⚠️ 以下所有内容总结都来自于 大语言模型的能力,如有错误,仅供参考,谨慎使用
🔴 请注意:千万不要用于严肃的学术场景,只能用于论文阅读前的初筛!
💗 如果您觉得我们的项目对您有帮助 ChatPaperFree ,还请您给我们一些鼓励!⭐️ HuggingFace免费体验
2025-02-13 更新
BF-GAN: Development of an AI-driven Bubbly Flow Image Generation Model Using Generative Adversarial Networks
Authors:Wen Zhou, Shuichiro Miwa, Yang Liu, Koji Okamoto
A generative AI architecture called bubbly flow generative adversarial networks (BF-GAN) is developed, designed to generate realistic and high-quality bubbly flow images through physically conditioned inputs, jg and jf. Initially, 52 sets of bubbly flow experiments under varying conditions are conducted to collect 140,000 bubbly flow images with physical labels of jg and jf for training data. A multi-scale loss function is then developed, incorporating mismatch loss and pixel loss to enhance the generative performance of BF-GAN further. Regarding evaluative metrics of generative AI, the BF-GAN has surpassed conventional GAN. Physically, key parameters of bubbly flow generated by BF-GAN are extracted and compared with measurement values and empirical correlations, validating BF-GAN’s generative performance. The comparative analysis demonstrate that the BF-GAN can generate realistic and high-quality bubbly flow images with any given jg and jf within the research scope. BF-GAN offers a generative AI solution for two-phase flow research, substantially