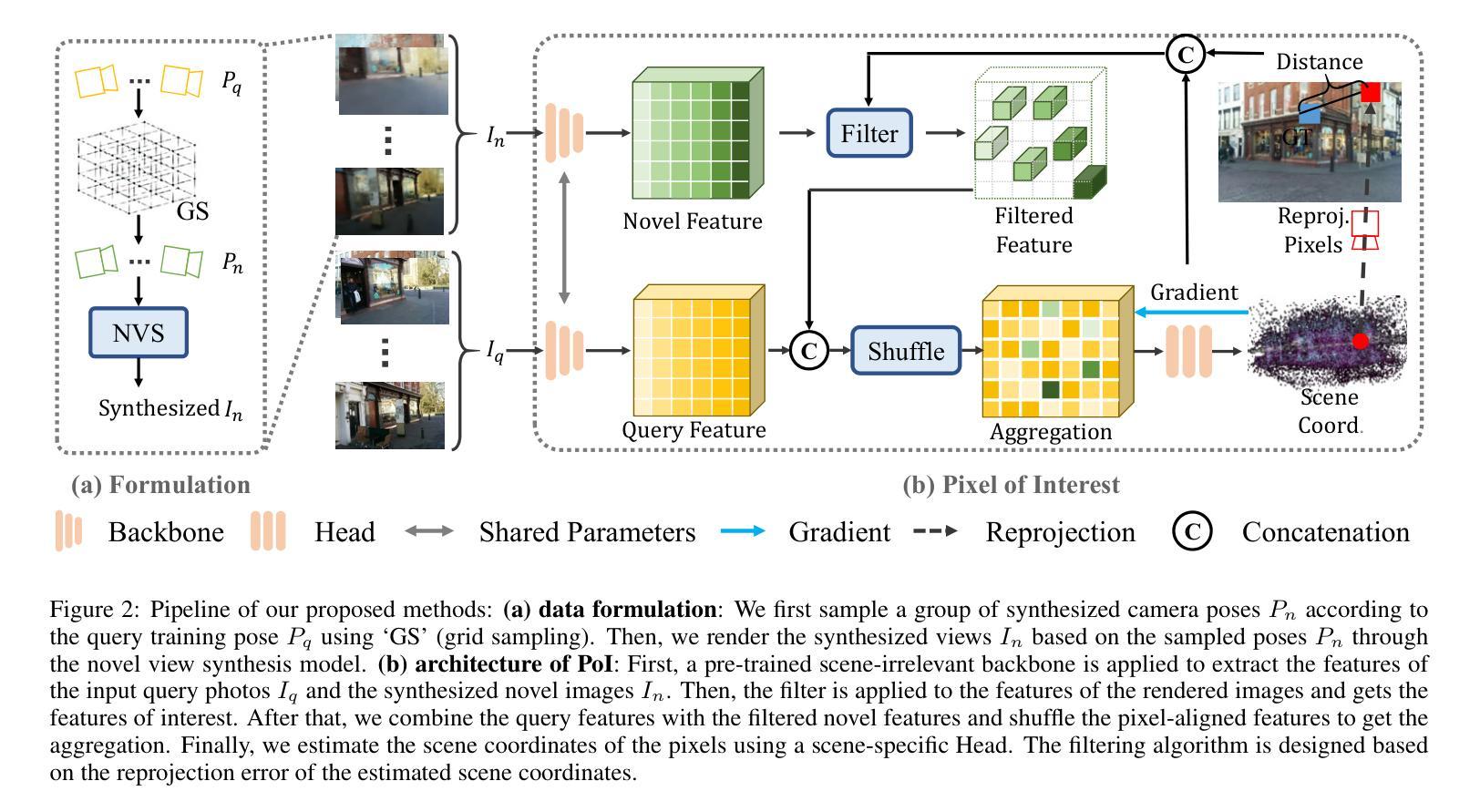
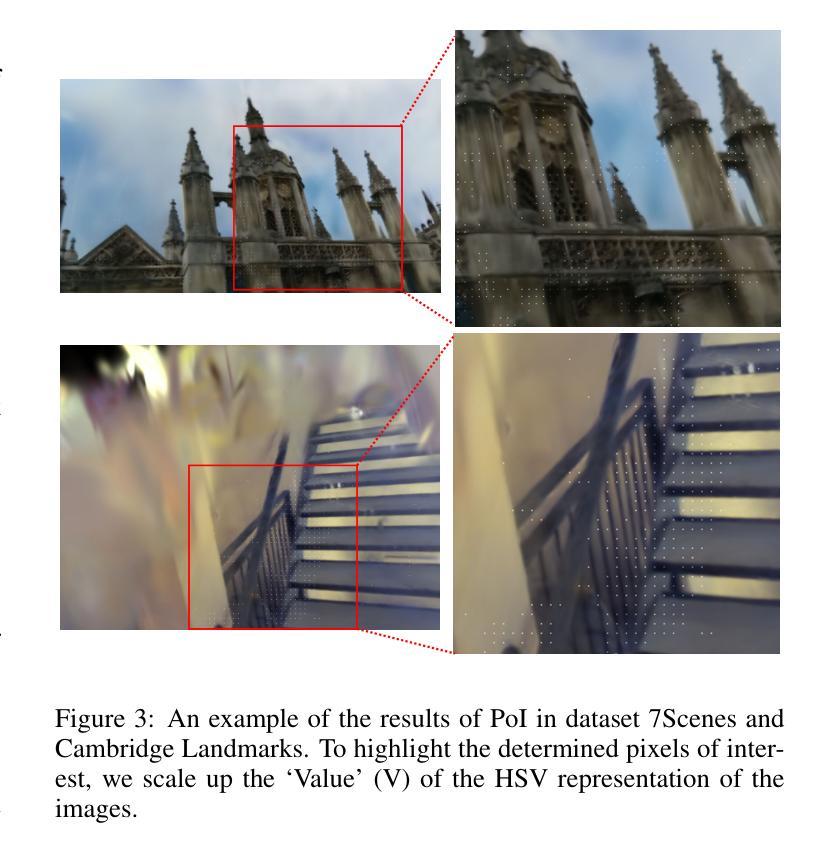
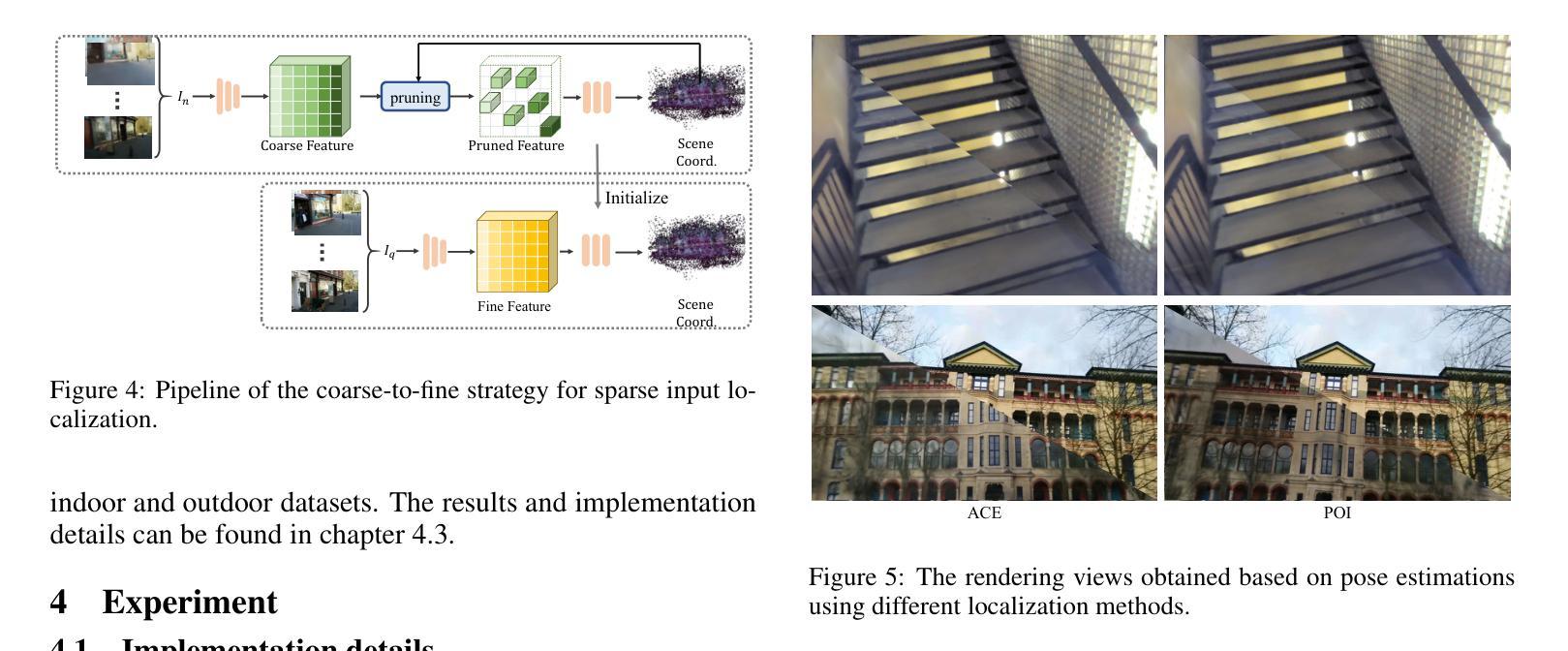
AniSDF: Fused-Granularity Neural Surfaces with Anisotropic Encoding for High-Fidelity 3D Reconstruction
Authors:Jingnan Gao, Zhuo Chen, Xiaokang Yang, Yichao Yan
Neural radiance fields have recently revolutionized novel-view synthesis and achieved high-fidelity renderings. However, these methods sacrifice the geometry for the rendering quality, limiting their further applications including relighting and deformation. How to synthesize photo-realistic rendering while reconstructing accurate geometry remains an unsolved problem. In this work, we present AniSDF, a novel approach that learns fused-granularity neural surfaces with physics-based encoding for high-fidelity 3D reconstruction. Different from previous neural surfaces, our fused-granularity geometry structure balances the overall structures and fine geometric details, producing accurate geometry reconstruction. To disambiguate geometry from reflective appearance, we introduce blended radiance fields to model diffuse and specularity following the anisotropic spherical Gaussian encoding, a physics-based rendering pipeline. With these designs, AniSDF can reconstruct objects with complex structures and produce high-quality renderings. Furthermore, our method is a unified model that does not require complex hyperparameter tuning for specific objects. Extensive experiments demonstrate that our method boosts the quality of SDF-based methods by a great scale in both geometry reconstruction and novel-view synthesis.
