⚠️ 以下所有内容总结都来自于 大语言模型的能力,如有错误,仅供参考,谨慎使用
🔴 请注意:千万不要用于严肃的学术场景,只能用于论文阅读前的初筛!
💗 如果您觉得我们的项目对您有帮助 ChatPaperFree ,还请您给我们一些鼓励!⭐️ HuggingFace免费体验
2025-02-11 更新
Evaluating Standard and Dialectal Frisian ASR: Multilingual Fine-tuning and Language Identification for Improved Low-resource Performance
Authors:Reihaneh Amooie, Wietse de Vries, Yun Hao, Jelske Dijkstra, Matt Coler, Martijn Wieling
Automatic Speech Recognition (ASR) performance for low-resource languages is still far behind that of higher-resource languages such as English, due to a lack of sufficient labeled data. State-of-the-art methods deploy self-supervised transfer learning where a model pre-trained on large amounts of data is fine-tuned using little labeled data in a target low-resource language. In this paper, we present and examine a method for fine-tuning an SSL-based model in order to improve the performance for Frisian and its regional dialects (Clay Frisian, Wood Frisian, and South Frisian). We show that Frisian ASR performance can be improved by using multilingual (Frisian, Dutch, English and German) fine-tuning data and an auxiliary language identification task. In addition, our findings show that performance