lowering the time and cost required to obtain high-quality data. In addition, it can function as a benchmark dataset generator for bubbly flow detection and segmentation algorithms, enhancing overall productivity in this research domain. The BF-GAN model is available online (https://github.com/zhouzhouwen/BF-GAN).
提出了一种称为冒泡流生成对抗网络(BF-GAN)的生成式人工智能架构,该架构旨在通过物理条件输入jg和jf生成逼真的高质量冒泡流图像。最初,在不同条件下进行了52组冒泡流实验,收集了14万张带有jg和jf物理标签的冒泡流图像作为训练数据。然后开发了一种多尺度损失函数,结合了不匹配损失和像素损失,以进一步提高BF-GAN的生成性能。在生成式人工智能的评估指标方面,BF-GAN的表现超越了传统GAN。从物理角度对BF-GAN生成的冒泡流的关键参数进行了提取,并与测量值和经验相关性进行了比较,验证了BF-GAN的生成性能。对比分析表明,BF-GAN在研究的范围内能够生成给定任何jg和jf的逼真且高质量的冒泡流图像。BF-GAN为两相流研究提供了生成式人工智能解决方案,大大降低了获取高质量数据所需的时间和成本。此外,它还可以作为冒泡流检测和分割算法的基准数据集生成器,提高该研究领域整体的生产力。BF-GAN模型可在网上找到(https://github.com/zhouzhouwen/BF-GAN)。
论文及项目相关链接
Summary
BF-GAN是一种生成式人工智能架构,用于通过物理条件输入生成逼真的高质量的气泡流动图像。通过进行气泡流动实验收集训练数据,并开发多尺度损失函数以提高BF-GAN的生成性能。BF-GAN在生成气泡流动图像方面的性能超过了传统GAN,可作为两相流研究的高效数据生成解决方案,降低获取高质量数据的时间和成本,也可作为气泡流动检测和分割算法的基准数据集生成器。
Key Takeaways
- BF-GAN是一种生成式人工智能架构,旨在通过物理条件输入生成高质量的气泡流动图像。
- 通过实验收集了用于训练BF-GAN的气泡流动图像数据。
- 开发了一种多尺度损失函数,以提高BF-GAN的生成性能。
- BF-GAN的性能超过了传统GAN在生成气泡流动图像方面的性能。
- BF-GAN可作为两相流研究的高效数据生成解决方案,降低了获取高质量数据的时间和成本。
- BF-GAN可以作为一个基准数据集生成器,用于气泡流动检测和分割算法的开发。
点此查看论文截图
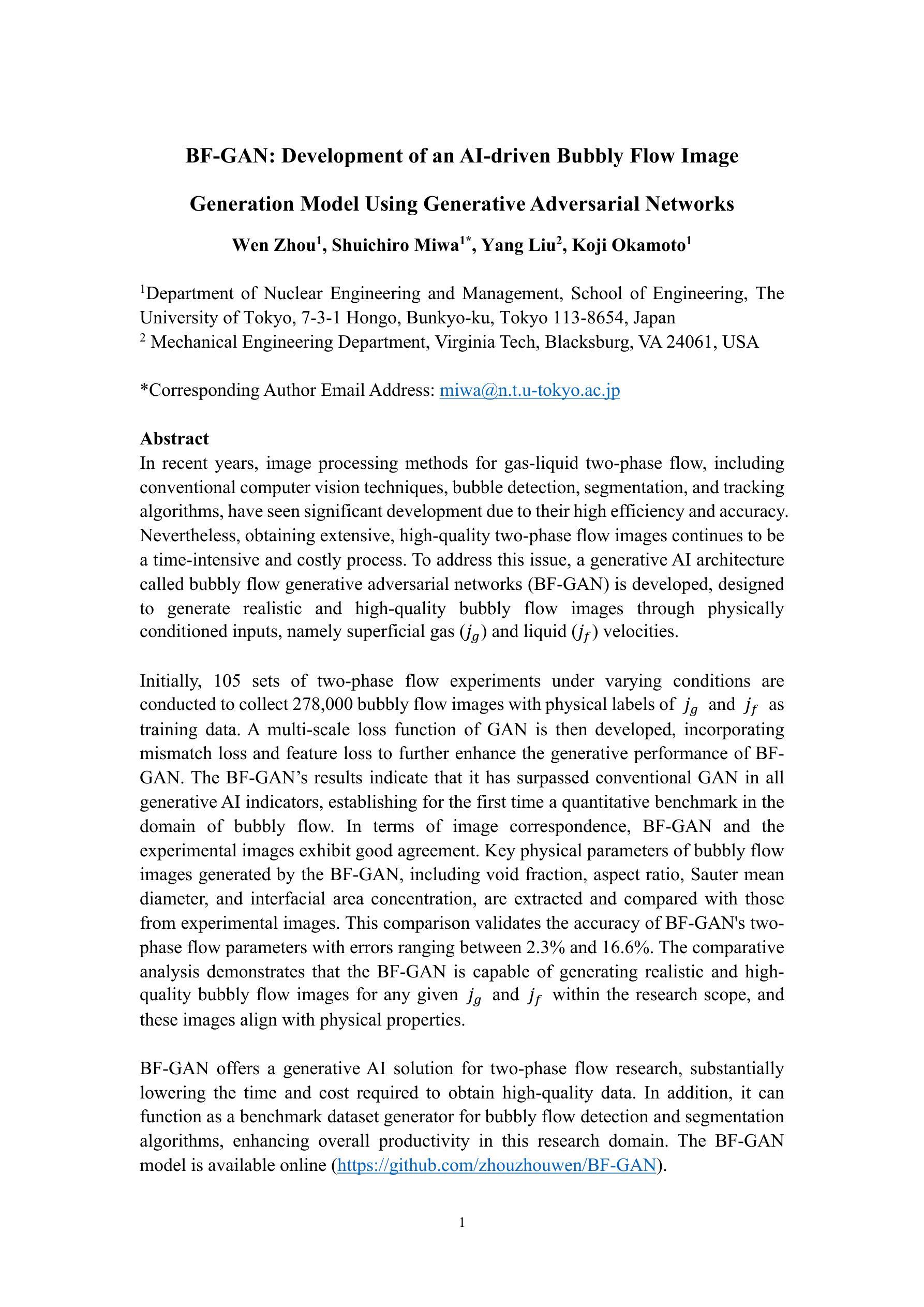
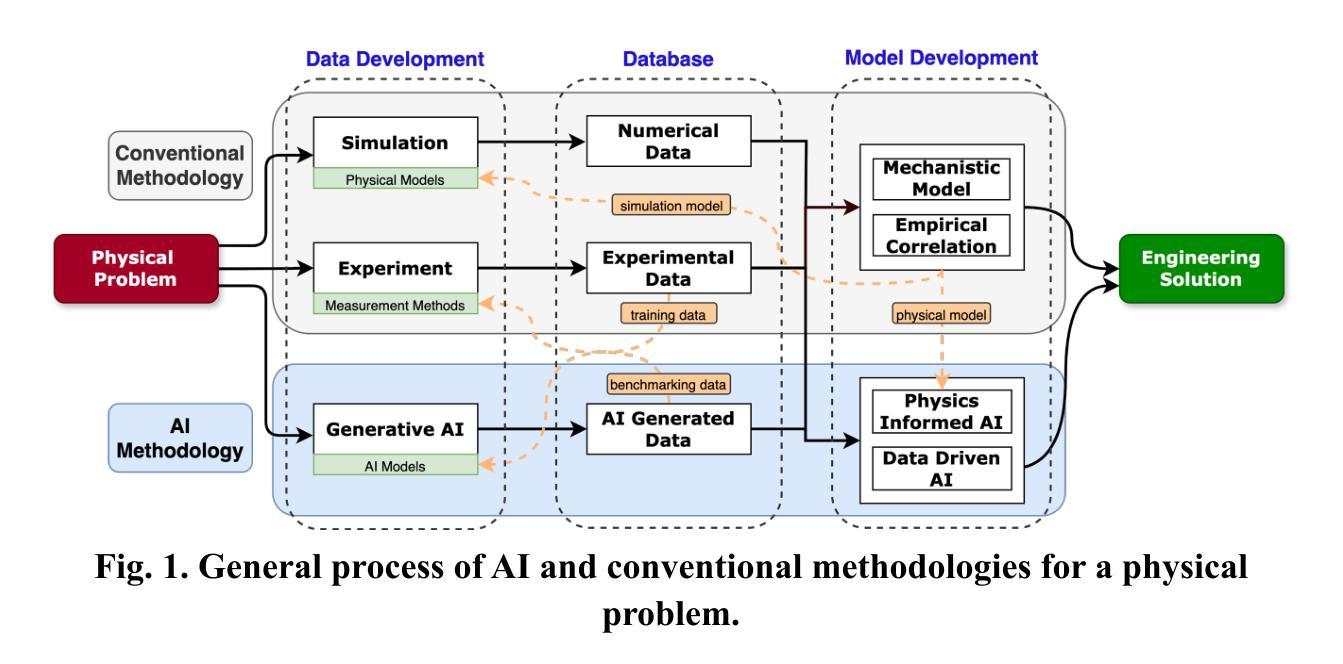
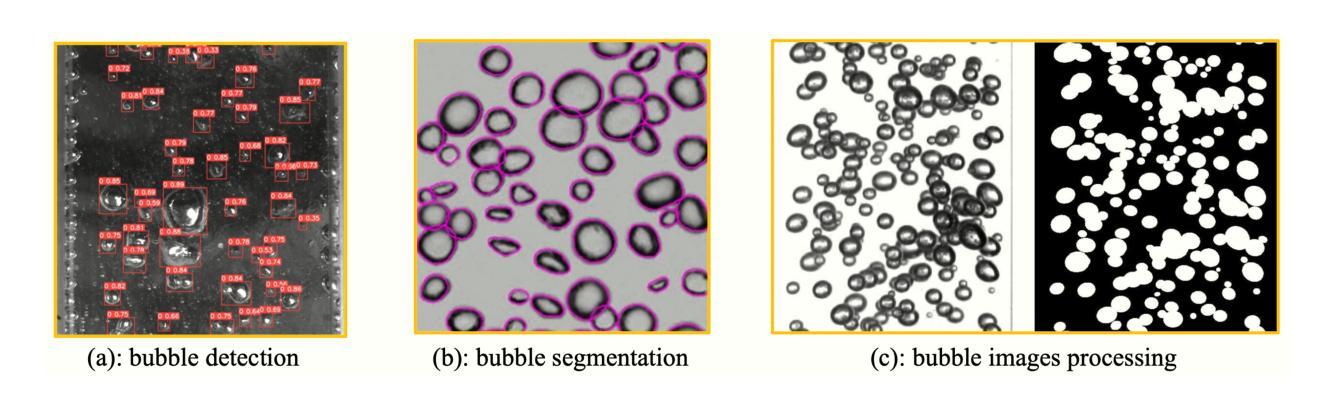
