⚠️ 以下所有内容总结都来自于 大语言模型的能力,如有错误,仅供参考,谨慎使用
🔴 请注意:千万不要用于严肃的学术场景,只能用于论文阅读前的初筛!
💗 如果您觉得我们的项目对您有帮助 ChatPaperFree ,还请您给我们一些鼓励!⭐️ HuggingFace免费体验
2025-02-13 更新
What Is That Talk About? A Video-to-Text Summarization Dataset for Scientific Presentations
Authors:Dongqi Liu, Chenxi Whitehouse, Xi Yu, Louis Mahon, Rohit Saxena, Zheng Zhao, Yifu Qiu, Mirella Lapata, Vera Demberg
Transforming recorded videos into concise and accurate textual summaries is a growing challenge in multimodal learning. This paper introduces VISTA, a dataset specifically designed for video-to-text summarization in scientific domains. VISTA contains 18,599 recorded AI conference presentations paired with their corresponding paper abstracts. We benchmark the performance of state-of-the-art large models and apply a plan-based framework to better capture the structured nature of abstracts. Both human and automated evaluations confirm that explicit planning enhances summary quality and factual consistency. However, a considerable gap remains between models and human performance, highlighting the challenges of scientific video summarization.
将录制的视频转化为简洁准确的文本摘要,这是多模态学习中的一个日益增长的挑战。本文介绍了专为科学领域视频到文本摘要而设计的VISTA数据集。VISTA包含18599个录制的AI会议演讲与其相应的论文摘要配对。我们评估了最先进的大型模型的性能,并应用了一个基于计划的框架来更好地捕捉摘要的结构性。人类和自动化评估都证实,明确的计划可以提高摘要的质量和事实一致性。然而,模型和人类性能之间仍存在很大差距,这突出了科学视频摘要的挑战性。
论文及项目相关链接
PDF arXiv admin note: text overlap with arXiv:2306.02873 by other authors
Summary:
本文介绍了为视频到文本摘要任务设计的VISTA数据集及其在人工智能领域的应用。该数据集包含与论文摘要相对应的AI会议演讲视频。文章通过对比先进的模型性能,验证了基于计划的框架可以更好地捕捉摘要的结构性特征。同时指出,虽然模型性能有所提升,但仍存在与人的表现差距,凸显了科学视频摘要的挑战性。
Key Takeaways:
- VISTA数据集专注于视频到文本摘要的科学领域,包含AI会议演讲视频和对应的论文摘要。
- 论文评估了先进的模型性能,发现基于计划的框架有助于捕捉摘要的结构性特征。
- 基于计划的框架能够提高摘要的质量和事实一致性。
- 当前模型与人类性能之间存在差距,显示科学视频摘要的挑战性。
- 视频到文本摘要是一个持续增长的挑战,需要更多的研究和发展来克服现有的困难。
- 视频摘要任务不仅需要自然语言处理技术,还需要理解视频内容及其上下文的能力。
点此查看论文截图
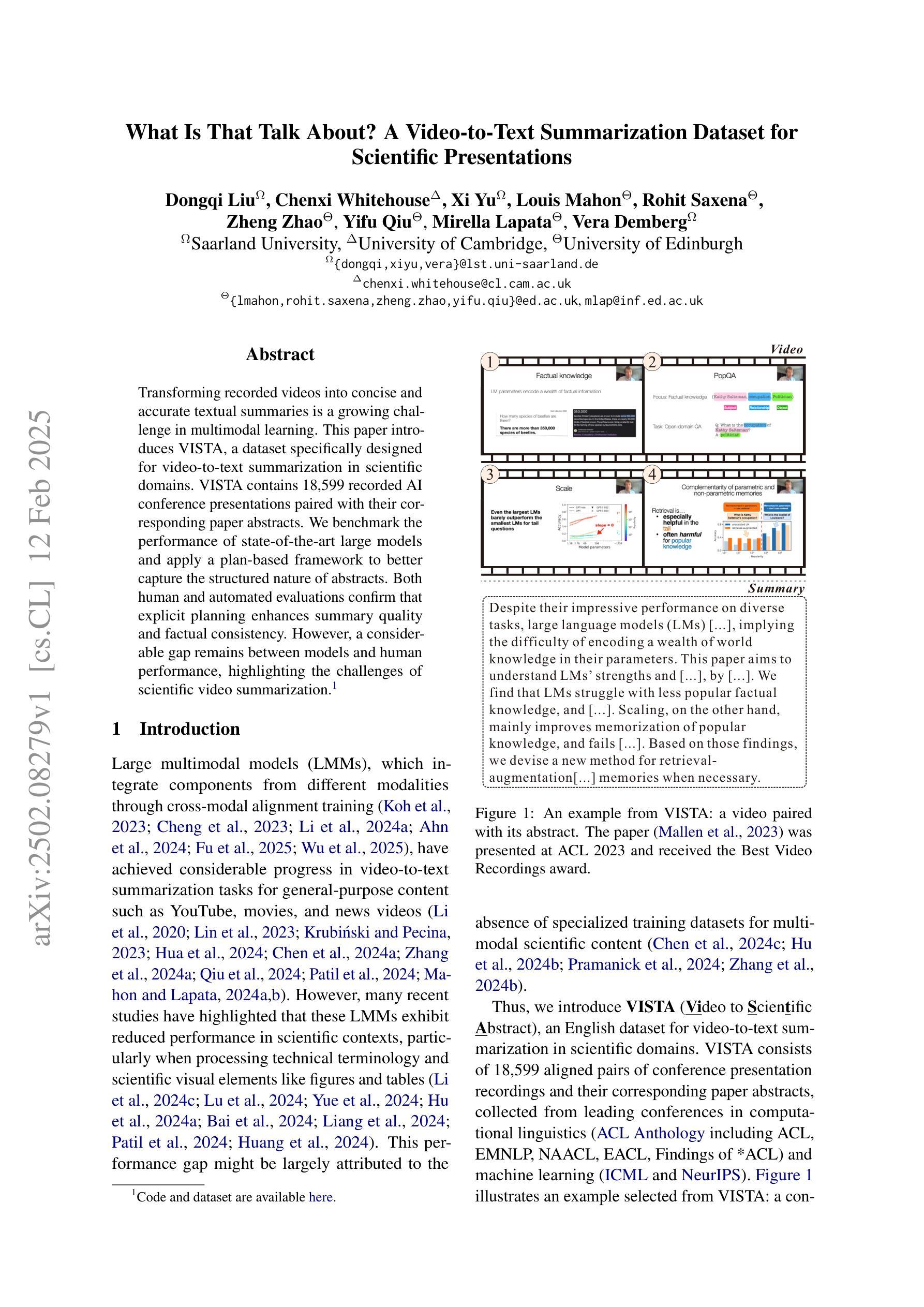
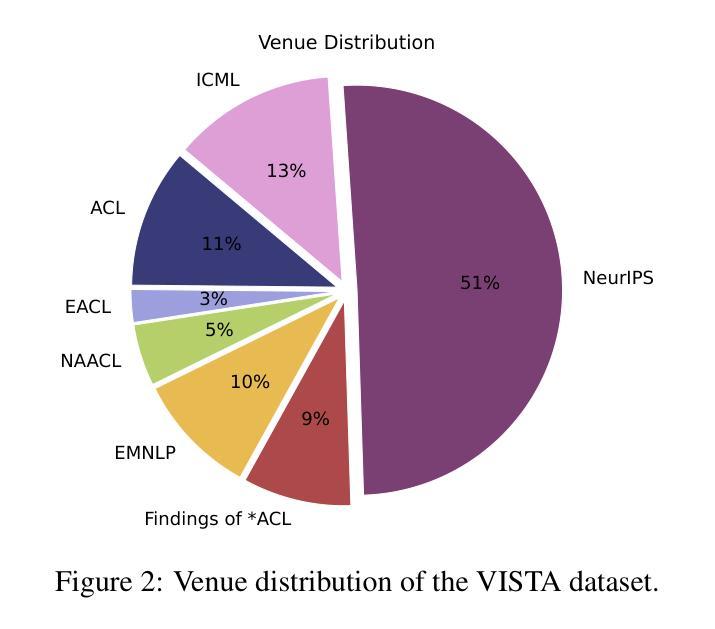
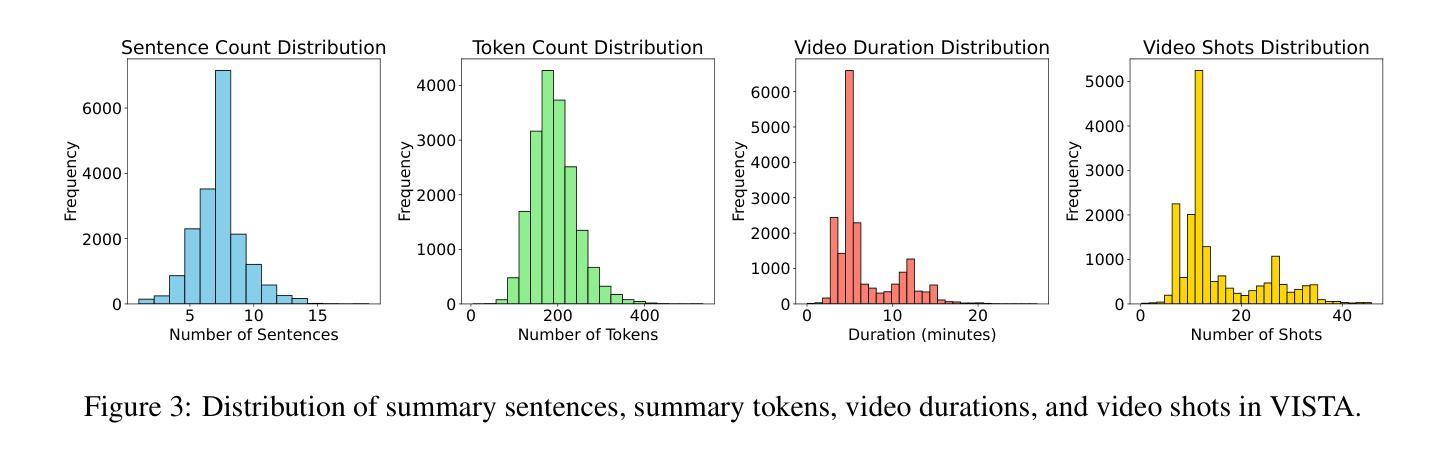
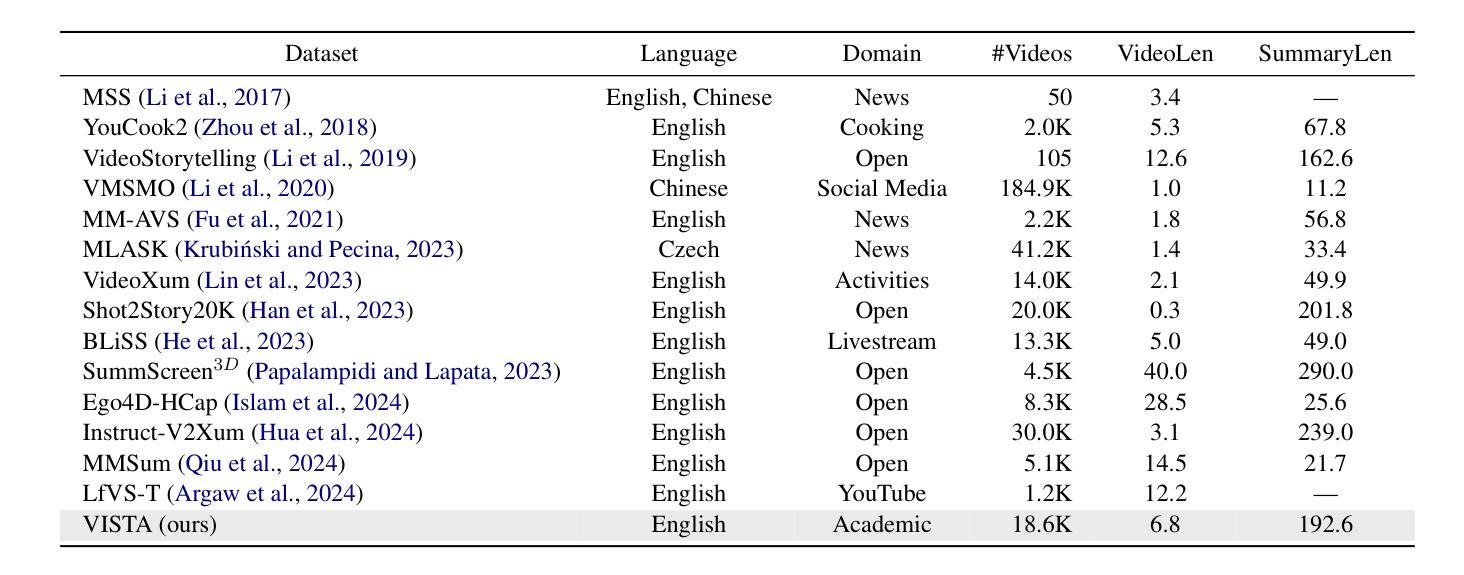
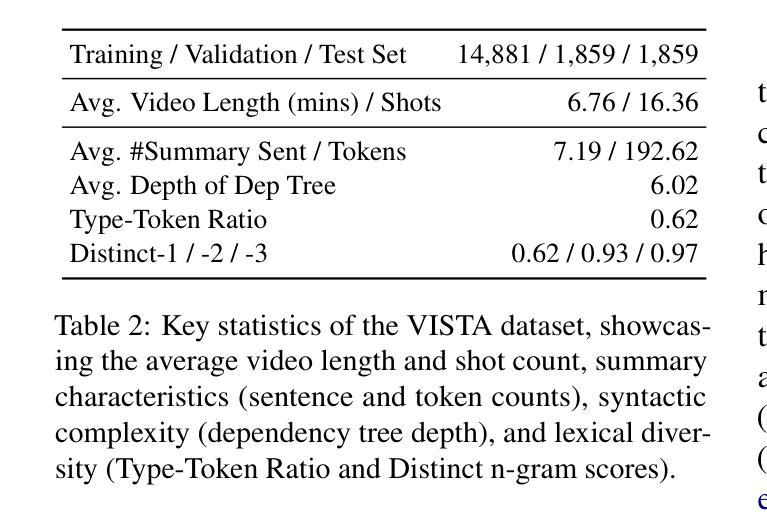
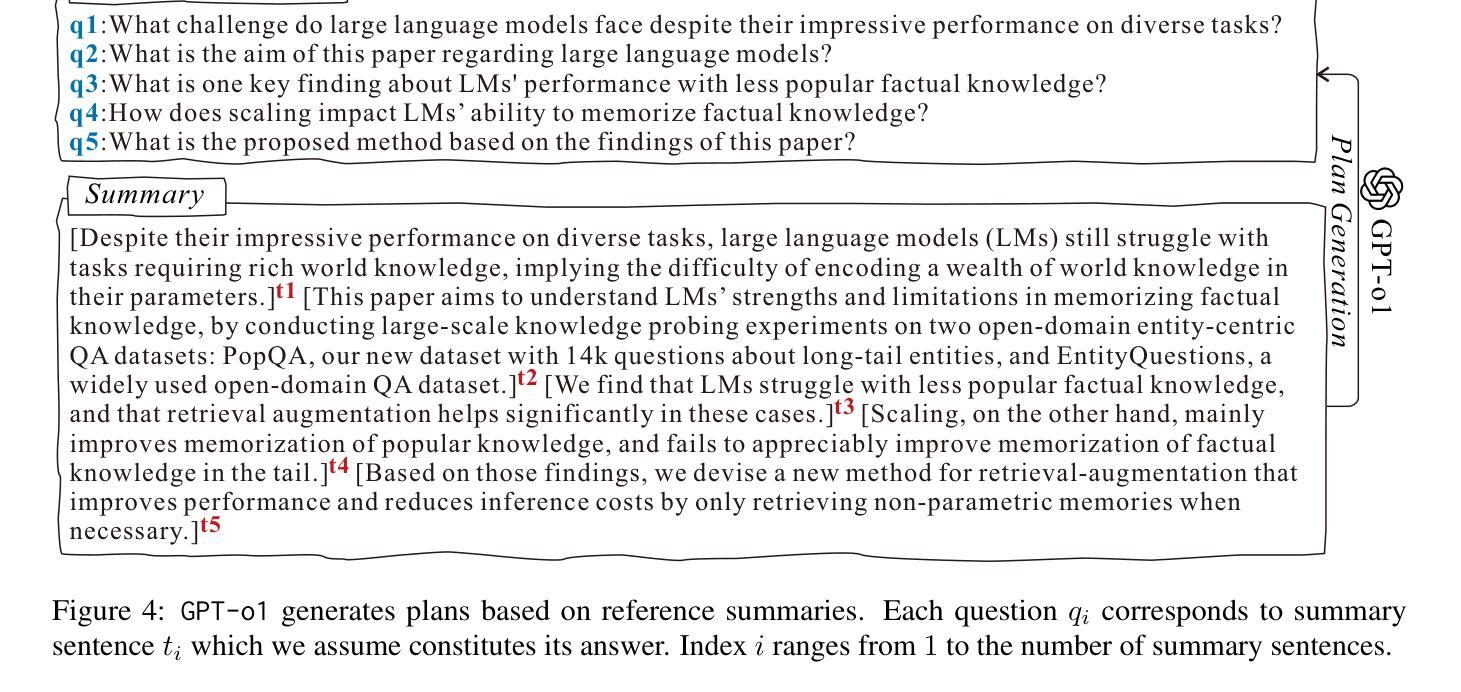
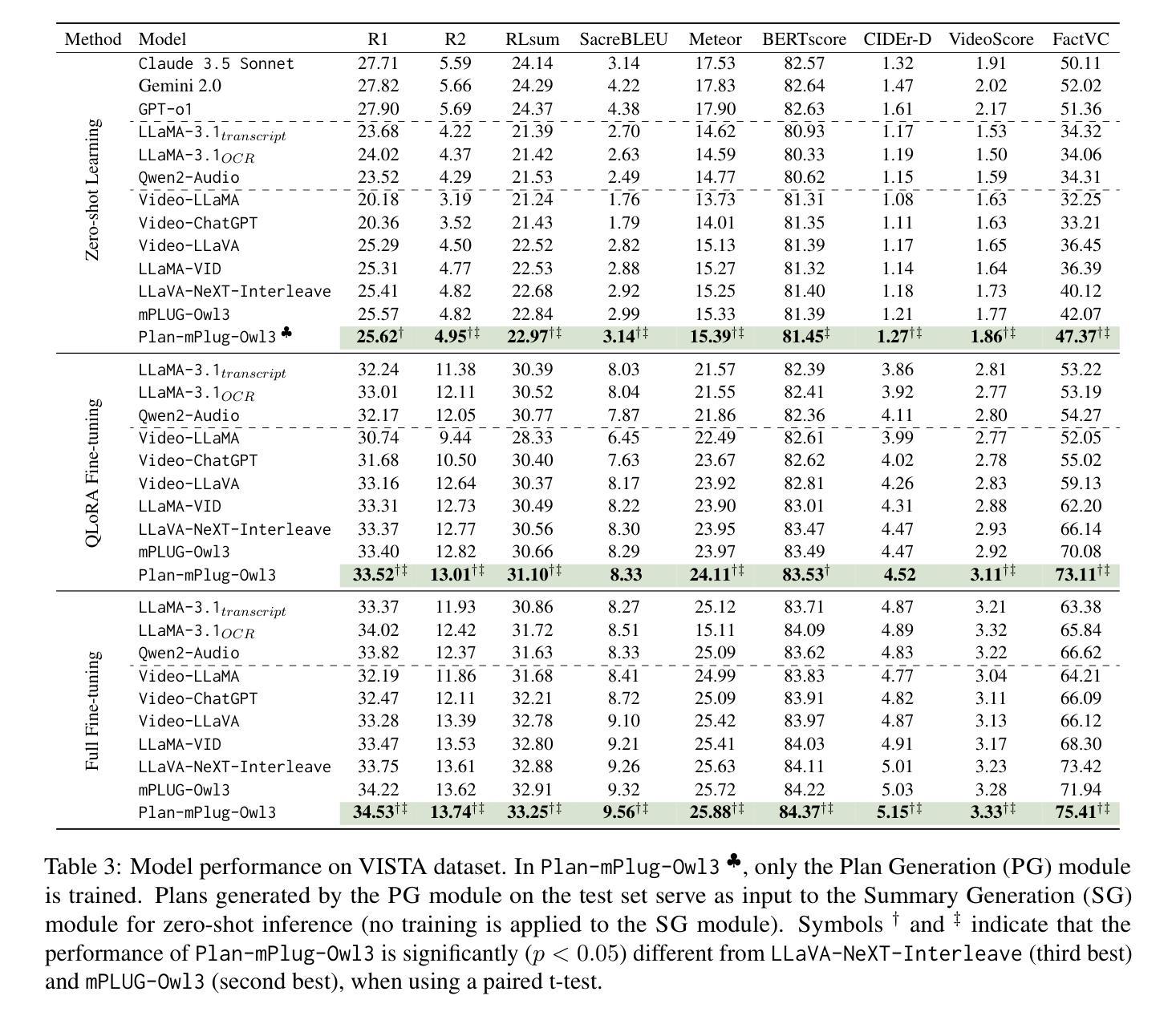
Playmate: Flexible Control of Portrait Animation via 3D-Implicit Space Guided Diffusion
Authors:Xingpei Ma, Jiaran Cai, Yuansheng Guan, Shenneng Huang, Qiang Zhang, Shunsi Zhang
Recent diffusion-based talking face generation models have demonstrated impressive potential in synthesizing videos that accurately match a speech audio clip with a given reference identity. However, existing approaches still encounter significant challenges due to uncontrollable factors, such as inaccurate lip-sync, inappropriate head posture and the lack of fine-grained control over facial expressions. In order to introduce more face-guided conditions beyond speech audio clips, a novel two-stage training framework Playmate is proposed to generate more lifelike facial expressions and talking faces. In the first stage, we introduce a decoupled implicit 3D representation along with a meticulously designed motion-decoupled module to facilitate more accurate attribute disentanglement and generate expressive talking videos directly from audio cues. Then, in the second stage, we introduce an emotion-control module to encode emotion control information into the latent space, enabling fine-grained control over emotions and thereby achieving the ability to generate talking videos with desired emotion. Extensive experiments demonstrate that Playmate outperforms existing state-of-the-art methods in terms of video quality and lip-synchronization, and improves flexibility in controlling emotion and head pose. The code will be available at https://playmate111.github.io.