on dialectal speech suffers substantially, and, importantly, that this effect is moderated by the elicitation approach used to collect the dialectal data. Our findings also particularly suggest that relying solely on standard language data for ASR evaluation may underestimate real-world performance, particularly in languages with substantial dialectal variation.
自动语音识别(ASR)在低资源语言上的表现仍然远远落后于英语等资源丰富语言,这主要是由于缺乏足够的标记数据。最先进的方法采用自监督迁移学习,其中预训练在大量数据上的模型使用少量目标低资源语言的标记数据进行微调。在本文中,我们提出并研究了一种基于SSL的模型的微调方法,以提高弗里西亚语及其方言(克莱弗里西亚语、伍德弗里西亚语和南部弗里西亚语)的性能。我们表明,通过使用多语言(弗里西亚语、荷兰语、英语和德语)微调数据和辅助语言识别任务,可以改善弗里西亚语的ASR性能。此外,我们的研究结果表明,方言语音的性能受到很大影响,而且这一影响受到用于收集方言数据的激发方法的影响。我们的研究还特别表明,仅依赖标准语言数据进行ASR评估可能会低估现实世界中的性能,特别是在具有大量方言差异的语言中。
论文及项目相关链接
Summary
本文主要探讨了针对低资源语言(如弗里斯兰语及其方言)的自动语音识别(ASR)性能提升的问题。文章指出由于缺乏足够的标注数据,使得这些语言的ASR性能相较于资源丰富的语言(如英语)仍有较大差距。文章提出了一种基于自监督学习(SSL)模型的微调方法,通过多语言(弗里斯兰语、荷兰语、英语和德语)的微调数据和辅助语言识别任务来提升弗里斯兰语的ASR性能。文章还发现,方言语音的性能会受到很大影响,且这种影响受到采集方言数据所用诱发方法的调节。此外,仅依赖标准语言数据进行ASR评估可能会低估真实世界性能,特别是在具有显著方言差异的语言中。
Key Takeaways
- 低资源语言的ASR性能提升面临的主要挑战是缺乏足够的标注数据。
- 自监督转移学习方法可以改善低资源语言的ASR性能。
- 通过多语言微调数据和辅助语言识别任务可以提升弗里斯兰语的ASR性能。
- 方言语音的性能会受到很大影响。
- 采集方言数据所用的诱发方法会影响方言语音的性能。
- 仅依赖标准语言数据进行ASR评估可能会低估真实世界性能。