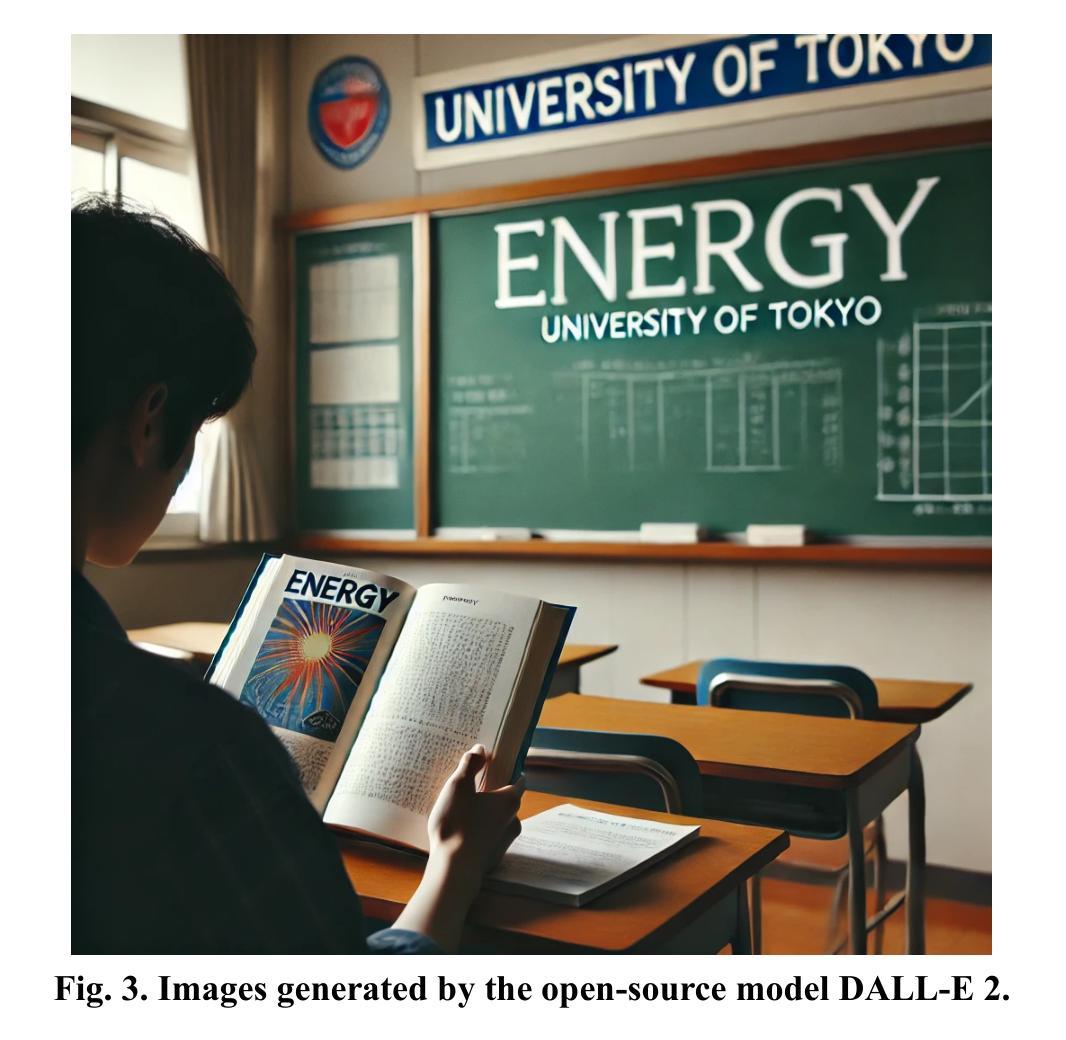
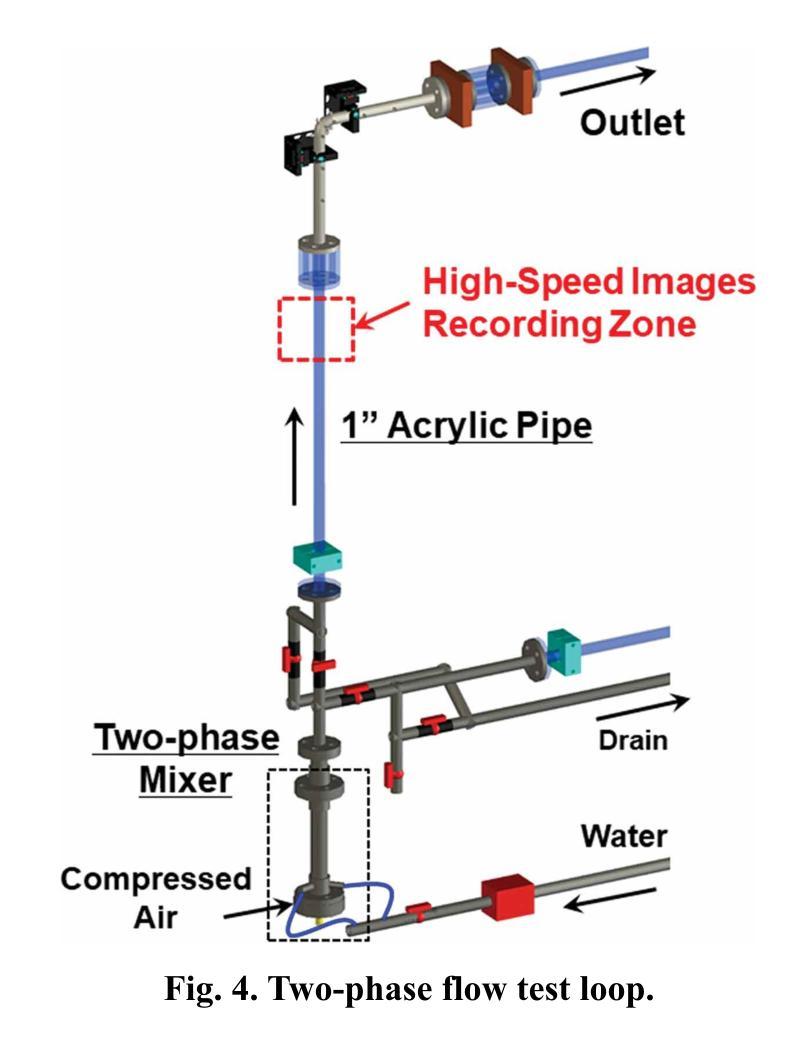
ViSIR: Vision Transformer Single Image Reconstruction Method for Earth System Models
Authors:Ehsan Zeraatkar, Salah Faroughi, Jelena Tešić
Purpose: Earth system models (ESMs) integrate the interactions of the atmosphere, ocean, land, ice, and biosphere to estimate the state of regional and global climate under a wide variety of conditions. The ESMs are highly complex, and thus, deep neural network architectures are used to model the complexity and store the down-sampled data. In this paper, we propose the Vision Transformer Sinusoidal Representation Networks (ViSIR) to improve the single image SR (SR) reconstruction task for the ESM data. Methods: ViSIR combines the SR capability of Vision Transformers (ViT) with the high-frequency detail preservation of the Sinusoidal Representation Network (SIREN) to address the spectral bias observed in SR tasks. Results: The ViSIR outperforms ViT by 4.1 dB, SIREN by 7.5 dB, and SR-Generative Adversarial (SR-GANs) by 7.1dB PSNR on average for three different measurements. Conclusion: The proposed ViSIR is evaluated and compared with state-of-the-art methods. The results show that the proposed algorithm is outperforming other methods in terms of Mean Square Error(MSE), Peak-Signal-to-Noise-Ratio(PSNR), and Structural Similarity Index Measure(SSIM).
