⚠️ 以下所有内容总结都来自于 大语言模型的能力,如有错误,仅供参考,谨慎使用
🔴 请注意:千万不要用于严肃的学术场景,只能用于论文阅读前的初筛!
💗 如果您觉得我们的项目对您有帮助 ChatPaperFree ,还请您给我们一些鼓励!⭐️ HuggingFace免费体验
2025-02-17 更新
OmniHuman-1: Rethinking the Scaling-Up of One-Stage Conditioned Human Animation Models
Authors:Gaojie Lin, Jianwen Jiang, Jiaqi Yang, Zerong Zheng, Chao Liang
End-to-end human animation, such as audio-driven talking human generation, has undergone notable advancements in the recent few years. However, existing methods still struggle to scale up as large general video generation models, limiting their potential in real applications. In this paper, we propose OmniHuman, a Diffusion Transformer-based framework that scales up data by mixing motion-related conditions into the training phase. To this end, we introduce two training principles for these mixed conditions, along with the corresponding model architecture and inference strategy. These designs enable OmniHuman to fully leverage data-driven motion generation, ultimately achieving highly realistic human video generation. More importantly, OmniHuman supports various portrait contents (face close-up, portrait, half-body, full-body), supports both talking and singing, handles human-object interactions and challenging body poses, and accommodates different image styles. Compared to existing end-to-end audio-driven methods, OmniHuman not only produces more realistic videos, but also offers greater flexibility in inputs. It also supports multiple driving modalities (audio-driven, video-driven and combined driving signals). Video samples are provided on the ttfamily project page (https://omnihuman-lab.github.io)
近年来,端到端的人脸动画(如音频驱动的人脸生成)取得了显著的进步。然而,现有方法仍然难以扩展为大型通用视频生成模型,从而限制了其在真实应用中的潜力。在本文中,我们提出了OmniHuman,这是一个基于Diffusion Transformer的框架,通过混合运动相关条件来扩大训练阶段的数据规模。