⚠️ 以下所有内容总结都来自于 大语言模型的能力,如有错误,仅供参考,谨慎使用
🔴 请注意:千万不要用于严肃的学术场景,只能用于论文阅读前的初筛!
💗 如果您觉得我们的项目对您有帮助 ChatPaperFree ,还请您给我们一些鼓励!⭐️ HuggingFace免费体验
2025-02-19 更新
FrGNet: A fourier-guided weakly-supervised framework for nuclear instance segmentation
Authors:Peng Ling
Nuclear instance segmentation has played a critical role in pathology image analysis. The main challenges arise from the difficulty in accurately segmenting instances and the high cost of precise mask-level annotations for fully-supervised training.In this work, we propose a fourier guidance framework for solving the weakly-supervised nuclear instance segmentation problem. In this framework, we construct a fourier guidance module to fuse the priori information into the training process of the model, which facilitates the model to capture the relevant features of the nuclear.Meanwhile, in order to further improve the model’s ability to represent the features of nuclear, we propose the guide-based instance level contrastive module. This module makes full use of the framework’s own properties and guide information to effectively enhance the representation features of nuclear. We show on two public datasets that our model can outperform current SOTA methods under fully-supervised design, and in weakly-supervised experiments, with only a small amount of labeling our model still maintains close to the performance