目的:地球系统模型(ESMs)整合了大气、海洋、陆地、冰层和生物圈的相互作用,以在多种条件下估计区域和全球气候的状态。由于ESMs高度复杂,因此使用深度神经网络架构来对其复杂性进行建模并存储降采样数据。在本文中,我们提出了Vision Transformer Sinusoidal Representation Networks(ViSIR),以改进ESM数据的单图像超分辨率(SR)重建任务。
方法:ViSIR结合了Vision Transformers(ViT)的超分辨率能力和Sinusoidal Representation Network(SIREN)的高频细节保留能力,以解决SR任务中观察到的光谱偏置。
结果:ViSIR在三个不同测量上的平均PSNR值比ViT高出4.1 dB,比SIREN高出7.5 dB,比SR-生成对抗网络(SR-GANs)高出7.1 dB。
论文及项目相关链接
Summary:本文提出了Vision Transformer Sinusoidal Representation Networks(ViSIR),结合了Vision Transformers(ViT)的超分辨率(SR)能力和Sinusoidal Representation Network(SIREN)的高频细节保留功能,改进了地球系统模型数据的单图像超分辨率重建任务,并在三项不同测量中平均超越了其他方法。
Key Takeaways:
- ESMs(地球系统模型)整合多个系统的交互以预测不同条件下的区域和全球气候状态。
- ESM数据处理的复杂性需要使用深度神经网络架构进行建模。
- ViSIR结合了ViT的SR能力和SIREN的高频细节保留功能,解决了SR任务中的光谱偏置问题。
- ViSIR在PSNR上平均比ViT、SIREN和SR-GANs高出4.1dB、7.5dB和7.1dB。
- ViSIR在MSE、PSNR和SSIM等指标上均表现出超越其他方法的性能。
- ViSIR对于处理ESM数据的单图像超分辨率重建任务具有显著优势。
点此查看论文截图
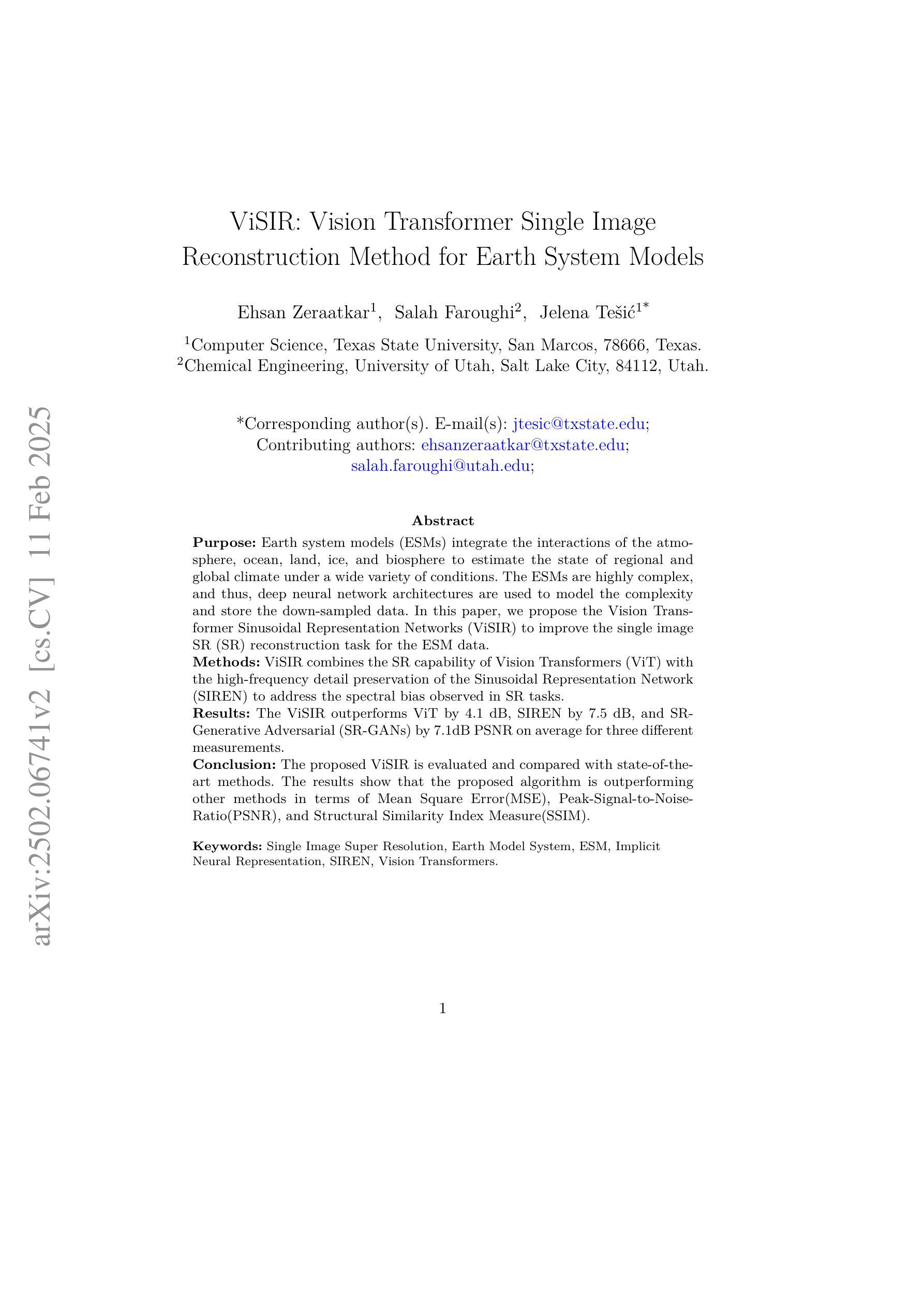
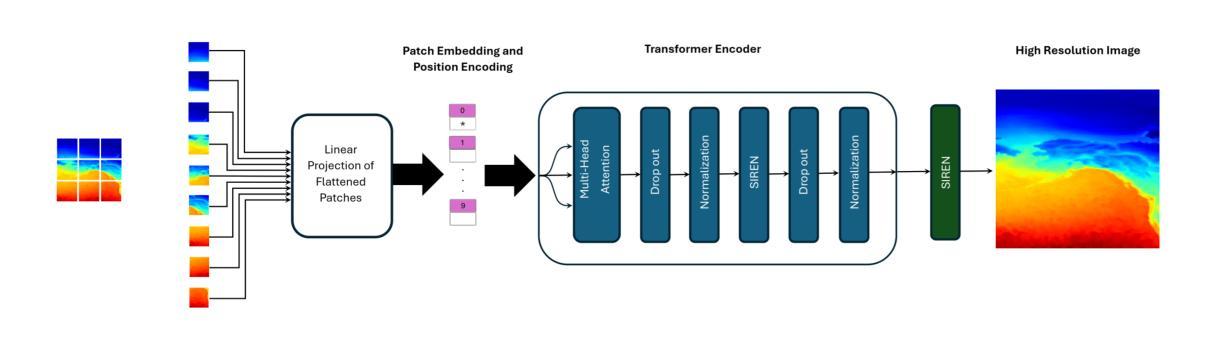
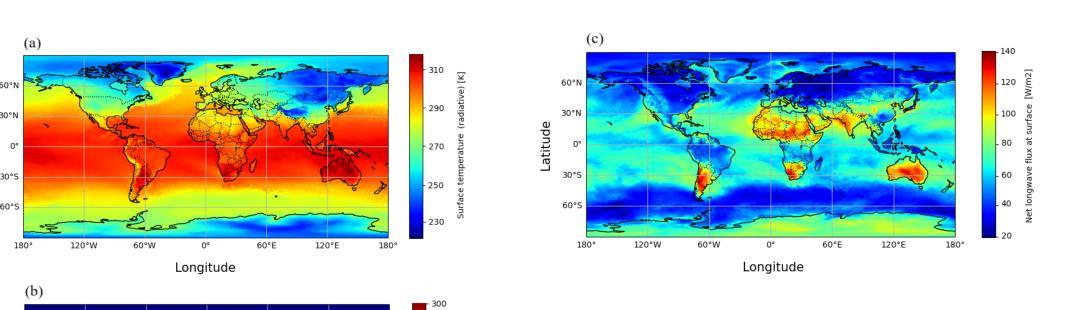
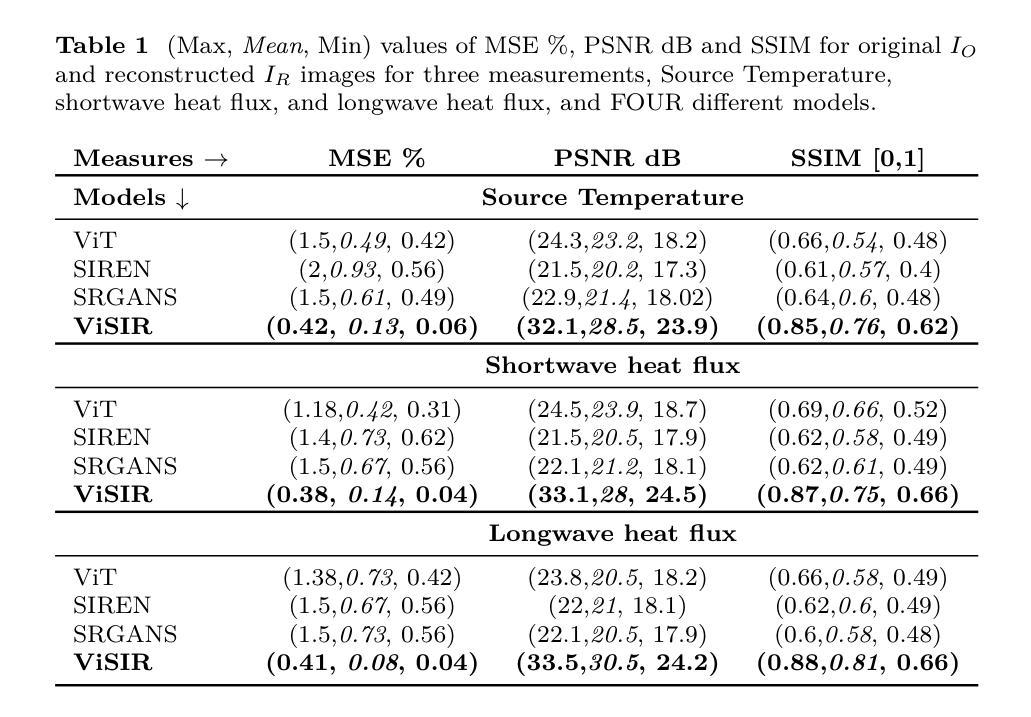
Physics-augmented Deep Learning with Adversarial Domain Adaptation: Applications to STM Image Denoising
Authors:Jianxin Xie, Wonhee Ko, Rui-Xing Zhang, Bing Yao
Image denoising is a critical task in various scientific fields such as medical imaging and material characterization, where the accurate recovery of underlying structures from noisy data is essential. Although supervised