点此查看论文截图
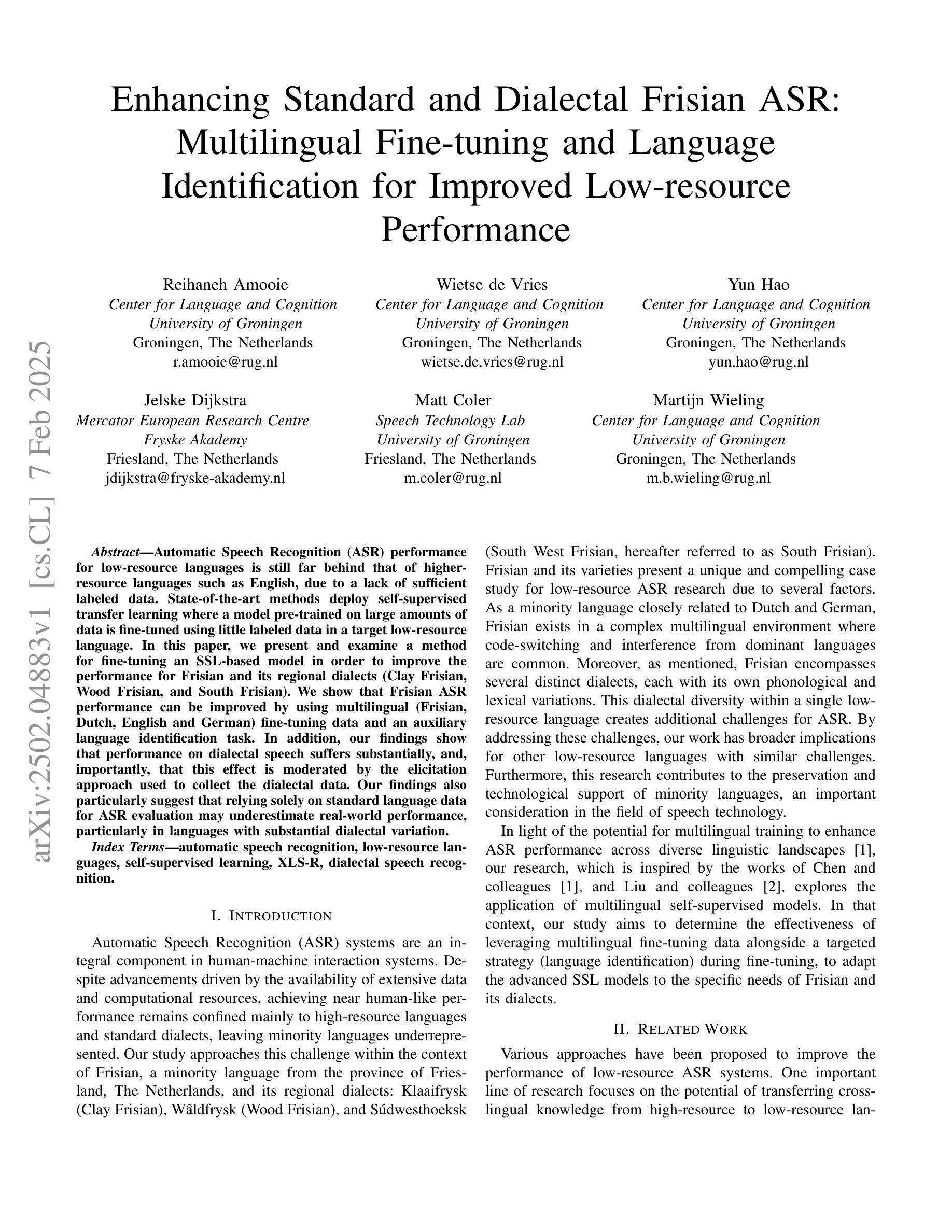
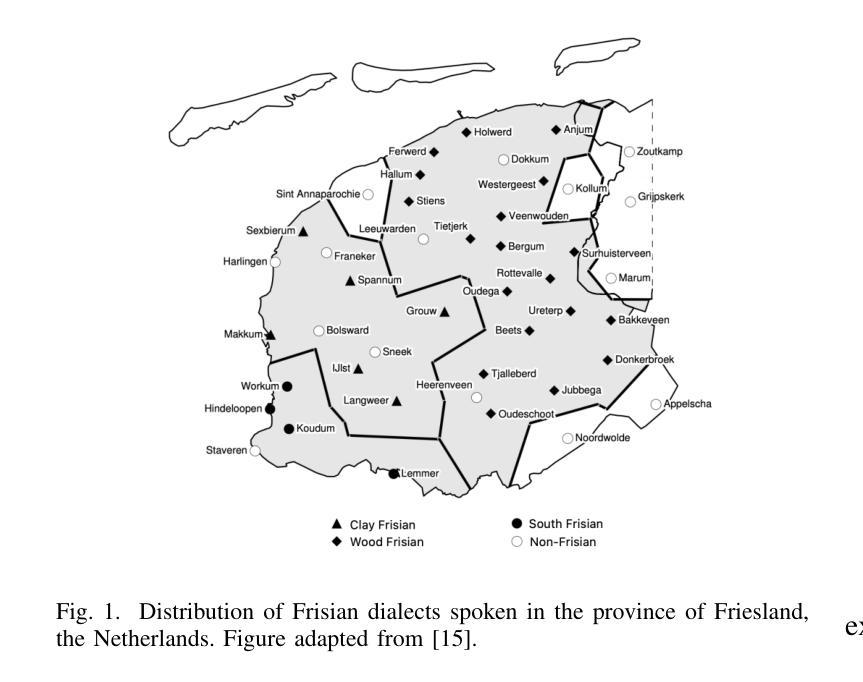
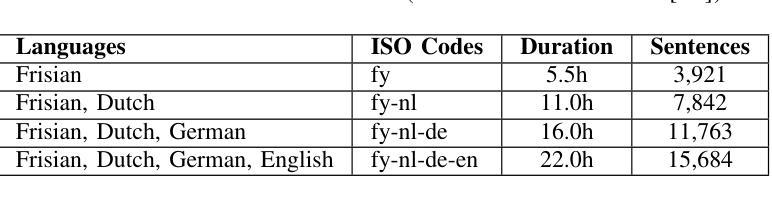
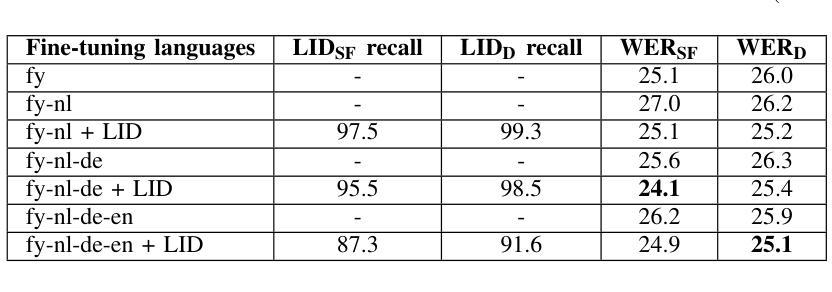
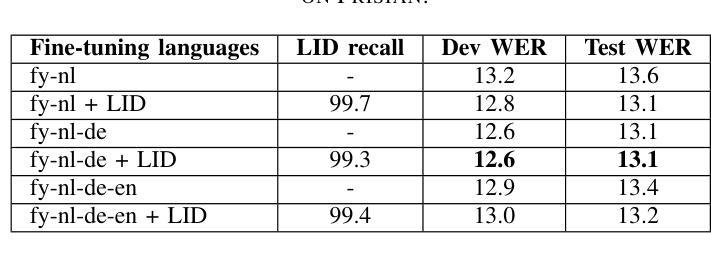
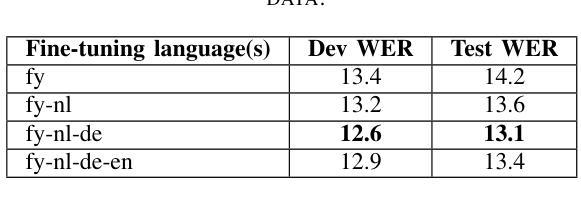
Dynamic Frequency-Adaptive Knowledge Distillation for Speech Enhancement
Authors:Xihao Yuan, Siqi Liu, Hanting Chen, Lu Zhou, Jian Li, Jie Hu
Deep learning-based speech enhancement (SE) models have recently outperformed traditional techniques, yet their deployment on resource-constrained devices remains challenging due to high computational and memory demands. This paper introduces a novel dynamic frequency-adaptive knowledge distillation (DFKD) approach to effectively compress SE models. Our method dynamically assesses the model’s output, distinguishing between high and low-frequency components, and adapts the learning objectives to meet the unique requirements of different frequency bands, capitalizing on the SE task’s inherent characteristics. To evaluate the DFKD’s efficacy, we conducted experiments on three state-of-the-art models: DCCRN, ConTasNet, and DPTNet. The results demonstrate that our method not only significantly enhances the performance of the compressed model (student model) but also surpasses other logit-based knowledge distillation methods specifically for SE tasks.
