⚠️ 以下所有内容总结都来自于 大语言模型的能力,如有错误,仅供参考,谨慎使用
🔴 请注意:千万不要用于严肃的学术场景,只能用于论文阅读前的初筛!
💗 如果您觉得我们的项目对您有帮助 ChatPaperFree ,还请您给我们一些鼓励!⭐️ HuggingFace免费体验
2025-02-13 更新
Ultrasound Image Generation using Latent Diffusion Models
Authors:Benoit Freiche, Anthony El-Khoury, Ali Nasiri-Sarvi, Mahdi S. Hosseini, Damien Garcia, Adrian Basarab, Mathieu Boily, Hassan Rivaz
Diffusion models for image generation have been a subject of increasing interest due to their ability to generate diverse, high-quality images. Image generation has immense potential in medical imaging because open-source medical images are difficult to obtain compared to natural images, especially for rare conditions. The generated images can be used later to train classification and segmentation models. In this paper, we propose simulating realistic ultrasound (US) images by successive fine-tuning of large diffusion models on different publicly available databases. To do so, we fine-tuned Stable Diffusion, a state-of-the-art latent diffusion model, on BUSI (Breast US Images) an ultrasound breast image dataset. We successfully generated high-quality US images of the breast using simple prompts that specify the organ and pathology, which appeared realistic to three experienced US scientists and a US radiologist. Additionally, we provided user control by conditioning the model with segmentations through ControlNet. We will release the source code at http://code.sonography.ai/ to allow fast US image generation to the scientific community.
由于扩散模型能够生成多样化、高质量的图片,图像生成已成为了一个日益受到关注的研究领域。在医学成像领域,图像生成具有巨大的潜力,因为与自然图像相比,获取开源医学图像更为困难,尤其是对于罕见疾病的图像。