⚠️ 以下所有内容总结都来自于 大语言模型的能力,如有错误,仅供参考,谨慎使用
🔴 请注意:千万不要用于严肃的学术场景,只能用于论文阅读前的初筛!
💗 如果您觉得我们的项目对您有帮助 ChatPaperFree ,还请您给我们一些鼓励!⭐️ HuggingFace免费体验
2025-02-13 更新
LoRP-TTS: Low-Rank Personalized Text-To-Speech
Authors:Łukasz Bondaruk, Jakub Kubiak
Speech synthesis models convert written text into natural-sounding audio. While earlier models were limited to a single speaker, recent advancements have led to the development of zero-shot systems that generate realistic speech from a wide range of speakers using their voices as additional prompts. However, they still struggle with imitating non-studio-quality samples that differ significantly from the training datasets. In this work, we demonstrate that utilizing Low-Rank Adaptation (LoRA) allows us to successfully use even single recordings of spontaneous speech in noisy environments as prompts. This approach enhances speaker similarity by up to $30pp$ while preserving content and naturalness. It represents a significant step toward creating truly diverse speech corpora, that is crucial in all speech-related tasks.
语音合成模型将书面文字转换为自然听感的音频。虽然早期模型仅限于单一发言人,但最近的进步已经催生出了一种零样本系统,这种系统可以利用各种发言人的声音作为额外的提示来生成逼真的语音。然而,它们仍然难以模仿与训练数据集差异很大的非工作室质量样本。在这项工作中,我们证明利用低秩自适应(LoRA)技术,即使使用单一环境下的自发语音录音作为提示,也能成功地进行使用。这种方法在保持内容和自然性的同时,提高了高达30pp的说话人相似性。这是朝着创建真正多样化的语音语料库迈出的重要一步,这对于所有与语音相关的任务都是至关重要的。
论文及项目相关链接
Summary
本文介绍了语音合成模型的发展,包括零样本系统可以生成多种声音逼真度高的语音。尽管该技术在模仿非工作室环境下的样本方面仍有挑战,但采用低秩适应技术可以成功使用单个录音作为提示,提高说话人的相似性,同时保持内容和自然度。这为创建真正多样化的语音语料库迈出了重要的一步。
Key Takeaways
- 语音合成模型能将书面文本转化为自然流畅的音频。
- 早期的语音合成模型仅限于单一说话人,但最新技术已发展到零样本系统,可从广泛的说话人生成逼真的语音。
- 非工作室环境下的样本模仿对模型构成挑战。
- 采用低秩适应技术可以成功使用单个录音作为提示,提高说话人的相似性。
- 低秩适应技术能提高说话人相似性高达30pp,同时保持内容和自然度。
- 此技术对于创建真正多样化的语音语料库至关重要。
点此查看论文截图
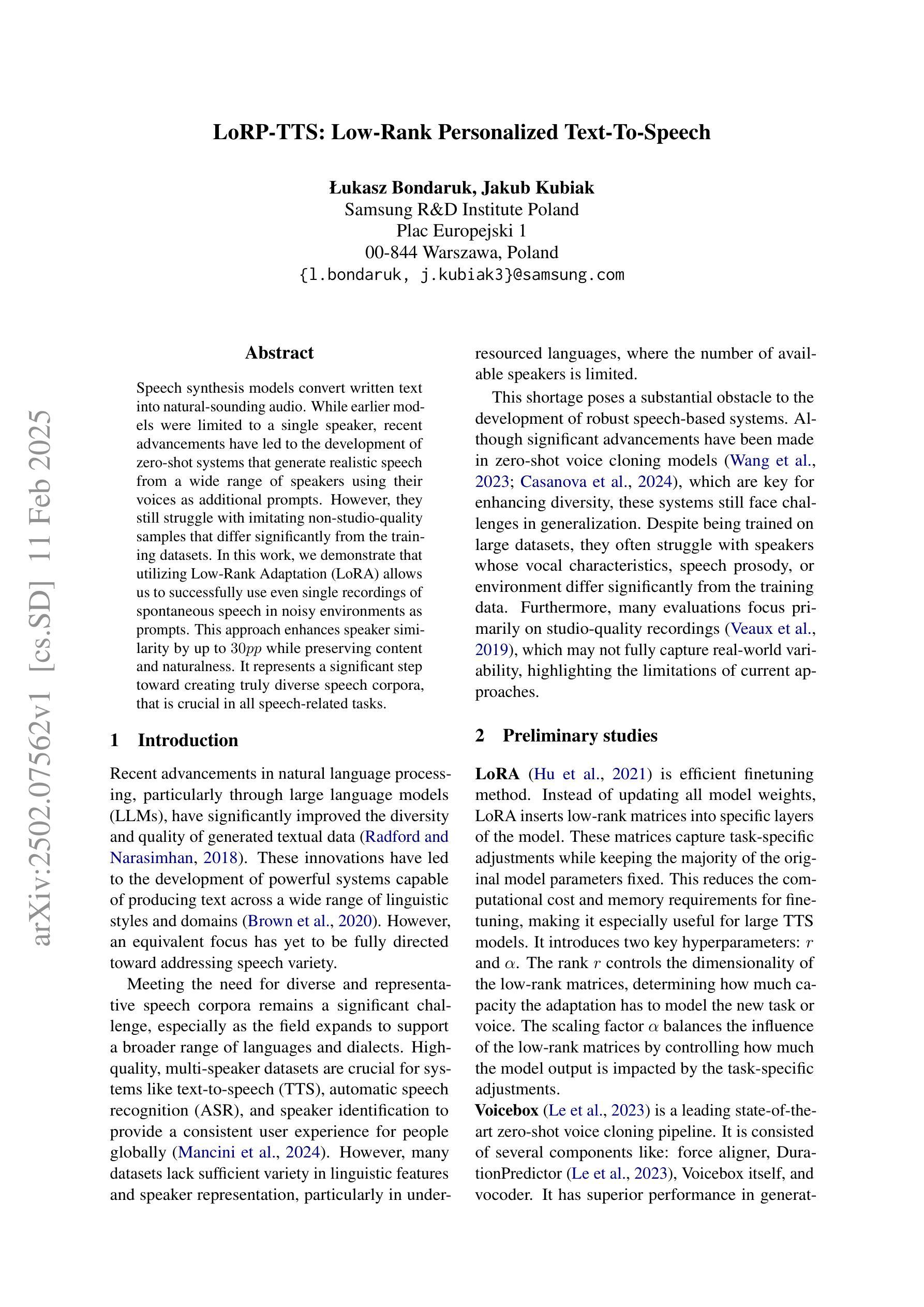
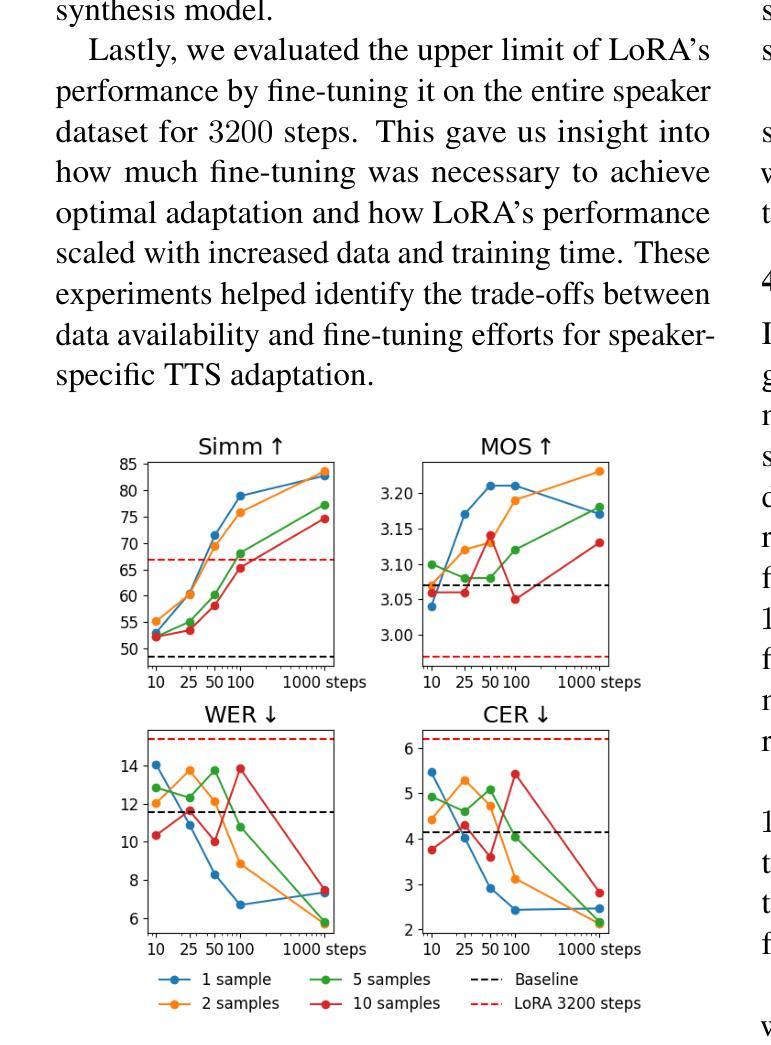
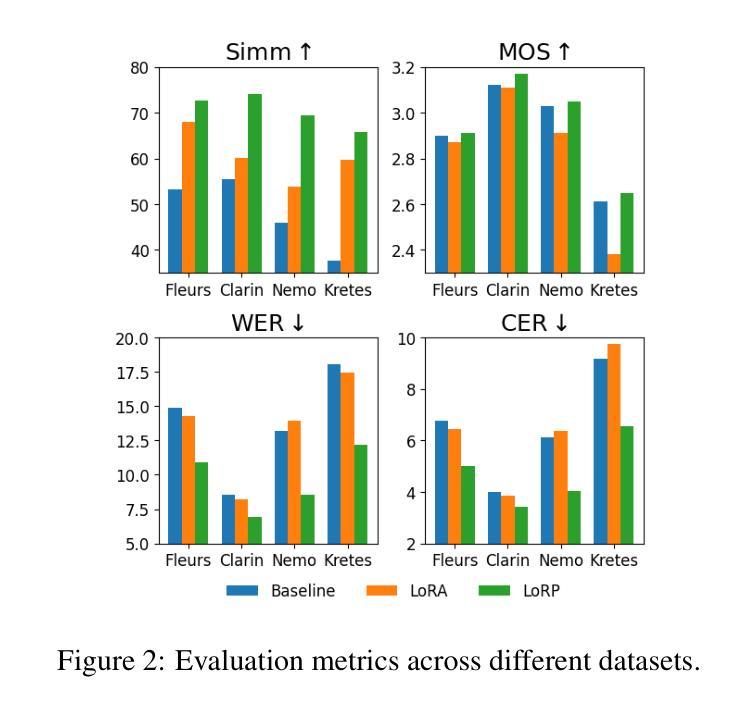
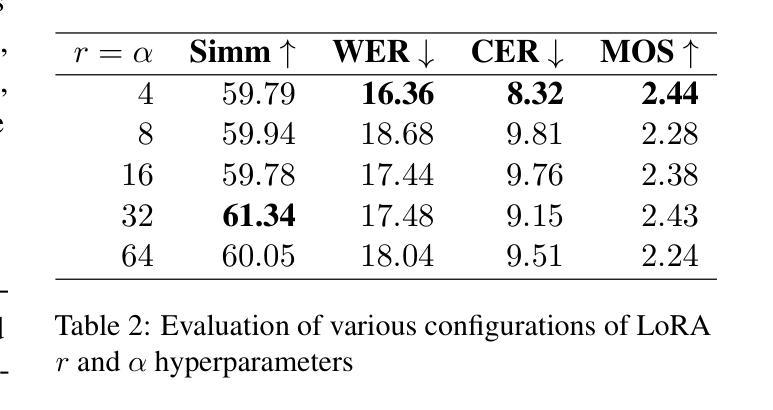
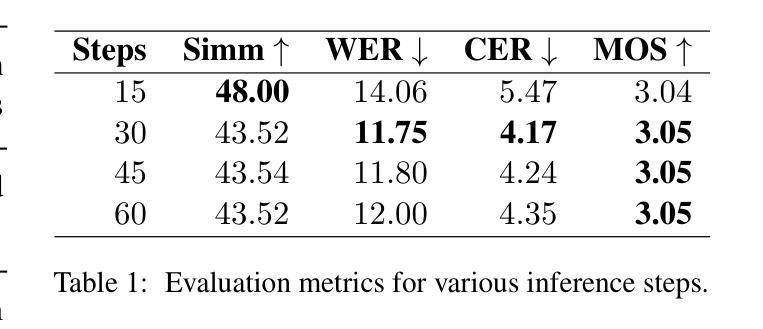
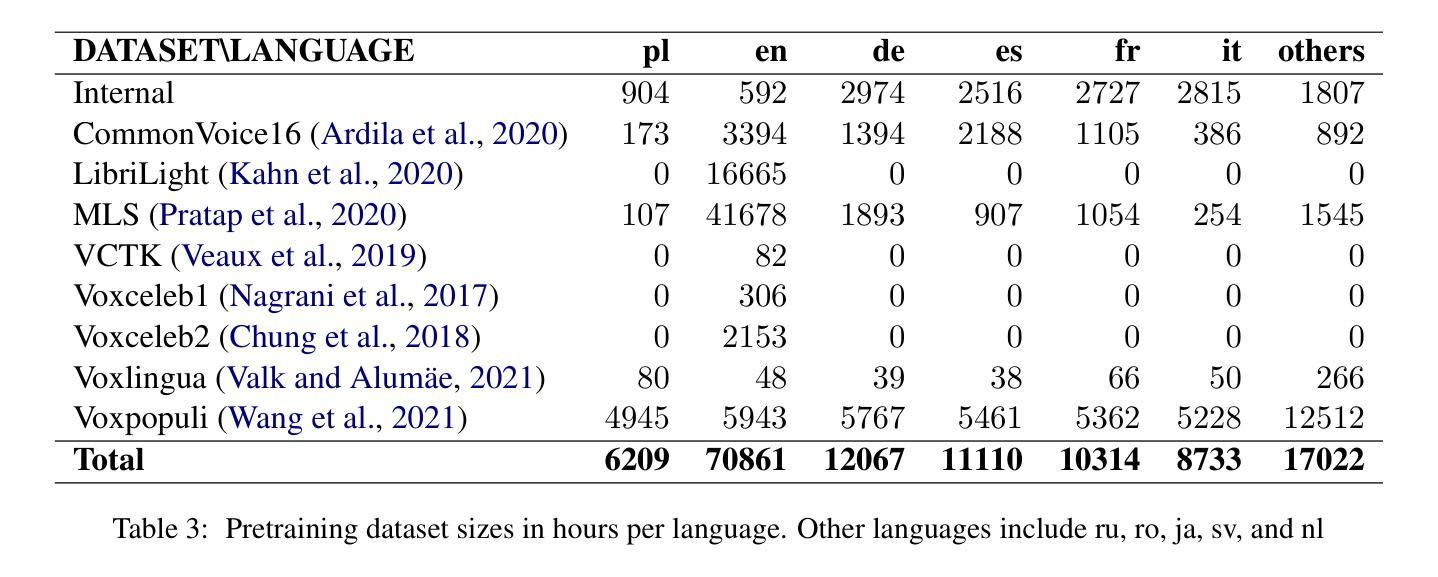
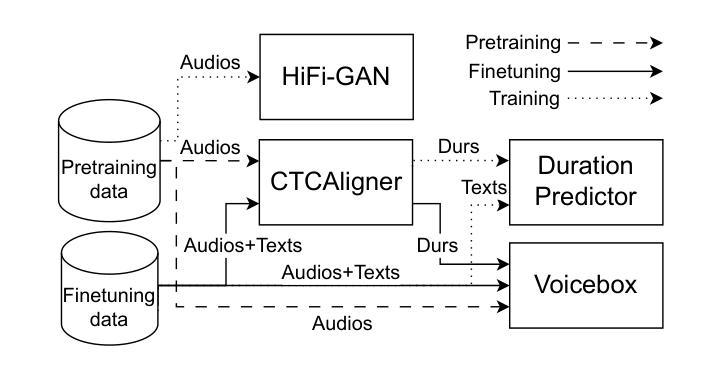
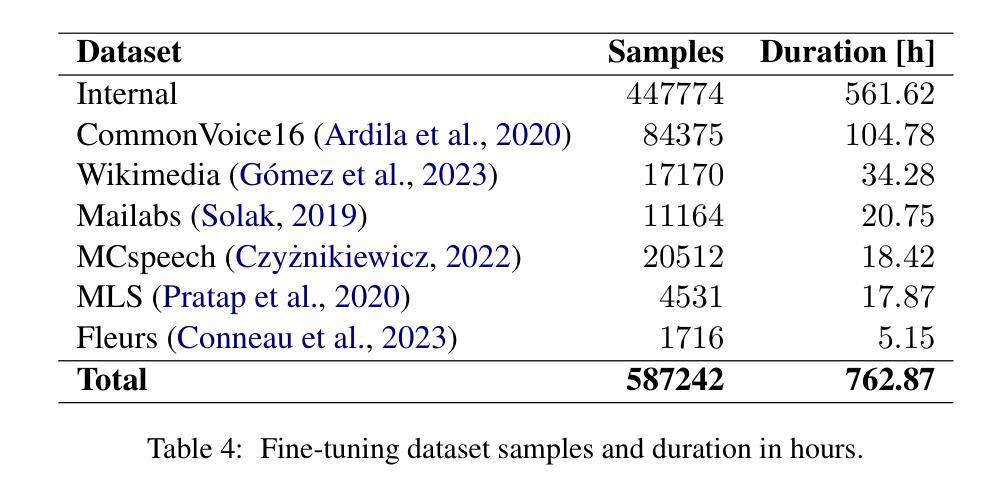
Advanced Zero-Shot Text-to-Speech for Background Removal and Preservation with Controllable Masked Speech Prediction
Authors:Leying Zhang, Wangyou Zhang, Zhengyang Chen, Yanmin Qian
The acoustic background plays a crucial role in natural conversation. It provides context and helps listeners understand the environment, but a strong background makes it difficult for listeners to understand spoken words. The appropriate handling of these backgrounds is situation-dependent: Although it may be necessary to remove background to ensure speech clarity, preserving the background is sometimes crucial to maintaining the contextual integrity of the speech. Despite recent advancements in zero-shot Text-to-Speech technologies, current systems often struggle with speech prompts containing backgrounds. To address these challenges, we propose a Controllable Masked Speech Prediction strategy coupled with a dual-speaker encoder, utilizing a task-related control signal to guide the prediction of dual background removal and preservation targets. Experimental results demonstrate that our approach enables precise control over the removal or preservation of background across various acoustic conditions and exhibits strong generalization capabilities in unseen scenarios.