近期基于扩散的说话人脸生成模型在合成视频方面展现出了令人印象深刻的潜力,能够准确地根据给定的参考身份与语音音频片段匹配。然而,现有方法仍然面临着由不可控因素带来的重大挑战,例如不准确的唇同步、不适当的头部姿势以及对面部表情的精细控制不足。为了引入除语音音频片段之外更多的面部引导条件,我们提出了一种新颖的两阶段训练框架Playmate,以生成更逼真的面部表情和说话人脸。在第一阶段,我们引入了一个解耦的隐式3D表示以及一个精心设计的运动解耦模块,以更准确地实现属性分解,并直接从音频线索生成富有表现力的说话视频。然后,在第二阶段,我们引入了一个情感控制模块,将情感控制信息编码到潜在空间,实现对情感的精细控制,从而能够生成具有所需情感的说话视频。大量实验表明,Playmate在视频质量和唇同步方面超越了现有的最先进的方法,并在控制情感和头部姿势方面提高了灵活性。代码将在https://playmate111.github.io上提供。
论文及项目相关链接
Summary
本文介绍了一种名为Playmate的新型两阶段训练框架,用于生成更逼真的面部表情和说话面部。第一阶段引入了解耦隐式3D表示和精心设计的运动解耦模块,以更准确地进行属性分解,并直接从音频线索生成表情丰富的说话视频。第二阶段引入情感控制模块,将情感控制信息编码到潜在空间,实现对情感的精细控制,从而生成具有所需情感的说话视频。该框架在视频质量和唇同步方面优于现有先进方法,并提高了对情感和头部姿势的控制灵活性。
Key Takeaways
- Playmate是一种新型的两阶段训练框架,用于生成与语音音频剪辑匹配的说话面部视频。
