⚠️ 以下所有内容总结都来自于 大语言模型的能力,如有错误,仅供参考,谨慎使用
🔴 请注意:千万不要用于严肃的学术场景,只能用于论文阅读前的初筛!
💗 如果您觉得我们的项目对您有帮助 ChatPaperFree ,还请您给我们一些鼓励!⭐️ HuggingFace免费体验
2025-02-13 更新
PrismAvatar: Real-time animated 3D neural head avatars on edge devices
Authors:Prashant Raina, Felix Taubner, Mathieu Tuli, Eu Wern Teh, Kevin Ferreira
We present PrismAvatar: a 3D head avatar model which is designed specifically to enable real-time animation and rendering on resource-constrained edge devices, while still enjoying the benefits of neural volumetric rendering at training time. By integrating a rigged prism lattice with a 3D morphable head model, we use a hybrid rendering model to simultaneously reconstruct a mesh-based head and a deformable NeRF model for regions not represented by the 3DMM. We then distill the deformable NeRF into a rigged mesh and neural textures, which can be animated and rendered efficiently within the constraints of the traditional triangle rendering pipeline. In addition to running at 60 fps with low memory usage on mobile devices, we find that our trained models have comparable quality to state-of-the-art 3D avatar models on desktop devices.
我们推出了PrismAvatar:一款专门设计用于在资源受限的边缘设备上实现实时动画和渲染的3D头部虚拟模型。在训练期间,它仍然享有神经体积渲染的优势。通过将刚性棱镜格网与3D可变头部模型相结合,我们使用混合渲染模型同时重建基于网格的头部和未由3DMM表示的区域的变形NeRF模型。然后,我们将可变形NeRF蒸馏成刚性网格和神经纹理,这些纹理可以在传统的三角形渲染管道的限制内进行有效动画和渲染。除了在手机设备上以低内存使用率达到60帧每秒外,我们还发现我们的训练模型的质量与桌面设备上的最新3D虚拟模型的质量相当。
论文及项目相关链接
PDF 8 pages, 5 figures
Summary
PrismAvatar是一款专为边缘设备设计的实时动画和渲染的3D头像模型。它结合了可控制的棱柱网格与3D可变头部模型,使用混合渲染模型同时重建网格头部和可变NeRF模型以进行精细渲染。通过提炼可变形NeRF模型为可控制的网格和神经网络纹理,可在传统三角形渲染管道中高效动画和渲染。该模型在移动设备上以每秒60帧的速度运行,内存使用率低,并且质量在桌面设备上的效果堪比最新一代的3D头像模型。
Key Takeaways
- PrismAvatar是专为资源受限的边缘设备设计的实时动画和渲染的3D头像模型。
- 它结合了棱柱网格与3D可变头部模型,实现了高质量的渲染效果。
- 使用混合渲染模型进行精细渲染,同时重建网格头部和可变NeRF模型。
- 通过提炼可变形NeRF模型为可控制的网格和神经网络纹理,提高模型的效率和兼容性。
- 该模型可在移动设备上以每秒60帧的速度运行,具有较低的内存使用率。
- PrismAvatar的质量与桌面设备上的最新一代3D头像模型相当。
点此查看论文截图
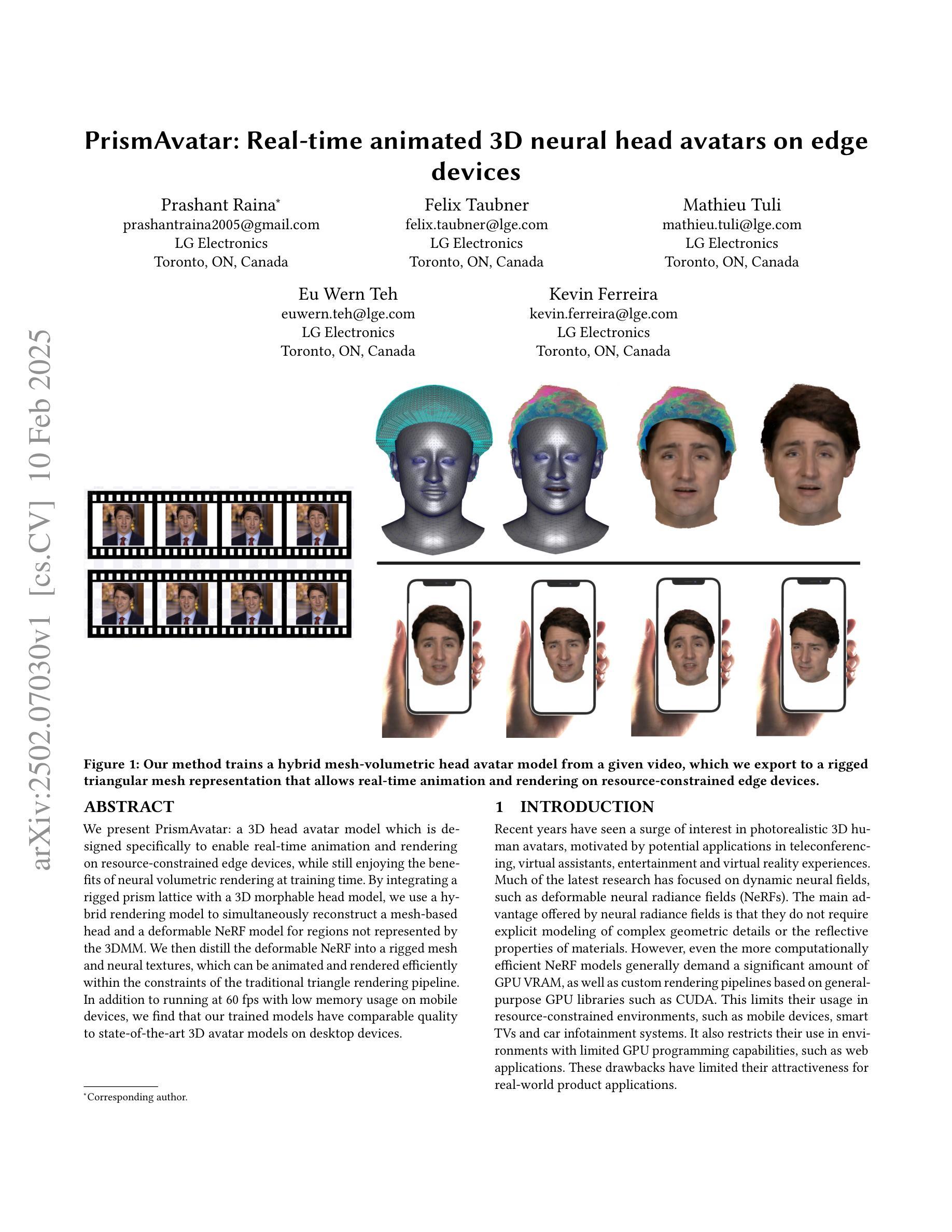
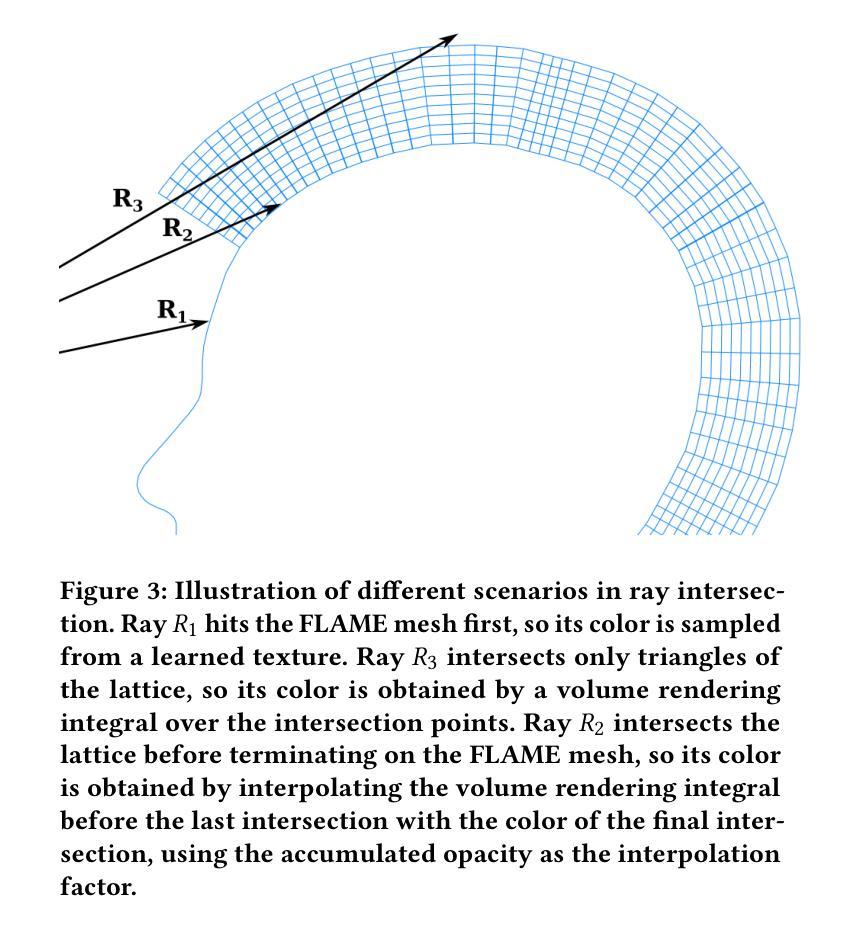
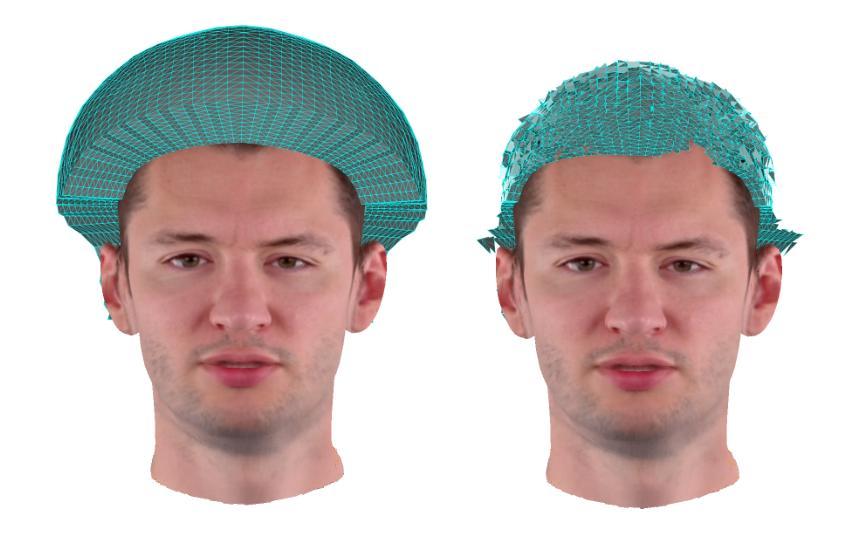
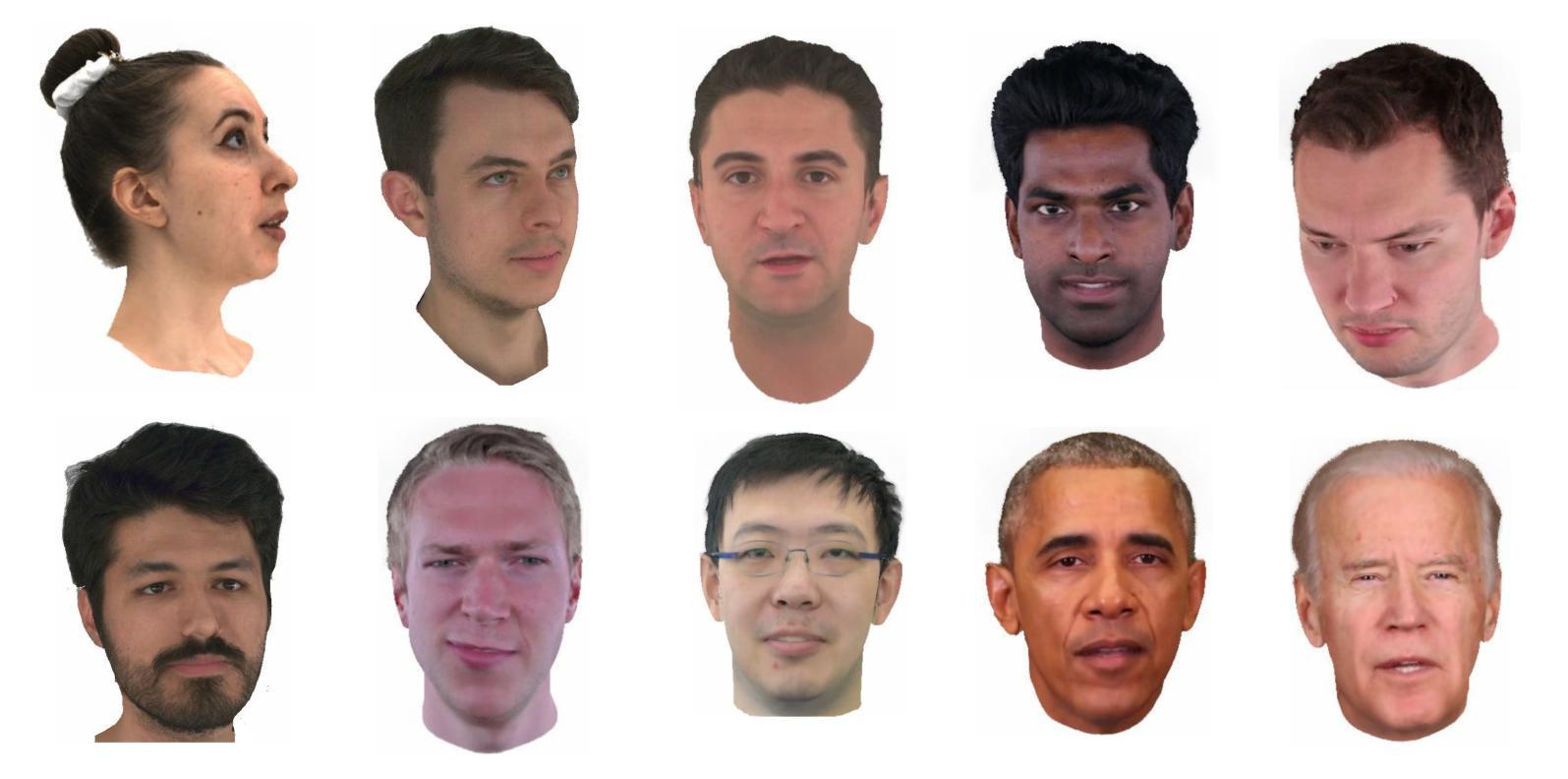