denoising techniques have achieved significant advancements, they typically require large datasets of paired clean-noisy images for training. Unsupervised methods, while not reliant on paired data, typically necessitate a set of unpaired clean images for training, which are not always accessible. In this paper, we propose a physics-augmented deep learning with adversarial domain adaption (PDA-Net) framework for unsupervised image denoising, with applications to denoise real-world scanning tunneling microscopy (STM) images. Our PDA-Net leverages the underlying physics to simulate and envision the ground truth for denoised STM images. Additionally, built upon Generative Adversarial Networks (GANs), we incorporate a cycle-consistency module and a domain adversarial module into our PDA-Net to address the challenge of lacking paired training data and achieve information transfer between the simulated and real experimental domains. Finally, we propose to implement feature alignment and weight-sharing techniques to fully exploit the similarity between simulated and real experimental images, thereby enhancing the denoising performance in both the simulation and experimental domains. Experimental results demonstrate that the proposed PDA-Net successfully enhances the quality of STM images, offering promising applications to enhance scientific discovery and accelerate experimental quantum material research.
图像去噪是医学成像和材料表征等各个领域中的一项重要任务,从噪声数据中准确恢复底层结构是至关重要的。尽管监督去噪技术在图像去噪方面取得了重大进展,但它们通常需要大量的配对干净和带噪声的图像数据集来进行训练。虽然无监督的方法不依赖于配对数据,但它们通常需要一组未配对的干净图像来进行训练,而这些图像并不总是可获得的。在本文中,我们提出了一种基于物理增强深度学习的无监督图像去噪框架(PDA-Net),并将其应用于去除现实世界中的扫描隧道显微镜(STM)图像的噪声。我们的PDA-Net利用基础物理原理来模拟和预测去噪STM图像的真相。此外,基于生成对抗网络(GANs),我们将循环一致性模块和域对抗模块纳入我们的PDA-Net中,以解决缺乏配对训练数据的挑战,并在模拟和真实实验域之间实现信息传输。最后,我们提出实施特征对齐和权重共享技术,以充分利用模拟图像和真实实验图像之间的相似性,从而提高仿真和实验域的去噪性能。实验结果表明,所提出的PDA-Net成功提高了STM图像的质量,在促进科学发现和加速实验量子材料研究方面显示出有前景的应用。
论文及项目相关链接
Summary
图像去噪在医学成像和材料表征等领域至关重要。传统去噪技术通常需要大量的配对干净与带噪图像数据集进行训练,但在无配对数据或仅有无监督方法可用的情况下,实现准确去噪仍是一大挑战。本文提出了一种基于物理增强深度学习和对抗域适应(PDA-Net)的无监督图像去噪框架,并应用于真实扫描隧道显微镜(STM)图像去噪。利用物理模拟和GAN技术,PDA-Net可在无配对数据的情况下实现信息转移和模拟与真实实验域之间的对齐。实验结果证明,PDA-Net成功提高了STM图像质量,为科学发现和量子材料研究提供了有力支持。
Key Takeaways
- 图像去噪在医学成像、材料表征等领域具有关键作用,需要有效的技术恢复底层结构。
- 传统的监督去噪技术需要大量的配对干净与带噪图像数据集,而无监督方法则面临缺乏配对数据的问题。
- PDA-Net框架结合了物理模拟和GAN技术,实现了无监督图像去噪,特别适用于真实STM图像去噪。
- PDA-Net通过利用物理模拟来模拟真实图像的底层结构,提高了去噪性能。
- GAN中的循环一致性和域对抗模块解决了缺乏配对训练数据的问题,实现了模拟与真实实验域之间的信息转移。
- 特征对齐和权重共享技术被用于增强模拟和真实实验图像之间的相似性,进一步提高了去噪性能。
点此查看论文截图
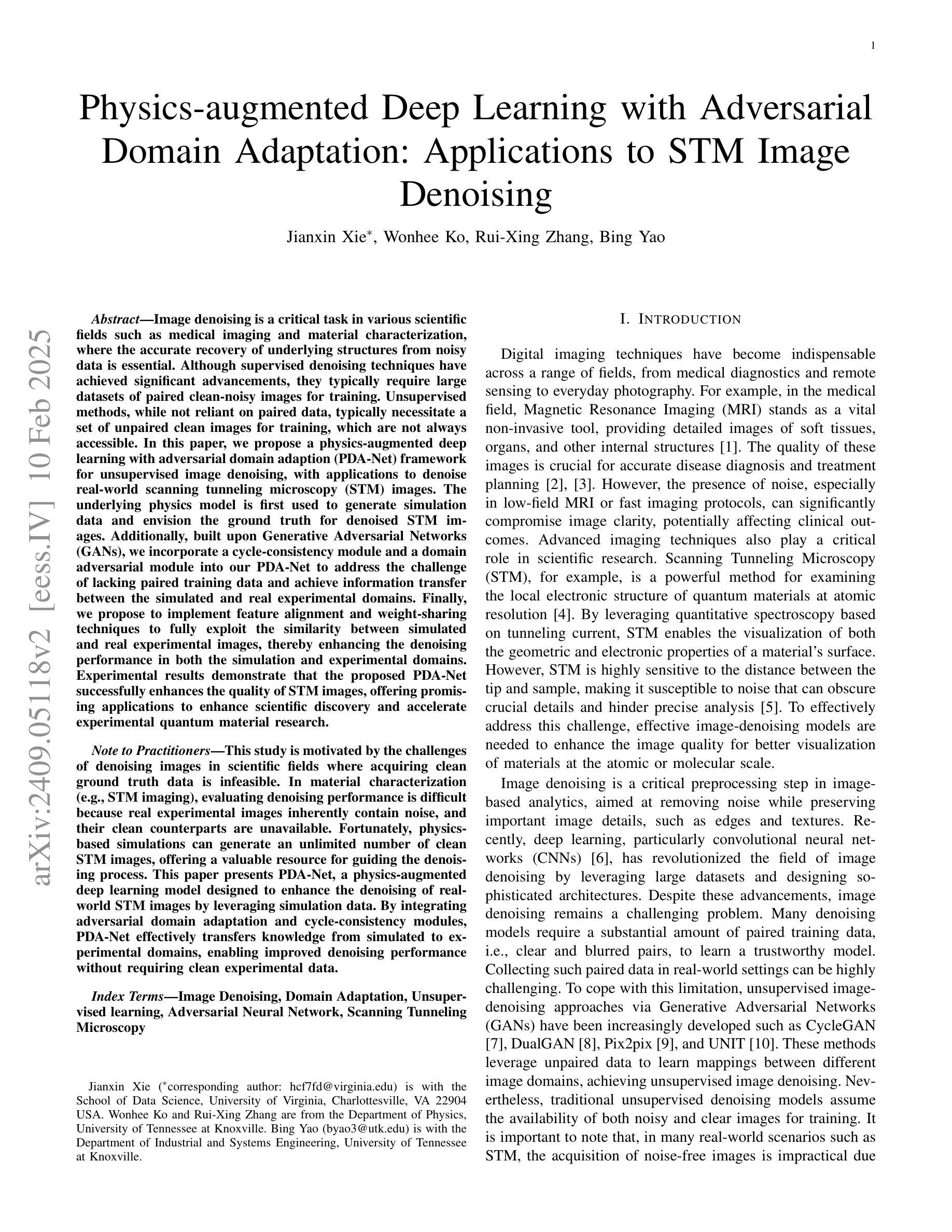
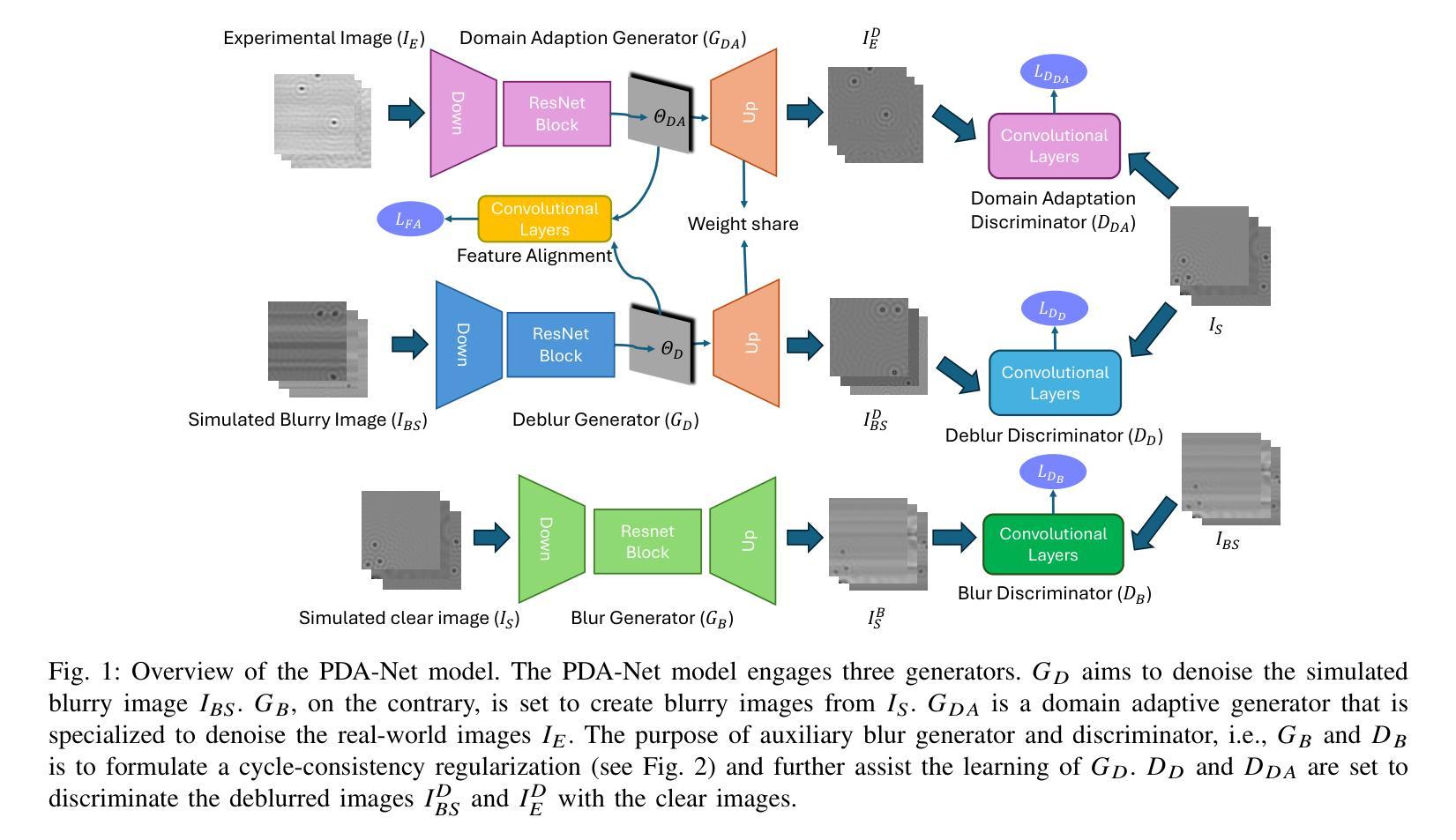
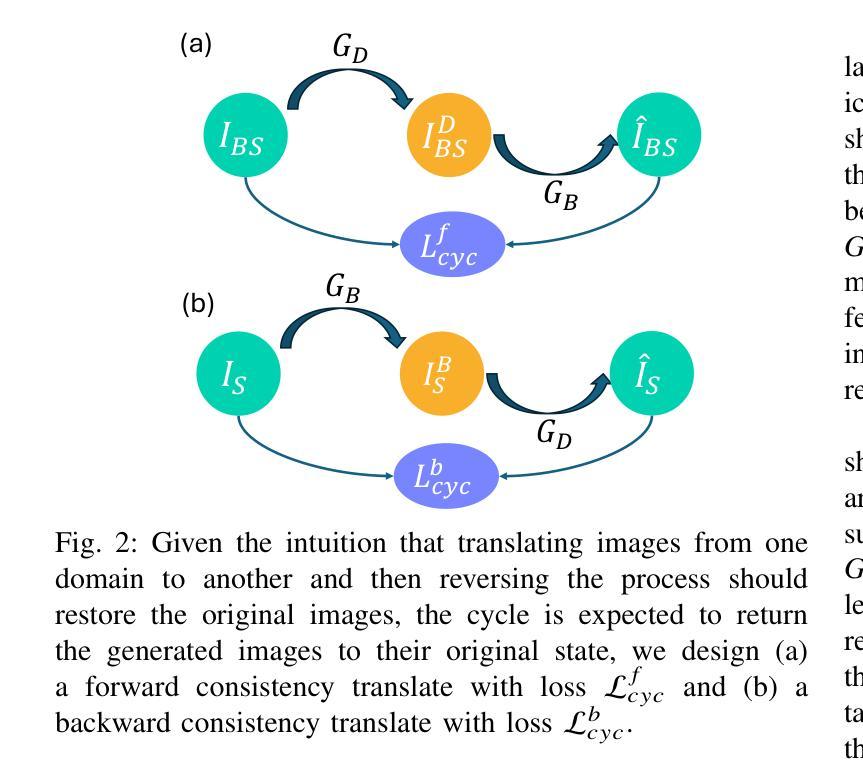
Adapt then Unlearn: Exploring Parameter Space Semantics for Unlearning in Generative Adversarial Networks
Authors:Piyush Tiwary, Atri Guha, Subhodip Panda, Prathosh A. P