- 第一阶段引入了解耦隐式3D表示和运动解耦模块,实现更准确的属性分解和表情丰富的说话视频生成。
- 第二阶段引入了情感控制模块,使模型能够生成具有特定情感的说话视频,并对情感和头部姿势实现更精细的控制。
- Playmate在视频质量和唇同步方面优于现有方法。
- 该框架的代码将在https://playmate111.github.io上提供。
- Playmate框架有助于合成更逼真的面部表情和谈话视频。
点此查看论文截图
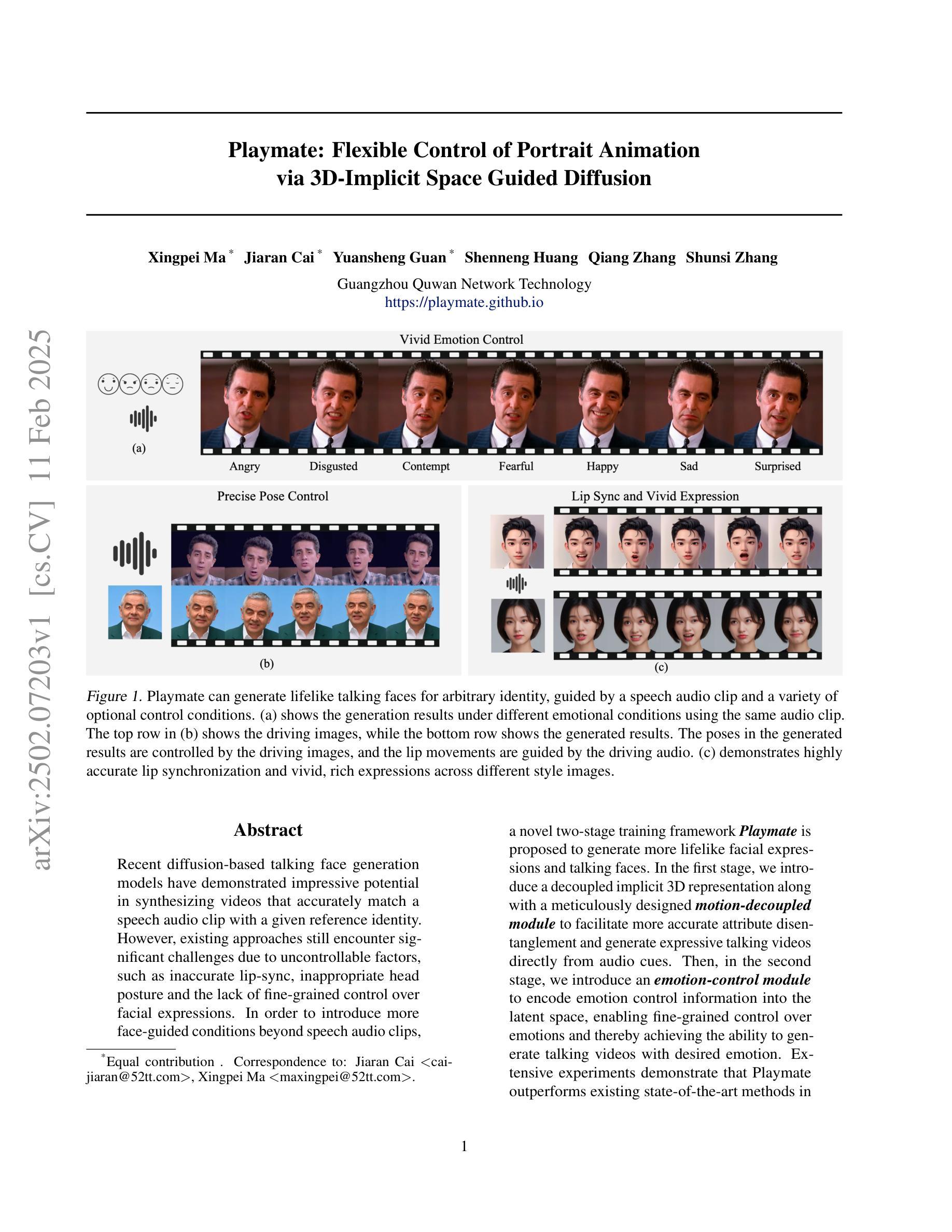
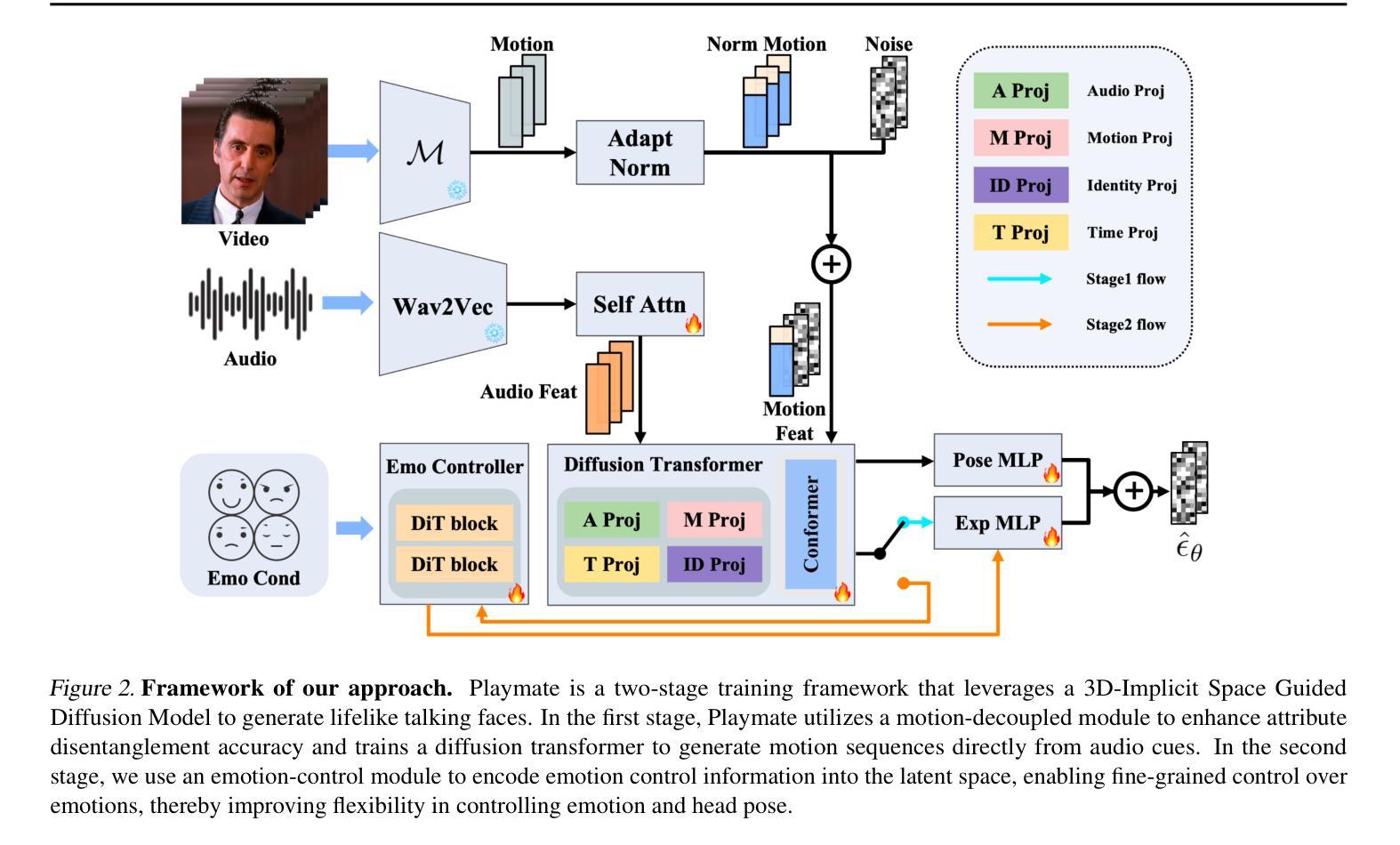
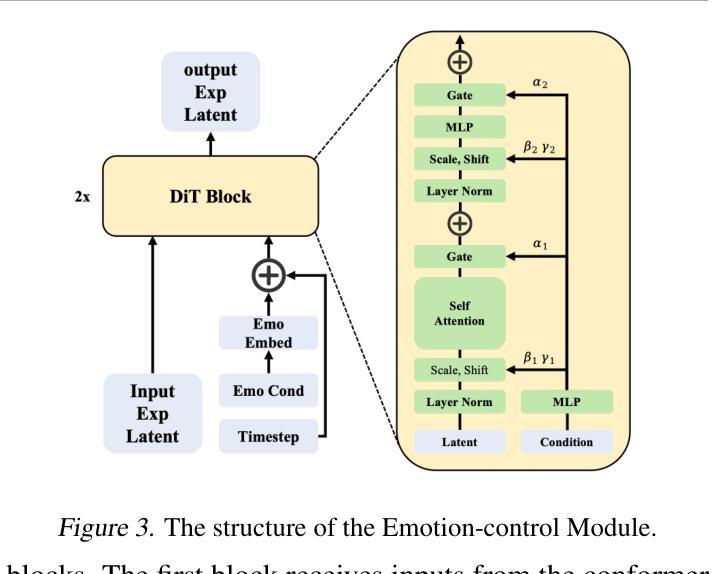
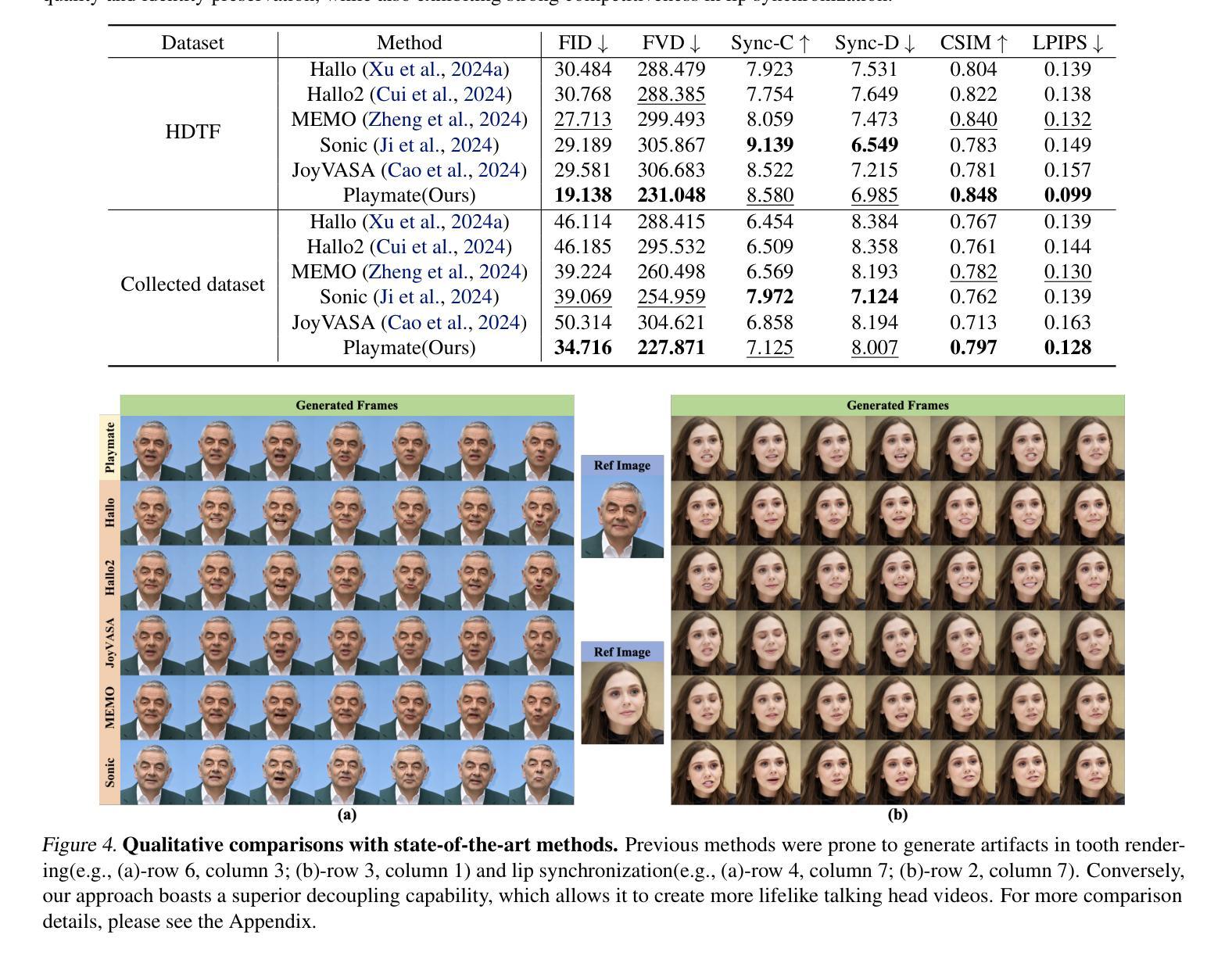