⚠️ 以下所有内容总结都来自于 大语言模型的能力,如有错误,仅供参考,谨慎使用
🔴 请注意:千万不要用于严肃的学术场景,只能用于论文阅读前的初筛!
💗 如果您觉得我们的项目对您有帮助 ChatPaperFree ,还请您给我们一些鼓励!⭐️ HuggingFace免费体验
2025-02-20 更新
Is Noise Conditioning Necessary for Denoising Generative Models?
Authors:Qiao Sun, Zhicheng Jiang, Hanhong Zhao, Kaiming He
It is widely believed that noise conditioning is indispensable for denoising diffusion models to work successfully. This work challenges this belief. Motivated by research on blind image denoising, we investigate a variety of denoising-based generative models in the absence of noise conditioning. To our surprise, most models exhibit graceful degradation, and in some cases, they even perform better without noise conditioning. We provide a theoretical analysis of the error caused by removing noise conditioning and demonstrate that our analysis aligns with empirical observations. We further introduce a noise-unconditional model that achieves a competitive FID of 2.23 on CIFAR-10, significantly narrowing the gap to leading noise-conditional models. We hope our findings will inspire the community to revisit the foundations and formulations of denoising generative models.
普遍认为噪声调节对于去噪扩散模型的成功运作是必不可少的。然而,这项工作对此提出了质疑。受盲图像去噪研究的启发,我们调查了没有噪声调节的各种基于去噪的生成模型。令人惊讶的是,大多数模型表现出了优雅的退化性能,并且在某些情况下,它们在无需噪声调节的情况下表现更佳。