⚠️ 以下所有内容总结都来自于 大语言模型的能力,如有错误,仅供参考,谨慎使用
🔴 请注意:千万不要用于严肃的学术场景,只能用于论文阅读前的初筛!
💗 如果您觉得我们的项目对您有帮助 ChatPaperFree ,还请您给我们一些鼓励!⭐️ HuggingFace免费体验
2025-02-20 更新
Detection and Geographic Localization of Natural Objects in the Wild: A Case Study on Palms
Authors:Kangning Cui, Rongkun Zhu, Manqi Wang, Wei Tang, Gregory D. Larsen, Victor P. Pauca, Sarra Alqahtani, Fan Yang, David Segurado, David Lutz, Jean-Michel Morel, Miles R. Silman
Palms are ecologically and economically indicators of tropical forest health, biodiversity, and human impact that support local economies and global forest product supply chains. While palm detection in plantations is well-studied, efforts to map naturally occurring palms in dense forests remain limited by overlapping crowns, uneven shading, and heterogeneous landscapes. We develop PRISM (Processing, Inference, Segmentation, and Mapping), a flexible pipeline for detecting and localizing palms in dense tropical forests using large orthomosaic images. Orthomosaics are created from thousands of aerial images and spanning several to hundreds of gigabytes. Our contributions are threefold. First, we construct a large UAV-derived orthomosaic dataset collected across 21 ecologically diverse sites in western Ecuador, annotated with 8,830 bounding boxes and 5,026 palm center points. Second, we evaluate multiple state-of-the-art object detectors based on efficiency and performance, integrating zero-shot SAM 2 as the segmentation backbone, and refining the results for precise geographic mapping. Third, we apply calibration methods to align confidence scores with IoU and explore saliency maps for feature explainability. Though optimized for palms, PRISM is adaptable for identifying other natural objects, such as eastern white pines. Future work will explore transfer learning for lower-resolution datasets (0.5 to 1m).