基于深度学习的语音增强(SE)模型最近已经超越了传统技术,但由于计算和内存需求较高,它们在资源受限设备上的部署仍然具有挑战性。本文介绍了一种新的动态频率自适应知识蒸馏(DFKD)方法,以有效地压缩SE模型。我们的方法动态评估模型输出,区分高低频成分,并适应学习目标以满足不同频带的独特要求,充分利用SE任务的固有特性。为了评估DFKD的有效性,我们在三种最新模型:DCCRN、ConTasNet和DPTNet上进行了实验。结果表明,我们的方法不仅显著提高了压缩模型(学生模型)的性能,而且超越了其他专门针对SE任务的对数知识蒸馏方法。
论文及项目相关链接
PDF 5 pages, 2 figures, accepted by ICASSP2025
Summary
深度学习在语音增强领域的应用取得了突破性进展,然而资源受限的设备部署仍然面临挑战。本文提出了一种新颖的基于动态频率自适应知识蒸馏(DFKD)的方法,以压缩和优化语音增强模型。通过动态评估模型输出、区分不同频率成分,并根据不同频率段的特点调整学习目标,DFKD策略有效提高了模型的性能并减少了资源消耗。通过实验验证了该方法的优异表现。
Key Takeaways
- 深度学习在语音增强任务上展现出强大性能。
- 传统的语音增强技术在资源受限的设备上部署具有挑战。
- 动态频率自适应知识蒸馏(DFKD)是一种新颖的压缩和优化语音增强模型的方法。
- DFKD策略通过动态评估模型输出和调整学习目标以提高模型性能。
- DFKD方法显著提高了压缩模型的性能,并超越了专门为语音增强任务设计的logit知识蒸馏方法。
- 实验验证了DFKD方法在不同先进模型(如DCCRN、ConTasNet和DPTNet)上的有效性。
点此查看论文截图
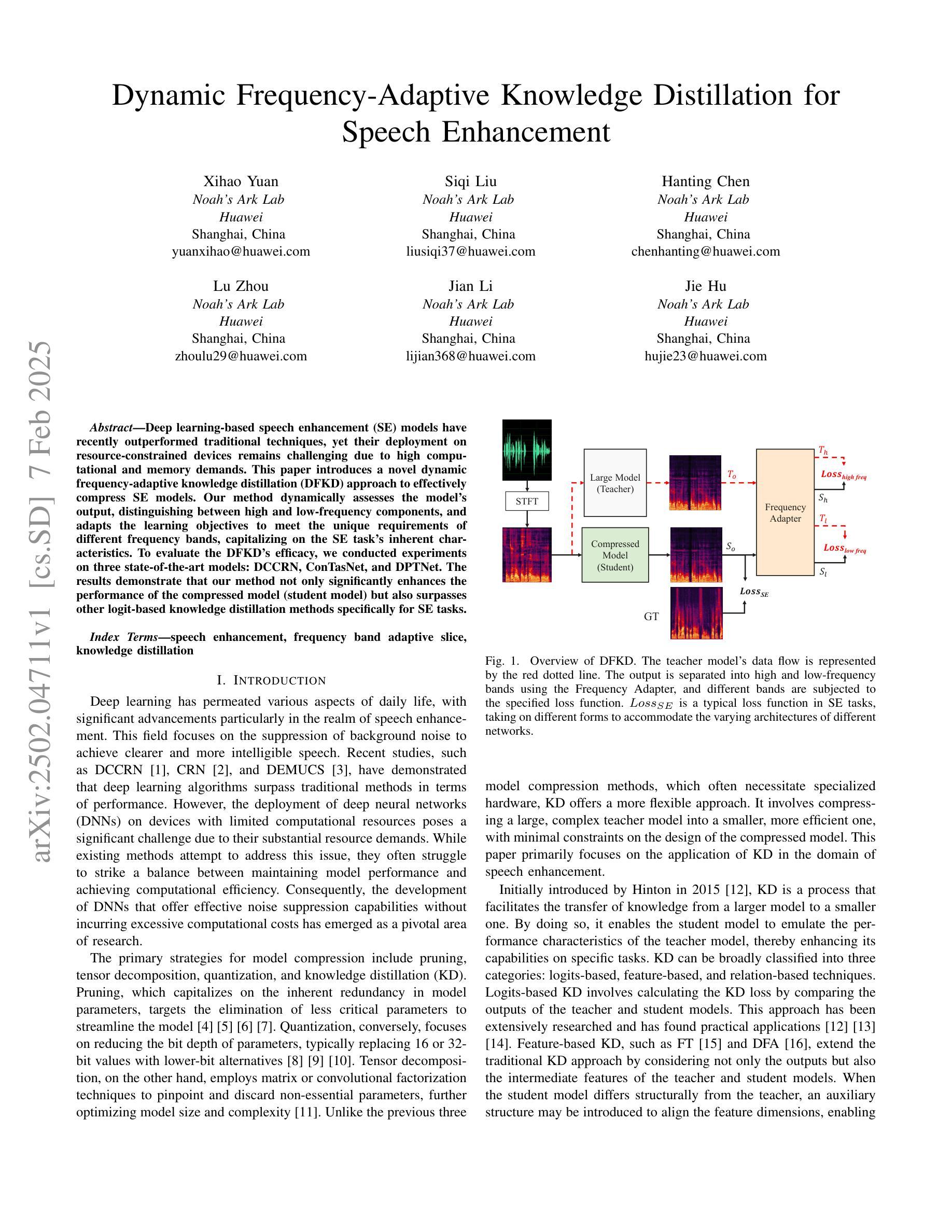
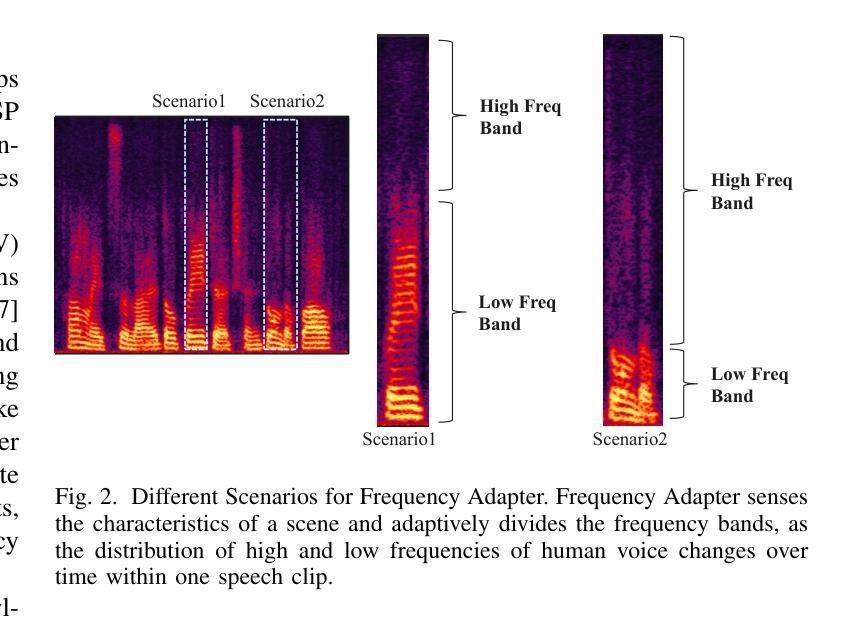
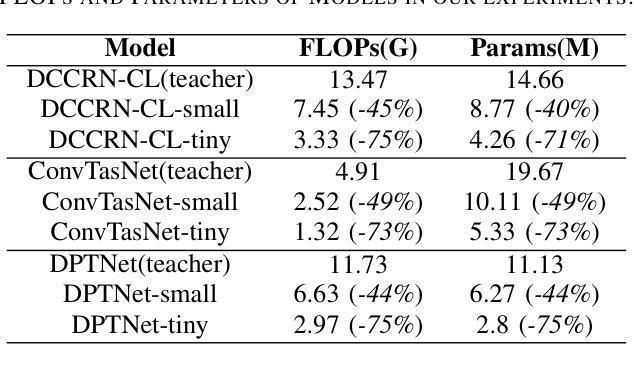
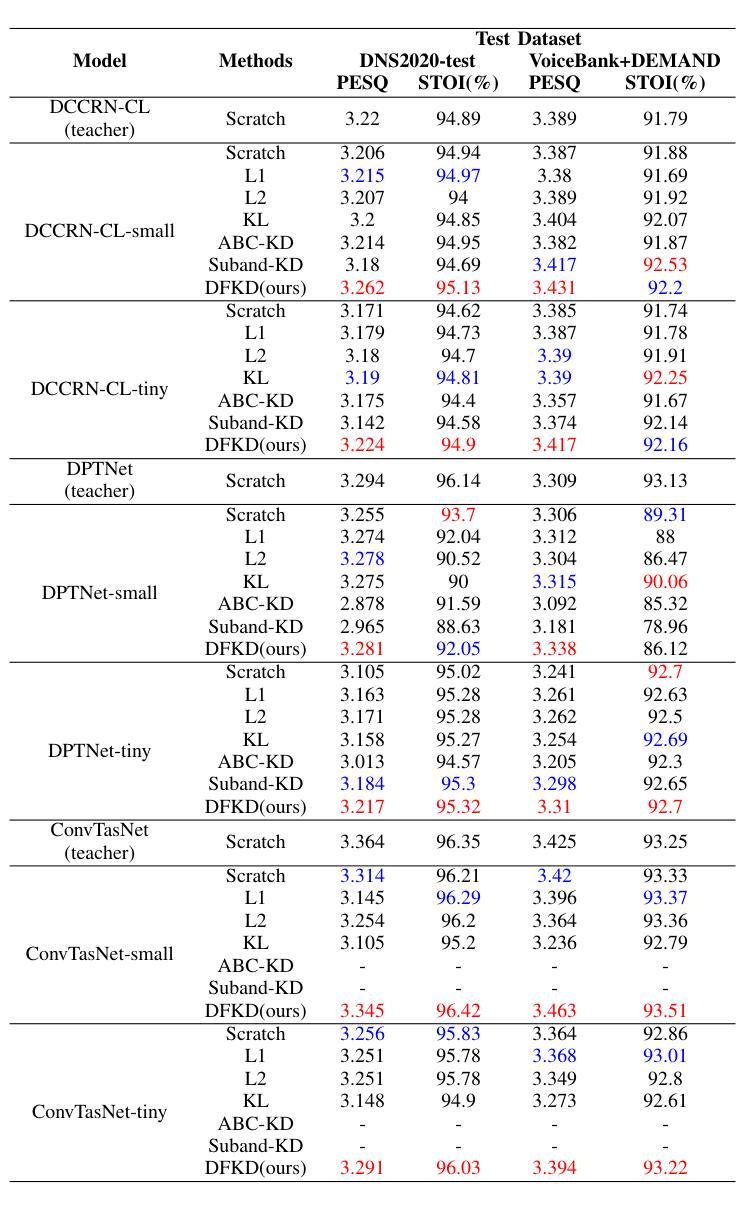
GenVC: Self-Supervised Zero-Shot Voice Conversion
Authors:Zexin Cai, Henry Li Xinyuan, Ashi Garg, Leibny Paola García-Perera, Kevin Duh, Sanjeev Khudanpur, Matthew Wiesner, Nicholas Andrews
Zero-shot voice conversion has recently made substantial progress, but many models still depend on external supervised systems to disentangle speaker identity and linguistic content. Furthermore, current methods often use parallel conversion, where the converted speech inherits the source utterance’s temporal structure, restricting speaker similarity and privacy. To overcome these limitations, we introduce GenVC, a generative zero-shot voice conversion model. GenVC learns to disentangle linguistic content and speaker style in a self-supervised manner, eliminating the need for external models and enabling efficient training on large, unlabeled datasets. Experimental results show that GenVC achieves state-of-the-art speaker similarity while maintaining naturalness competitive with leading approaches. Its autoregressive generation also allows the converted speech to deviate from the source utterance’s temporal structure. This feature makes GenVC highly effective for voice anonymization, as it minimizes the preservation of source prosody and speaker characteristics, enhancing privacy protection.
