⚠️ 以下所有内容总结都来自于 大语言模型的能力,如有错误,仅供参考,谨慎使用
🔴 请注意:千万不要用于严肃的学术场景,只能用于论文阅读前的初筛!
💗 如果您觉得我们的项目对您有帮助 ChatPaperFree ,还请您给我们一些鼓励!⭐️ HuggingFace免费体验
2025-02-14 更新
SAM-DiffSR: Structure-Modulated Diffusion Model for Image Super-Resolution
Authors:Chengcheng Wang, Zhiwei Hao, Yehui Tang, Jianyuan Guo, Yujie Yang, Kai Han, Yunhe Wang
Diffusion-based super-resolution (SR) models have recently garnered significant attention due to their potent restoration capabilities. But conventional diffusion models perform noise sampling from a single distribution, constraining their ability to handle real-world scenes and complex textures across semantic regions. With the success of segment anything model (SAM), generating sufficiently fine-grained region masks can enhance the detail recovery of diffusion-based SR model. However, directly integrating SAM into SR models will result in much higher computational cost. In this paper, we propose the SAM-DiffSR model, which can utilize the fine-grained structure information from SAM in the process of sampling noise to improve the image quality without additional computational cost during inference. In the process of training, we encode structural position information into the segmentation mask from SAM. Then the encoded mask is integrated into the forward diffusion process by modulating it to the sampled noise. This adjustment allows us to independently adapt the noise