生成的图像随后可用于训练和分割模型。在本文中,我们提出通过连续微调大型扩散模型来模拟真实的超声(US)图像,这些模型基于不同的公开数据库。为此,我们对最先进的潜在扩散模型Stable Diffusion进行了微调,针对BUSI(乳腺超声图像)数据库中的乳腺超声图像数据集。我们成功地使用简单的提示生成了高质量的乳腺超声图像,这些提示指定了器官和病理特征,对于三位经验丰富的超声科学家和一位超声放射科医生来说,这些生成的图像看起来非常逼真。此外,我们通过使用ControlNet对模型进行分段处理来提供用户控制。我们将在http://code.sonography.ai/发布源代码,以加快向科学界生成超声图像的速度。
论文及项目相关链接
PDF 6 pages conference paper for SPIE medical imaging
Summary
本文介绍了通过连续微调大型扩散模型在不同公开数据库上模拟真实超声(US)图像的方法。研究团队对先进的潜在扩散模型Stable Diffusion进行了微调,以适应超声乳腺图像数据集BUSI。通过简单的提示指定器官和病理,成功生成了高质量的乳腺超声图像,被三位经验丰富的超声科学家和一位超声放射科医生认为是真实的。此外,通过ControlNet对模型进行分段控制,提供了用户控制。相关源代码将发布在http://code.sonography.ai/上,以供科学界快速生成超声图像。