under full supervision.In addition, we also perform generalization experiments on a private dataset, and without any labeling, our model is able to segment nuclear images that have not been seen during training quite effectively. As open science, all codes and pre-trained models are available at https://github.com/LQY404/FrGNet.
核实例分割在病理学图像分析中发挥了关键作用。主要挑战来自于准确分割实例的困难和全监督训练对精确掩膜级别标注的高成本。在这项工作中,我们提出了一个傅里叶引导框架来解决弱监督核实例分割问题。在这个框架中,我们构建了一个傅里叶引导模块,将先验信息融合到模型的训练过程中,这有助于模型捕捉核的相关特征。同时,为了进一步提高模型对核特征的表达能能力,我们提出了基于引导的实例级对比模块。该模块充分利用了框架自身的属性和引导信息,有效地增强了核的特征表达。我们在两个公开数据集上展示了我们的模型在全监督设计下的性能超越了当前的最佳方法,而在弱监督实验中,只有少量的标注,我们的模型仍然能保持接近全监督下的性能。此外,我们还对一个私有数据集进行了泛化实验,在无标注的情况下,我们的模型能够有效地对训练期间未见过的核图像进行分割。本着开放科学的精神,所有代码和预训练模型都可以在https://github.com/LQY404/FrGNet找到。
论文及项目相关链接
Summary:
本文提出了一种基于傅里叶引导框架的弱监督核实例分割方法。通过构建傅里叶引导模块,将先验信息融合到模型训练过程中,并引入基于引导的实例级对比模块,提高模型对细胞核特征的表示能力。在公开数据集上的实验表明,该方法在全监督设计和弱监督实验中的性能均优于当前最先进的方法。此外,该模型在未标注的私有数据集上也能进行有效的核图像分割。所有代码和预训练模型均可在公开GitHub仓库中找到。
Key Takeaways:
- 核实例分割在病理学图像分析中扮演关键角色,主要挑战在于准确分割实例以及精确掩膜级标注的高成本。
- 提出了基于傅里叶引导框架的弱监督核实例分割方法,通过构建傅里叶引导模块融合先验信息。
- 引入基于引导的实例级对比模块,提高模型对细胞核特征的表示能力。
- 在公开数据集上的实验表明,该方法在全监督和弱监督实验中的性能均优于当前最先进的方法。
- 模型在私有数据集上进行了泛化实验,未标注的图像也能进行有效分割。
- 所有代码和预训练模型均公开可用。
点此查看论文截图
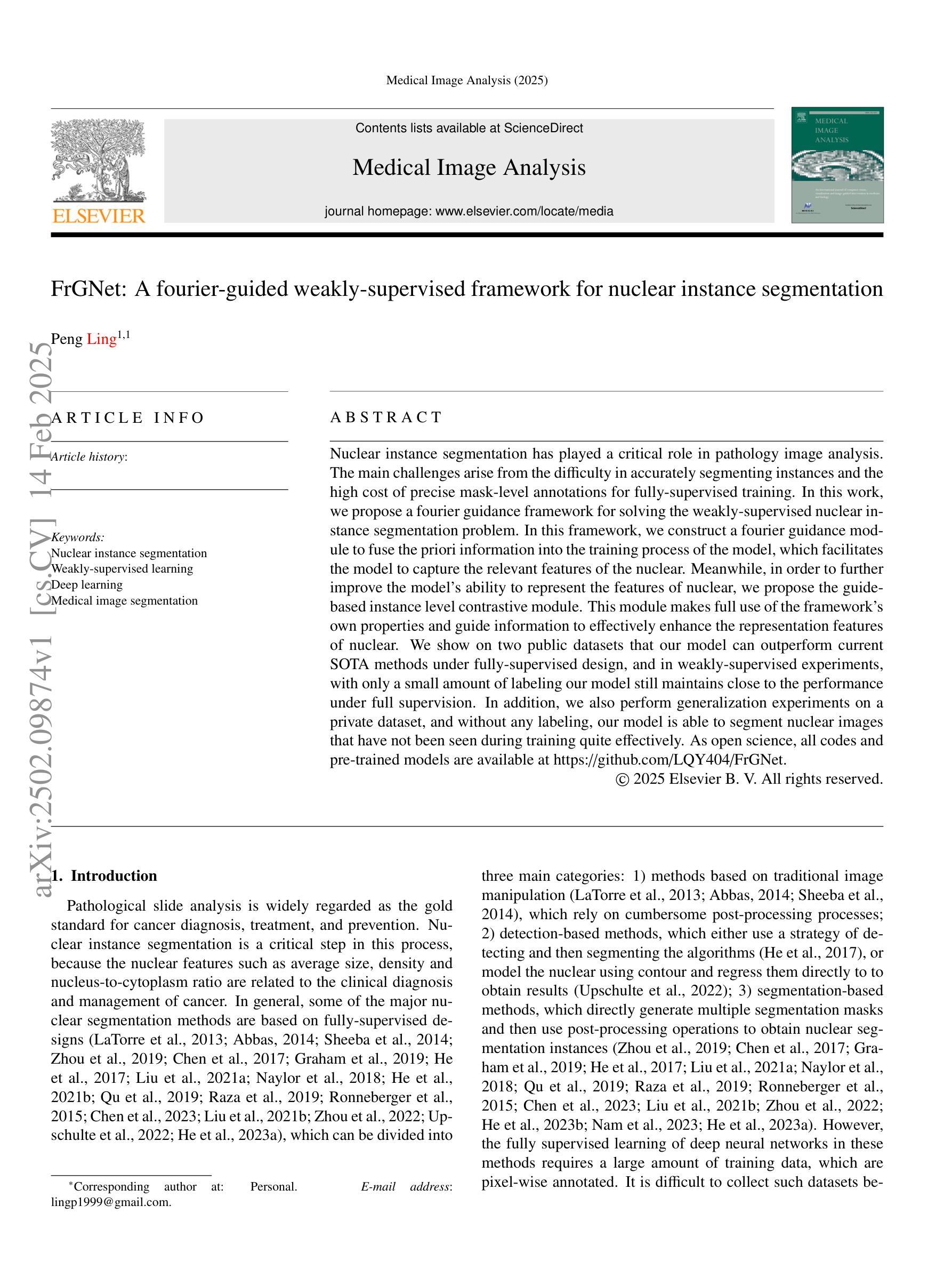
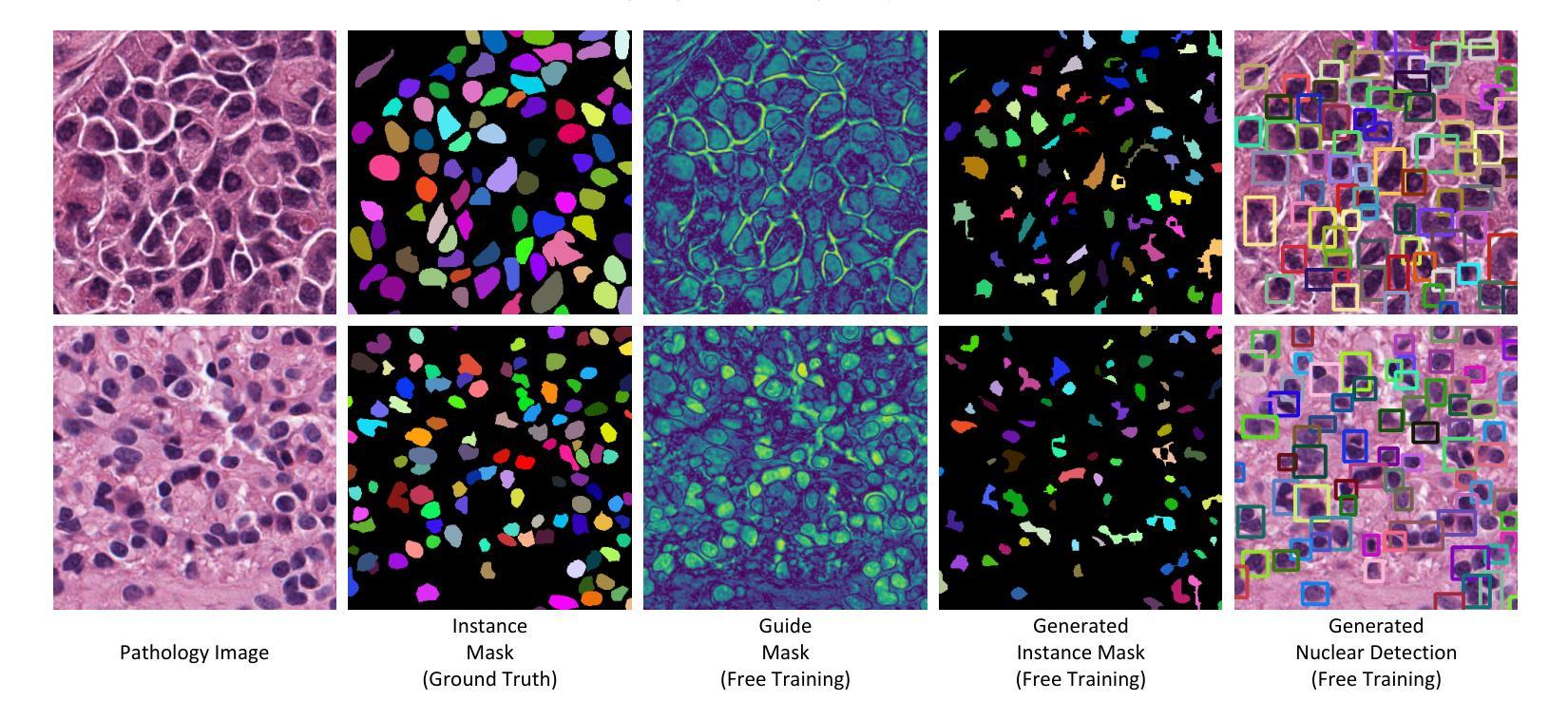
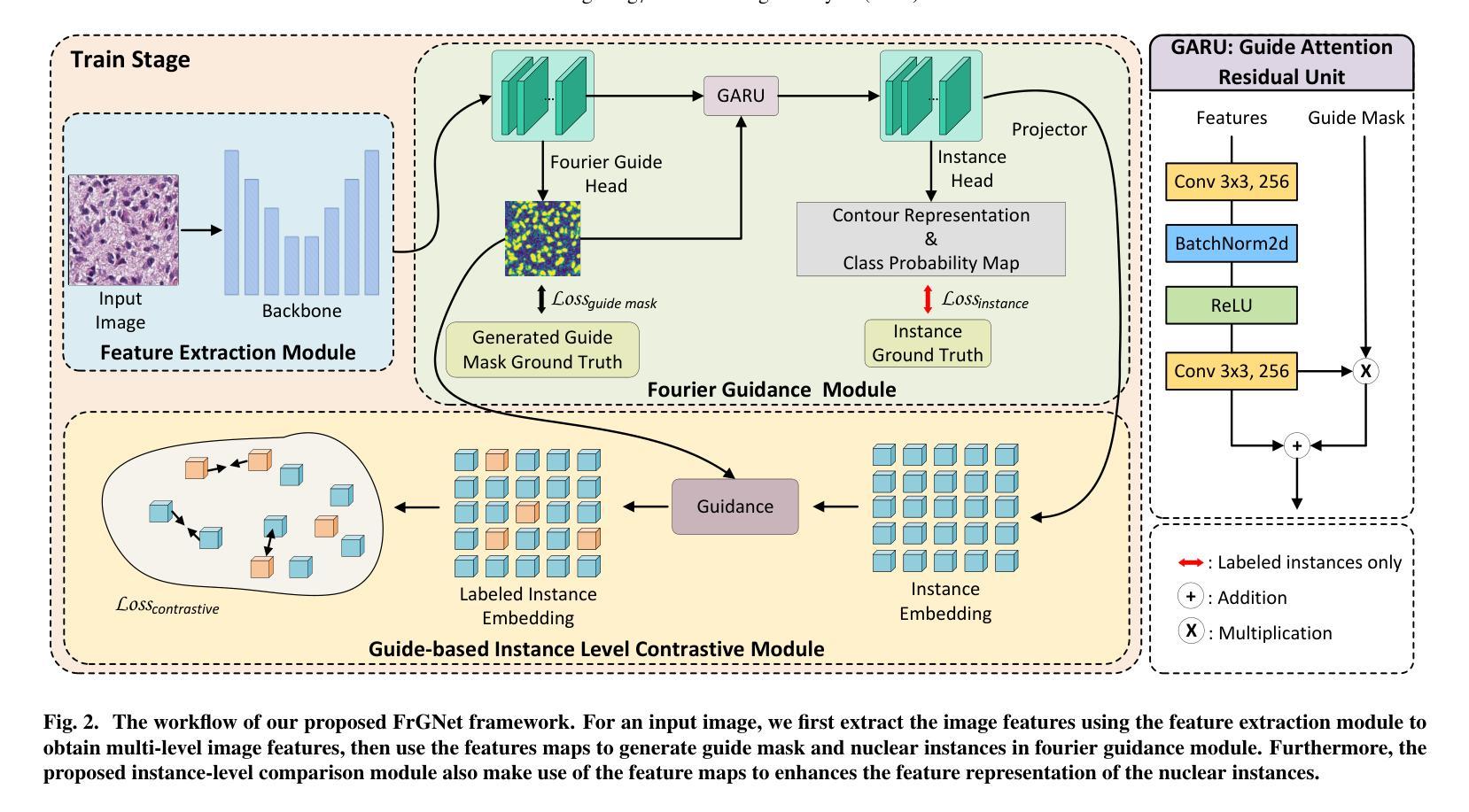
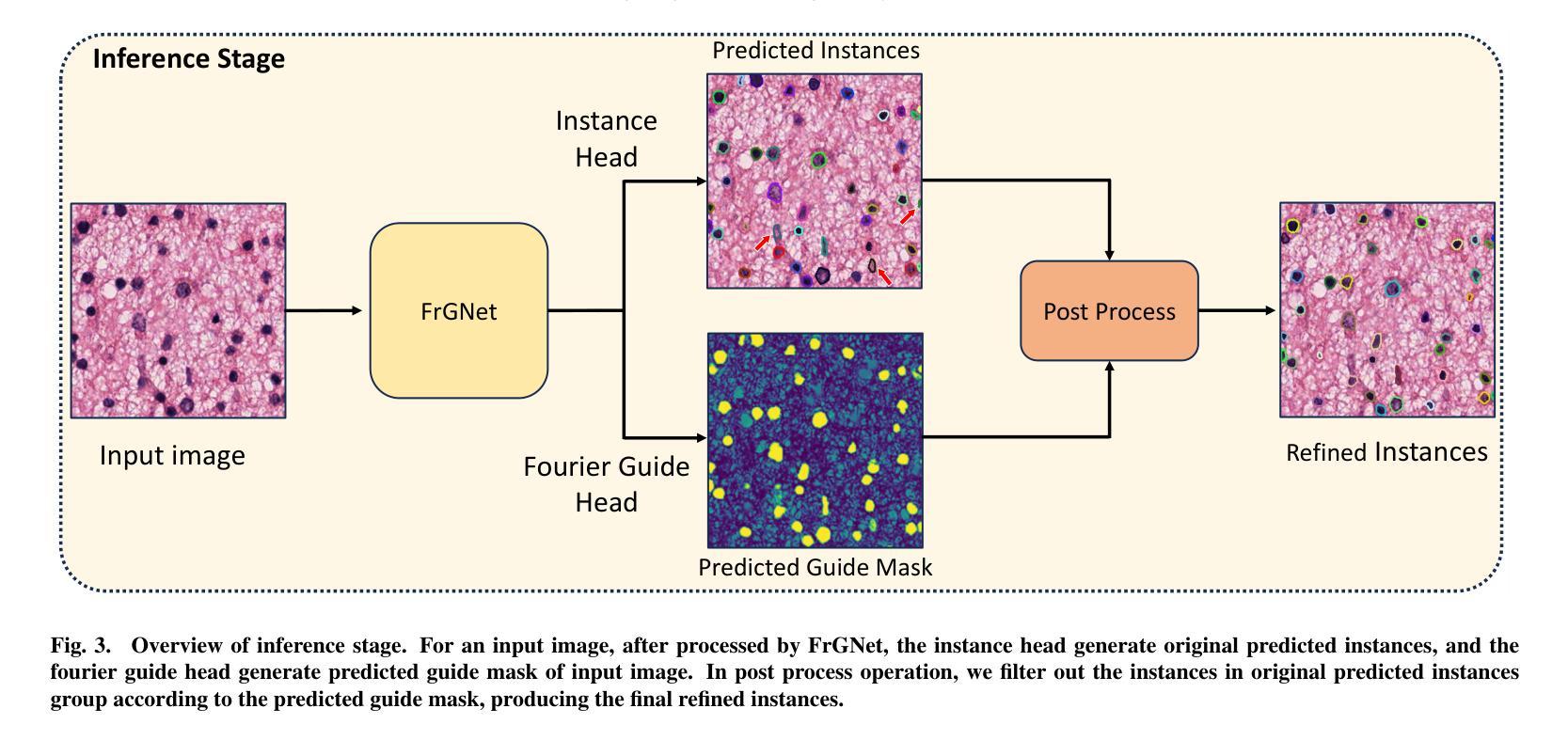