棕榈树在生态和经济上是热带森林健康、生物多样性和人类影响的指标,支持当地经济和全球森林产品供应链。虽然棕榈树在种植园的检测已经得到了很好的研究,但在密集森林中自然生长的棕榈树的地图绘制工作仍受到树冠重叠、阴影不均和地形复杂等因素的限制。我们开发了PRISM(处理、推理、分割和映射),这是一个灵活的管道,用于使用大型正射镶嵌图像检测和定位密集热带森林中的棕榈树。正射镶嵌图像由数千张航空照片制成,占据几到数百吉字节。我们的贡献有三点。首先,我们构建了一个大型无人机衍生的正射镶嵌数据集,该数据集收集了厄瓜多尔西部21个生态不同站点的数据,用8830个边界框和5026个棕榈树中心点进行标注。其次,我们评估了多个最新物体检测器的效率和性能,以零样本SAM 2作为分割主干进行整合,并优化结果进行精确地理映射。第三,我们应用校准方法将置信度得分与IoU对齐,并探索显著性图进行特征解释。虽然PRISM是针对棕榈树优化的,但它也可以用于识别其他自然物体,如东部白皮松。未来的工作将探索在较低分辨率数据集(0.5至1米)上的迁移学习。
论文及项目相关链接
PDF 15 pages, 8 figures, 4 tables
Summary
该文主要研究了利用PRISM管道技术检测热带森林中的棕榈树。通过利用无人机收集的大量生态多样区域的正射影像数据,构建了一个大型数据集,并评估了多种先进的物体检测器,实现了对棕榈树的精准定位与识别。该技术可以灵活应用于其他自然物体的识别,并具有在较低分辨率数据集上进行迁移学习的潜力。
Key Takeaways
- 棕榈树作为热带森林的生态和经济指标,对当地经济和全球森林产品供应链有重要作用。
- 目前对棕榈树的检测主要集中在种植园,而自然森林中的棕榈树检测仍面临树冠重叠、阴影不均和景观多样性的问题。
- PRISM是一种灵活的管道技术,可用于检测热带森林中的棕榈树,该技术基于大型正射影像图像。
- PRISM的贡献包括构建大型无人机正射影像数据集、评估多种先进的物体检测器并优化结果以实现精准地理定位。
- PRISM技术不仅可以用于棕榈树的识别,还可以灵活应用于其他自然物体的识别。
- PRISM技术具有在较低分辨率数据集上进行迁移学习的潜力。
点此查看论文截图
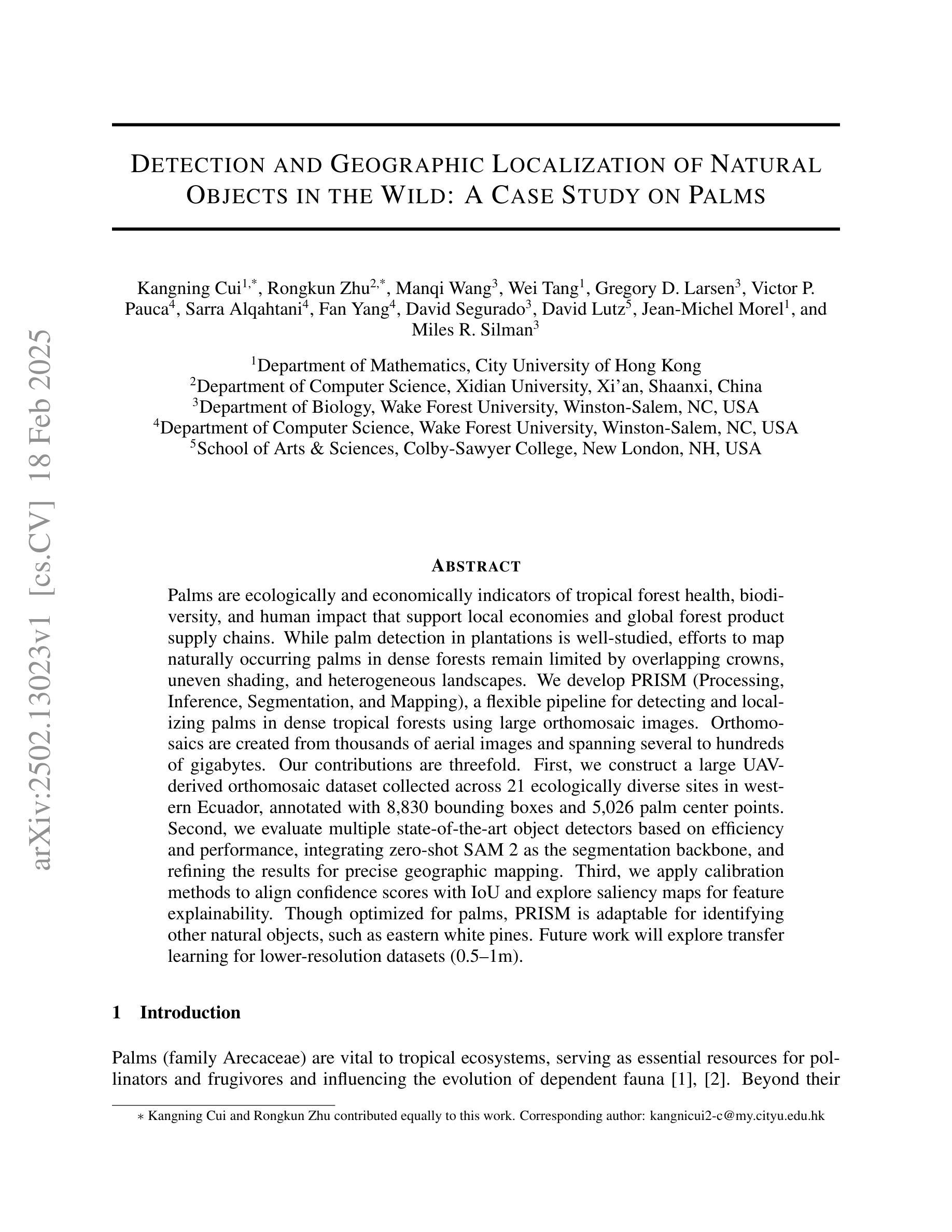
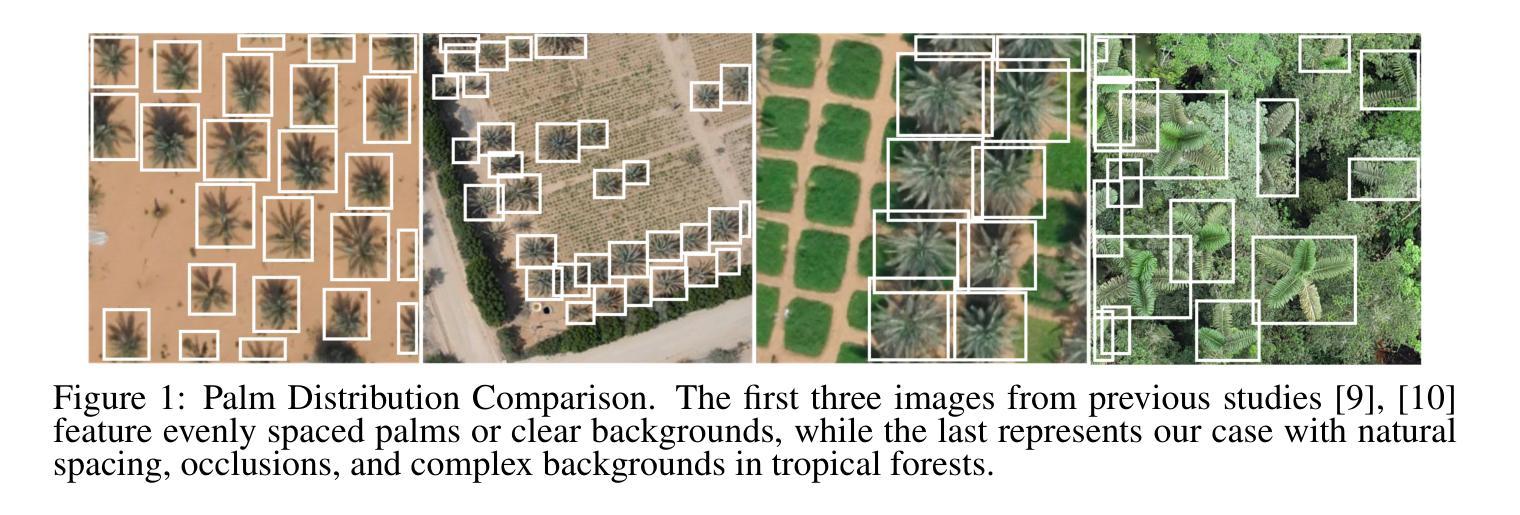
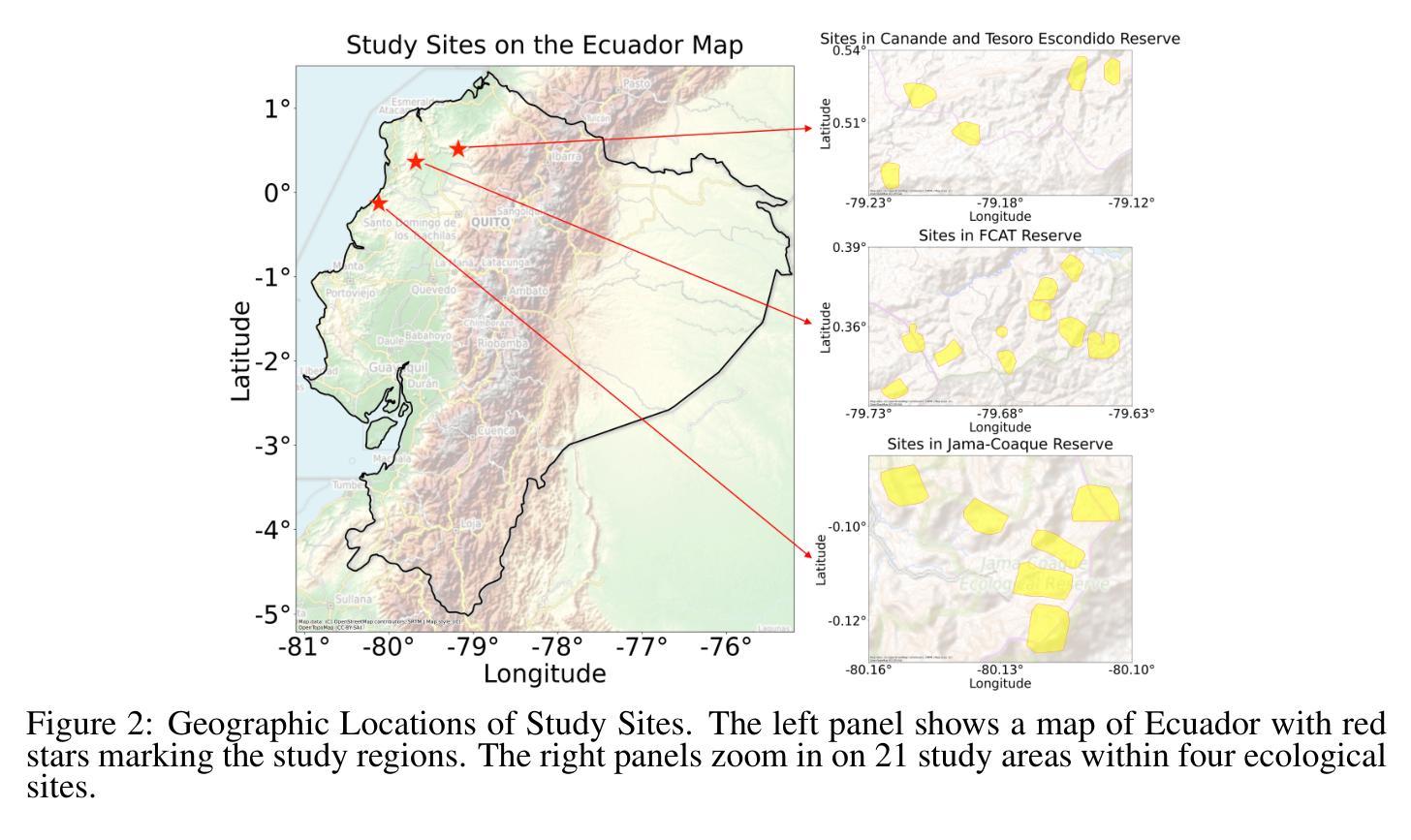
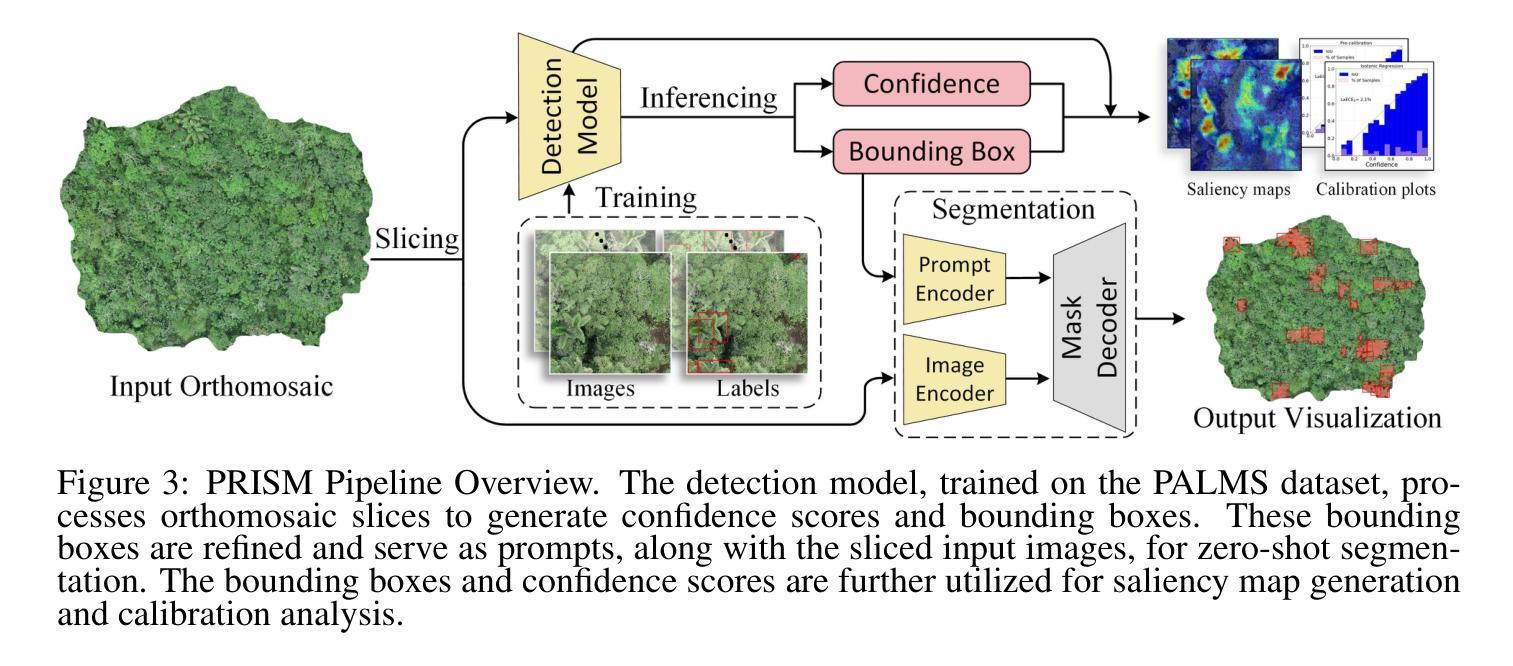
Instance-Level Moving Object Segmentation from a Single Image with Events
Authors:Zhexiong Wan, Bin Fan, Le Hui, Yuchao Dai, Gim Hee Lee
Moving object segmentation plays a crucial role in understanding dynamic scenes involving multiple moving objects, while the difficulties lie in taking into account both spatial texture structures and temporal motion cues. Existing methods based on video frames encounter difficulties in distinguishing whether pixel displacements of an object are caused by camera motion or object motion due to the complexities of accurate image-based motion modeling. Recent advances exploit the motion sensitivity of novel event cameras to counter conventional images’ inadequate motion modeling capabilities, but instead lead to challenges in segmenting pixel-level object masks due to the lack of dense texture structures in events. To address these two limitations imposed by unimodal settings, we propose