零样本语音转换最近取得了重大进展,但许多模型仍然依赖外部监督系统来分离说话人身份和语言内容。此外,当前的方法经常使用并行转换,在这种转换中,转换后的语音继承了源话语的时间结构,限制了说话人的相似性和隐私保护。为了克服这些局限性,我们引入了GenVC,这是一个生成式零样本语音转换模型。GenVC以自监督的方式学习分离语言内容和说话人风格,从而无需外部模型,并能够在大型无标签数据集上进行高效训练。实验结果表明,GenVC在保持自然性的同时,实现了最先进的说话人相似性。其自回归生成也允许转换后的语音偏离源话语的时间结构。这一特点使得GenVC在语音匿名化方面非常有效,因为它最大限度地减少了源韵律和说话人特征的保留,增强了隐私保护。
论文及项目相关链接
Summary
新一代零样本语音转换技术GenVC,通过自监督方式学习分离语言内容和说话人风格,无需外部模型,可在大规模无标签数据集上进行高效训练。它实现了出色的说话人相似性,同时保持自然度,并支持偏离源语音的生成,为语音匿名化和隐私保护提供了强大支持。
Key Takeaways
- GenVC是一种先进的零样本语音转换模型。
- 它采用自监督方式学习分离语言内容和说话人风格。
- GenVC无需依赖外部模型,可高效利用大规模无标签数据集进行训练。
- GenVC实现了出色的说话人相似性,同时保持自然度。