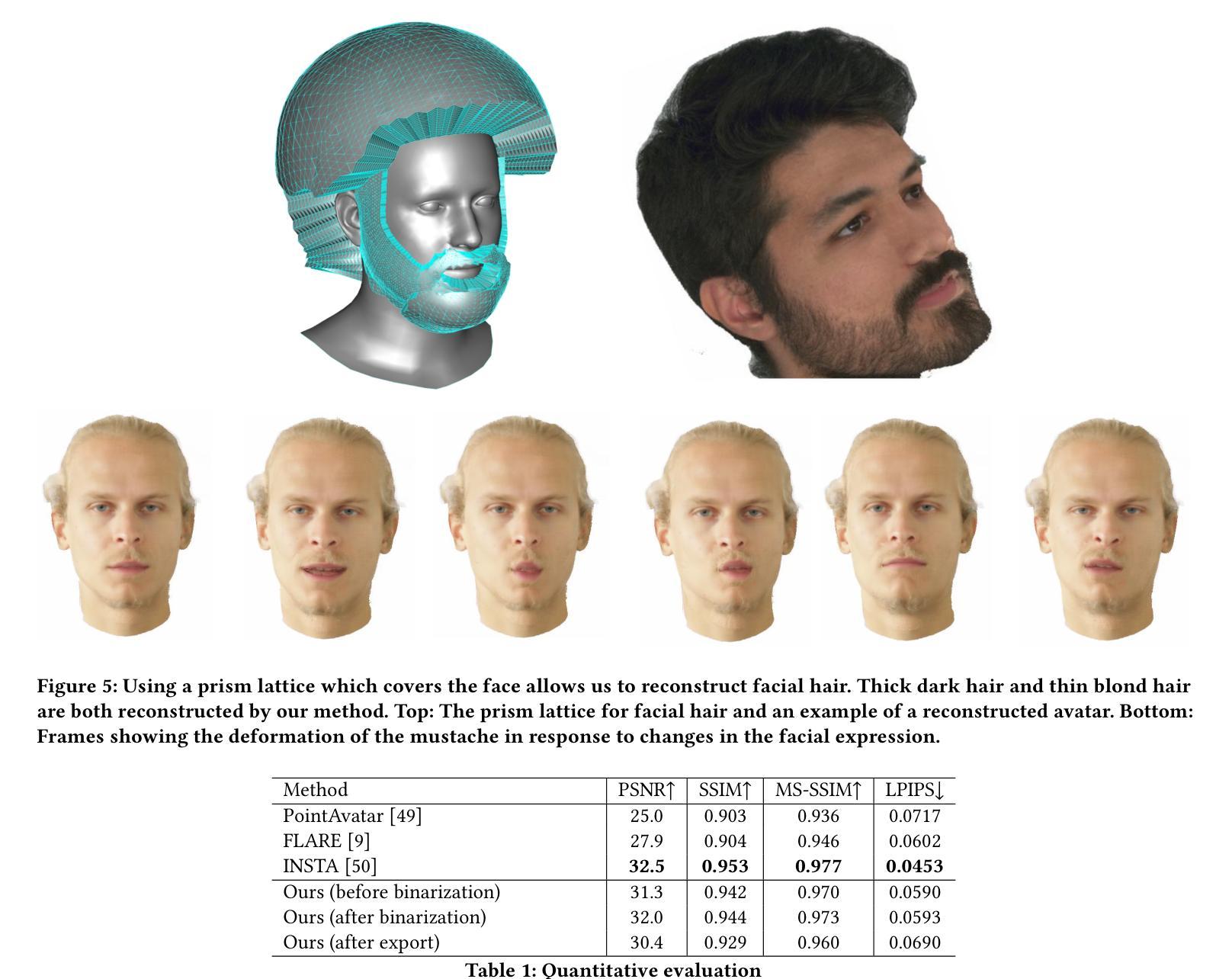
GAS: Generative Avatar Synthesis from a Single Image
Authors:Yixing Lu, Junting Dong, Youngjoong Kwon, Qin Zhao, Bo Dai, Fernando De la Torre
We introduce a generalizable and unified framework to synthesize view-consistent and temporally coherent avatars from a single image, addressing the challenging problem of single-image avatar generation. While recent methods employ diffusion models conditioned on human templates like depth or normal maps, they often struggle to preserve appearance information due to the discrepancy between sparse driving signals and the actual human subject, resulting in multi-view and temporal inconsistencies. Our approach bridges this gap by combining the reconstruction power of regression-based 3D human reconstruction with the generative capabilities of a diffusion model. The dense driving signal from the initial reconstructed human provides comprehensive conditioning, ensuring high-quality synthesis faithful to the