背景声音在自然对话中扮演着至关重要的角色。它提供了上下文并帮助听众理解环境,但强烈的背景声音会让听众难以理解说话的内容。这些背景的处理是否得当取决于具体情境:虽然为了确保言语清晰可能需要消除背景,但有时保留背景对于维持言语的上下文完整性至关重要。尽管零样本文本到语音技术最近有所进展,但当前的系统在处理包含背景的语音提示时经常遇到困难。为了应对这些挑战,我们提出了一种可控的掩码语音预测策略,并结合了双讲者编码器,利用与任务相关的控制信号来指导双背景去除和保留目标的预测。实验结果表明,我们的方法能够精确控制不同声学条件下的背景去除或保留,并在未见过的场景中表现出强大的泛化能力。
论文及项目相关链接
PDF Accepted by ICASSP 2025
Summary
背景声音在自然对话中扮演重要角色,既提供语境又帮助听者理解环境。但强烈的背景声音会使听者难以理解口语。背景的处理方式因情况而异,既要确保言语清晰,又要保持语境完整性。尽管文本转语音技术近年来有所发展,但当前系统对含有背景的语音提示仍有困难。为解决这些问题,我们提出了可控的掩蔽语音预测策略,并结合双说话人编码器,利用任务相关控制信号来指导双背景去除和保留目标的预测。实验结果表明,我们的方法能够在各种声学条件下精确地控制背景的去除或保留,并在未见过的场景中表现出强大的泛化能力。
Key Takeaways
- 背景声音在自然对话中至关重要,提供语境并帮助理解环境。
- 强烈的背景声音会影响听者对口语的理解。
- 背景声音的处理方式因情况而异,需平衡言语清晰与语境完整性。
- 当前文本转语音技术在处理含有背景的语音提示时面临挑战。
- 提出了可控的掩蔽语音预测策略结合双说话人编码器以解决问题。
- 任务相关控制信号用于指导双背景去除和保留目标的预测。
点此查看论文截图
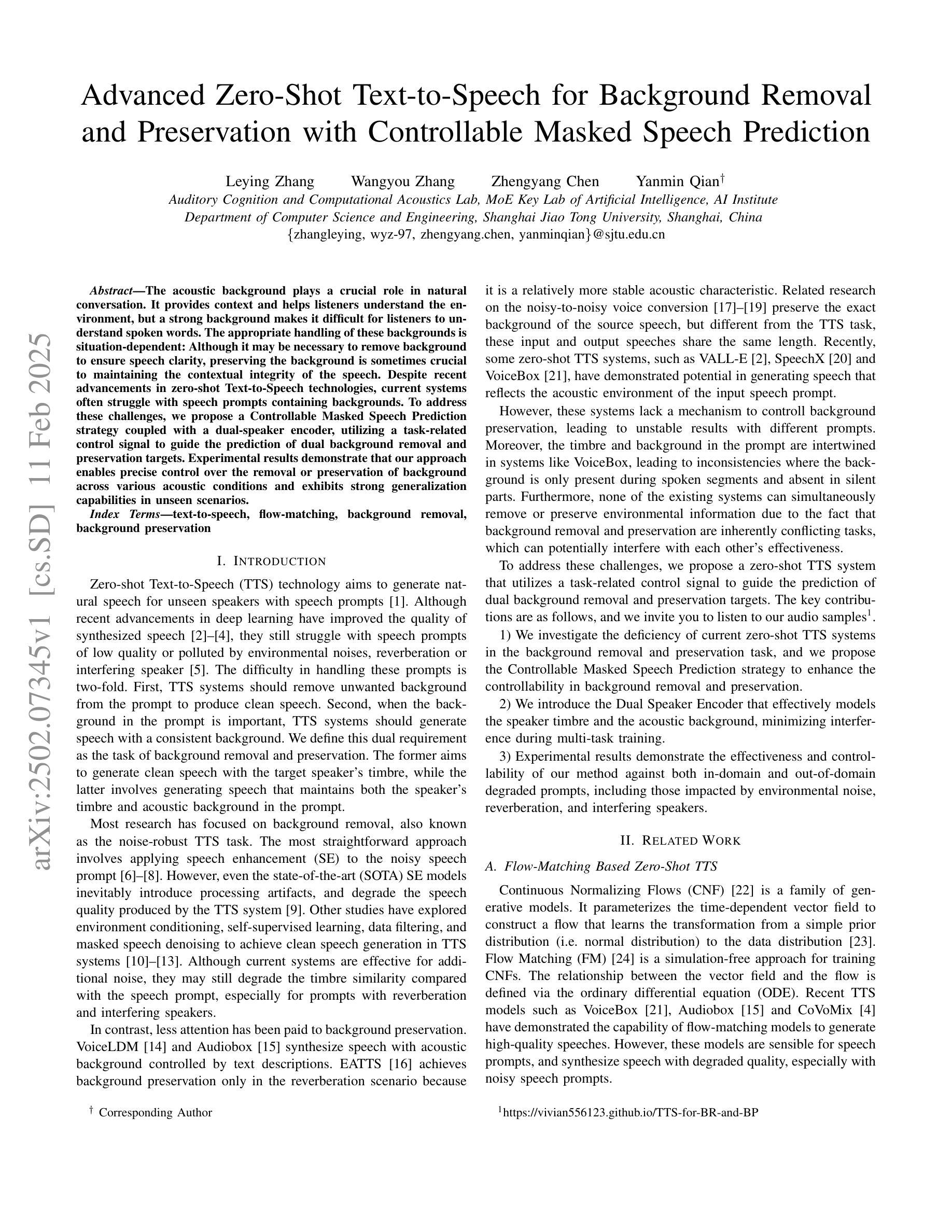
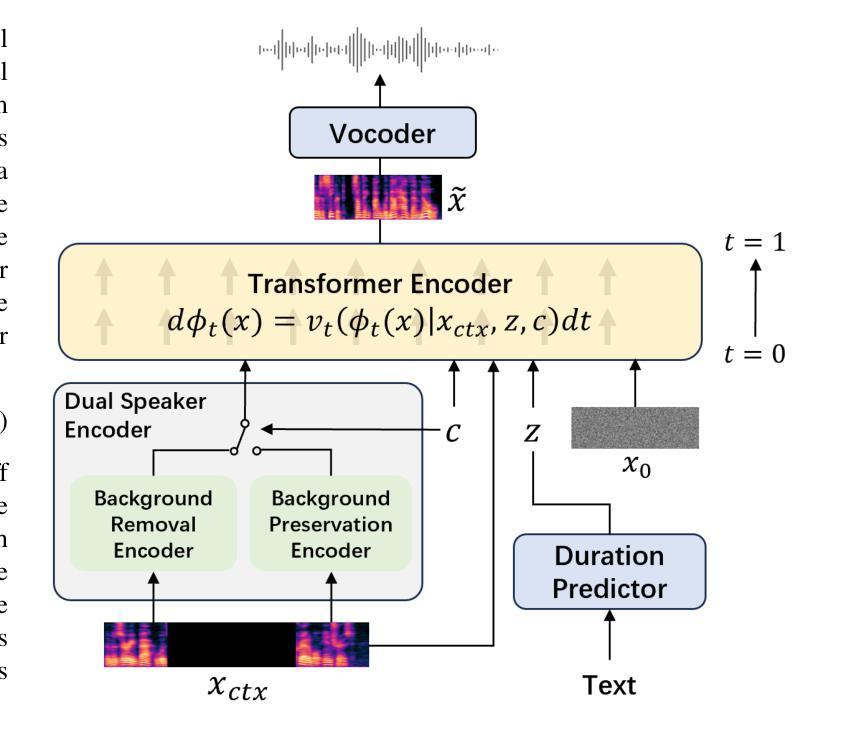


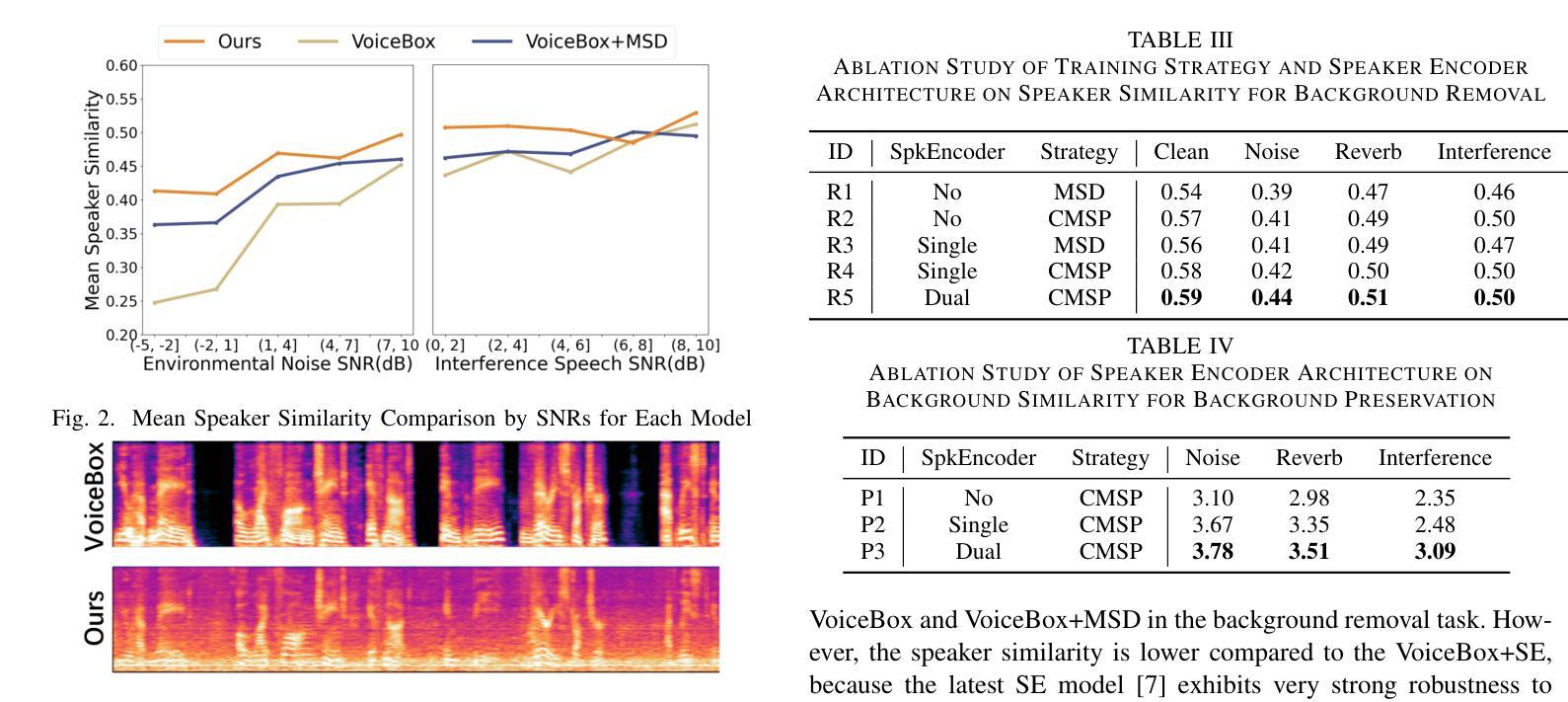