- GenVC支持偏离源语音的生成,增强了语音匿名化和隐私保护能力。
- 现有模型常采用平行转换,限制了说话人的相似性和隐私保护。而GenVC则突破了这一局限。
点此查看论文截图
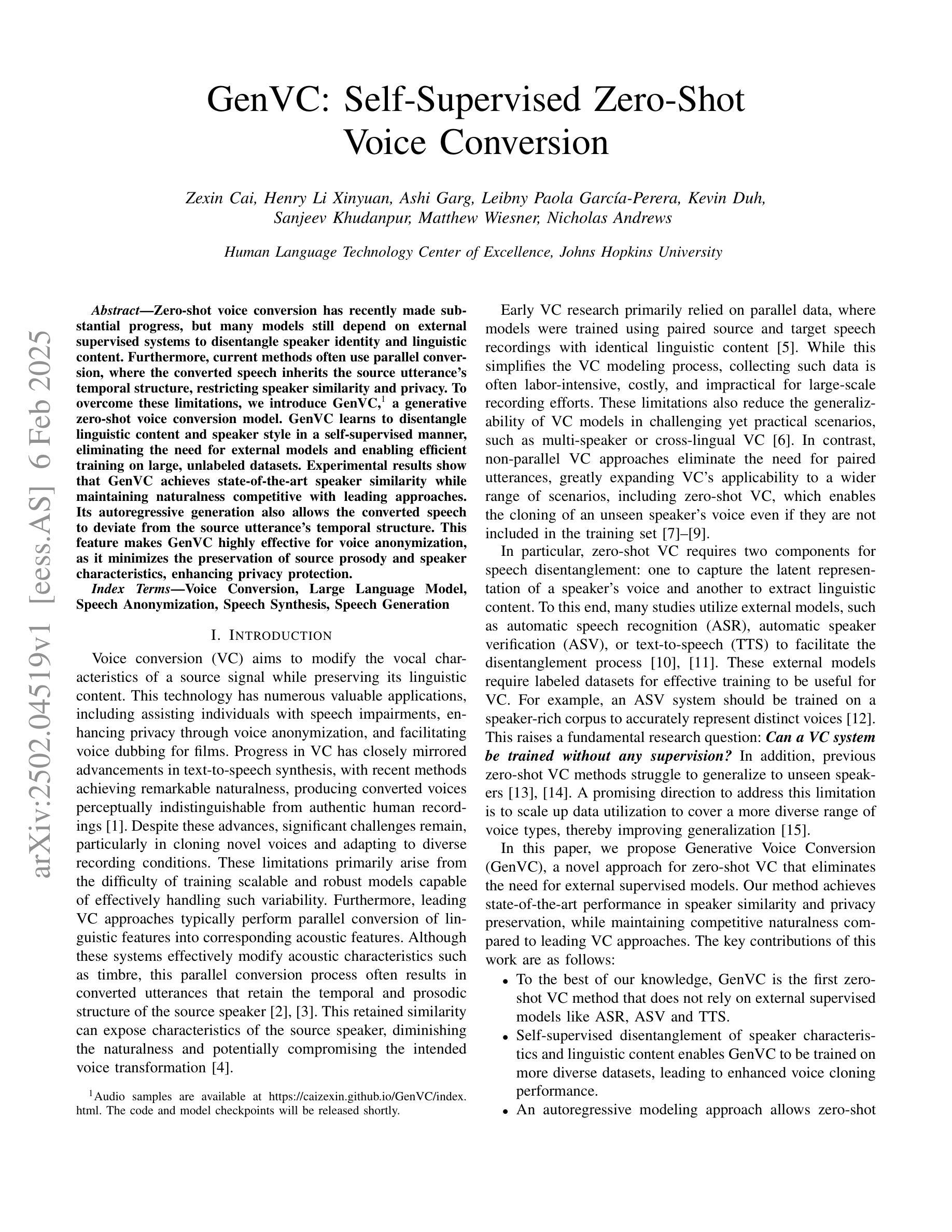
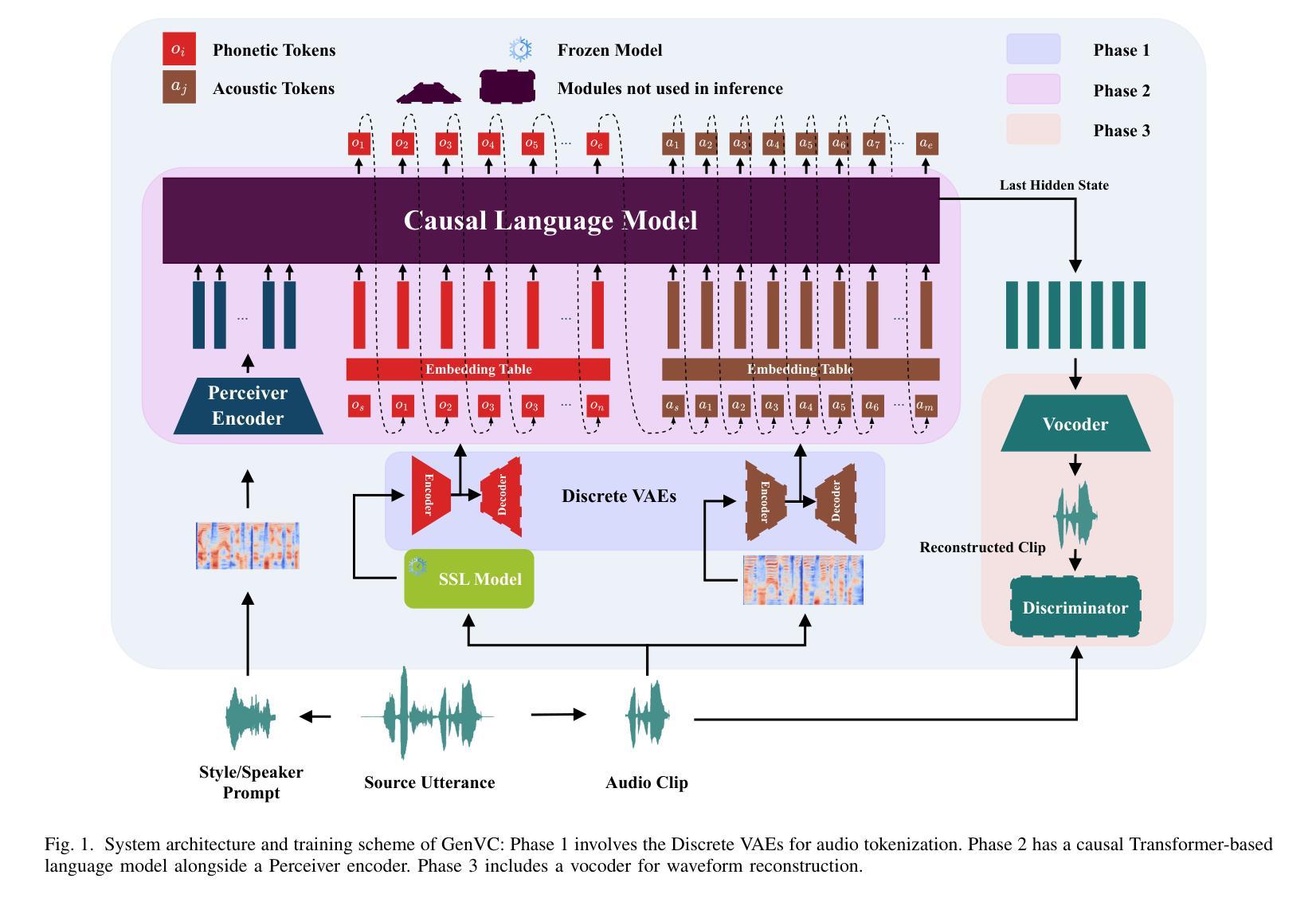
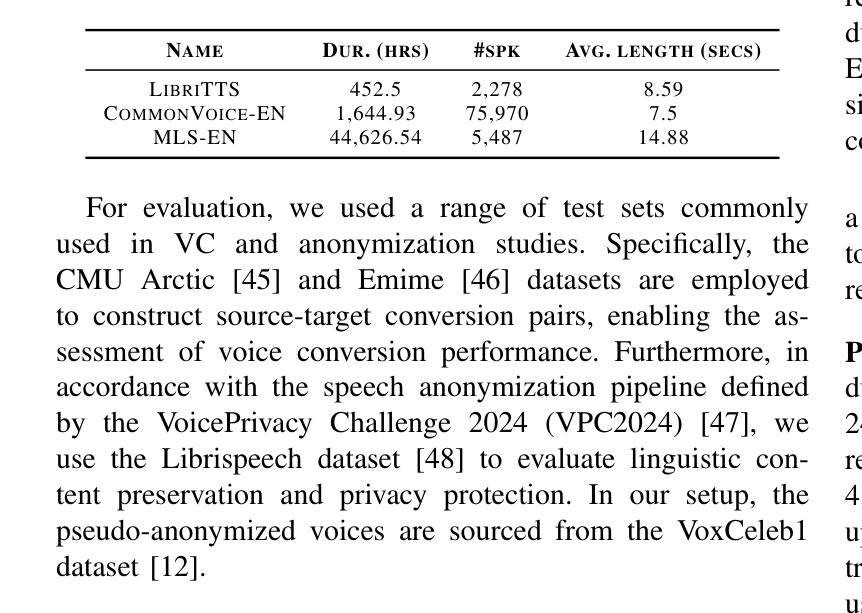
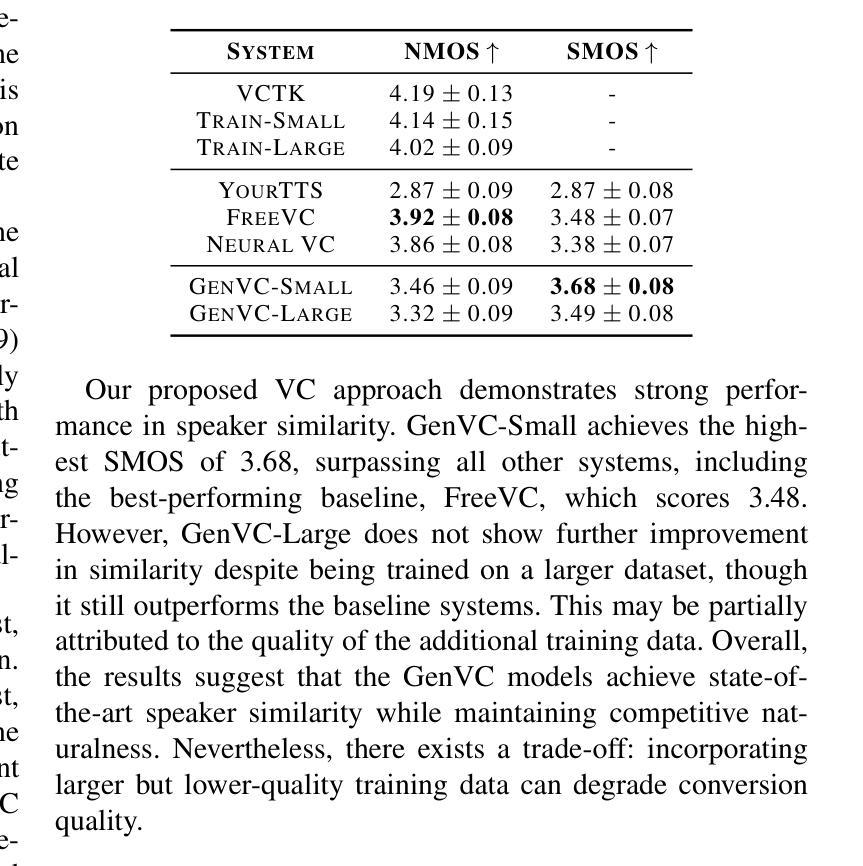
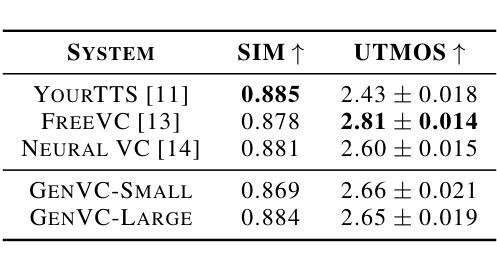