⚠️ 以下所有内容总结都来自于 大语言模型的能力,如有错误,仅供参考,谨慎使用
🔴 请注意:千万不要用于严肃的学术场景,只能用于论文阅读前的初筛!
💗 如果您觉得我们的项目对您有帮助 ChatPaperFree ,还请您给我们一些鼓励!⭐️ HuggingFace免费体验
2025-02-14 更新
Contextual Gesture: Co-Speech Gesture Video Generation through Context-aware Gesture Representation
Authors:Pinxin Liu, Pengfei Zhang, Hyeongwoo Kim, Pablo Garrido, Ari Sharpio, Kyle Olszewski
Co-speech gesture generation is crucial for creating lifelike avatars and enhancing human-computer interactions by synchronizing gestures with speech. Despite recent advancements, existing methods struggle with accurately identifying the rhythmic or semantic triggers from audio for generating contextualized gesture patterns and achieving pixel-level realism. To address these challenges, we introduce Contextual Gesture, a framework that improves co-speech gesture video generation through three innovative components: (1) a chronological speech-gesture alignment that temporally connects two modalities, (2) a contextualized gesture tokenization that incorporate speech context into motion pattern representation through distillation, and (3) a structure-aware refinement module that employs edge connection to link gesture keypoints to improve video generation. Our extensive experiments demonstrate that