为此,我们介绍了两种针对这些混合条件的训练原则,以及相应的模型结构和推理策略。这些设计使OmniHuman能够充分利用数据驱动的运动生成,最终实现高度逼真的人类视频生成。更重要的是,OmniHuman支持各种肖像内容(面部特写、肖像、半身、全身),支持说话和唱歌,处理人与物体的互动以及具有挑战性的身体姿势,并适应不同的图像风格。与现有的端到端音频驱动方法相比,OmniHuman不仅生成更逼真的视频,而且输入更具灵活性。它还支持多种驱动模式(音频驱动、视频驱动和组合驱动信号)。视频样本请参见ttfamily项目页面(https://omnihuman-lab.github.io)。
论文及项目相关链接
PDF https://omnihuman-lab.github.io/
Summary
该文章介绍了基于Diffusion Transformer的OmniHuman框架,它通过混合运动相关条件进行训练,实现了大规模数据驱动的运动生成,最终实现了高度逼真的人类视频生成。OmniHuman支持多种肖像内容、说话和唱歌、人机交互和具有挑战性的身体姿势,并具有不同的图像风格支持多种输入模态(音频驱动、视频驱动和组合驱动信号)。
Key Takeaways
- OmniHuman是一个基于Diffusion Transformer的框架,用于实现大规模数据驱动的运动生成。
- 它通过混合运动相关条件进行训练,以实现高度逼真的人类视频生成。
- OmniHuman支持多种肖像内容,包括面部特写、肖像、半身和全身。
- 该框架支持说话和唱歌,以及人机交互和具有挑战性的身体姿势。
- OmniHuman具有不同的图像风格支持能力。
- 与现有的端到端音频驱动方法相比,OmniHuman不仅产生更逼真的视频,而且输入更灵活。
点此查看论文截图
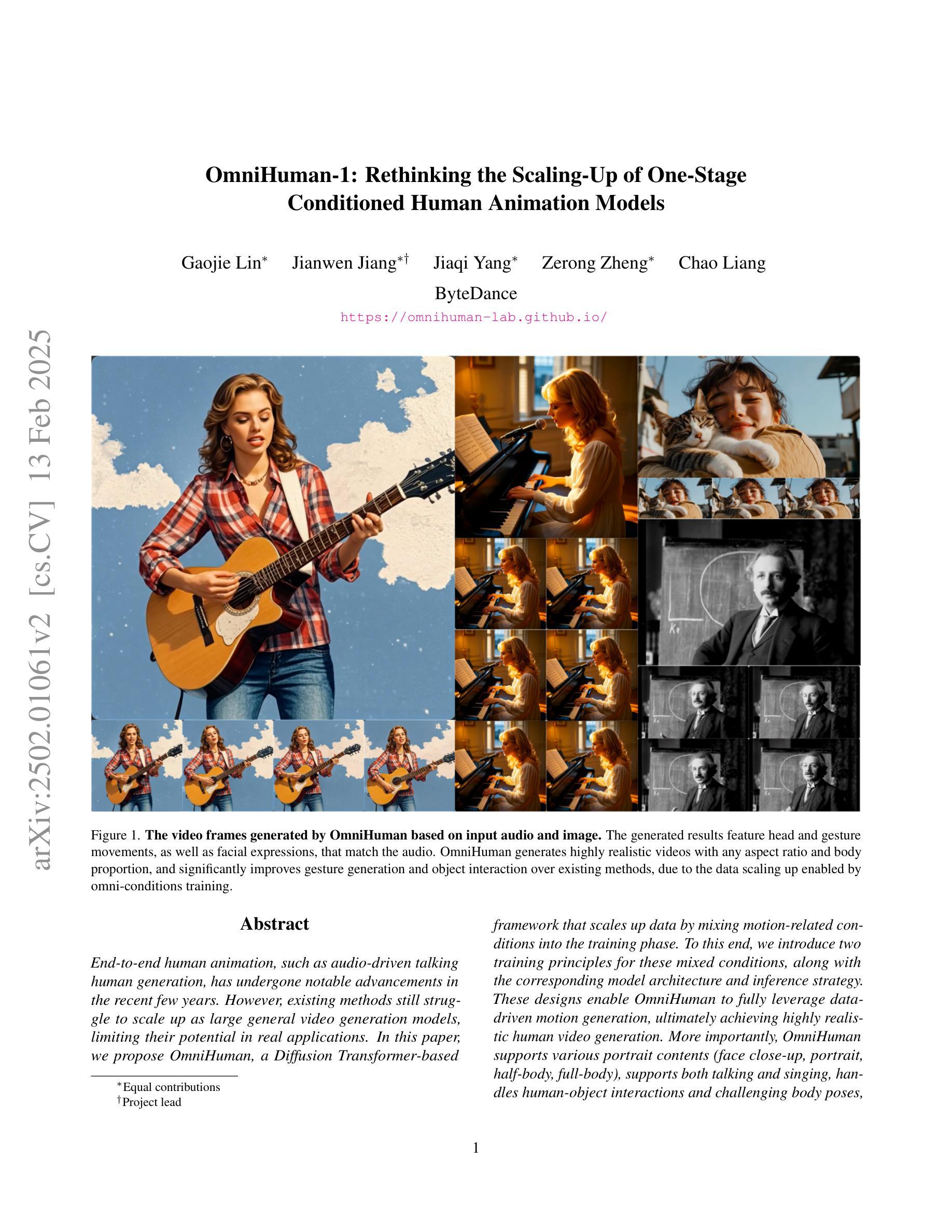
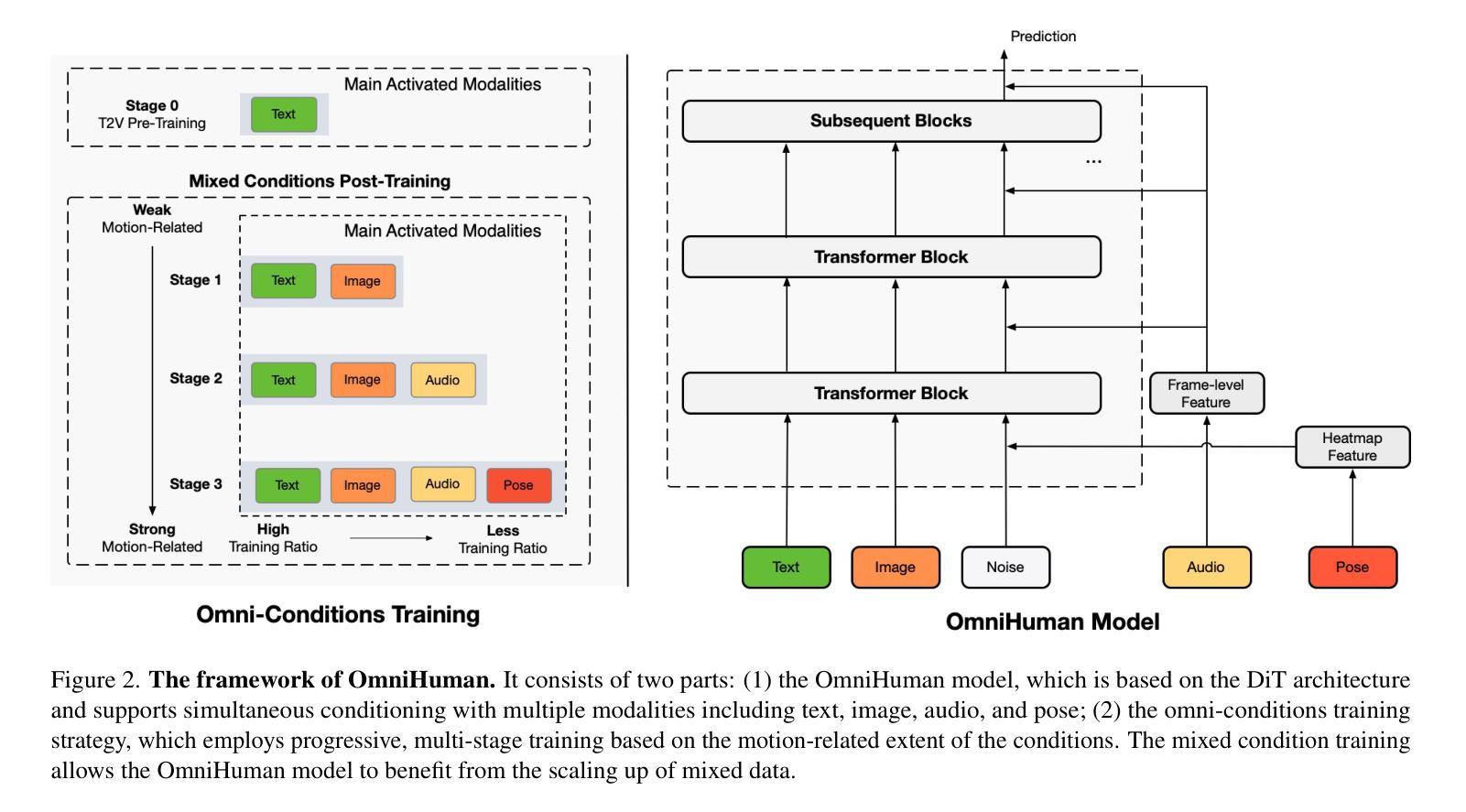
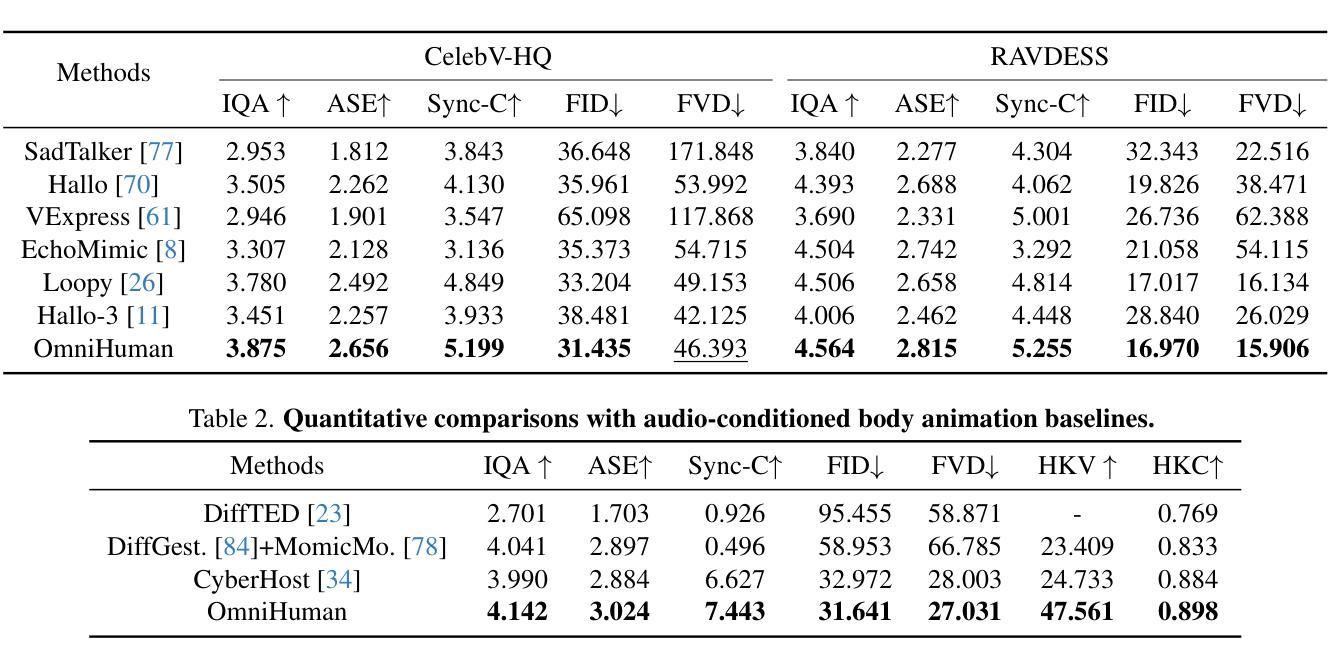