mean within each corresponding segmentation area. The diffusion model is trained to estimate this modulated noise. Crucially, our proposed framework does NOT change the reverse diffusion process and does NOT require SAM at inference. Experimental results demonstrate the effectiveness of our proposed method, showcasing superior performance in suppressing artifacts, and surpassing existing diffusion-based methods by 0.74 dB at the maximum in terms of PSNR on DIV2K dataset. The code and dataset are available at https://github.com/lose4578/SAM-DiffSR.
基于扩散的超分辨率(SR)模型由于其强大的恢复能力而最近引起了广泛关注。但是,传统的扩散模型从单一分布中进行噪声采样,这限制了它们处理真实场景和跨语义区域的复杂纹理的能力。随着Segment Anything Model(SAM)的成功,生成足够精细的粒度区域掩码可以增强基于扩散的SR模型的细节恢复能力。然而,直接将SAM集成到SR模型中会导致更高的计算成本。在本文中,我们提出了SAM-DiffSR模型,该模型可以在采样噪声的过程中利用SAM的精细结构信息,以提高图像质量,而在推理过程中不需要额外的计算成本。在训练过程中,我们将结构位置信息编码到SAM的分割掩码中。然后,将编码的掩码通过调制采样噪声集成到正向扩散过程中。这种调整允许我们独立地调整每个对应分割区域内的噪声均值。扩散模型经过训练以估计这种调制后的噪声。关键的是,我们提出的框架并不改变反向扩散过程,并且在推理过程中并不需要SAM。实验结果表明,我们的方法有效,在抑制伪影方面表现出卓越的性能,在DIV2K数据集上,与现有的基于扩散的方法相比,最高提高了0.74 dB的PSNR。代码和数据集可在https://github.com/lose4578/SAM-DiffSR获得。
论文及项目相关链接
摘要
扩散模型为基础的超分辨率(SR)模型因其强大的修复能力而备受关注。然而,传统的扩散模型从单一分布中进行噪声采样,限制了它们处理真实场景和跨语义区域的复杂纹理的能力。借助成功分割任何模型(SAM)的优势,生成足够精细的粒度的区域掩膜可以增强扩散式SR模型的细节恢复能力。然而,直接将SAM集成到SR模型中会导致更高的计算成本。本文提出了SAM-DiffSR模型,该模型可以在采样噪声的过程中利用SAM的精细结构信息,以提高图像质量,而无需在推理过程中增加计算成本。在训练过程中,我们将结构位置信息编码到SAM的分割掩膜中。然后,将编码后的掩膜通过调制采样噪声集成到正向扩散过程中。这种调整允许我们独立地调整每个相应分割区域内的噪声均值。