reference appearance and structure. Additionally, we propose a unified framework that enables the generalization learned from novel pose synthesis on in-the-wild videos to naturally transfer to novel view synthesis. Our video-based diffusion model enhances disentangled synthesis with high-quality view-consistent renderings for novel views and realistic non-rigid deformations in novel pose animation. Results demonstrate the superior generalization ability of our method across in-domain and out-of-domain in-the-wild datasets. Project page: https://humansensinglab.github.io/GAS/
我们引入了一个通用且统一的框架,从单张图像合成视角一致和时间上连贯的化身,以解决单图像化身生成这一具有挑战性的问题。虽然最近的方法使用扩散模型,以深度或法线图等人类模板为条件,但由于稀疏的驱动信号与实际人类主体之间的差异,它们往往难以保留外观信息,导致多视角和时间上的不一致性。我们的方法通过结合基于回归的3D人体重建的重建能力与扩散模型的生成能力,缩小了这一差距。来自初始重建人体的密集驱动信号提供了全面的条件,确保了高质量合成忠于参考外观和结构。此外,我们提出了一个统一的框架,使从野生视频中的新姿态合成中学到的泛化能力能够自然地转移到新视角的合成中。我们的视频扩散模型增强了独立合成的功能,为新颖视角提供高质量视角一致渲染,以及新颖姿态动画中的真实非刚性变形。结果证明了我们的方法在内域和外域野生数据集上的卓越泛化能力。项目页面:https://humansensinglab.github.io/GAS/
论文及项目相关链接
Summary