Vevo: Controllable Zero-Shot Voice Imitation with Self-Supervised Disentanglement
Authors:Xueyao Zhang, Xiaohui Zhang, Kainan Peng, Zhenyu Tang, Vimal Manohar, Yingru Liu, Jeff Hwang, Dangna Li, Yuhao Wang, Julian Chan, Yuan Huang, Zhizheng Wu, Mingbo Ma
The imitation of voice, targeted on specific speech attributes such as timbre and speaking style, is crucial in speech generation. However, existing methods rely heavily on annotated data, and struggle with effectively disentangling timbre and style, leading to challenges in achieving controllable generation, especially in zero-shot scenarios. To address these issues, we propose Vevo, a versatile zero-shot voice imitation framework with controllable timbre and style. Vevo operates in two core stages: (1) Content-Style Modeling: Given either text or speech’s content tokens as input, we utilize an autoregressive transformer to generate the content-style tokens, which is prompted by a style reference; (2) Acoustic Modeling: Given the content-style tokens as input, we employ a flow-matching transformer to produce acoustic representations, which is prompted by a timbre reference. To obtain the content and content-style tokens of speech, we design a fully self-supervised approach that progressively decouples the timbre, style, and linguistic content of speech. Specifically, we adopt VQ-VAE as the tokenizer for the continuous hidden features of HuBERT. We treat the vocabulary size of the VQ-VAE codebook as the information bottleneck, and adjust it carefully to obtain the disentangled speech representations. Solely self-supervised trained on 60K hours of audiobook speech data, without any fine-tuning on style-specific corpora, Vevo matches or surpasses existing methods in accent and emotion conversion