Key Takeaways
- 扩散模型在生成多样、高质量图像方面受到越来越多的关注。
- 在医学成像领域,图像生成具有巨大潜力,尤其对于难以获取自然图像的开源医学图像。
- 本文提出了通过连续微调大型扩散模型在公开数据库上模拟真实超声(US)图像的方法。
- 研究团队成功微调了Stable Diffusion模型,适应了超声乳腺图像数据集BUSI。
- 能够通过简单的提示生成高质量的乳腺超声图像,并被专业人士认为是真实的。
- 通过ControlNet提供了用户控制模型的条件。
点此查看论文截图
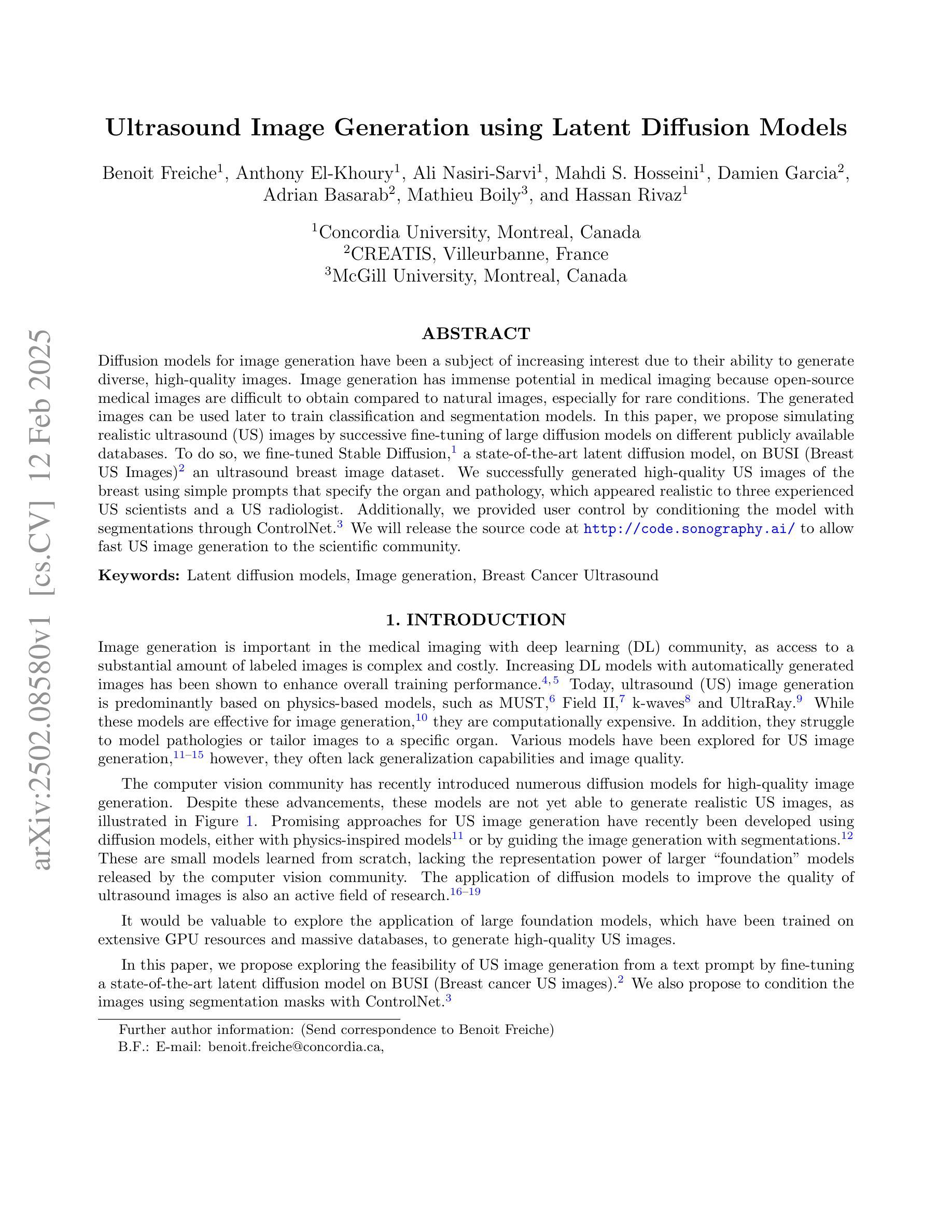
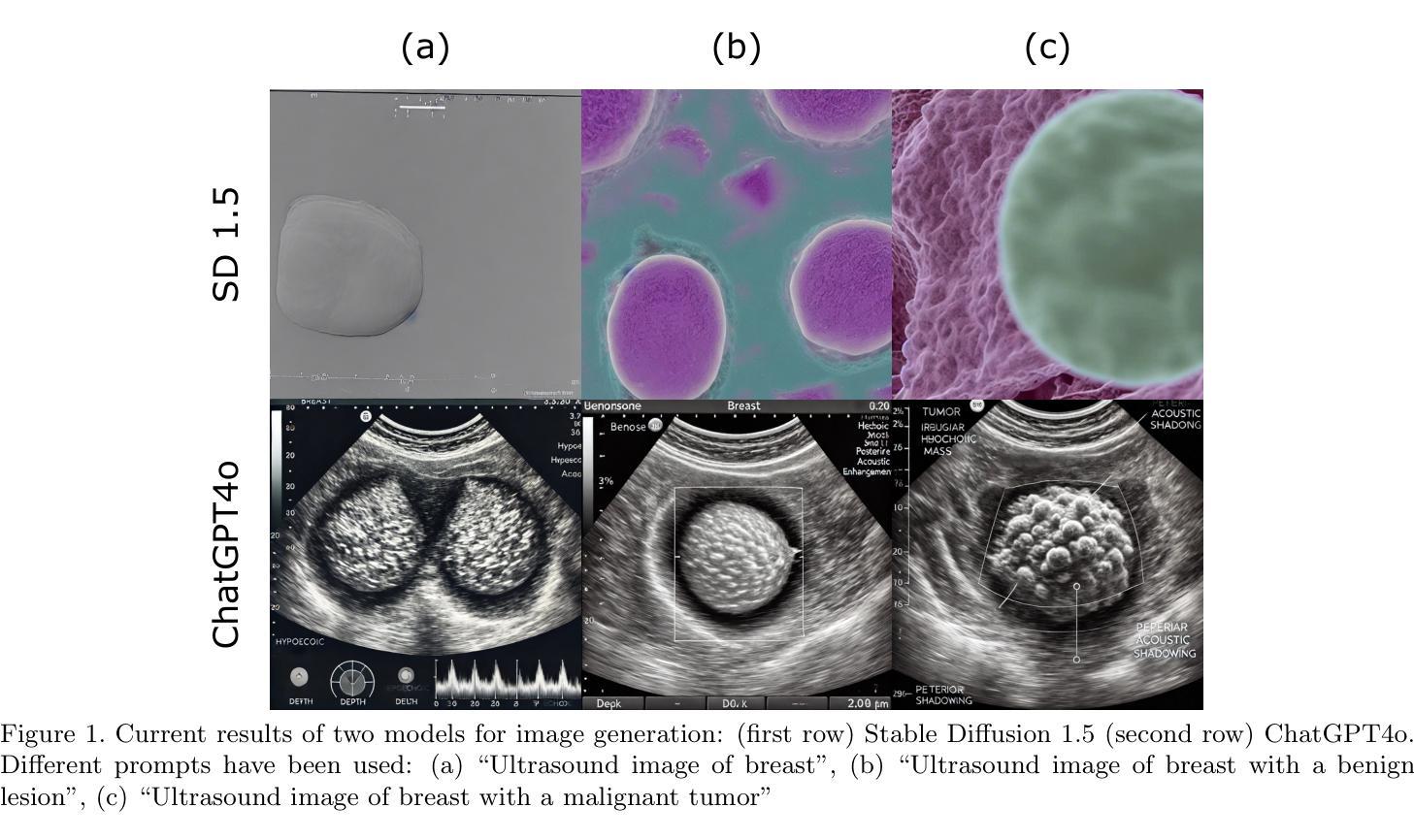
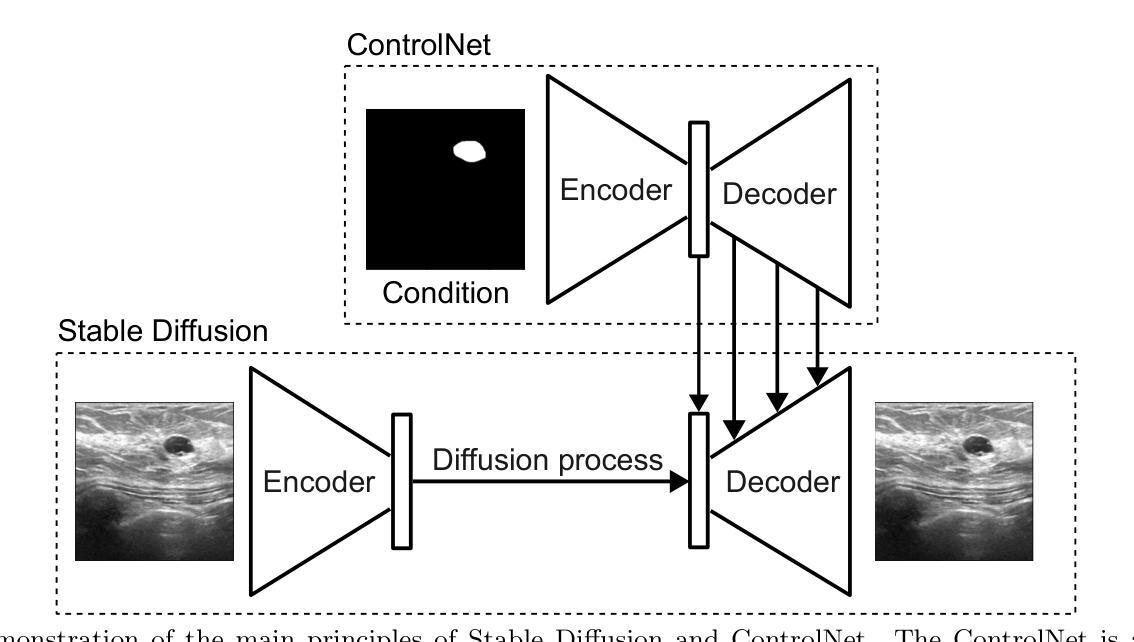
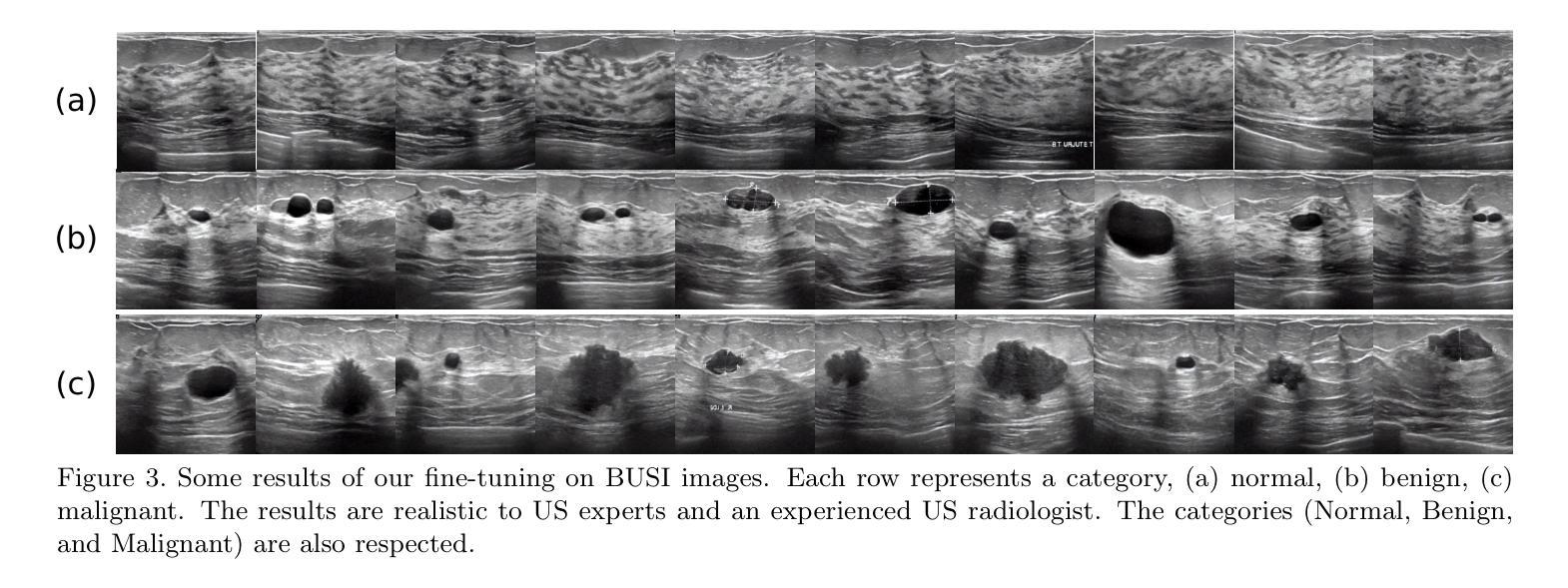
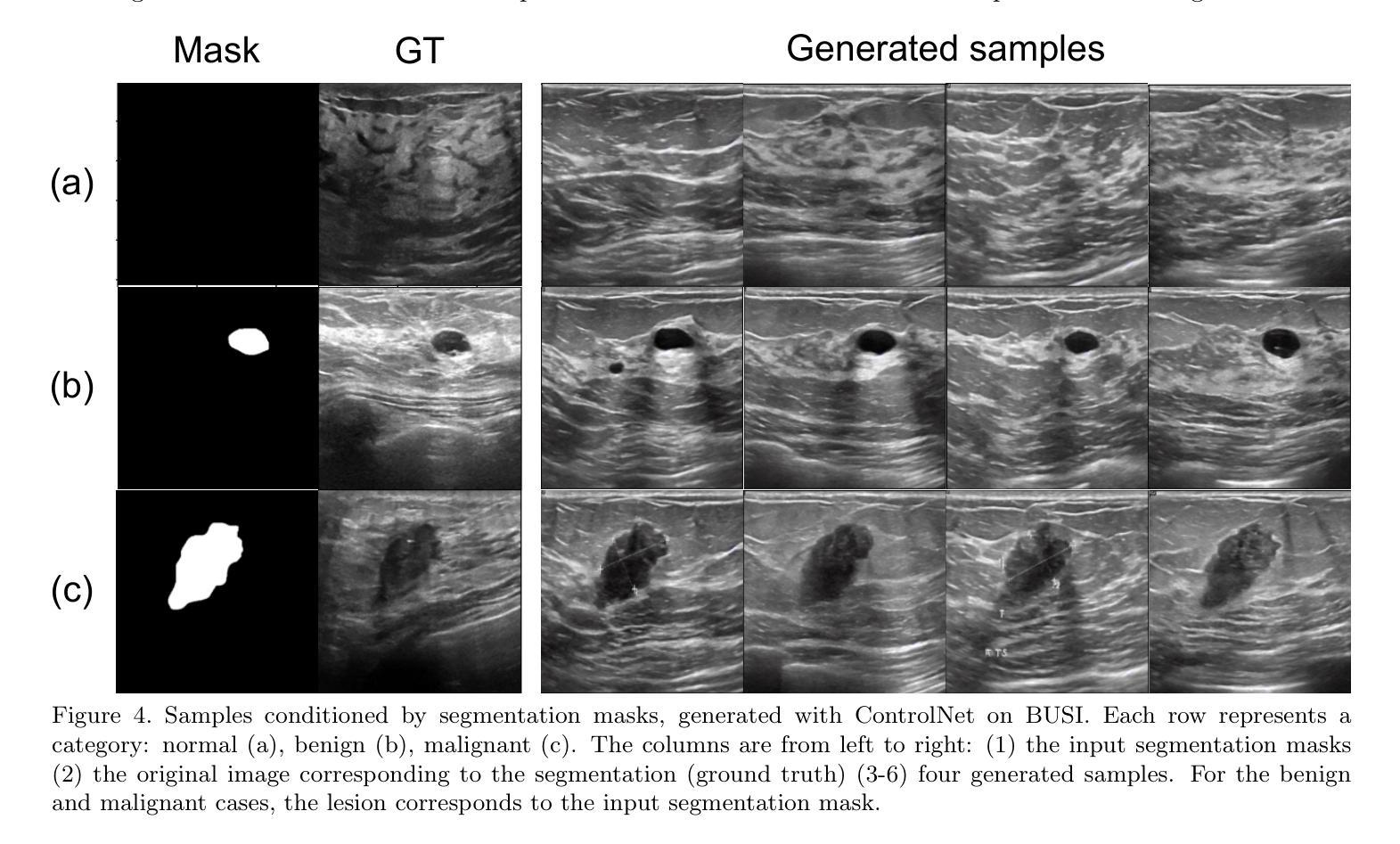