the first instance-level moving object segmentation framework that integrates complementary texture and motion cues. Our model incorporates implicit cross-modal masked attention augmentation, explicit contrastive feature learning, and flow-guided motion enhancement to exploit dense texture information from a single image and rich motion information from events, respectively. By leveraging the augmented texture and motion features, we separate mask segmentation from motion classification to handle varying numbers of independently moving objects. Through extensive evaluations on multiple datasets, as well as ablation experiments with different input settings and real-time efficiency analysis of the proposed framework, we believe that our first attempt to incorporate image and event data for practical deployment can provide new insights for future work in event-based motion related works. The source code with model training and pre-trained weights is released at https://npucvr.github.io/EvInsMOS
移动对象分割在理解涉及多个移动对象的动态场景时起着至关重要的作用,其难点在于需要考虑空间纹理结构和时间运动线索。基于视频帧的现有方法在区分对象的像素位移是由相机运动还是对象运动引起时遇到困难,这是由于基于图像的准确运动建模的复杂性。最近的进展利用新型事件相机的运动敏感性来弥补传统图像运动建模能力的不足,然而,由于缺乏事件中的密集纹理结构,导致在像素级别对象掩膜分割方面存在挑战。为了解决这两种由单模态设置引起的局限性,我们提出了第一个实例级移动对象分割框架,该框架融合了互补的纹理和运动线索。我们的模型结合了隐式跨模态掩模注意力增强、显式对比特征学习和流引导运动增强,分别利用来自单张图像的密集纹理信息和来自事件丰富的运动信息。通过利用增强的纹理和运动特征,我们将掩膜分割与运动分类分开,以处理数量不等的独立运动对象。通过多个数据集的综合评估,以及与不同输入设置的消融实验和所提出框架的实时效率分析,我们相信我们首次将图像和事件数据用于实际部署,可以为未来基于事件的运动相关工作提供新的见解。模型的源代码及训练好的权重已在https://npucvr.github.io/EvInsMOS发布。
论文及项目相关链接
PDF accepted by IJCV
Summary
移动物体分割在理解涉及多个移动物体的动态场景中起到关键作用,面临既要考虑空间纹理结构又要考虑时间运动线索的挑战。现有基于视频帧的方法在区分物体像素位移是由相机运动还是物体运动引起时遇到困难。最近的研究利用新型事件相机对运动的敏感性来克服传统图像运动建模能力不足的缺点,但缺乏密集纹理结构的事件导致像素级物体分割面临挑战。为解决这两种限制,我们提出了首个实例级移动物体分割框架,整合纹理和运动线索。我们的模型采用隐式跨模态掩膜注意力增强、显式对比特征学习和流引导运动增强技术,分别利用单图像的密集纹理信息和事件丰富的运动信息。通过增强的纹理和运动特征,我们将掩膜分割与运动分类分离,以处理数量不等的独立移动物体。
Key Takeaways
- 移动物体分割在理解动态场景时至关重要,需考虑空间纹理结构和时间运动线索。
- 现有方法难以区分像素位移的来源,难以准确进行运动建模。