tasks. Additionally, Vevo’s effectiveness in zero-shot voice conversion and text-to-speech tasks further demonstrates its strong generalization and versatility. Audio samples are available at https://versavoice.github.io.
针对特定语音属性(如音质和讲话风格)的语音模仿在语音生成中至关重要。然而,现有方法严重依赖于注释数据,并且在有效地分离音质和风格方面遇到困难,这导致在实现可控生成方面面临挑战,尤其在零样本场景中。为了解决这些问题,我们提出了Vevo,这是一个通用零样本语音模仿框架,具有可控的音质和风格。Vevo分为两个核心阶段:(1)内容-风格建模:给定文本或语音的内容标记作为输入,我们利用自回归变压器生成由风格参考提示的内容-风格标记;(2)声音建模:给定内容-风格标记作为输入,我们采用流匹配变压器来产生声音表现,这由音质参考提示。为了获得语音的内容和风格标记,我们设计了一种完全自监督的方法,逐步地解耦语音的音质、风格和语言内容。具体来说,我们采用VQ-VAE作为HuBERT连续隐藏特征的标记器。我们将VQ-VAE代码本的词汇量大小视为信息瓶颈,并小心地调整它以获得分离的语音表示。仅在6万小时的有声读物语音数据上进行自监督训练,无需在特定风格的语料库上进行微调,Vevo在口音和情绪转换任务中的表现与现有方法相匹配或超越。此外,Vevo在零样本语音转换和文本到语音任务中的有效性进一步证明了其强大的通用性和适应性。音频样本可在https://versavoice.github.io获取。
论文及项目相关链接
PDF Accepted by ICLR 2025
摘要