Contextual Gesture not only produces realistic and speech-aligned gesture videos but also supports long-sequence generation and video gesture editing applications, shown in Fig.1 Project Page: https://andypinxinliu.github.io/Contextual-Gesture/.
协同语音手势生成对于创建逼真的虚拟人物以及通过同步手势和语音来增强人机交互至关重要。尽管最近有进展,但现有方法在准确识别音频中的节奏或语义触发因素以生成上下文化的手势模式并实现像素级的真实性方面仍存在困难。为了解决这些挑战,我们引入了“上下文手势”框架,该框架通过三个创新组件改进了协同语音手势视频生成:(1)时序语音-手势对齐,在时间上连接两种模式;(2)上下文手势符号化,通过蒸馏将语音上下文融入运动模式表示;以及(3)结构感知细化模块,采用边缘连接将手势关键点连接起来,以提高视频生成质量。我们的广泛实验表明,“上下文手势”不仅生成了真实且语音同步的手势视频,还支持长序列生成和视频手势编辑应用程序,如图1所示。项目页面:https://andypinxinliu.github.io/Contextual-Gesture/。
论文及项目相关链接
Summary
本文介绍了针对协同语音手势生成的技术挑战,提出一种名为“Contextual Gesture”的框架,通过三个创新组件实现精准的手势与语音同步、结合语境的手势符号化表示以及结构感知的细化模块,从而提高协同语音手势视频生成的真实感和语境匹配度。
Key Takeaways
- 协同语音手势生成对于创建逼真虚拟形象和提高人机交互至关重要。
- 现有方法在准确识别音频中的节奏或语义触发因素以生成上下文化的手势模式方面存在挑战。
- Contextual Gesture框架通过三个创新组件解决这些问题:时间连接的语音-手势对齐、结合语境的手势符号化表示以及结构感知的细化模块。
- 该框架能产生真实、与语音同步的手势视频。
- 支持长序列生成和视频手势编辑应用。
- Contextual Gesture框架有助于提高手势视频生成的真实感和互动性。
点此查看论文截图
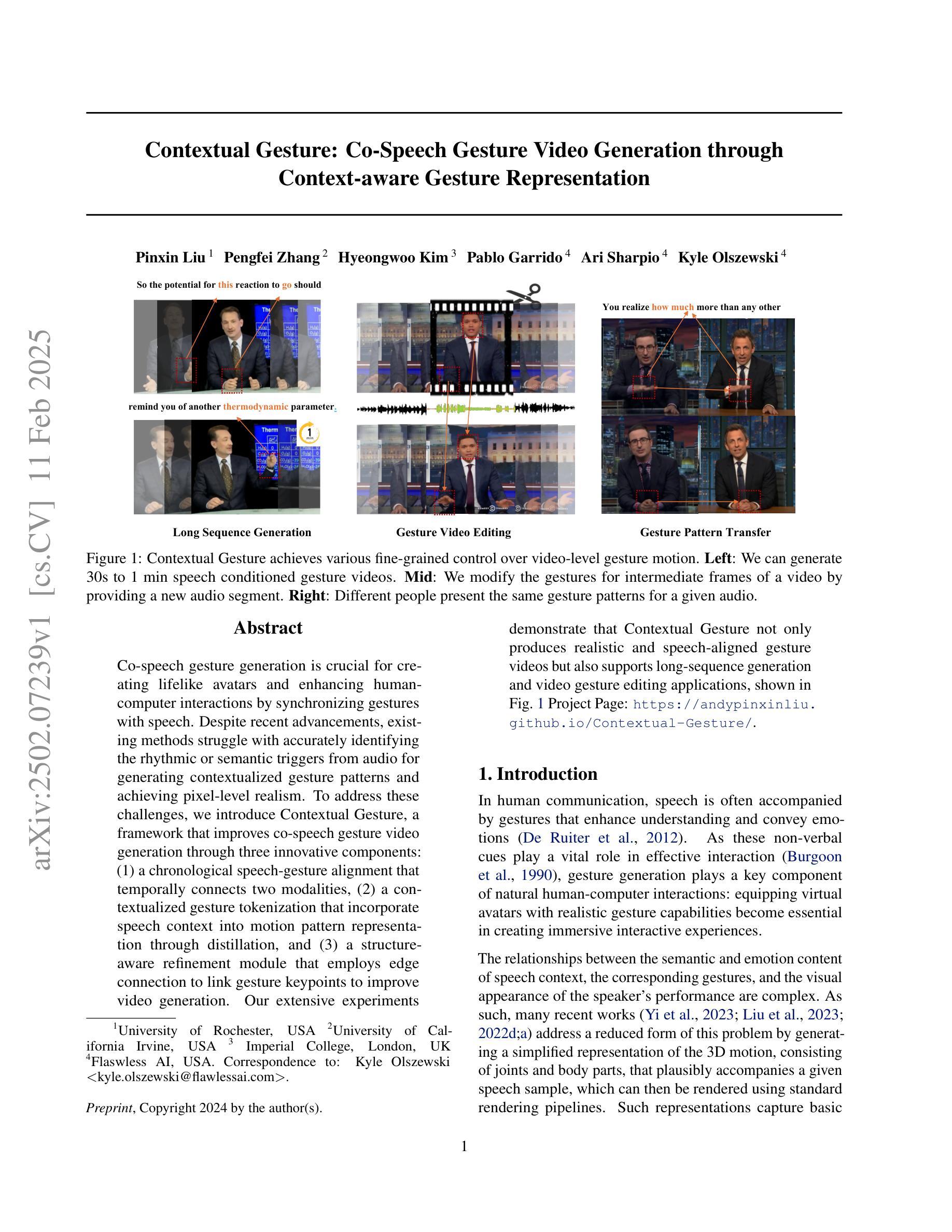
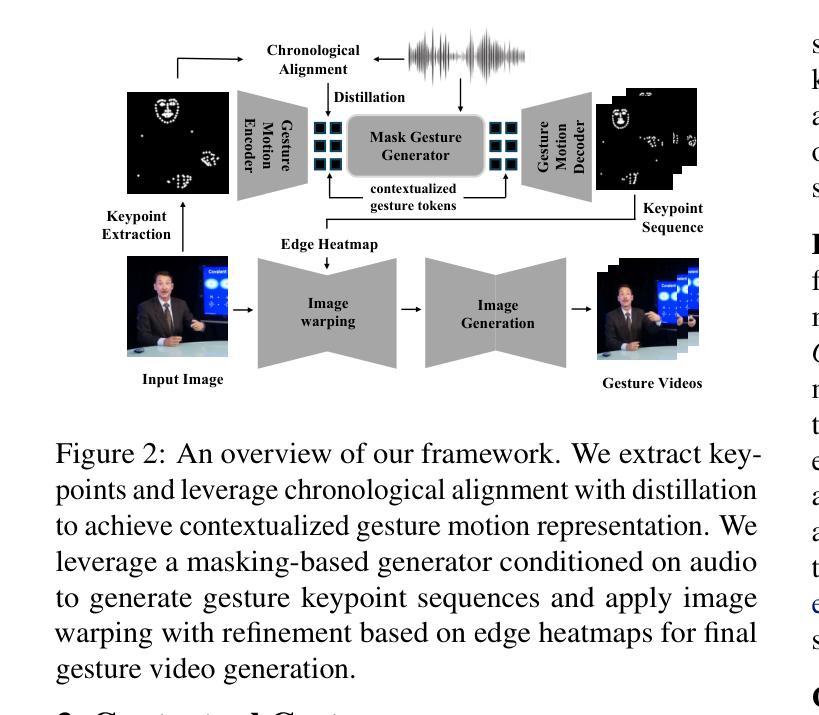
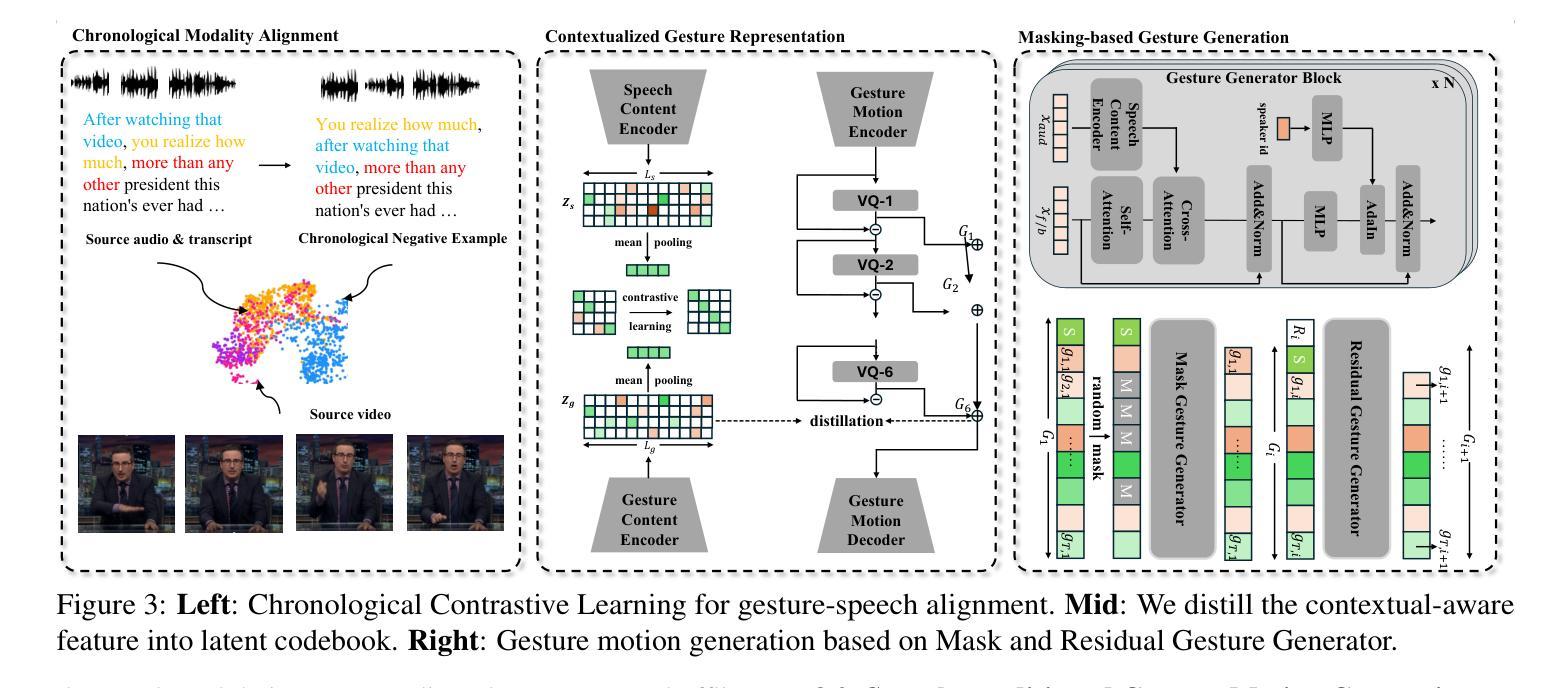
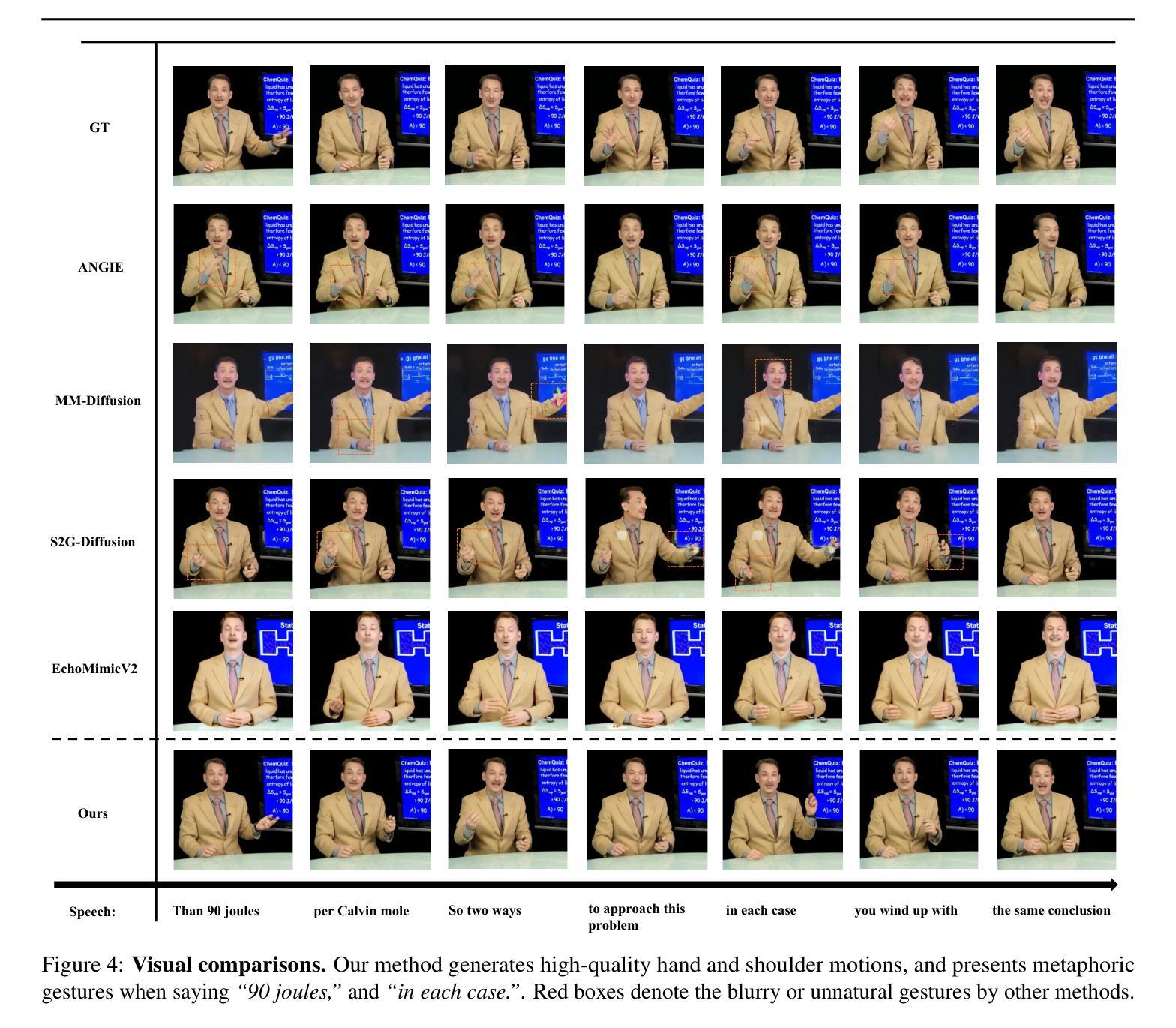
Learning Source Disentanglement in Neural Audio Codec
Authors:Xiaoyu Bie, Xubo Liu, Gaël Richard