- 事件相机对运动的敏感性有助于克服传统图像的不足,但事件缺乏密集纹理结构导致像素级物体分割挑战。
- 提出首个实例级移动物体分割框架,整合纹理和运动线索。
- 采用隐式跨模态掩膜注意力增强、显式对比特征学习和流引导运动增强技术。
- 通过增强的纹理和运动特征分离掩膜分割与运动分类,处理不同数量的独立移动物体。
点此查看论文截图
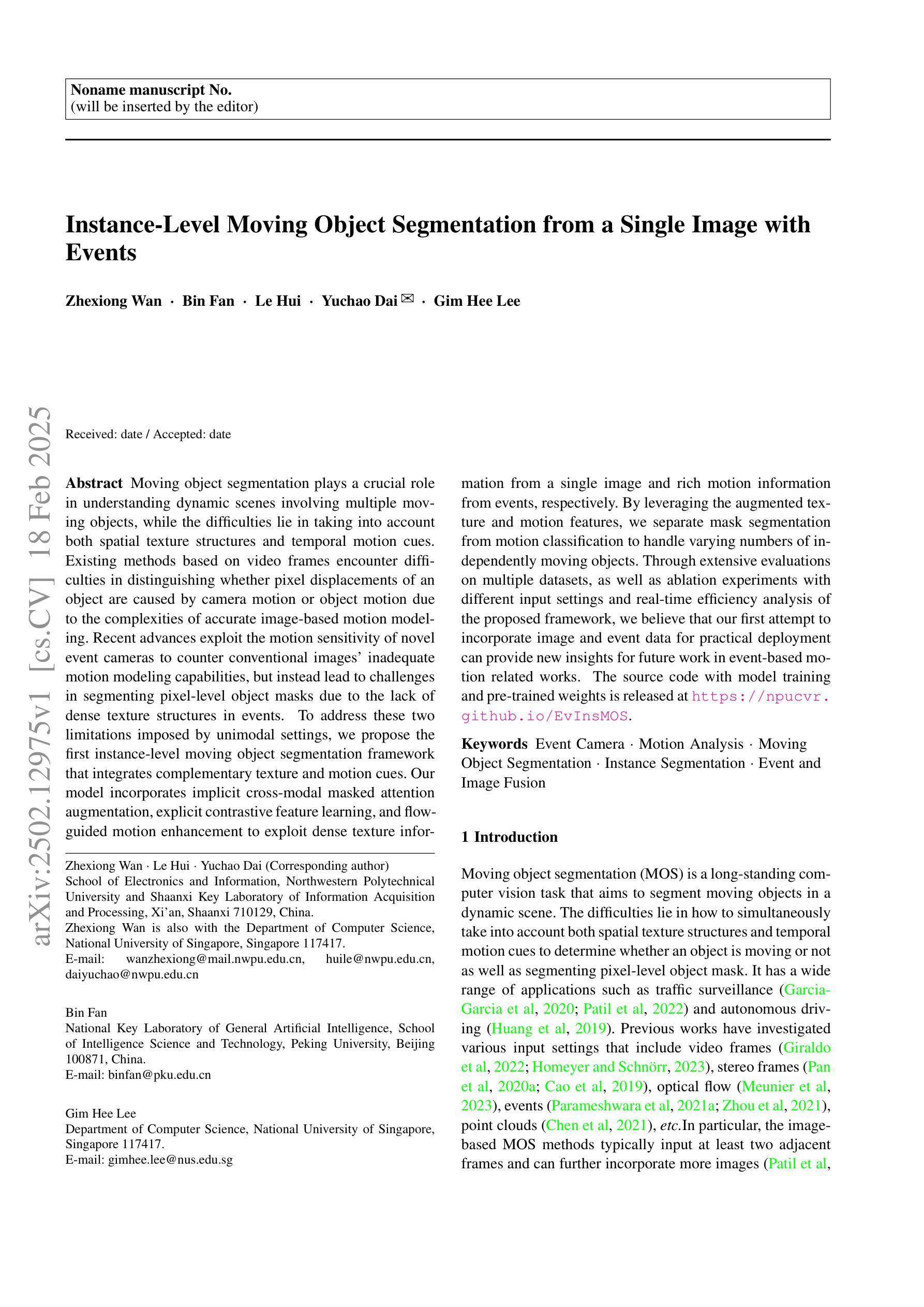
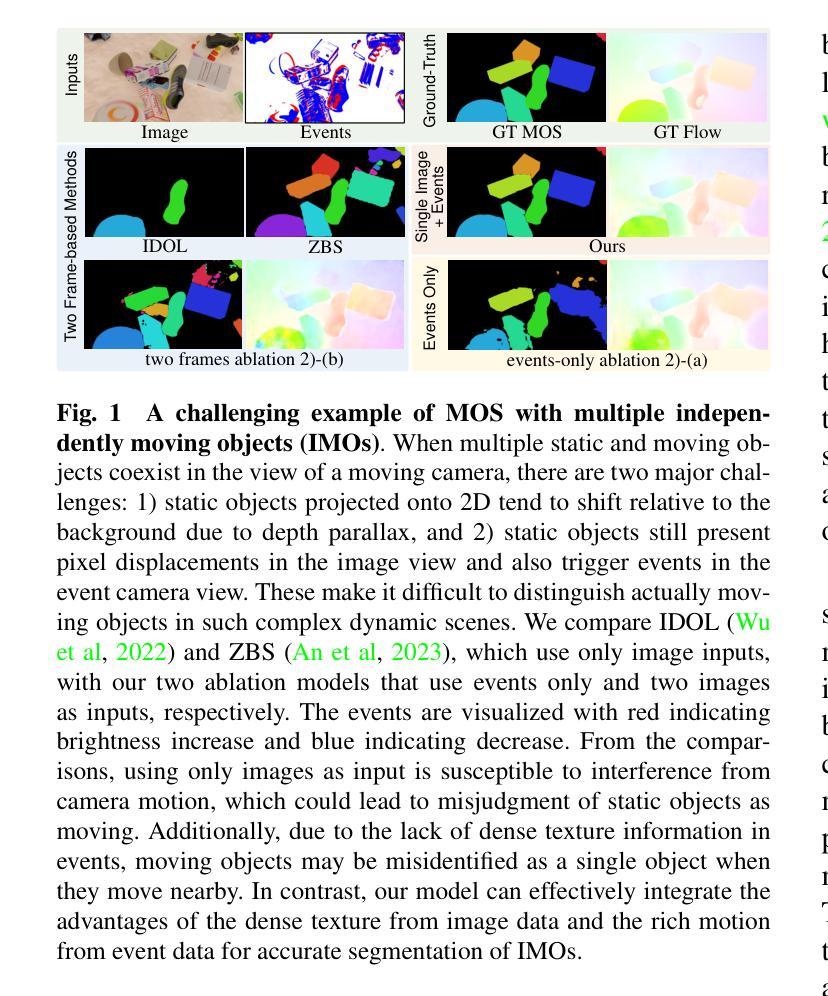
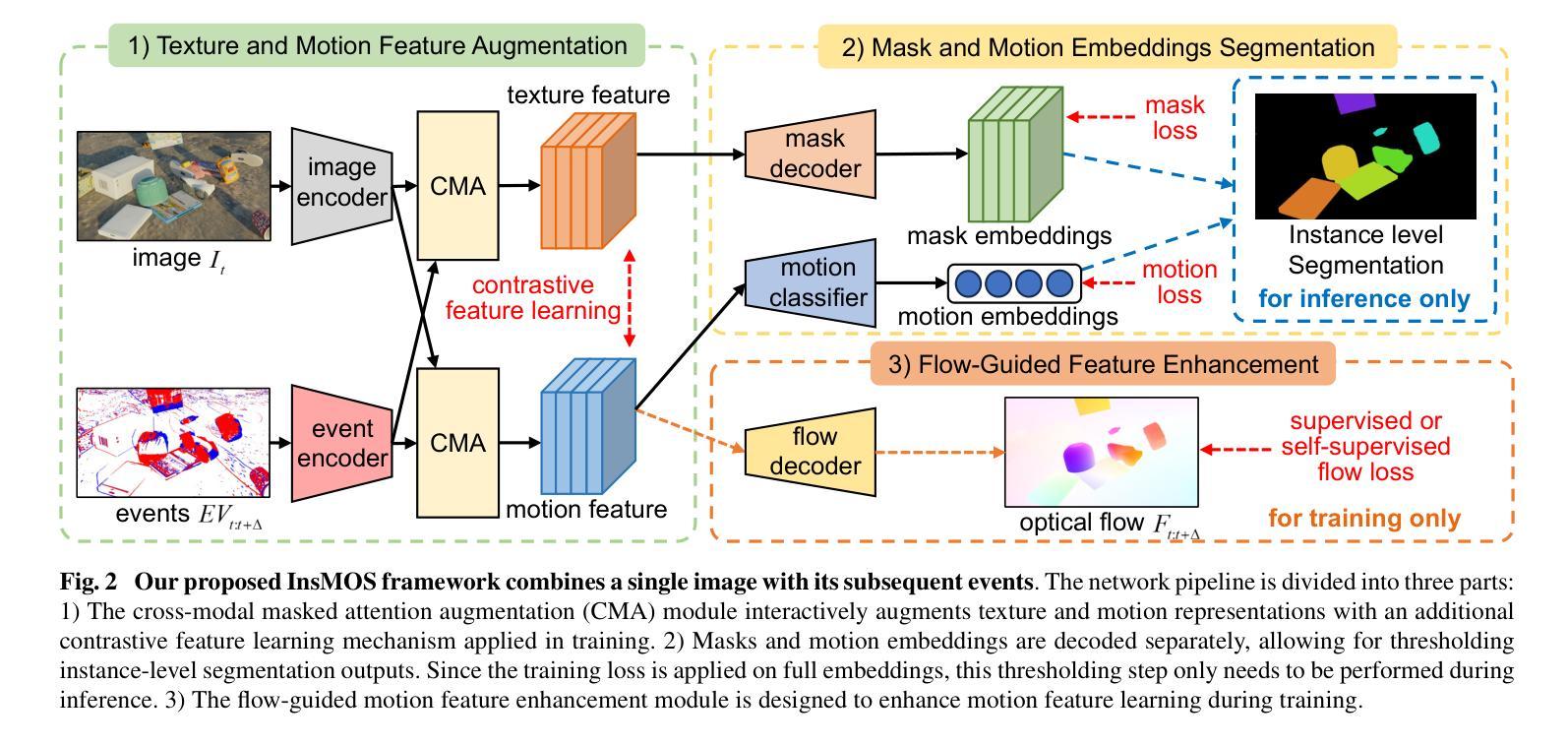
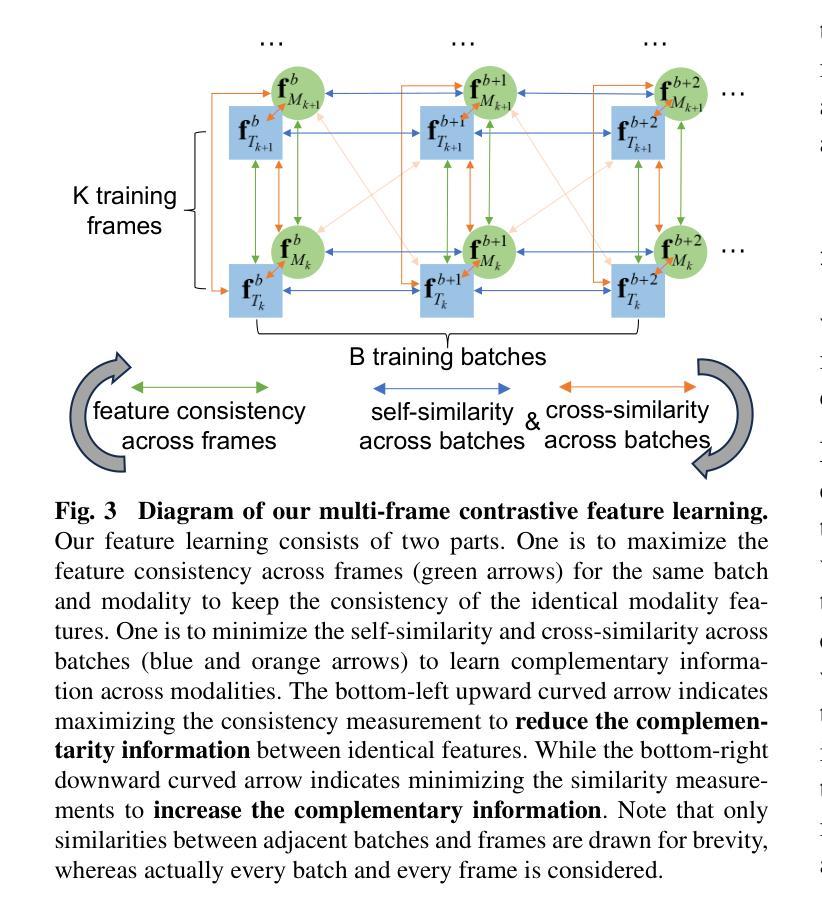
Task-Oriented Semantic Communication for Stereo-Vision 3D Object Detection
Authors:Zijian Cao, Hua Zhang, Le Liang, Haotian Wang, Shi Jin, Geoffrey Ye Li
With the development of computer vision, 3D object detection has become increasingly important in many real-world applications. Limited by the computing power of sensor-side hardware, the detection task is sometimes deployed on remote computing devices or the cloud to execute complex algorithms, which brings massive data transmission overhead. In response, this paper proposes an optical flow-driven semantic communication framework for the stereo-vision 3D object detection task. The proposed framework fully exploits the dependence of stereo-vision 3D detection on semantic information in images and prioritizes the transmission of this semantic information to reduce total transmission data sizes while ensuring the detection accuracy. Specifically, we develop an optical flow-driven module to jointly extract and recover semantics from the left and right images to reduce the loss of the left-right photometric alignment semantic information and improve the accuracy of depth inference. Then, we design a 2D semantic extraction module to identify and extract semantic meaning around the objects to enhance the transmission of semantic information in the key areas. Finally, a fusion network is used to fuse the recovered semantics, and reconstruct the stereo-vision images for 3D detection. Simulation results show that the proposed method improves the detection accuracy by nearly 70% and outperforms the traditional method, especially for the low signal-to-noise ratio regime.