扩散模型经过训练以估计这种调制后的噪声。关键的是,我们提出的框架并不会改变反向扩散过程,并且在推理过程中并不需要SAM。实验结果证明了我们的方法的有效性,在抑制伪影方面表现出优势,在DIV2K数据集上,以峰值信噪比(PSNR)衡量,我们的方法最多可提高0.74分贝。代码和数据集可在https://github.com/lose4578/SAM-DiffSR上找到。
要点归纳
- 扩散模型为基础的超分辨率模型受到关注,但传统方法存在处理复杂纹理和真实场景的局限性。
- 利用成功分割任何模型(SAM)的精细结构信息可以提高图像质量。
- 提出SAM-DiffSR模型,结合SAM的精细结构信息在噪声采样过程中,提高图像质量,且推理过程中无需增加计算成本。
- 训练过程中编码结构位置信息到SAM分割掩膜中,并集成到正向扩散过程中。
- 该框架独立适应每个分割区域内的噪声均值,提高模型估计调制噪声的能力。
- 提出的方法在抑制伪影方面表现出优势,并在DIV2K数据集上实现了性能提升。
点此查看论文截图
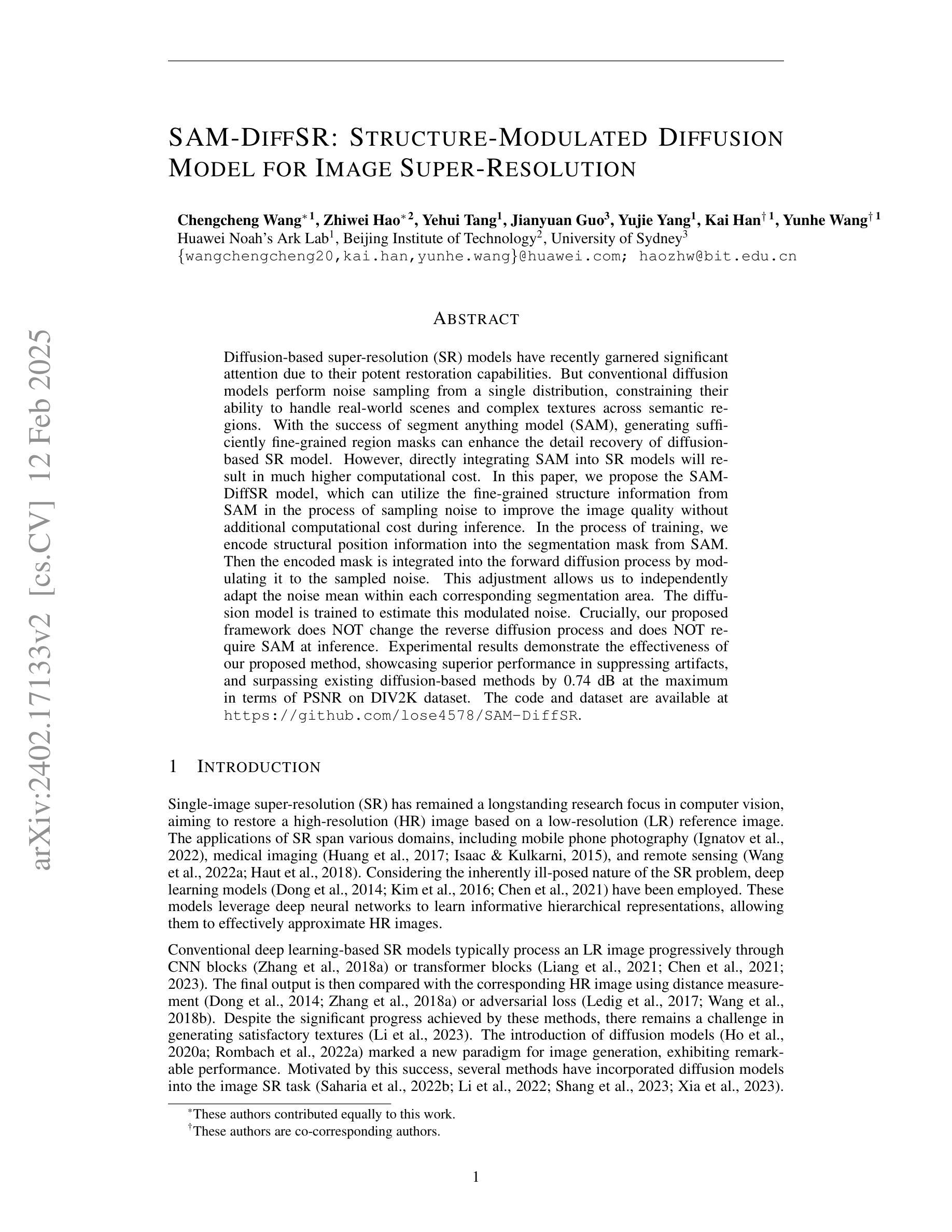
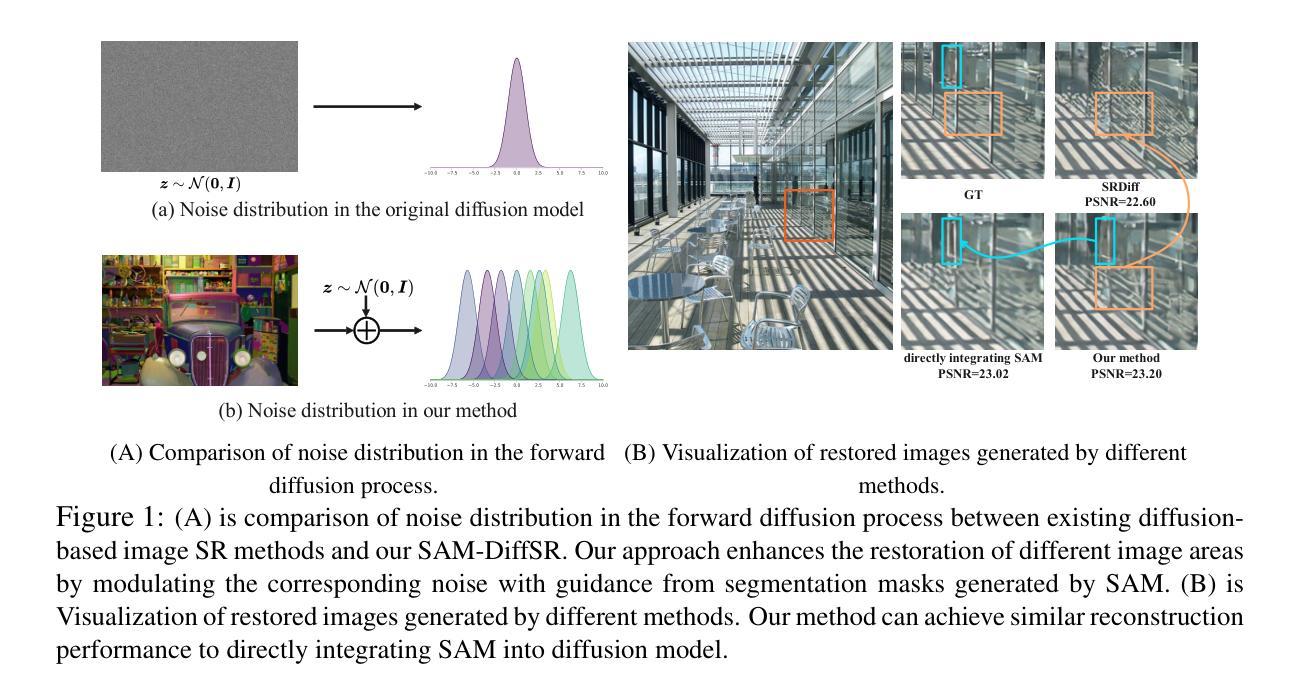
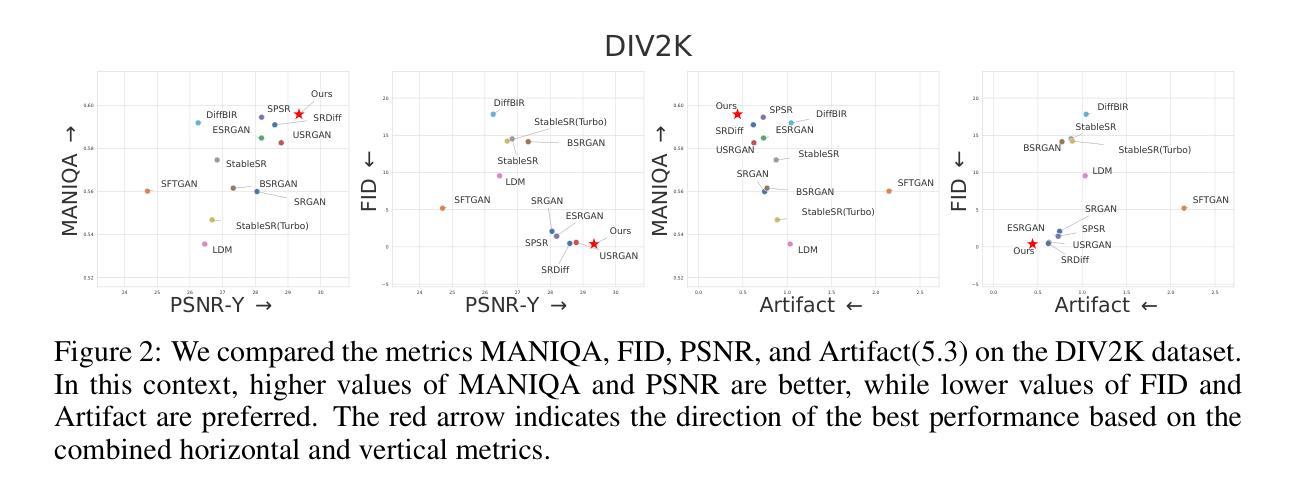
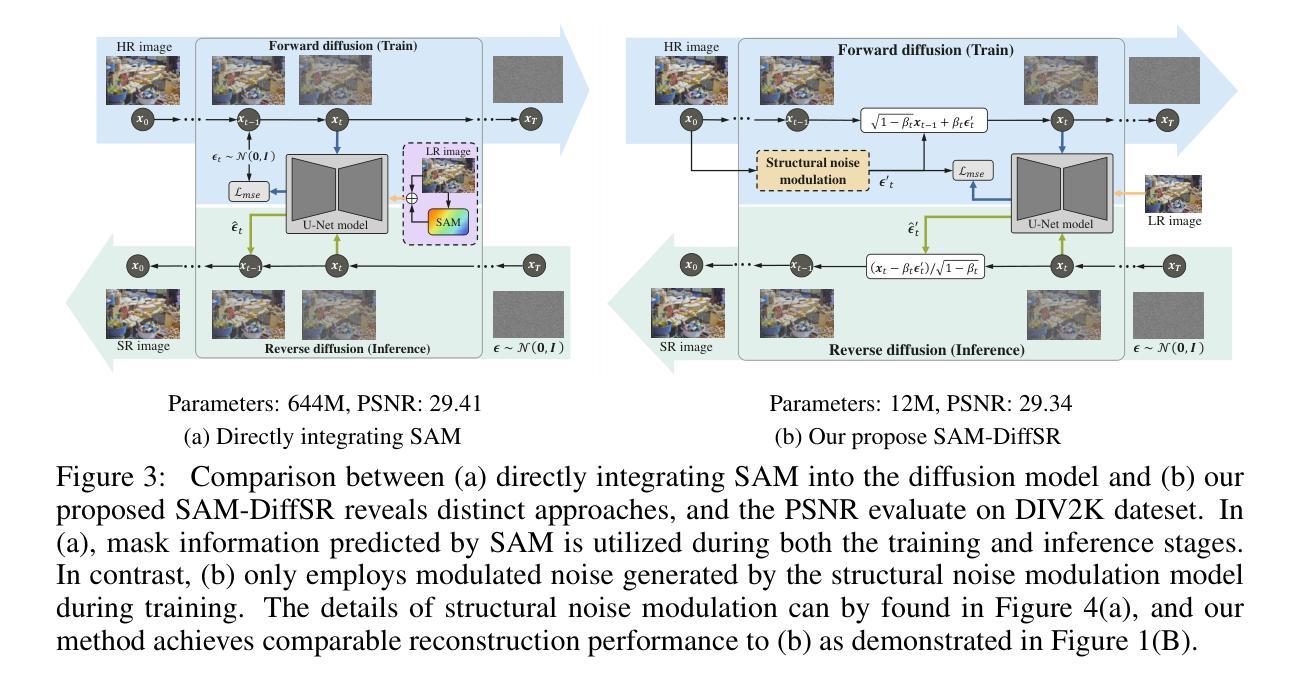
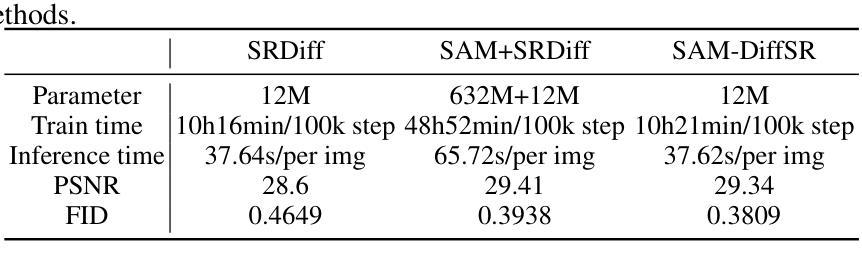
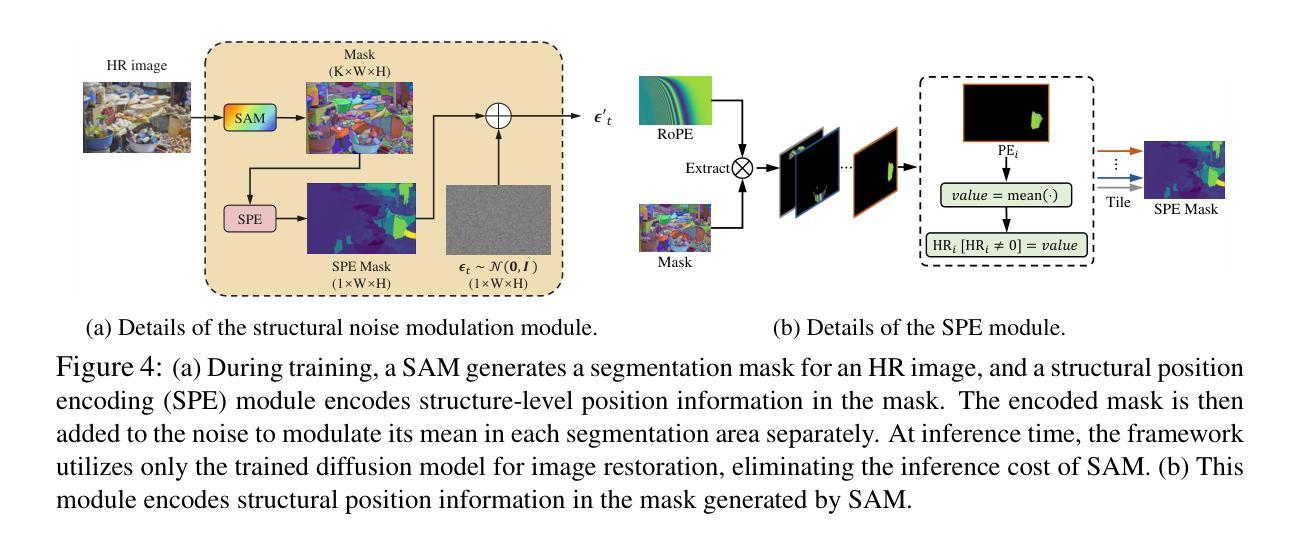