Neural audio codecs have significantly advanced audio compression by efficiently converting continuous audio signals into discrete tokens. These codecs preserve high-quality sound and enable sophisticated sound generation through generative models trained on these tokens. However, existing neural codec models are typically trained on large, undifferentiated audio datasets, neglecting the essential discrepancies between sound domains like speech, music, and environmental sound effects. This oversight complicates data modeling and poses additional challenges to the controllability of sound generation. To tackle these issues, we introduce the Source-Disentangled Neural Audio Codec (SD-Codec), a novel approach that combines audio coding and source separation. By jointly learning audio resynthesis and separation, SD-Codec explicitly assigns audio signals from different domains to distinct codebooks, sets of discrete representations. Experimental results indicate that SD-Codec not only maintains competitive resynthesis quality but also, supported by the separation results, demonstrates successful disentanglement of different sources in the latent space, thereby enhancing interpretability in audio codec and providing potential finer control over the audio generation process.
神经网络音频编解码器通过有效地将连续的音频信号转换为离散令牌,在音频压缩方面取得了重大进展。这些编解码器保留了高质量的音效,并通过在这些令牌上进行训练的生成模型实现了复杂的音效生成。然而,现有的神经网络编解码器模型通常在大规模、未分类的音频数据集上进行训练,忽略了语音、音乐和环境音效等声音领域之间的基本差异。这种疏忽使得数据建模变得复杂,并给声音生成的可控性带来了额外的挑战。为了解决这些问题,我们引入了源分离神经网络音频编解码器(SD编解码器),这是一种将音频编码和源分离相结合的新方法。通过联合学习音频再合成和分离,SD编解码器显式地将来自不同领域的音频信号分配给不同的代码簿,即一组离散表示。实验结果表明,SD编解码器不仅保持了具有竞争力的再合成质量,而且在分离结果的支撑下,在潜在空间中成功地解开了不同的来源,从而提高了音频编解码的可解释性,并为音频生成过程提供了更精细的控制潜力。
论文及项目相关链接
PDF ICASSP 2025, project page: https://xiaoyubie1994.github.io/sdcodec/
Summary
神经网络音频编码解码器通过高效地将连续音频信号转换为离散令牌,显著推动了音频压缩的发展。然而,现有神经网络编码解码器通常在大规模、无差别的音频数据集上进行训练,忽略了不同声音域(如语音、音乐和环境音效)之间的差异。