语音模仿对于语音生成至关重要,尤其是在模仿特定语音属性如音质和说话风格方面。然而,现有方法严重依赖于标注数据,并且在有效分离音质和风格方面面临挑战,尤其是在零样本场景中难以实现可控生成。为解决这些问题,我们提出了Vevo,一个通用零样本语音模仿框架,具有可控的音质和风格。Vevo分为两个阶段:内容风格建模和声音建模。在内容风格建模阶段,我们采用自回归转换器生成由风格提示驱动的内容风格令牌。在声音建模阶段,我们以内容风格令牌为输入,使用流匹配转换器产生由音质提示驱动的声学表示。为了获得语音的内容和风格令牌,我们设计了一种完全自监督的方法,逐步分离语音的音质、风格和语言内容。我们采用VQ-VAE作为HuBERT连续隐藏特征的令牌化器。我们将VQ-VAE代码本的词汇量大小视为信息瓶颈,并仔细调整以获得分离的语音表示。仅通过自监督方式在6万小时有声书籍语音数据上进行训练,无需在特定风格语料库上进行微调,Vevo在有声书和诗歌阅读任务的多个基准测试中达到或超越了现有方法的效果。此外,Vevo在零样本语音转换和文本到语音任务中的有效性进一步证明了其强大的通用性和灵活性。音频样本可在https://versavoice.github.io获取。
要点
- 语音模仿是语音生成中的关键,涉及特定语音属性的模仿,如音质和说话风格。
- 现有方法依赖标注数据,在分离音质和风格方面存在挑战,尤其在零样本场景中。
- 提出的Vevo框架通过两个阶段实现语音模仿:内容风格建模和声音建模。
- Vevo采用自监督学习方式,在大量语音数据上训练,无需特定风格语料库的微调。
- Vevo框架可以在零样本场景中进行语音转换和文本到语音任务,表现出强大的通用性和灵活性。
- Vevo在多个基准测试中表现优异,包括口音和情绪转换任务。
点此查看论文截图
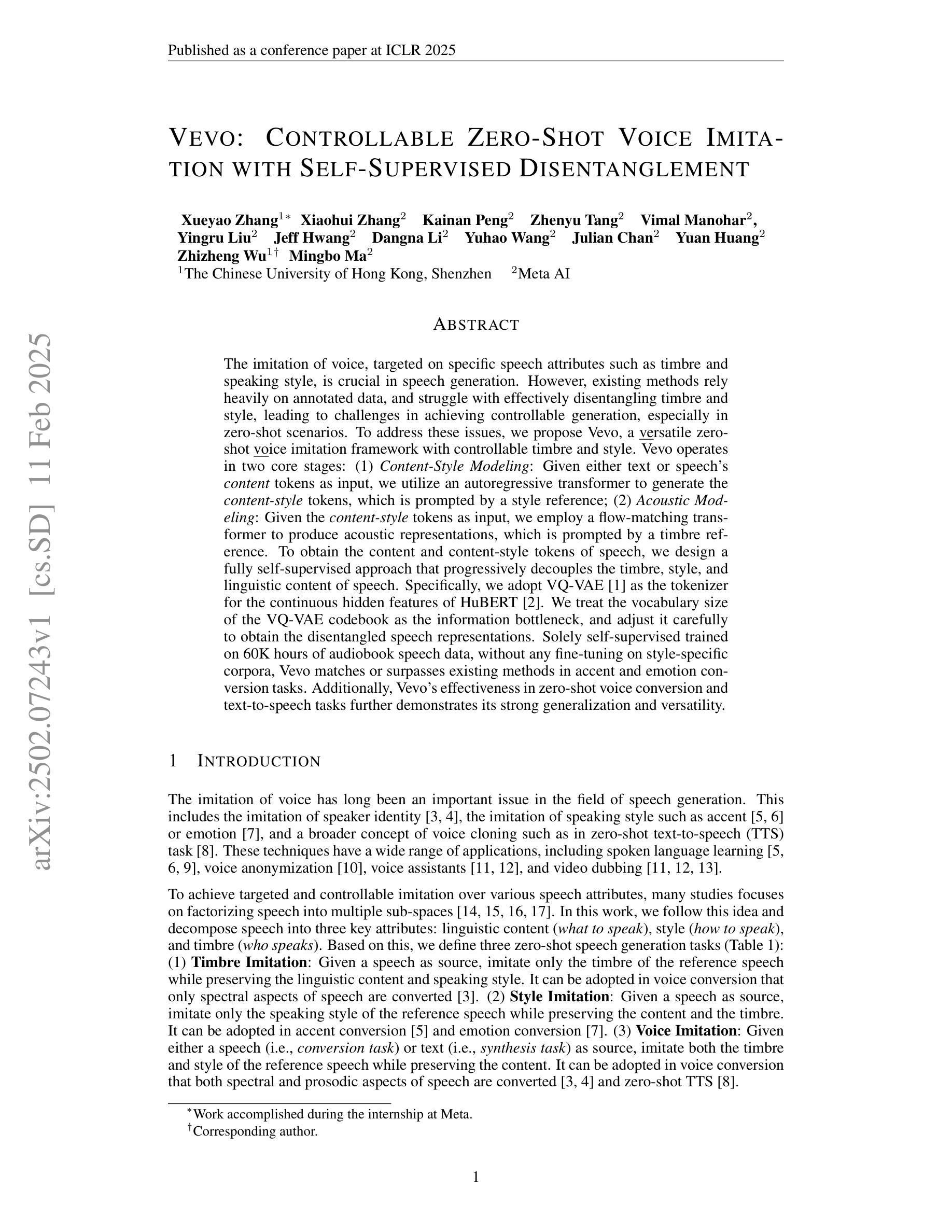
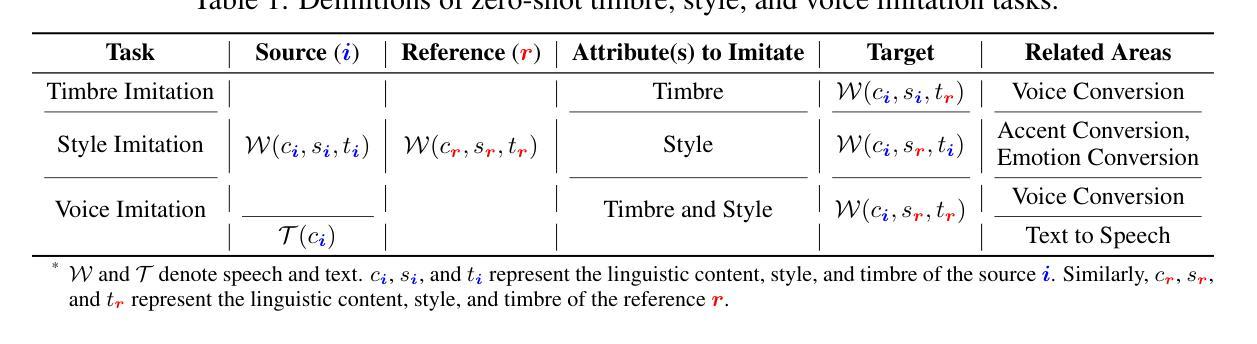
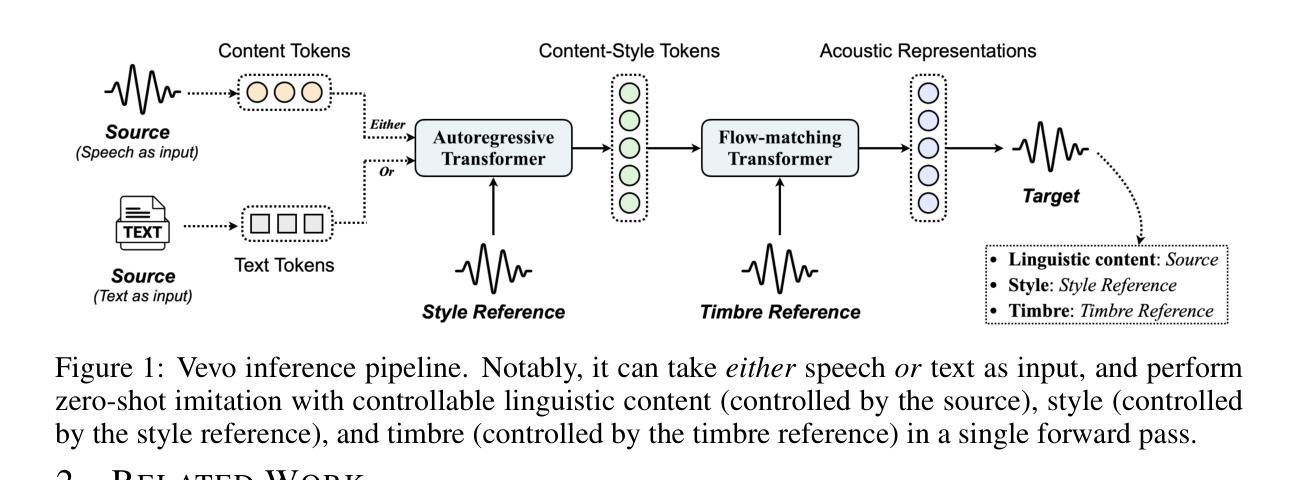
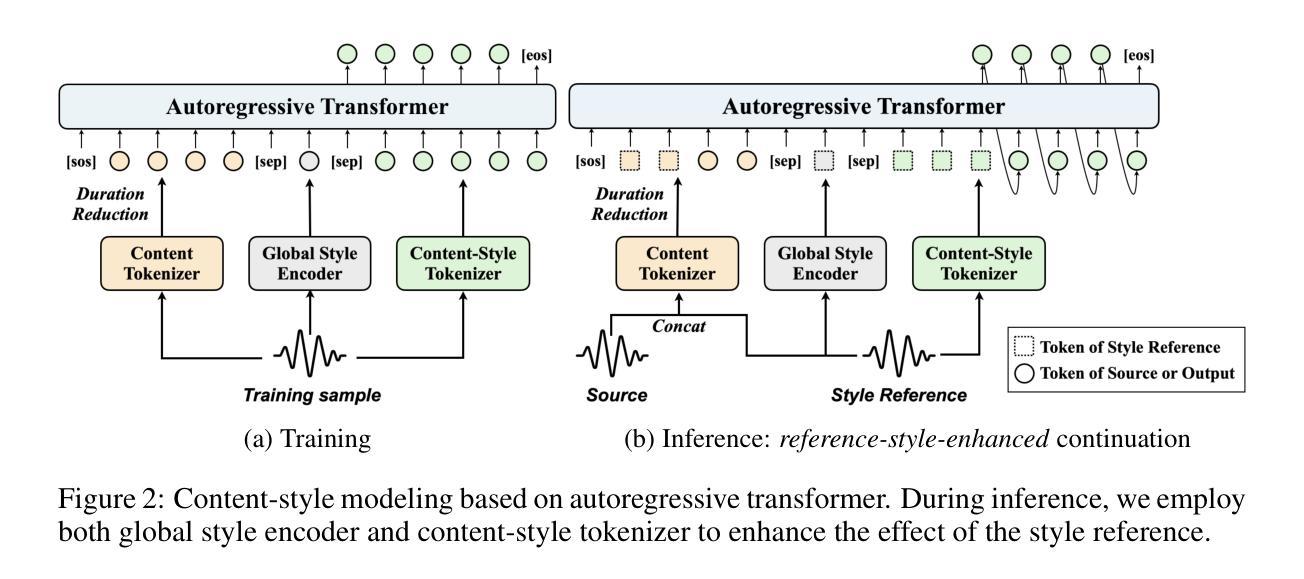
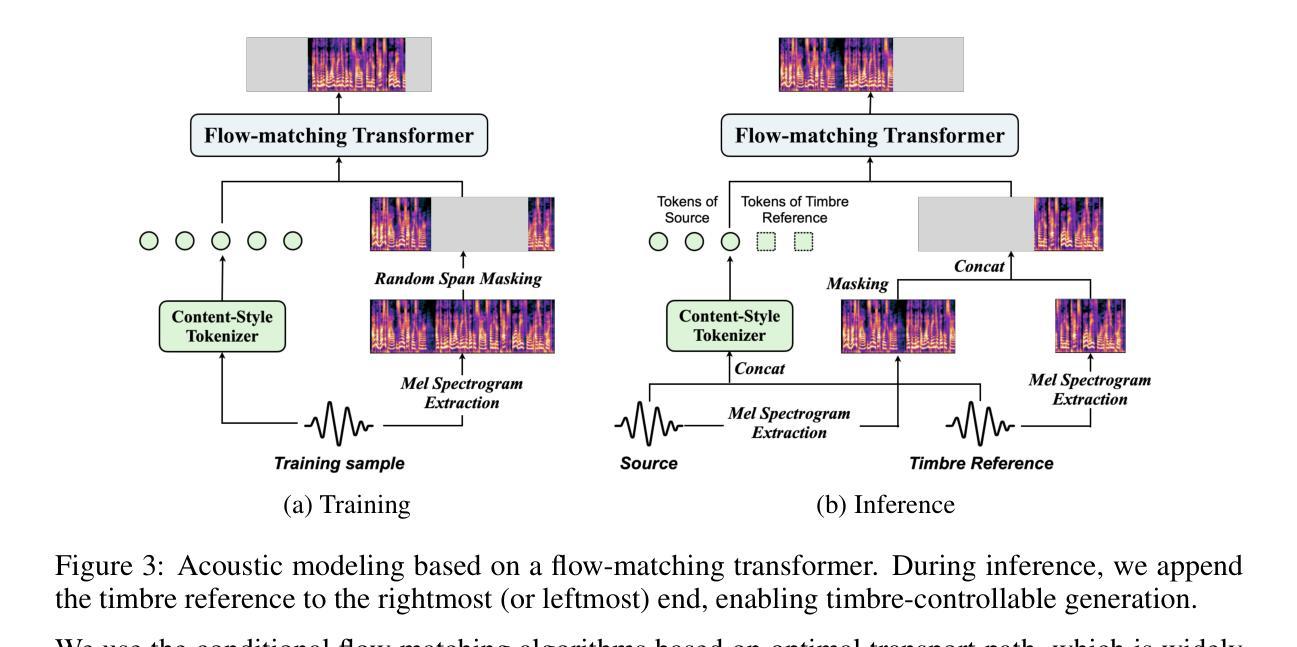