本文介绍了一个通用且统一的框架,能够从单一图像中合成视角一致、时间连贯的虚拟人物,解决了单一图像生成虚拟人物的问题。该框架结合了回归式三维人体重建的重建能力与扩散模型的生成能力,通过初始重建的人体提供的密集驱动信号,确保合成的高质量且忠于参考外观和结构。此外,该框架还能将在新姿态合成中学到的知识推广到新的视角合成中,实现了高质量的视角一致渲染和逼真的非刚性变形。
Key Takeaways
- 引入了一个通用框架,能够从单一图像合成视角一致、时间连贯的虚拟人物。
- 结合回归式三维人体重建与扩散模型的生成能力。
- 初始重建的人体提供的密集驱动信号,确保合成的高质量且忠于参考。
- 框架能够推广新姿态合成中学到的知识到新的视角合成。
- 实现高质量的视角一致渲染和逼真的非刚性变形。
- 框架具有优越的在域内和域外野生数据集上的泛化能力。
点此查看论文截图
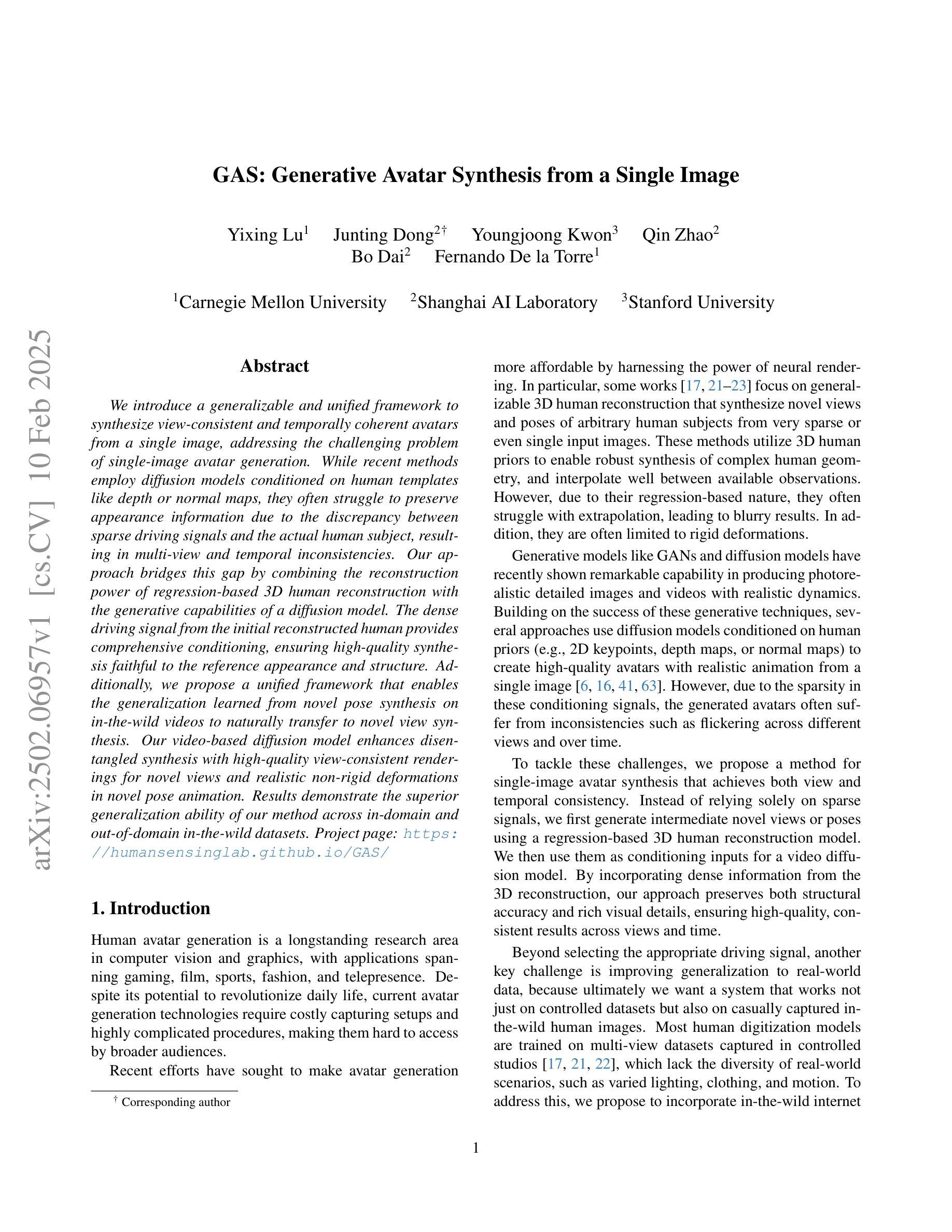
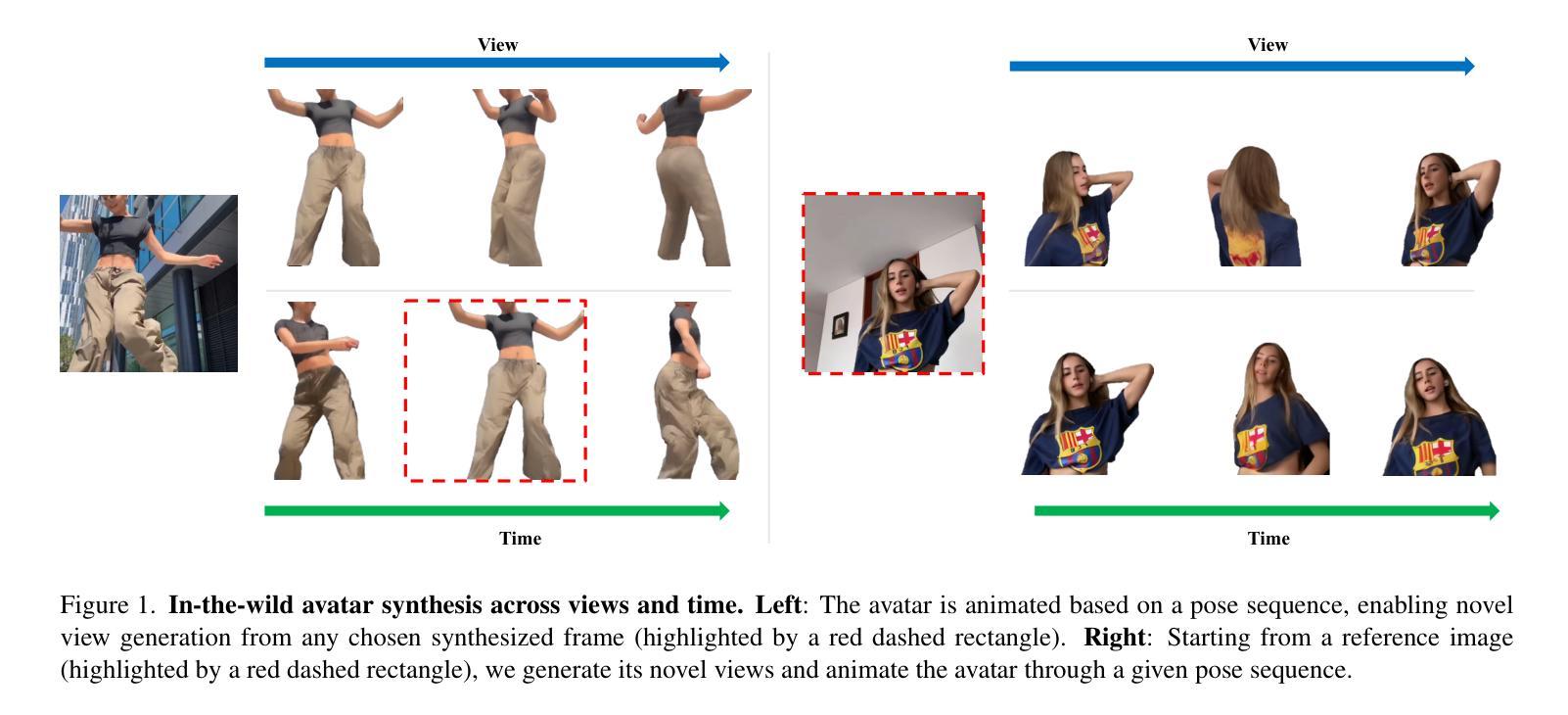
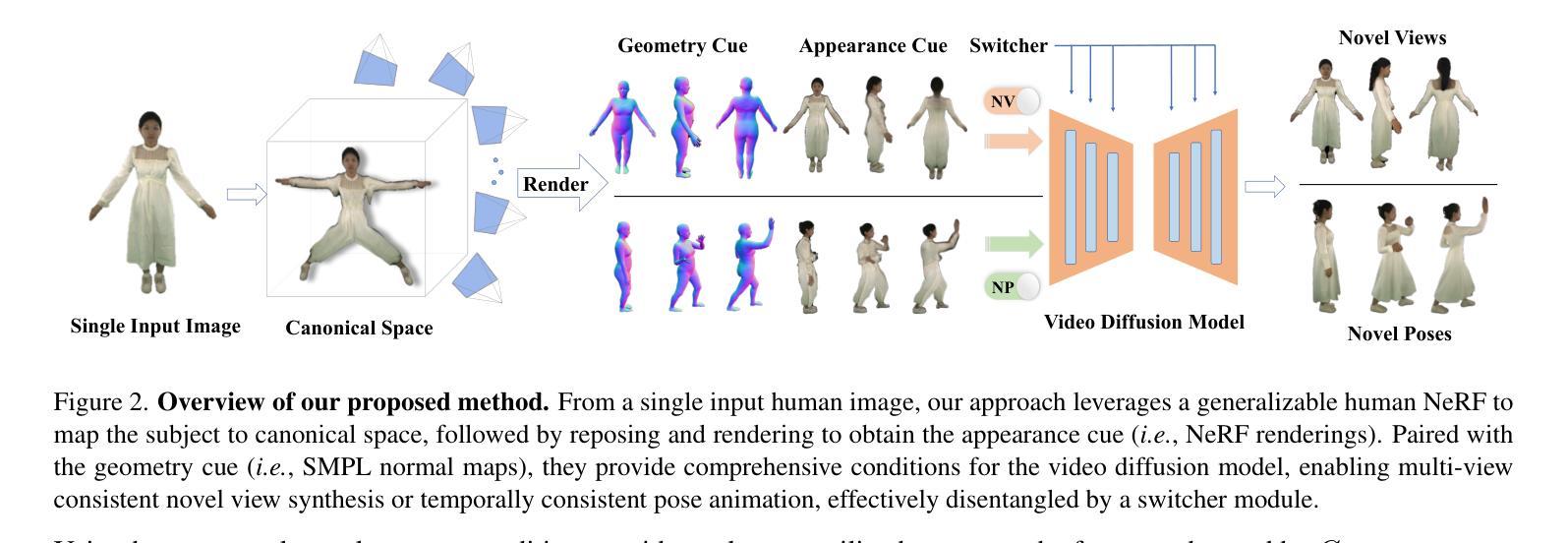
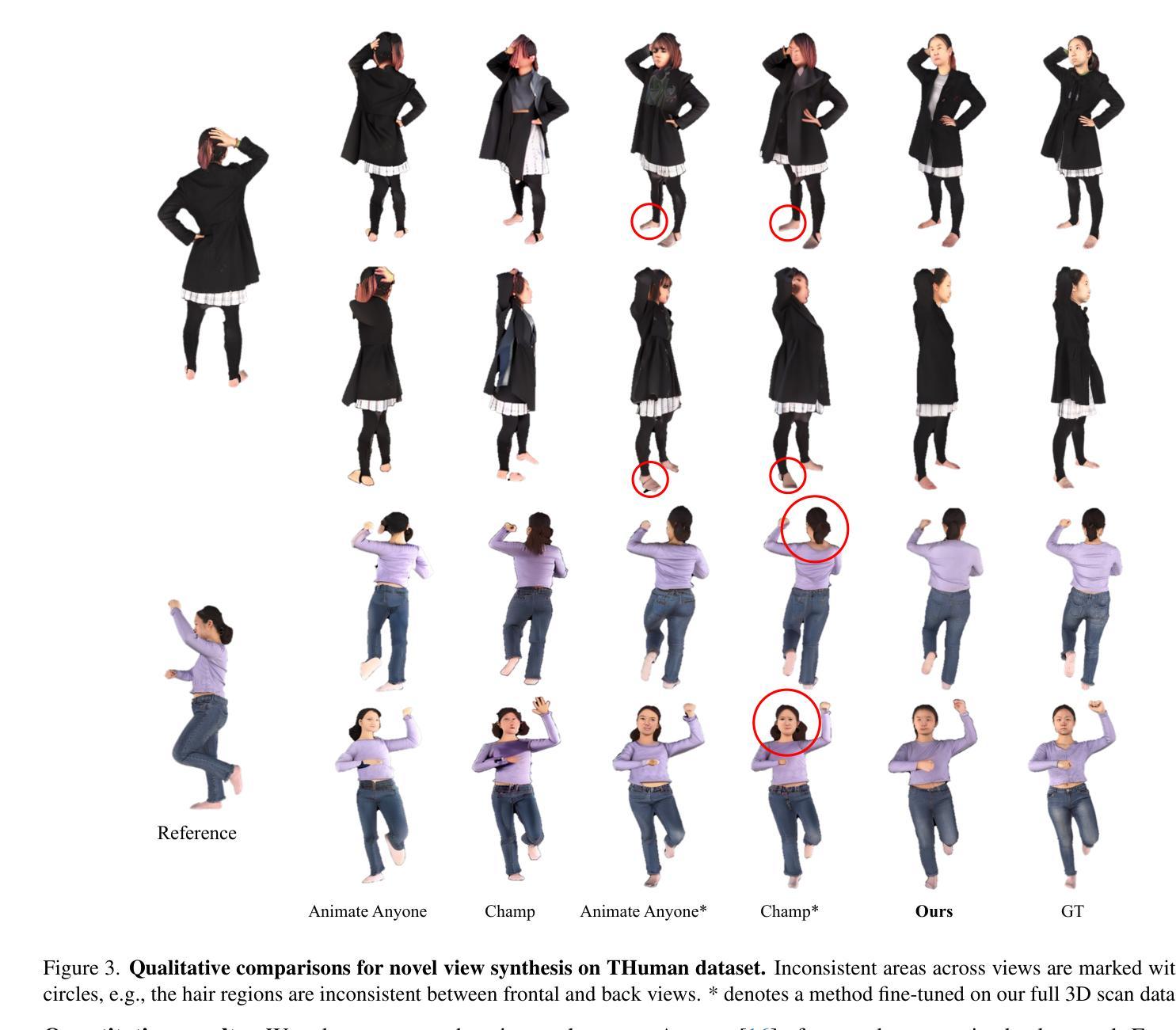
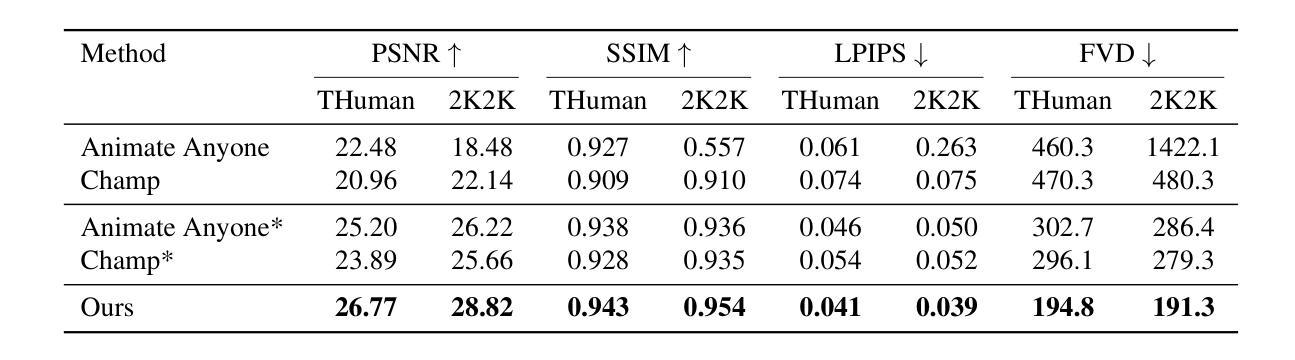
Drivable 3D Gaussian Avatars
Authors:Wojciech Zielonka, Timur Bagautdinov, Shunsuke Saito, Michael Zollhöfer, Justus Thies, Javier Romero
We present Drivable 3D Gaussian Avatars (D3GA), a multi-layered 3D controllable model for human bodies that utilizes 3D Gaussian primitives embedded into tetrahedral cages. The advantage of using cages compared to commonly employed linear blend skinning (LBS) is that primitives like 3D Gaussians are naturally re-oriented and their kernels are stretched via the deformation gradients of the encapsulating tetrahedron. Additional offsets are modeled for the tetrahedron vertices, effectively decoupling the low-dimensional driving poses from the extensive set of primitives to be rendered. This separation is achieved through the localized influence of each tetrahedron on 3D Gaussians, resulting in improved optimization. Using the cage-based deformation model, we introduce a compositional pipeline that decomposes an avatar into layers, such as garments, hands, or faces, improving the modeling of phenomena like garment sliding. These parts can be conditioned on different driving signals, such as keypoints for facial expressions or joint-angle vectors for garments and the body. Our experiments on two multi-view datasets with varied body shapes, clothes, and motions show higher-quality results. They surpass PSNR and SSIM metrics of other SOTA methods using the same data while offering greater flexibility and compactness.