Owing to the growing concerns about privacy and regulatory compliance, it is desirable to regulate the output of generative models. To that end, the objective of this work is to prevent the generation of outputs containing undesired features from a pre-trained Generative Adversarial Network (GAN) where the underlying training data set is inaccessible. Our approach is inspired by the observation that the parameter space of GANs exhibits meaningful directions that can be leveraged to suppress specific undesired features. However, such directions usually result in the degradation of the quality of generated samples. Our proposed two-stage method, known as ‘Adapt-then-Unlearn,’ excels at unlearning such undesirable features while also maintaining the quality of generated samples. In the initial stage, we adapt a pre-trained GAN on a set of negative samples (containing undesired features) provided by the user. Subsequently, we train the original pre-trained GAN using positive samples, along with a repulsion regularizer. This regularizer encourages the learned model parameters to move away from the parameters of the adapted model (first stage) while not degrading the generation quality. We provide theoretical insights into the proposed method. To the best of our knowledge, our approach stands as the first method addressing unlearning within the realm of high-fidelity GANs (such as StyleGAN). We validate the effectiveness of our method through comprehensive experiments, encompassing both class-level unlearning on the MNIST and AFHQ dataset and feature-level unlearning tasks on the CelebA-HQ dataset. Our code and implementation is available at: https://github.com/atriguha/Adapt_Unlearn.