随着计算机视觉的发展,3D对象检测在许多实际应用程序中的重要性日益增加。由于传感器端硬件计算能力的限制,检测任务有时会部署在远程计算设备或云端以执行复杂的算法,这带来了大量的数据传输开销。针对这一问题,本文提出了一个用于立体视觉3D对象检测任务的光流驱动语义通信框架。该框架充分利用了立体视觉3D检测对图像语义信息的依赖,并优先传输这些语义信息,以确保检测精度的同时减少总传输数据的大小。具体来说,我们开发了一个光流驱动模块,从左右图像中联合提取和恢复语义,以减少左右光度对齐语义信息的损失,提高深度推理的准确性。然后,我们设计了一个2D语义提取模块,用于识别和提取对象周围的语义含义,以增强关键区域语义信息的传输。最后,使用融合网络融合恢复的语义信息,并重建用于3D检测的立体视觉图像。仿真结果表明,该方法提高了近70%的检测精度,并且优于传统方法,特别是在低信噪比条件下。
论文及项目相关链接
Summary:
随着计算机视觉的发展,三维物体检测在现实世界应用中越来越重要。针对传感器端硬件计算能力的限制,本文提出一种光学流驱动语义通信框架,用于立体视觉三维物体检测任务。该框架充分利用立体视觉三维检测对图像语义信息的依赖,优先传输语义信息,确保检测精度的同时减少总数据传输数据量。通过光学流驱动模块联合提取和恢复左右图像的语义信息,减少左右光度对齐语义信息的损失并提高深度推理的准确性。仿真结果表明,该方法提高检测精度近70%,在低信噪比条件下表现尤其优异。
Key Takeaways:
- 光学流驱动语义通信框架用于立体视觉三维物体检测。
- 框架利用图像语义信息提高检测精度并减少数据传输。
- 光学流驱动模块联合提取和恢复左右图像的语义信息。
- 2D语义提取模块增强关键区域语义信息的传输。
- 融合网络用于融合恢复的语义信息并重建立体视觉图像。
- 仿真结果显示检测精度提高近70%。
点此查看论文截图
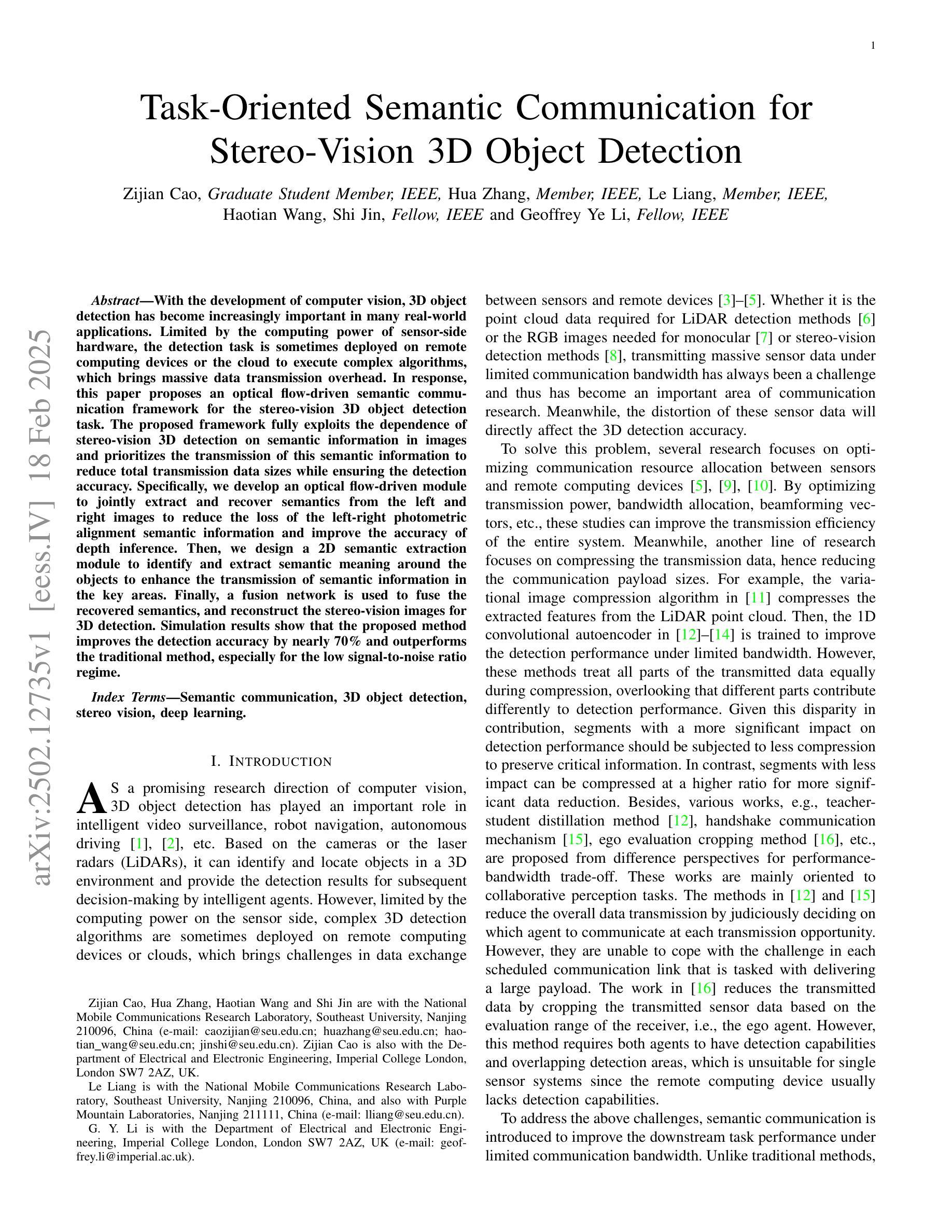
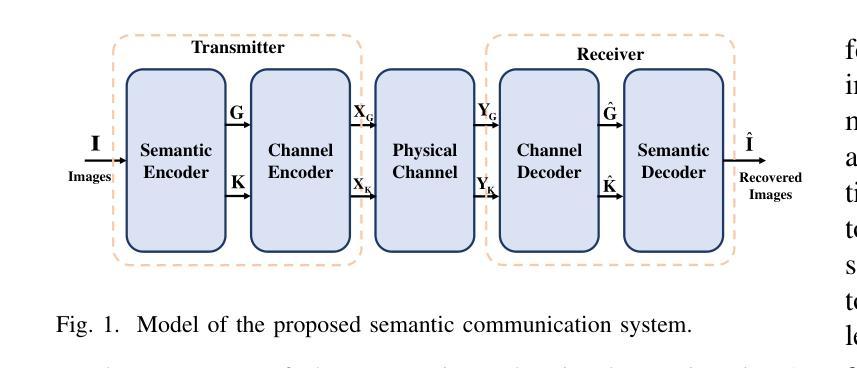
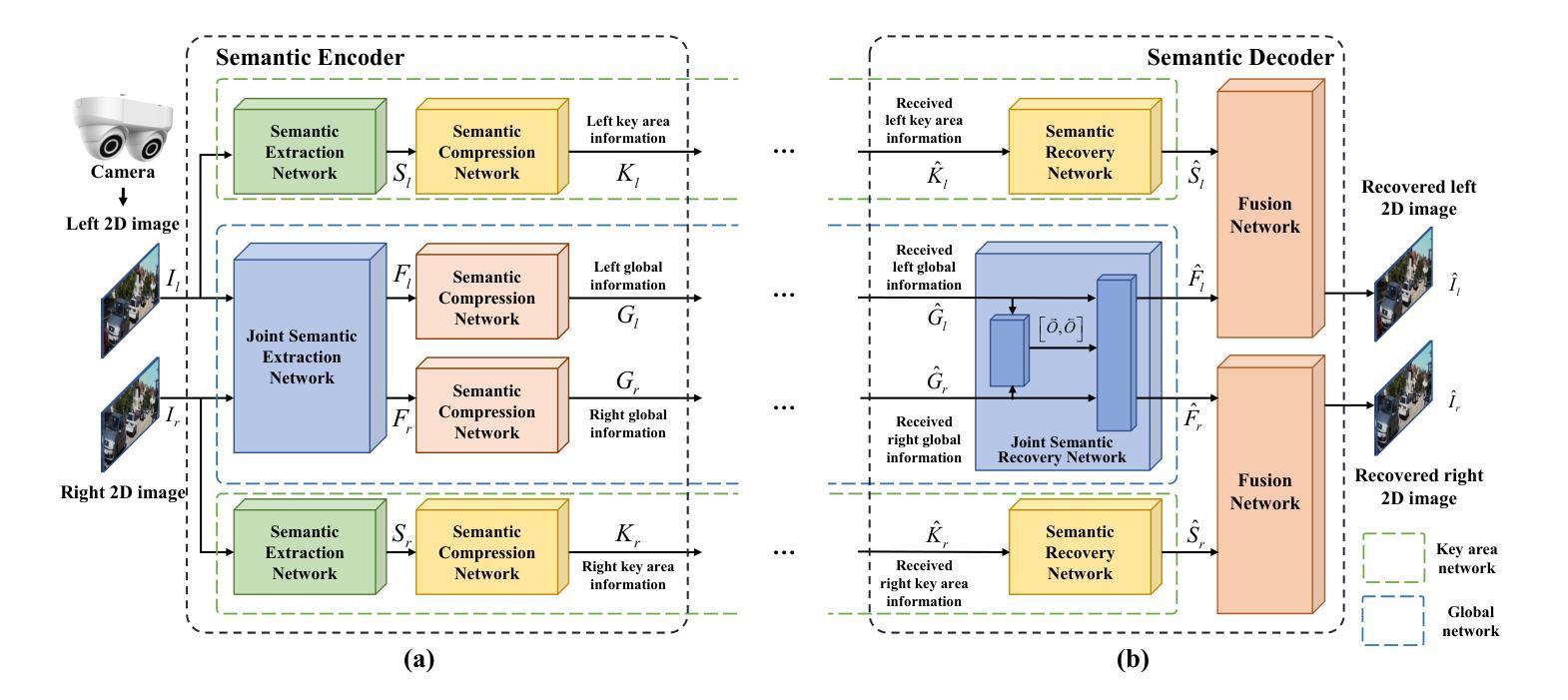
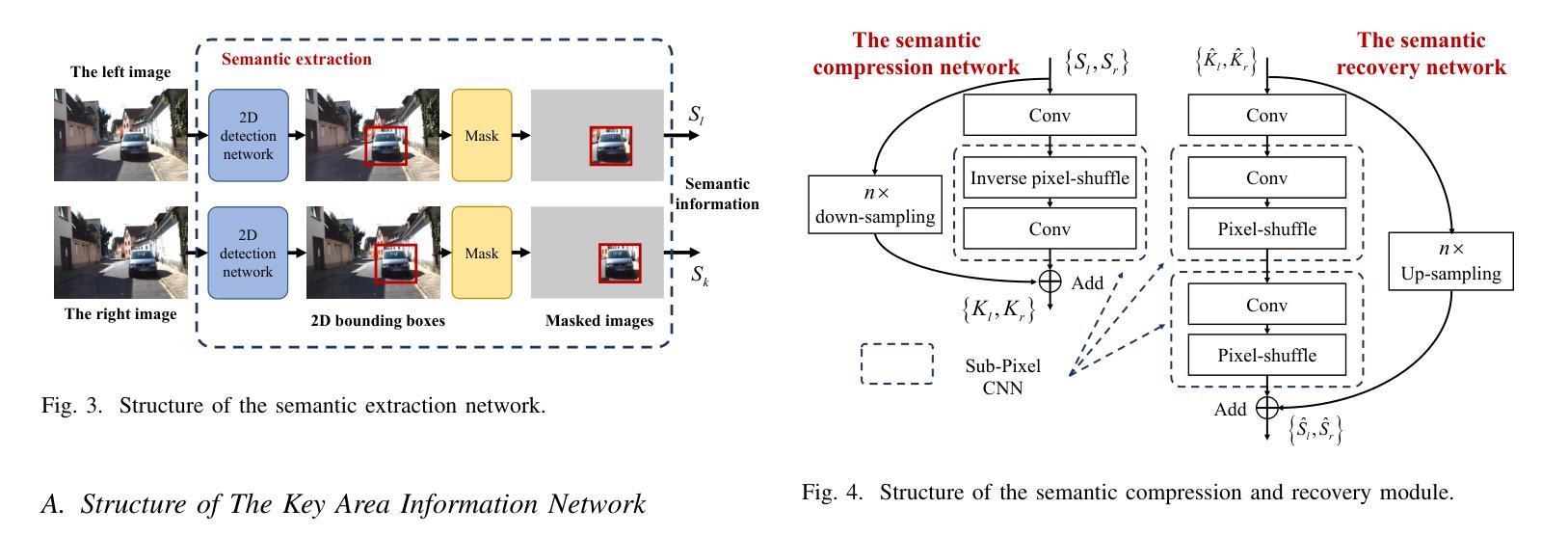
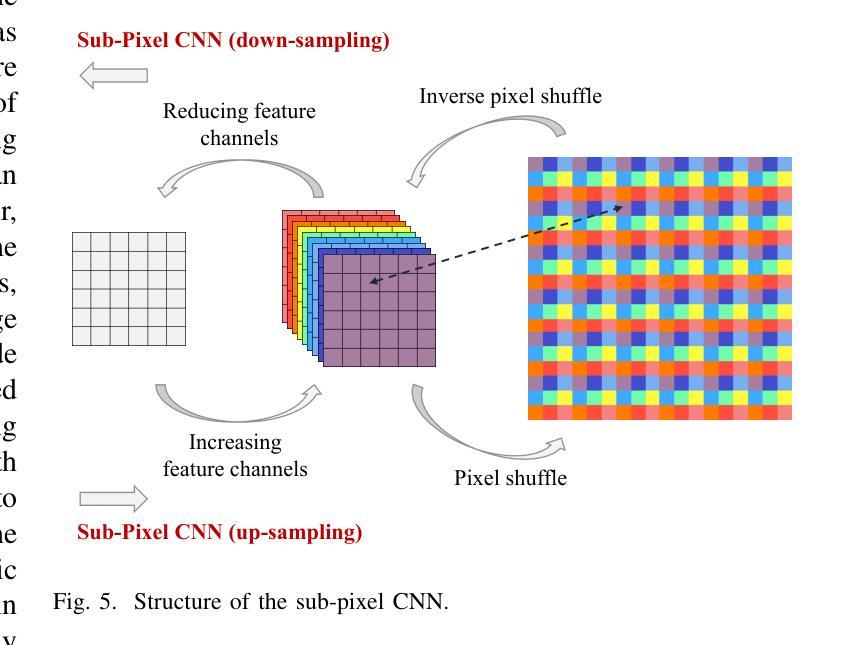
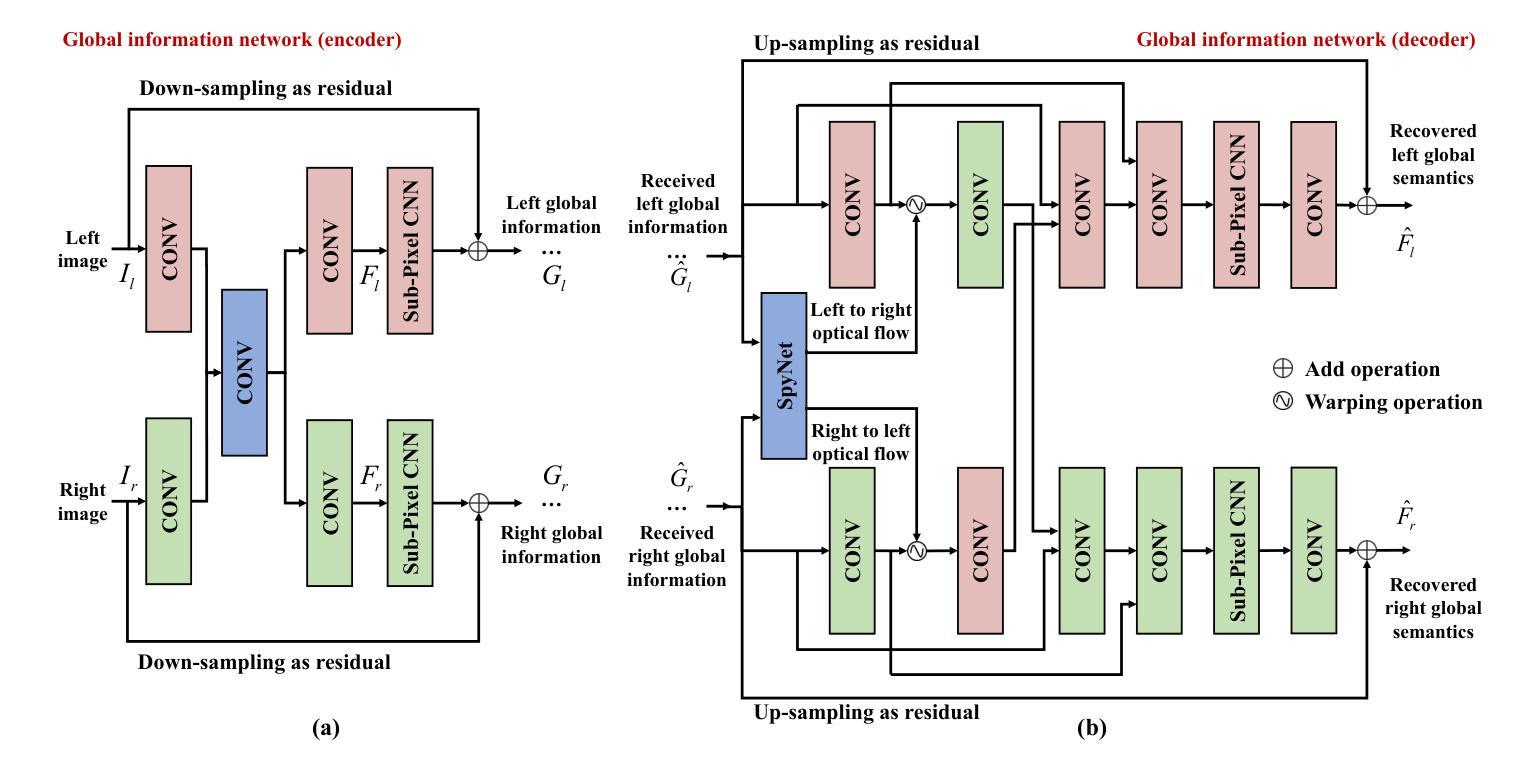
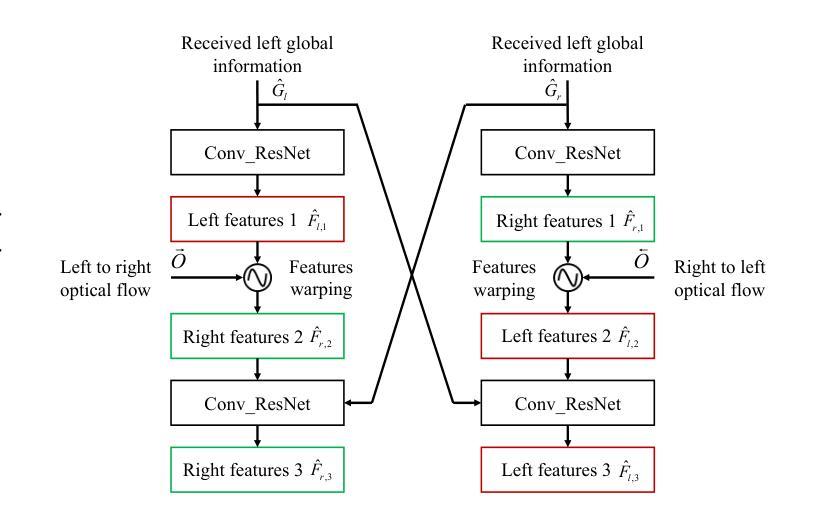
FrGNet: A fourier-guided weakly-supervised framework for nuclear instance segmentation
Authors:Peng Ling, Wenxiao Xiong
Nuclear instance segmentation has played a critical role in pathology image analysis. The main challenges arise from the difficulty in accurately segmenting instances and the high cost of precise mask-level annotations for fully-supervised training.In this work, we propose a fourier guidance framework for solving the weakly-supervised nuclear instance segmentation problem. In this framework, we construct a fourier guidance module to fuse the priori information into the training process of the model, which facilitates the model to capture the relevant features of the nuclear. Meanwhile, in order to further improve the model’s ability to represent the features of nuclear, we propose the guide-based instance level contrastive module. This module makes full use of the framework’s own properties and guide information to effectively enhance the representation features of nuclear. We show on two public datasets that our model can outperform current SOTA methods under fully-supervised design, and in weakly-supervised experiments, with only a small amount of labeling our model still maintains close to the performance under full supervision.In addition, we also perform generalization experiments on a private dataset, and without any labeling, our model is able to segment nuclear images that have not been seen during training quite effectively. As open science, all codes and pre-trained models are available at https://github.com/LQY404/FrGNet.