神经辐射场最近已经革新了新型视图合成技术,并实现了高保真渲染。然而,这些方法为了渲染质量牺牲了几何结构,限制了其在重新打光和变形等进一步应用中的使用。如何在重建准确几何结构的同时合成逼真的渲染仍然是一个未解决的问题。在这项工作中,我们提出了AniSDF,这是一种新型方法,它通过基于物理编码的融合粒度神经表面学习进行高保真3D重建。不同于之前的神经表面,我们的融合粒度几何结构在整体结构和精细几何细节之间达到了平衡,从而实现了准确的几何重建。为了从反射外观中区分几何结构,我们引入了混合辐射场,根据物理渲染管道采用各向异性球面高斯编码来模拟漫反射和光泽反射。通过这些设计,AniSDF能够重建具有复杂结构的物体并产生高质量的渲染。此外,我们的方法是一个统一模型,不需要针对特定物体的复杂超参数调整。大量实验表明,我们的方法在基于SDF的方法的几何重建和新型视图合成方面都有大幅度提升。
论文及项目相关链接
PDF Accepted by ICLR2025, Project Page: https://g-1nonly.github.io/AniSDF_Website/
Summary
神经网络辐射场(NeRF)已革新了新型视图合成并实现了高保真渲染。但现有方法为了渲染质量牺牲了几何结构,限制了其在重新照明和变形等应用中的使用。本文提出的AniSDF方法通过物理编码融合粒度神经表面重建实现高保真三维重建。相较于先前的神经表面技术,我们的融合粒度几何结构平衡了整体结构和精细几何细节,从而获得更准确的几何重建。我们引入混合辐射场来模拟漫反射和光泽反射,采用基于物理的渲染管道进行各向异性球面高斯编码来消除几何与外观之间的歧义。这些方法使AniSDF能重建复杂结构物体并产生高质量渲染。此外,我们的模型是统一模型,不需要针对特定物体进行复杂超参数调整。实验证明,在几何重建和新型视图合成方面,我们的方法极大地提高了基于SDF的方法的质量。
Key Takeaways
- NeRF技术已革新了视图合成领域,实现了高保真渲染。
- 现有NeRF技术为追求渲染质量牺牲了几何结构,限制了其在其他应用中的使用。
- 提出了一种名为AniSDF的新方法,通过融合粒度神经表面重建实现了几何与渲染的平衡。
- AniSDF采用物理编码技术,包括混合辐射场和各向异性球面高斯编码来模拟复杂的光照和反射现象。
- AniSDF能够重建具有复杂结构的物体并产生高质量渲染结果。
- 该方法是统一的模型,无需针对特定物体的复杂超参数调整。
点此查看论文截图
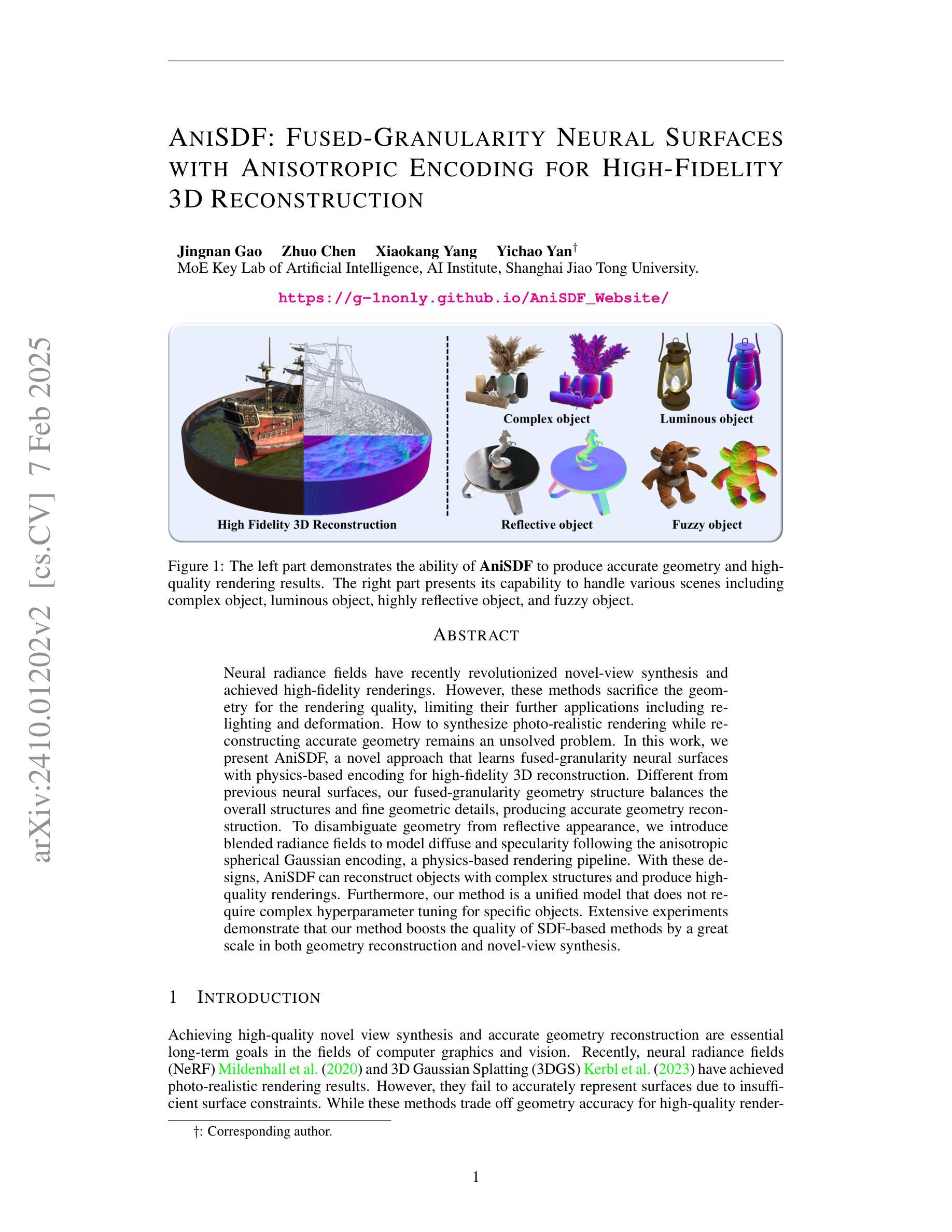
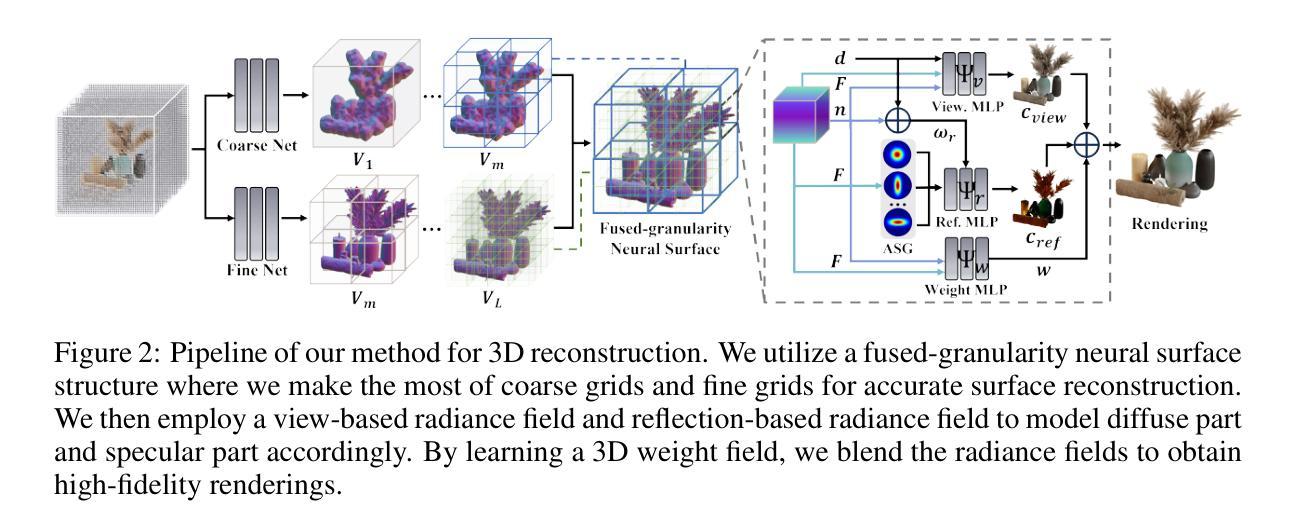