鉴于对隐私和合规性问题的日益关注,对生成模型的输出进行监管是理想的。为此,这项工作旨在防止从预训练的生成对抗网络(GAN)生成包含不需要的特征的输出,其中基础训练数据集无法访问。我们的方法受到以下观察结果的启发:GAN的参数空间表现出有意义的方向,可以借此抑制特定的不需要的特征。然而,这样的方向通常会导致生成的样本质量下降。我们提出的两阶段方法,被称为“适应然后遗忘”,在消除这些不需要的特征的同时,也保持了生成样本的质量。在第一阶段,我们根据用户提供的包含不需要特征的负样本集适应预训练的GAN。随后,我们使用正样本和排斥正则化器训练原始的预训练GAN。该正则化器鼓励学习到的模型参数远离适应模型(第一阶段)的参数,同时不降低生成质量。我们为所提出的方法提供了理论见解。据我们所知,我们的方法是第一个在高保真GAN(如StyleGAN)领域解决遗忘问题的方法。我们通过包括在MNIST和AFHQ数据集上进行类别级别的遗忘以及在CelebA-HQ数据集上进行特征级别的遗忘任务的全面实验验证了我们的方法的有效性。我们的代码和实现可在:https://github.com/atriguha/Adapt_Unlearn找到。
论文及项目相关链接
PDF Accepted at Transactions on Machine Learning Research (TMLR)
Summary
本论文针对生成模型输出监管问题展开研究,提出了一种基于生成对抗网络(GAN)的无需访问底层训练数据集的方法,用于抑制预训练GAN生成包含不需要特征的输出。该方法分为两个阶段:“适应后遗忘”。首先,通过用户提供的包含不需要特征的负样本集对预训练GAN进行适应。然后,使用正样本和排斥正则化器再次训练原始预训练GAN。该正则化器鼓励学习到的模型参数远离适应模型(第一阶段)的参数,同时不降低生成质量。该方法在保持样本质量的同时,实现了对不需要特征的遗忘。在MNIST、AFHQ和CelebA-HQ数据集上的实验验证了该方法的有效性。代码已公开发布在GitHub上。
Key Takeaways
- 为应对隐私和监管合规性的日益关注,需要调控生成模型的输出。
- 研究旨在从预训练的GAN中抑制不需要的特征输出,且无需访问底层训练数据集。
- GAN参数空间存在有意义的方向,可用来抑制特定不需要的特征,但这可能导致生成样本的质量下降。
- 提出的“适应后遗忘”的两阶段方法能够在保持生成样本质量的同时,抑制不需要的特征。
- 第一阶段通过负样本集适应预训练GAN;第二阶段使用正样本和排斥正则化器进行训练。
- 该方法提供了理论上的见解,并被认为是首个在高保真GAN(如StyleGAN)领域解决遗忘问题的方法。
点此查看论文截图
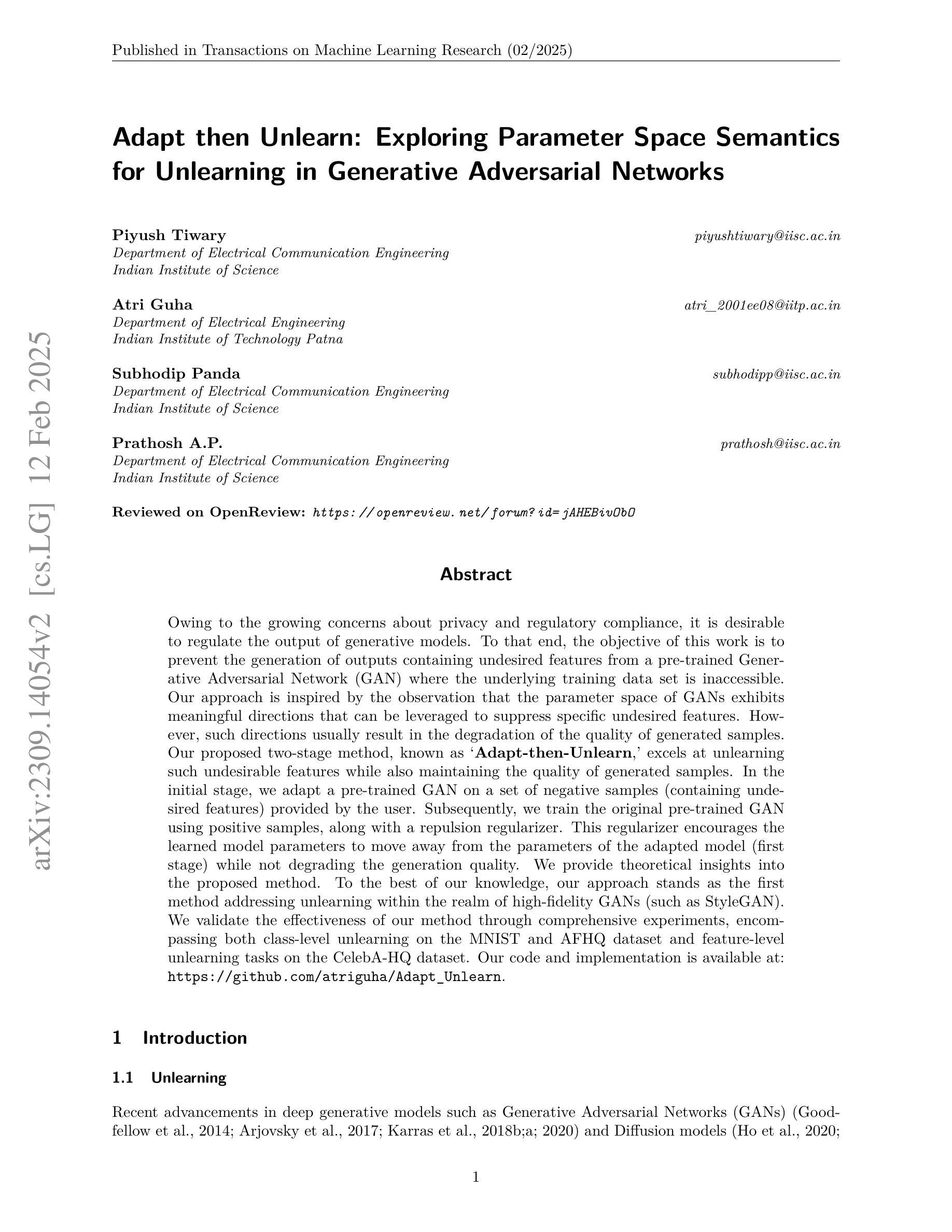
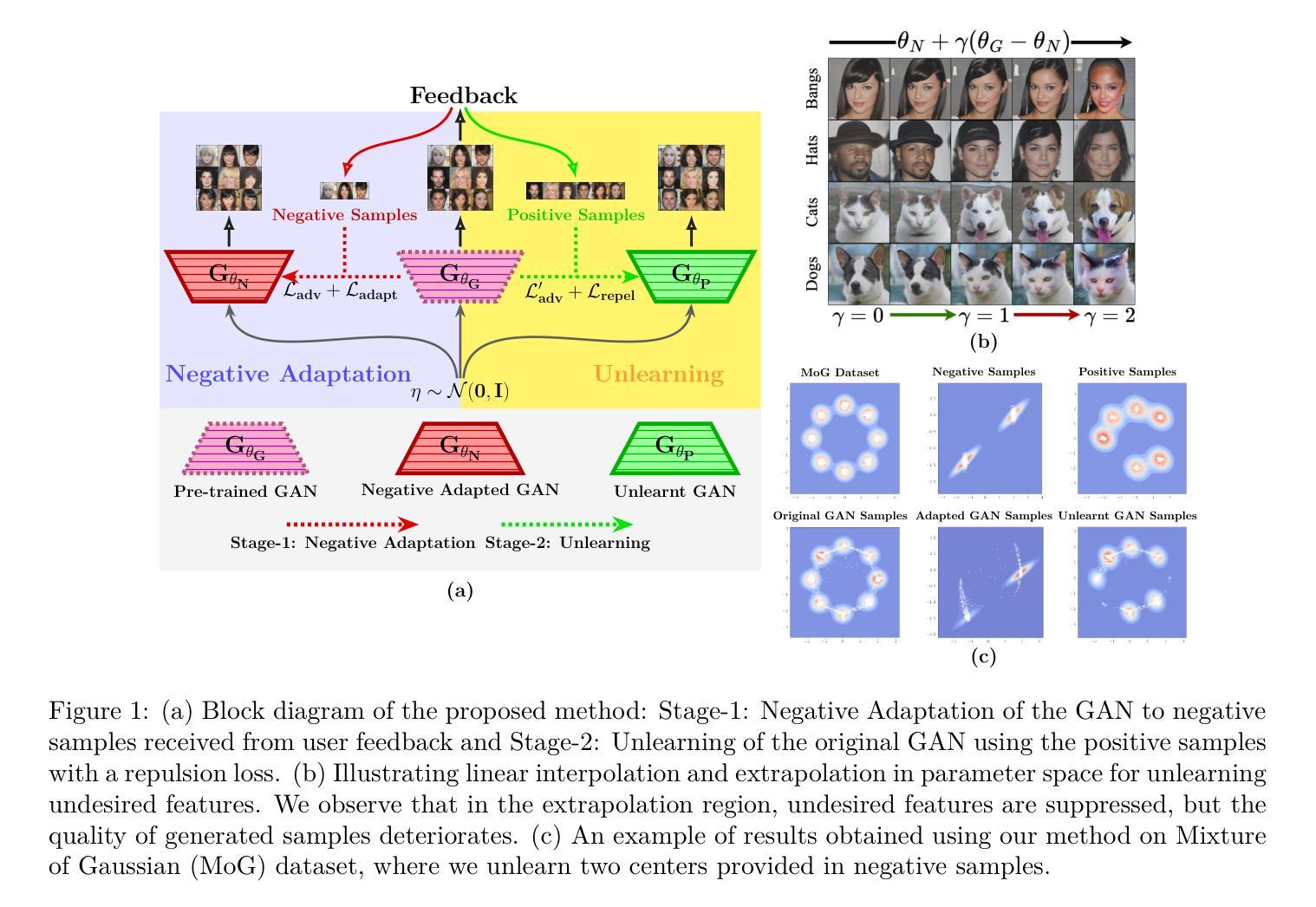