我们提出了可驾驶的3D高斯化身(D3GA),这是一个多层次的可控制人体3D模型,它利用嵌入四面体笼中的3D高斯基本体。与通常使用的线性混合皮肤(LBS)相比,使用笼子的优点是,像3D高斯这样的基本体可以自然重新定向,并且其内核通过封装四面体的变形梯度进行拉伸。对四面体顶点进行了附加偏移建模,有效地将低维驱动姿势从要渲染的大量基本体中解耦出来。这种分离是通过每个四面体对3D高斯的局部影响实现的,从而实现了优化的改进。使用基于笼的变形模型,我们引入了一种成分管道,该管道将化身分解为层次结构,例如服装、手或脸,从而改善了衣物滑动等现象的建模。这些部分可以基于不同的驱动信号进行条件设置,例如面部表情的关键点或衣物和身体的关节角度向量。我们在两个具有不同身体形状、衣物和动作的多视角数据集上的实验表明,其结果质量更高。他们在使用相同数据的其他最新方法的峰值信噪比(PSNR)和结构相似性度量(SSIM)指标上表现更好,同时提供了更大的灵活性和紧凑性。
论文及项目相关链接
PDF Accepted to 3DV25 Website: https://zielon.github.io/d3ga/
Summary
本文介绍了基于三维高斯基础的Drivable 3D Gaussian Avatars(D3GA)模型。此模型使用嵌入四面体笼的三维高斯基本体,通过笼子的变形梯度自然调整高斯基本体的方向并拉伸其内核。此模型实现了低维驱动姿势与渲染的大量基本体之间的有效分离,提高了优化效果。实验表明,该模型在多种数据集上的表现优于其他先进方法,具有更高的质量和灵活性。
Key Takeaways
- Drivable 3D Gaussian Avatars (D3GA) 是一种利用三维高斯基本体嵌入四面体笼的多层三维可控人体模型。
- 与常用的线性混合皮肤技术相比,使用四面体笼的优势在于可以自然调整高斯基本体的方向和内核拉伸。
- 该模型实现了低维驱动姿势与大量渲染基本体之间的有效分离,通过每个四面体对三维高斯局部的影响,提高了优化效果。
- 该模型通过分解角色为多个层次(如服装、手、脸等)改善了现象建模,如服装滑动等。
- 这些部分可以根据不同的驱动信号进行调节,如面部表情的关键点或服装和身体的角度向量。
- 在多种数据集上的实验表明,该模型的表现在图像质量上超越了其他先进方法,体现在PSNR和SSIM指标上。
点此查看论文截图
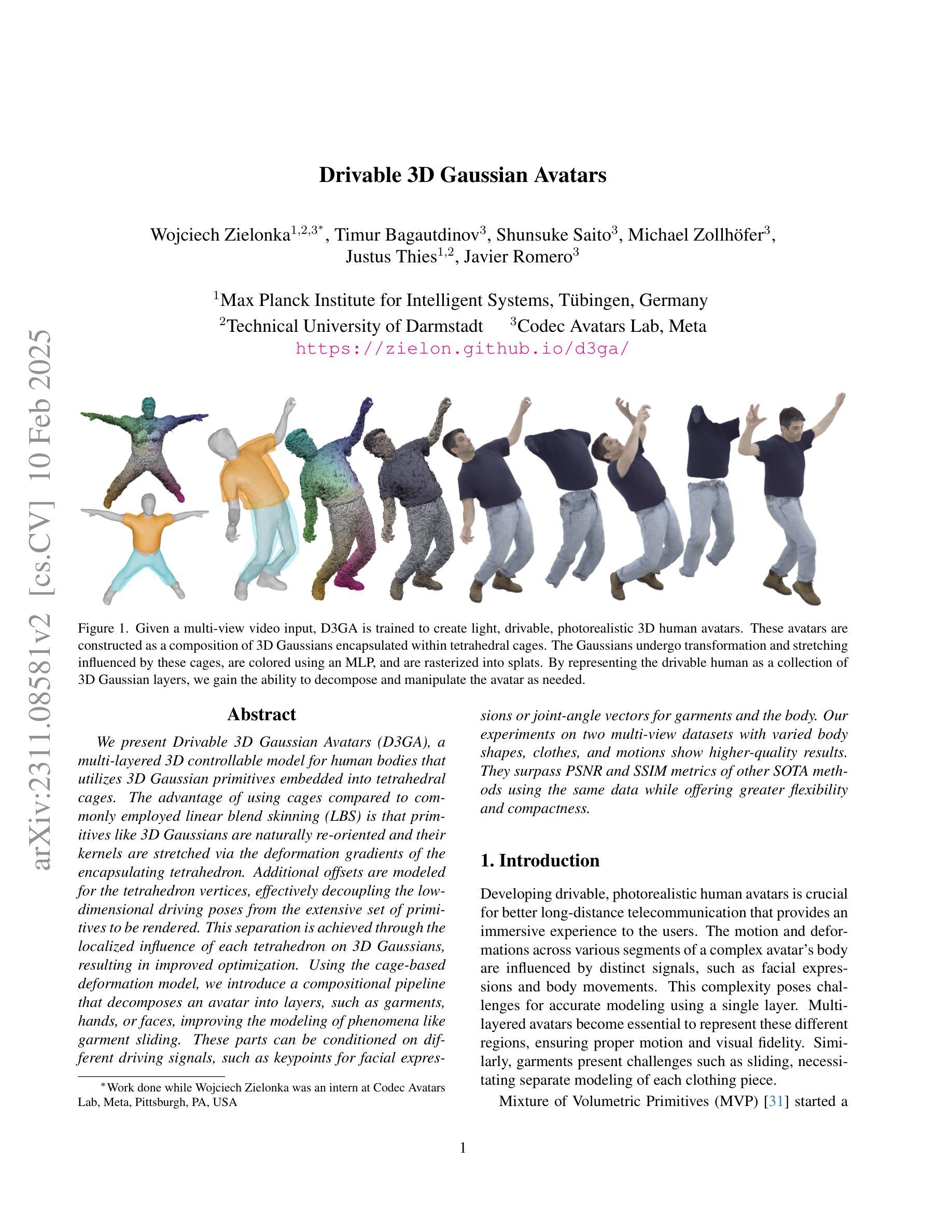
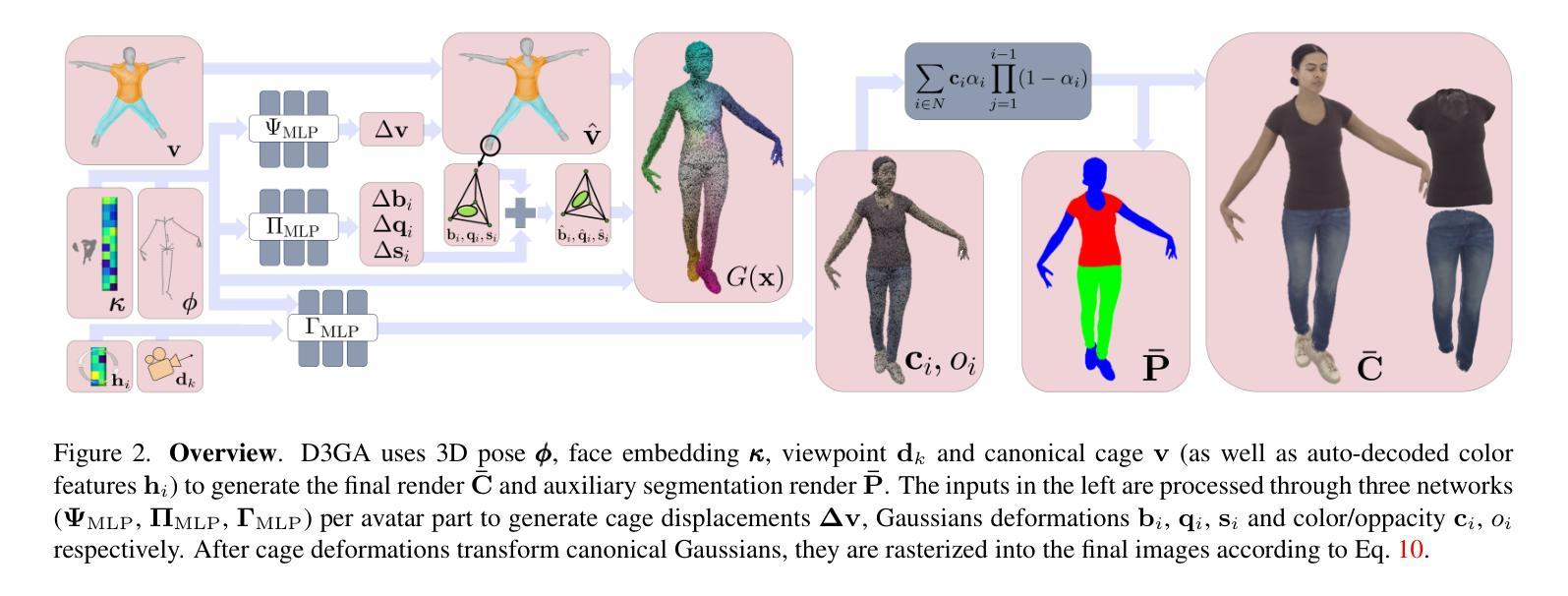
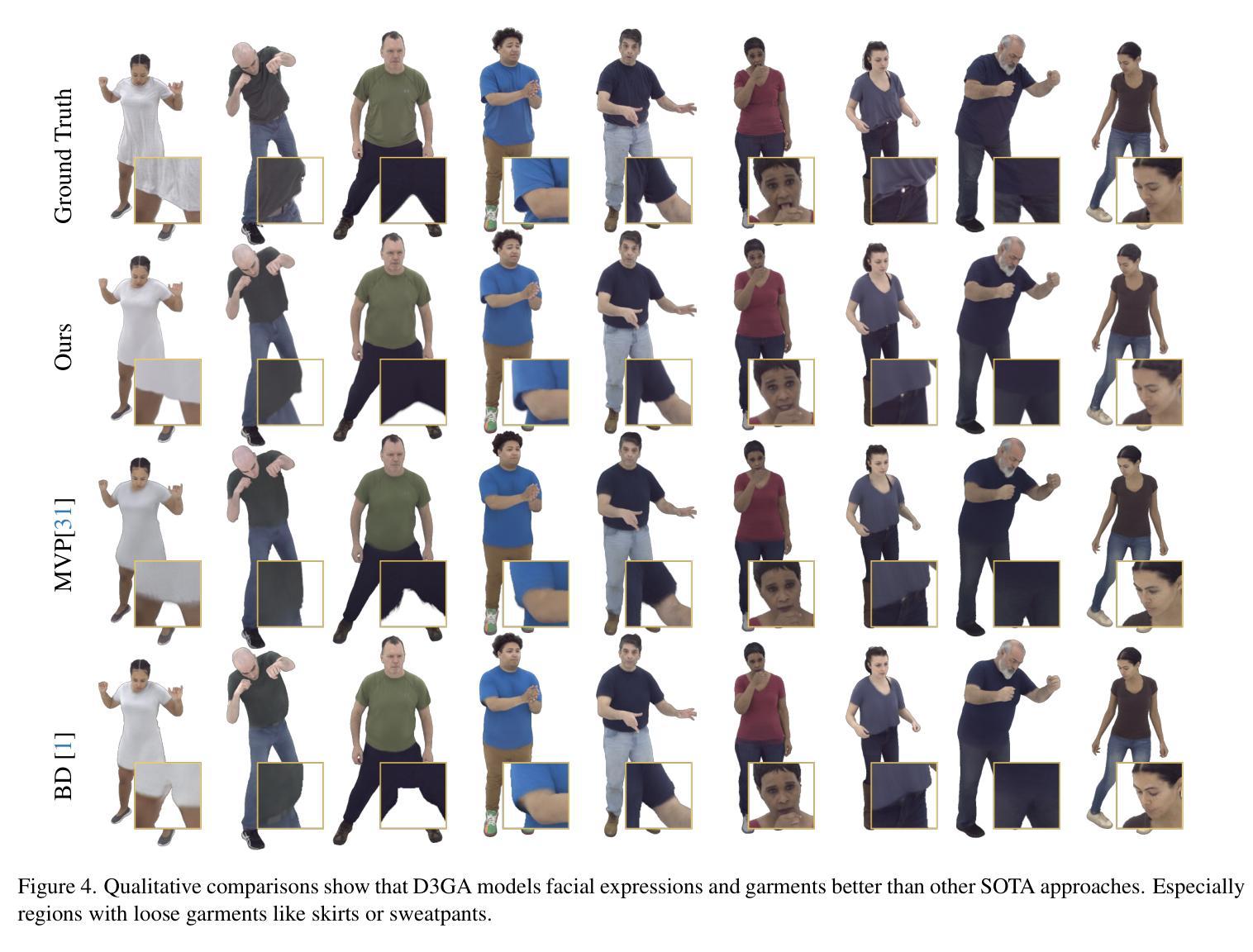