核实例分割在病理学图像分析中起到了至关重要的作用。主要挑战来自于准确分割实例的困难和为完全监督训练进行精确掩膜级别标注的高成本。在这项工作中,我们提出了一个傅里叶引导框架来解决弱监督核实例分割问题。在这个框架中,我们构建了一个傅里叶引导模块,将先验信息融合到模型的训练过程中,帮助模型捕捉核的相关特征。同时,为了进一步提高模型对核特征的表达能力,我们提出了基于引导的实例级对比模块。该模块充分利用了框架自身的属性和引导信息,有效地增强了核的表示特征。我们在两个公开数据集上展示,我们的模型在完全监督设计下能超越当前的最优方法,在弱监督实验中,只有少量的标注,我们的模型仍能保持接近完全监督下的性能。此外,我们还对一个私有数据集进行了泛化实验,无需任何标注,我们的模型就能够对训练期间未见过的核图像进行有效的分割。作为开放科学,所有代码和预训练模型都可在https://github.com/LQY404/FrGNet上找到。
论文及项目相关链接
Summary:
本文提出了一种基于傅里叶引导框架的弱监督核实例分割方法。通过构建傅里叶引导模块,将先验信息融入模型训练过程,并设计了基于指南的实例级对比模块,提高模型对核特征的表示能力。在公开数据集上的实验表明,该方法在全监督和弱监督设置下均优于当前最先进的方法,且在私有数据集上的无标签实验也能有效分割未见过的核图像。
Key Takeaways:
- 本文提出了基于傅里叶引导框架的弱监督核实例分割方法,解决了病理学图像分析中的核实例分割难题。
- 通过构建傅里叶引导模块,将先验信息融入模型训练,有助于模型捕捉核的相关特征。
- 设计了基于指南的实例级对比模块,提高模型对核特征的表示能力。
- 在公开数据集上的实验表明,该方法在全监督设置下性能优越。
- 在弱监督设置下,仅需要少量标注,模型仍能保持接近全监督的性能。
- 私有数据集上的无标签实验证明,模型能有效分割未见过的核图像。
点此查看论文截图
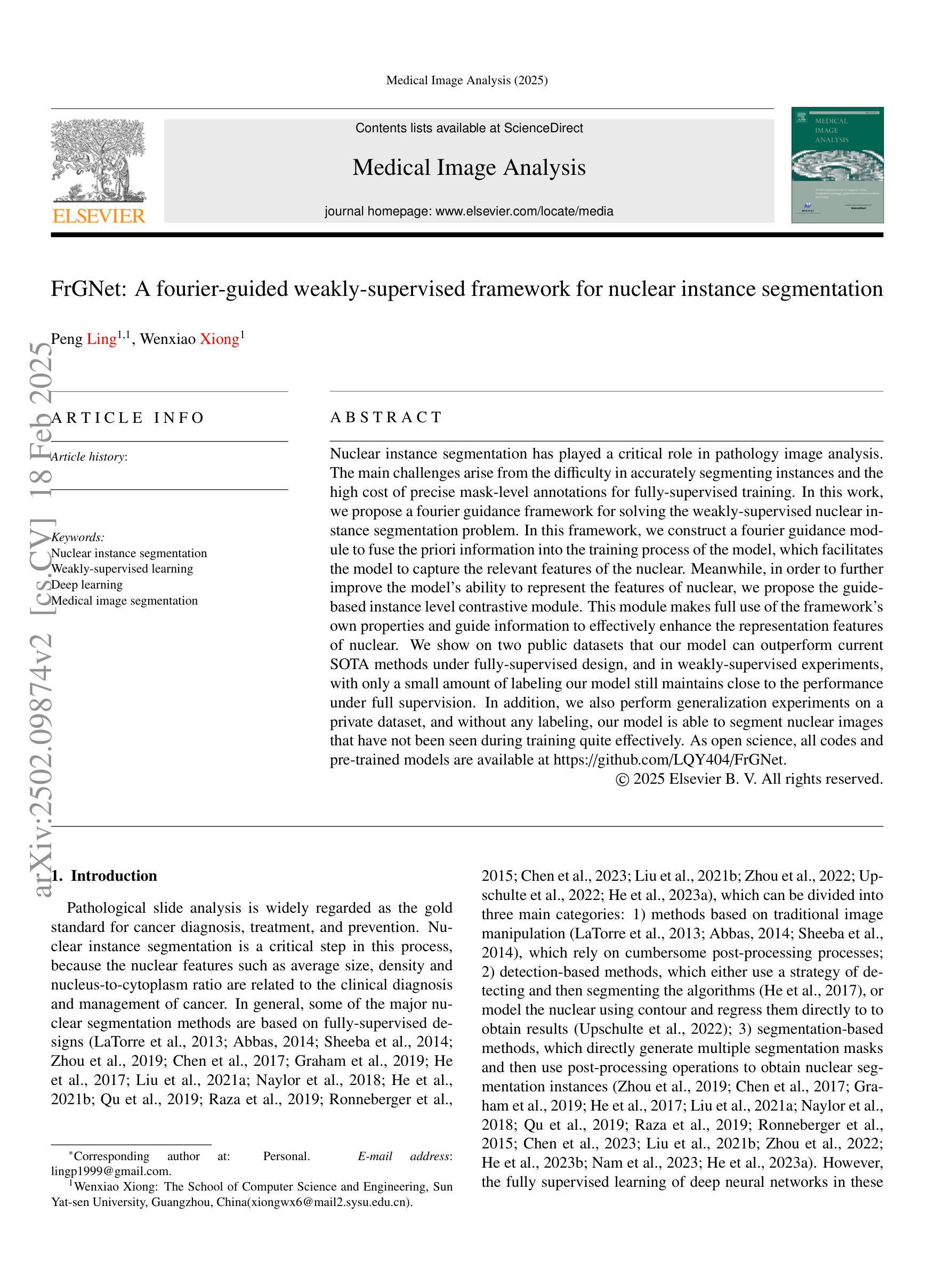
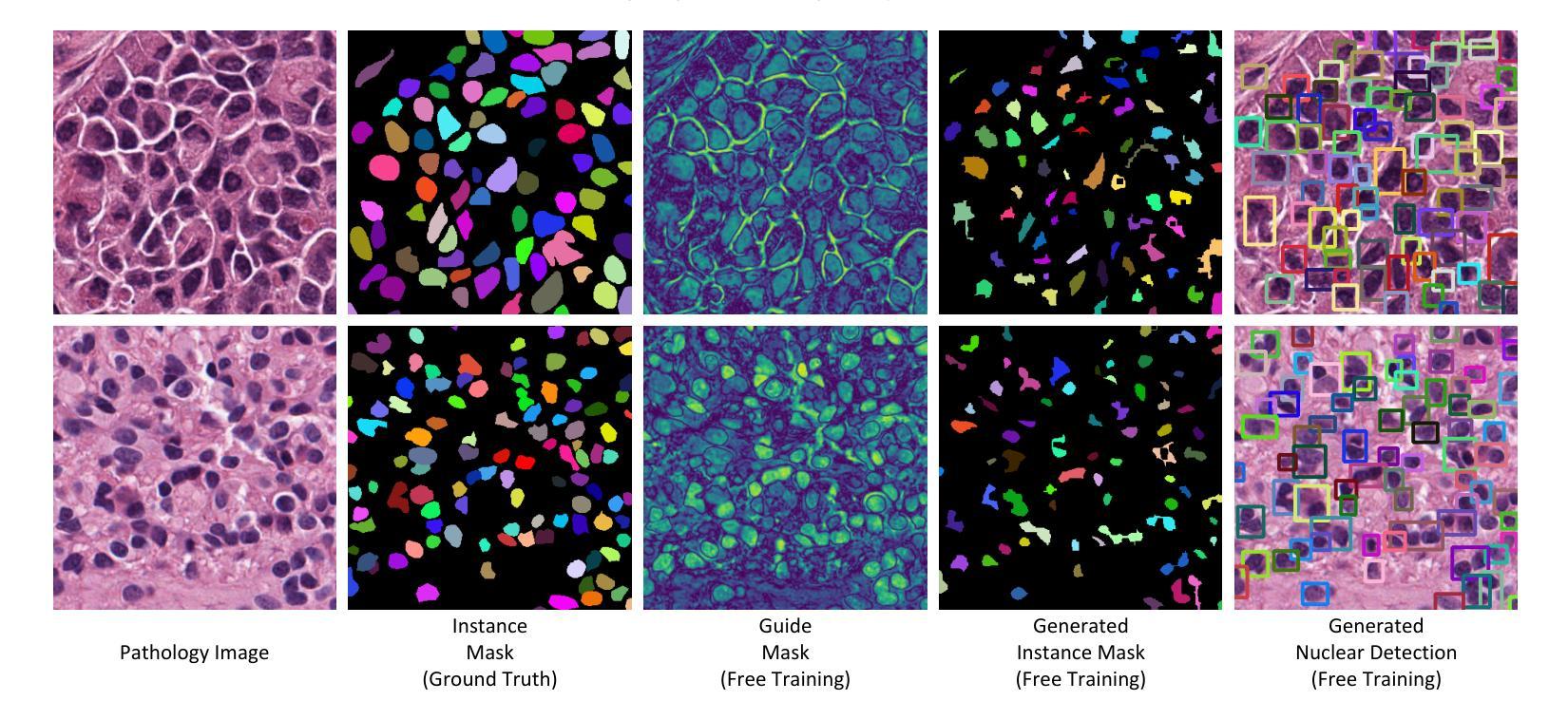
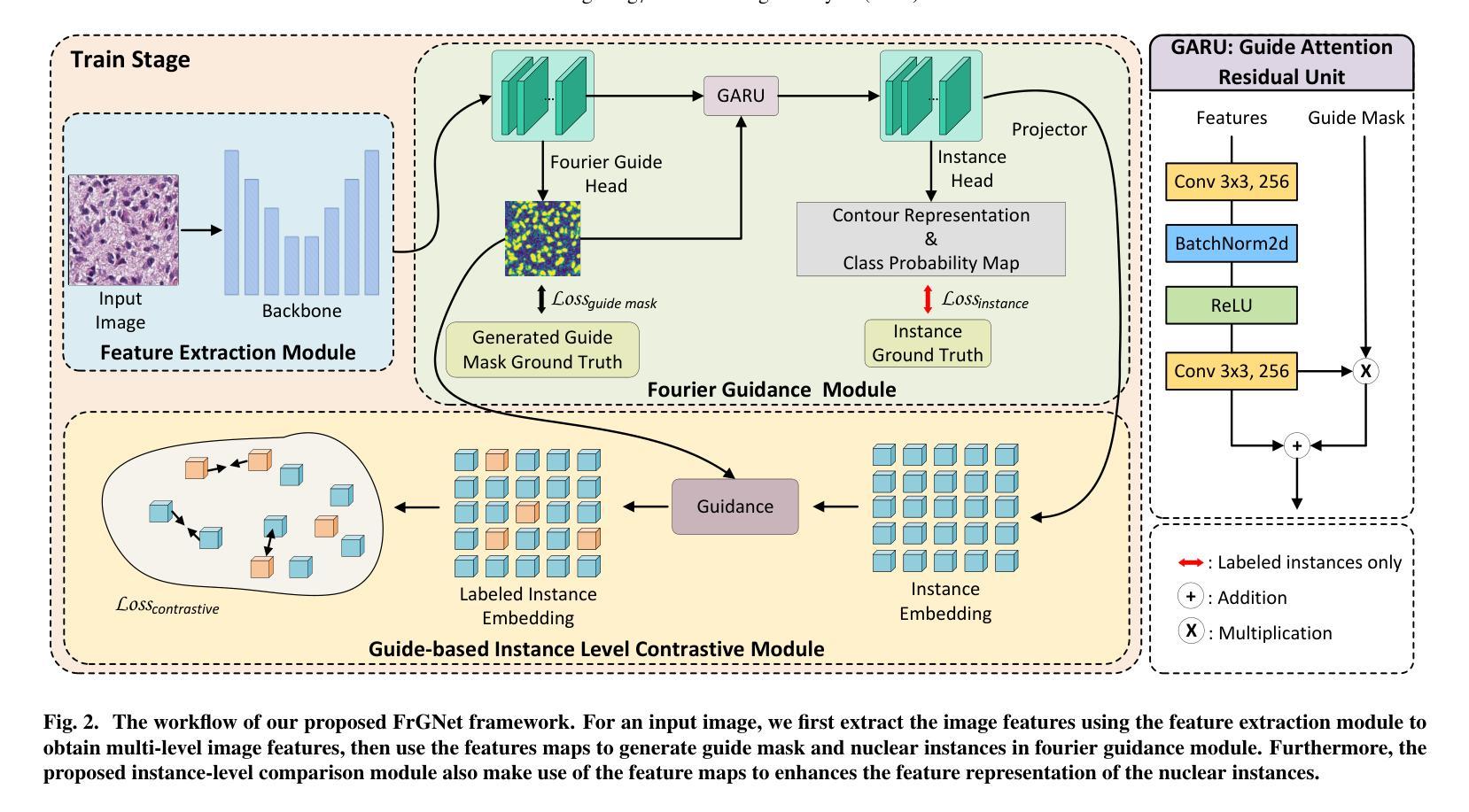
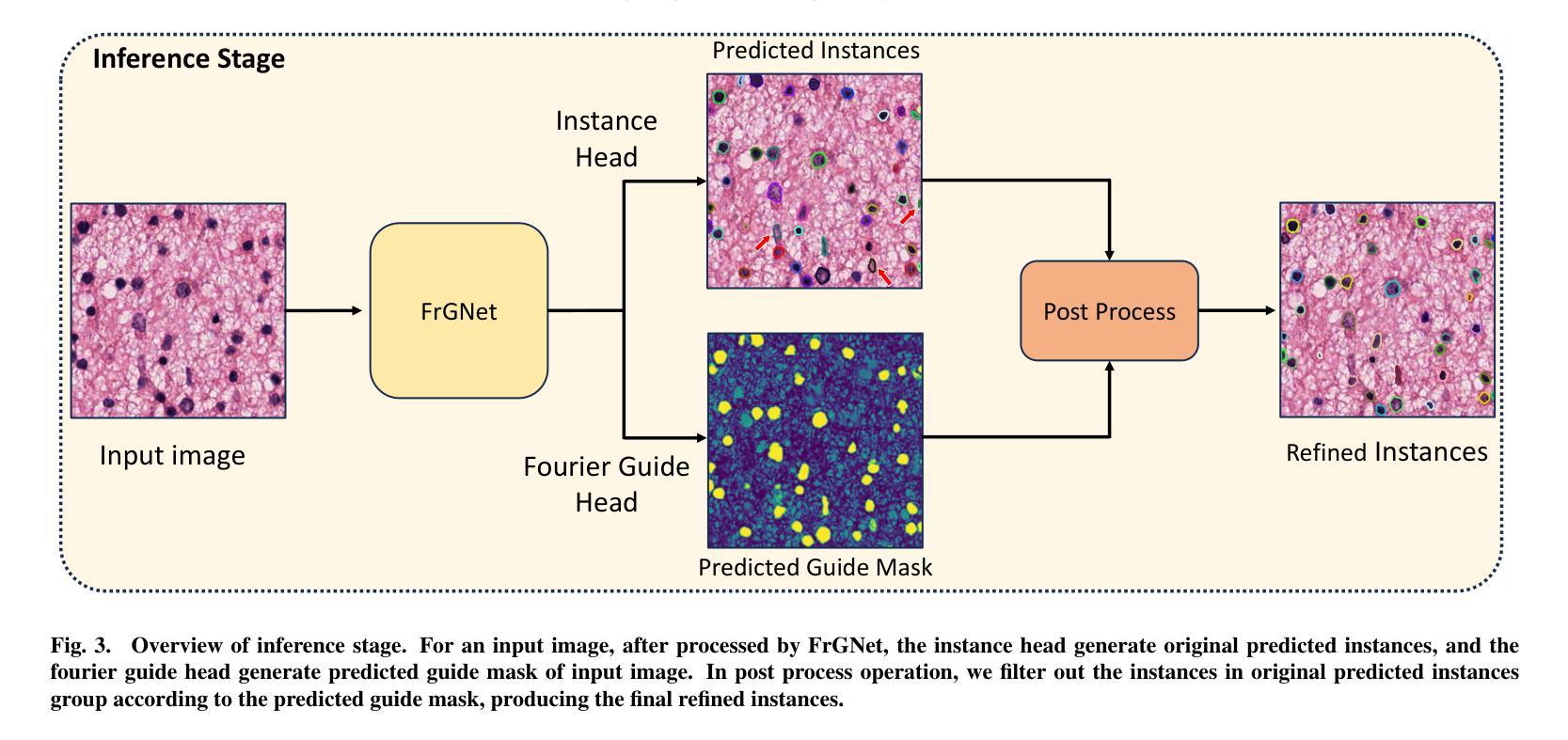