为解决这一问题,我们提出了源分离神经网络音频编码解码器(SD-Codec),它结合了音频编码和源分离。SD-Codec不仅能维持高效的重新合成质量,还通过在潜在空间内成功解开不同源,增强了音频编码的可解释性,并为音频生成过程提供了更精细的控制。
Key Takeaways
- 神经网络音频编码解码器可将连续音频信号转换为离散令牌,推动音频压缩的进步。
- 现有神经网络编码解码器忽略了不同声音域之间的差异,导致数据建模复杂且音频生成控制困难。
- SD-Codec结合音频编码和源分离,旨在解决上述问题。
- SD-Codec通过联合学习音频重新合成和分离,为不同声音域分配特定的码本。
- 实验结果表明,SD-Codec在保持重新合成质量的同时,成功解开不同源,增强了可解释性。
- SD-Codec有助于更精细地控制音频生成过程。
点此查看论文截图
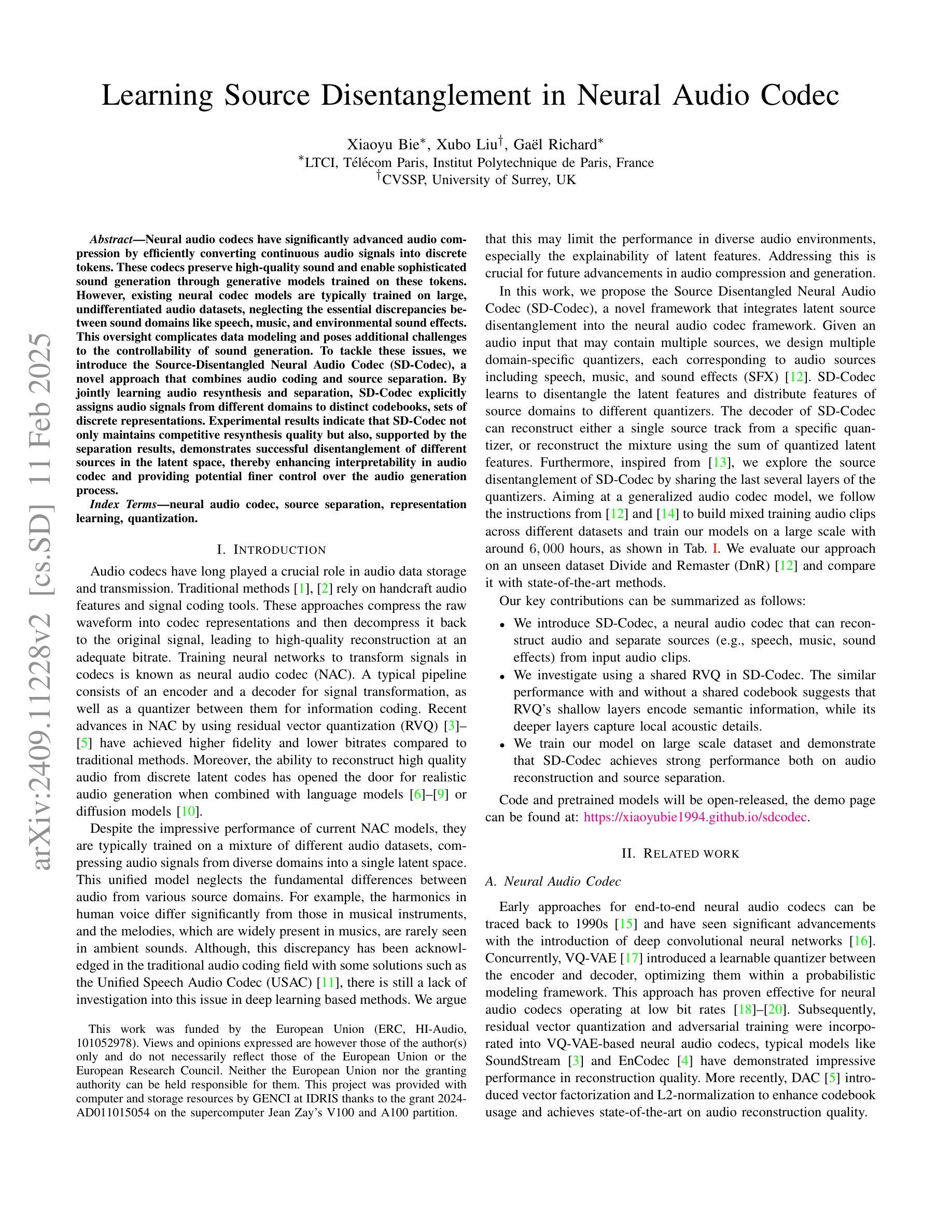
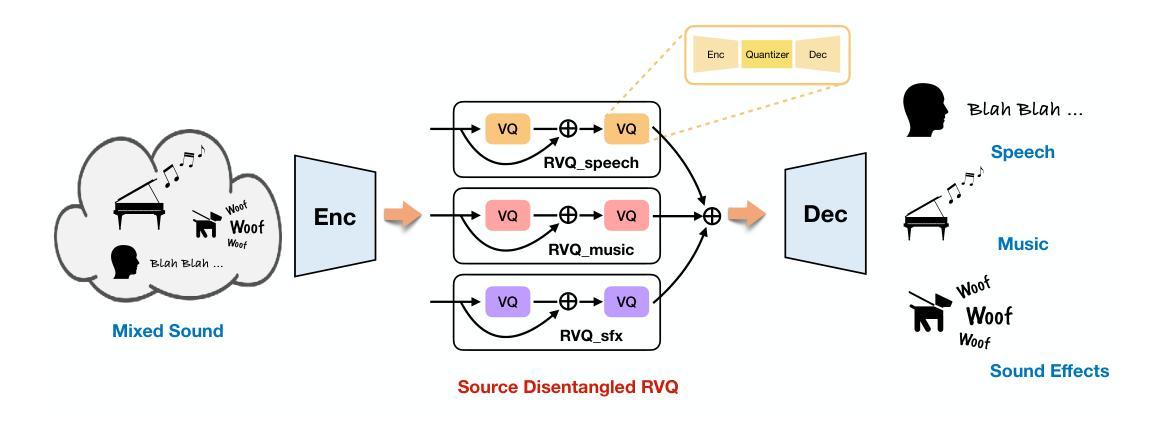
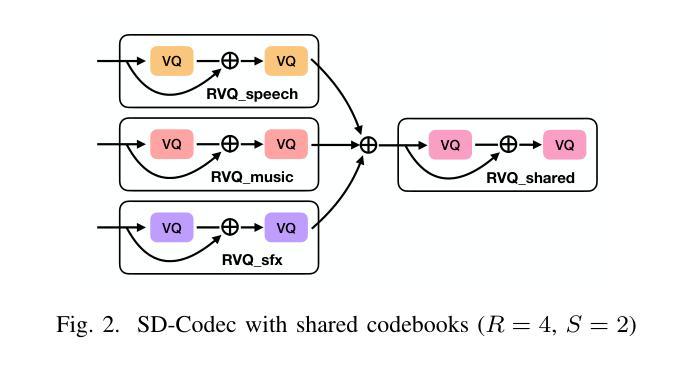
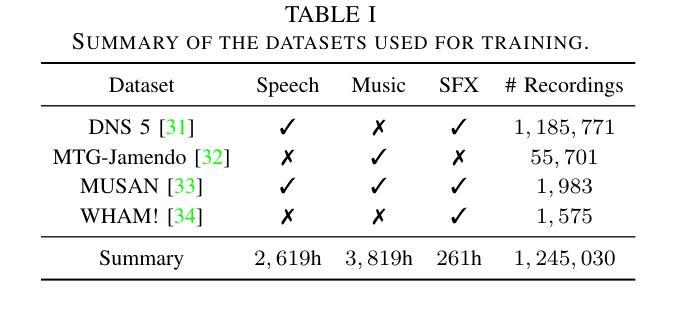
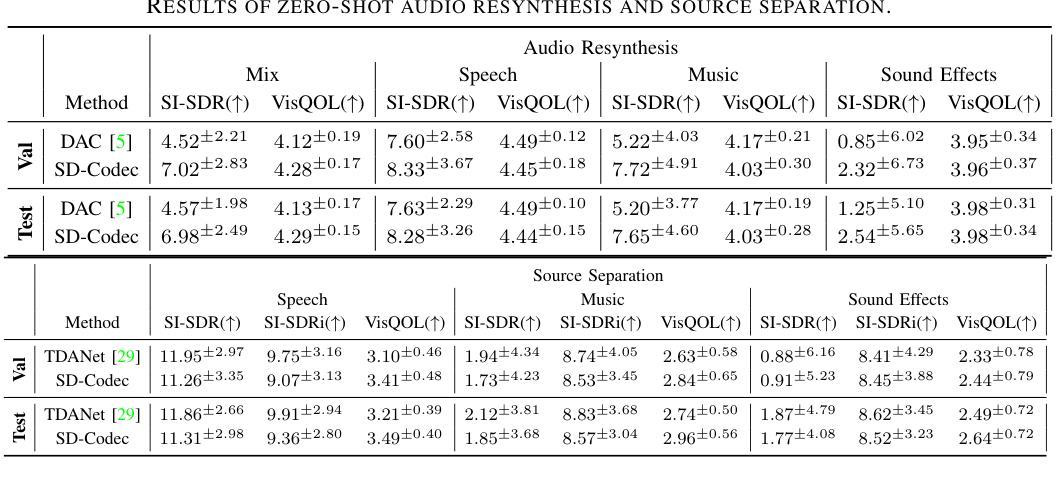
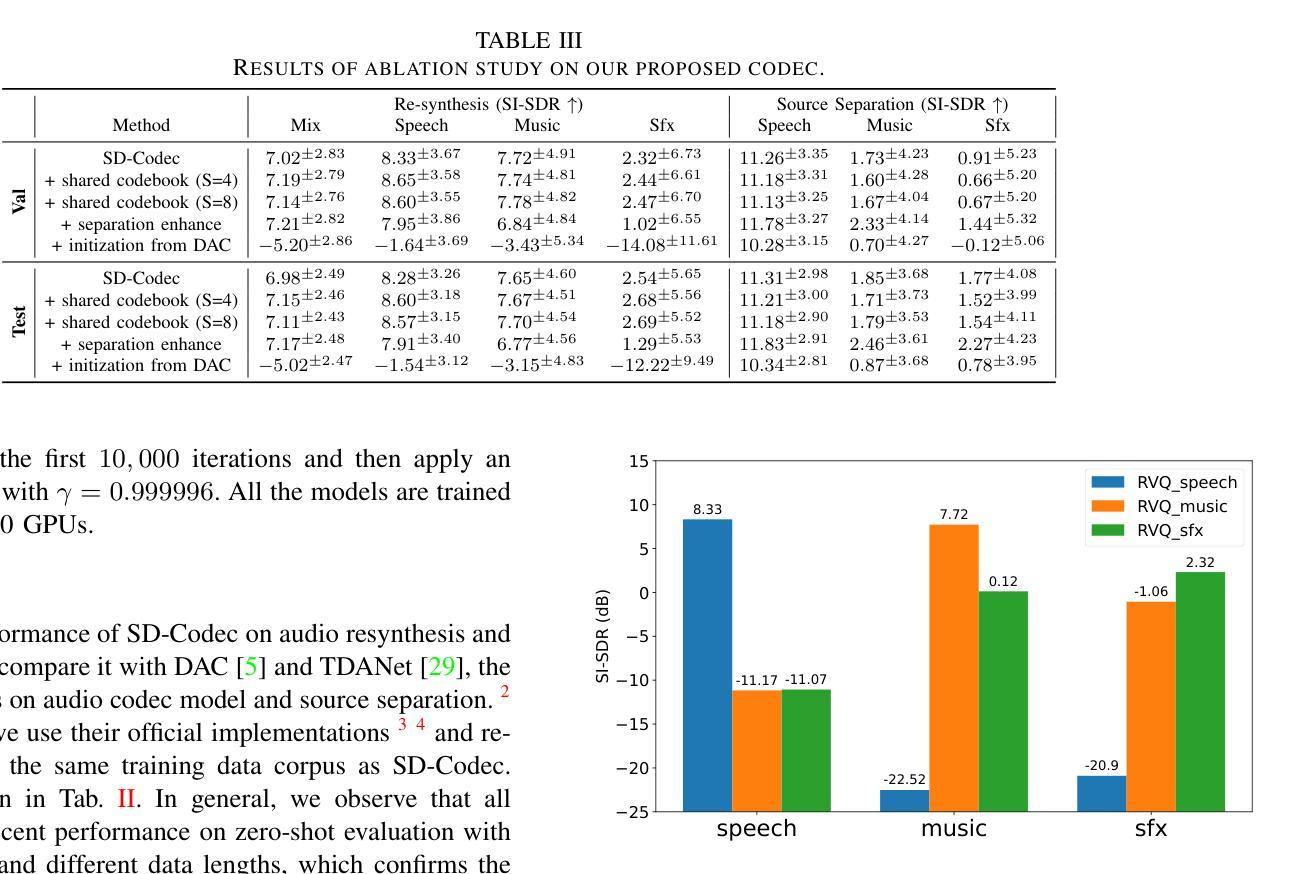