我们对因去除噪声调节而产生的误差进行了理论分析,并证明我们的分析与观察到的现象相符。我们还引入了一种无需噪声调节的模型,在CIFAR-10上实现了竞争性的FID分数2.23,显著缩小了与领先的噪声条件模型的差距。我们希望我们的发现能激励社区重新思考去噪生成模型的基础和公式。
论文及项目相关链接
Summary
本文挑战了广泛认为噪声调节对于降噪扩散模型成功运作不可或缺的观点。通过借鉴盲图像降噪研究,本文探索了没有噪声调节的基于降噪的生成模型。结果显示,大多数模型在去除噪声调节后仍能优雅地降级,某些情况下性能甚至更佳。本文提供了移除噪声调节所引起的误差的理论分析,并证明该分析与实证观察相符。此外,介绍了一个无需噪声调节的模型,在CIFAR-10上实现了竞争性的FID分数,显著缩小了与领先的噪声条件模型的差距。
Key Takeaways
- 挑战了广泛认为噪声调节对于降噪扩散模型成功运作不可或缺的观点。
- 通过借鉴盲图像降噪研究,在没有噪声调节的情况下探索了基于降噪的生成模型。
- 大多数模型在去除噪声调节后仍能优雅地降级。
- 在某些情况下,模型在无需噪声调节的情况下性能更佳。
- 提供了移除噪声调节所引起的误差的理论分析。
- 实证观察与理论分析相符。
点此查看论文截图
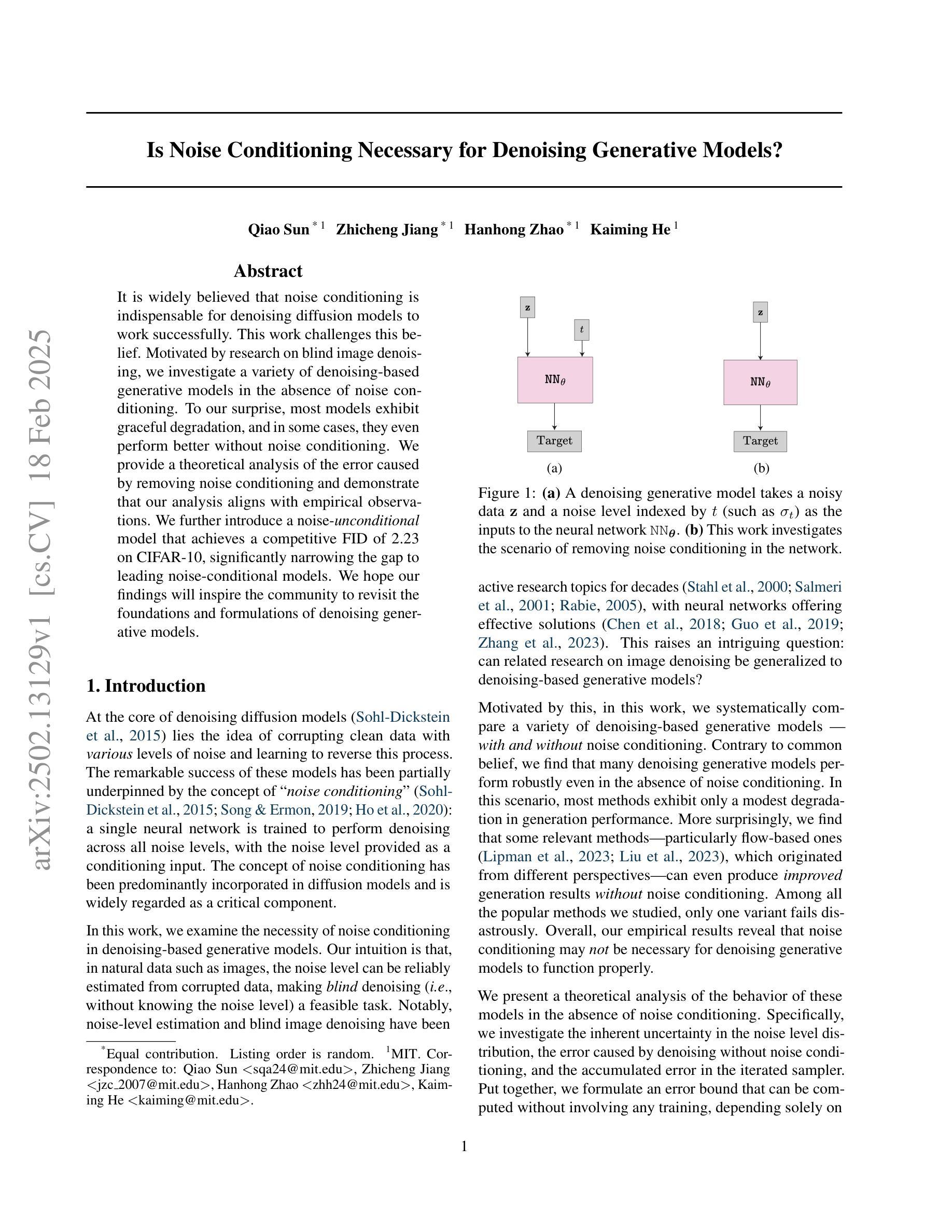
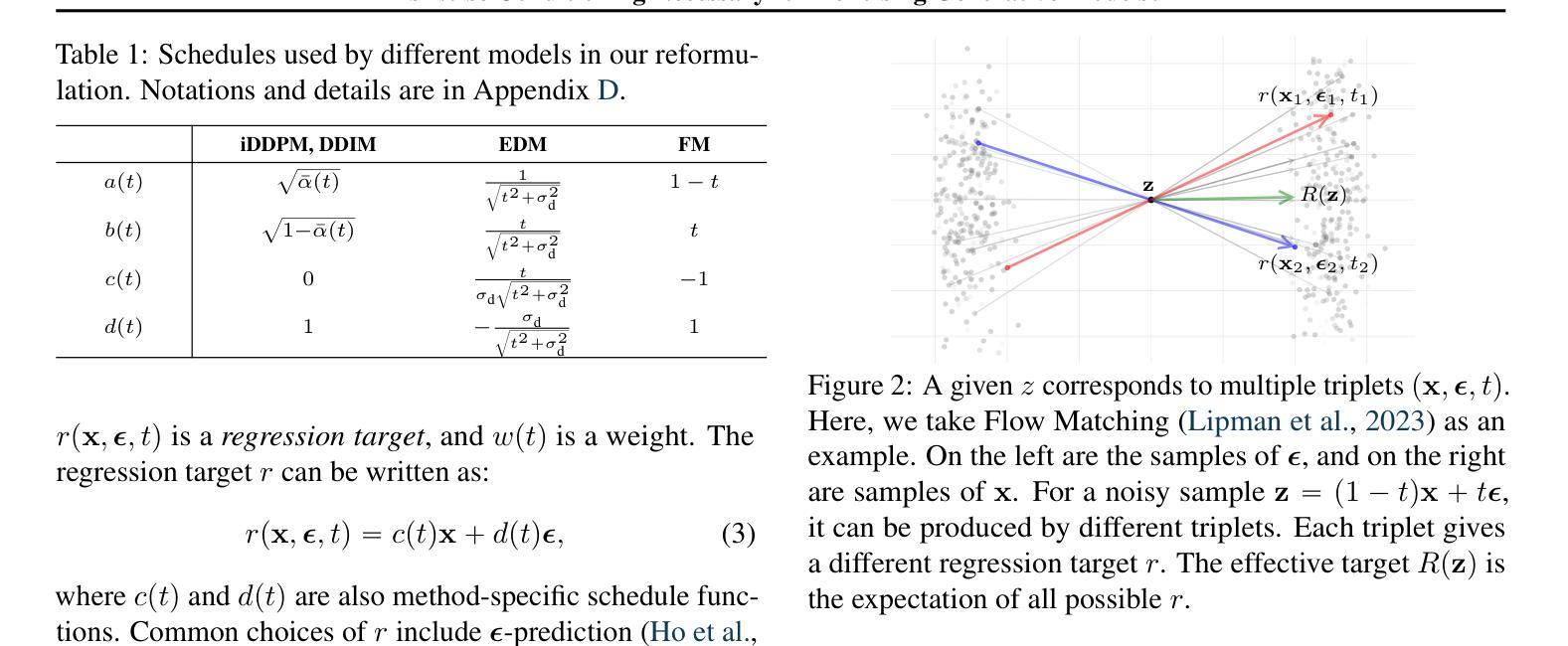
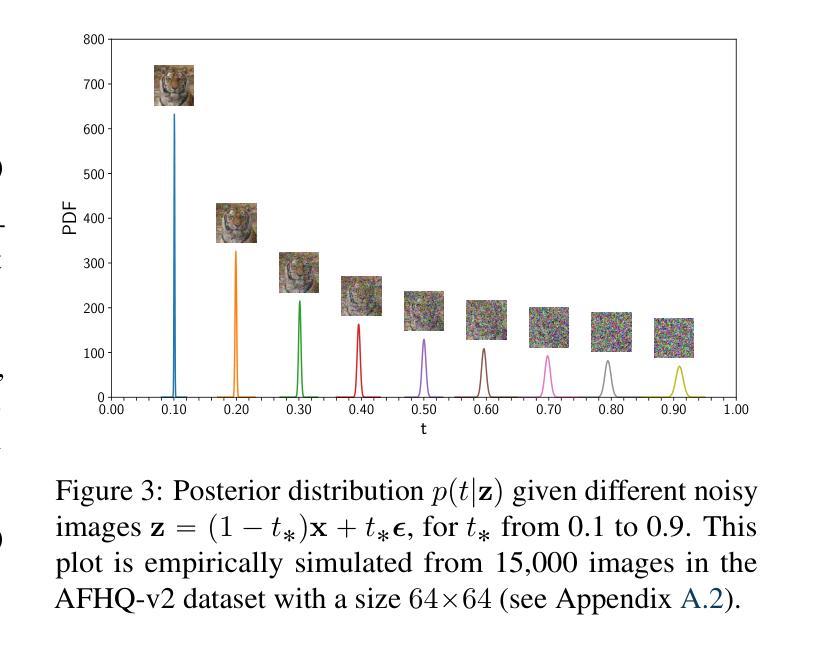
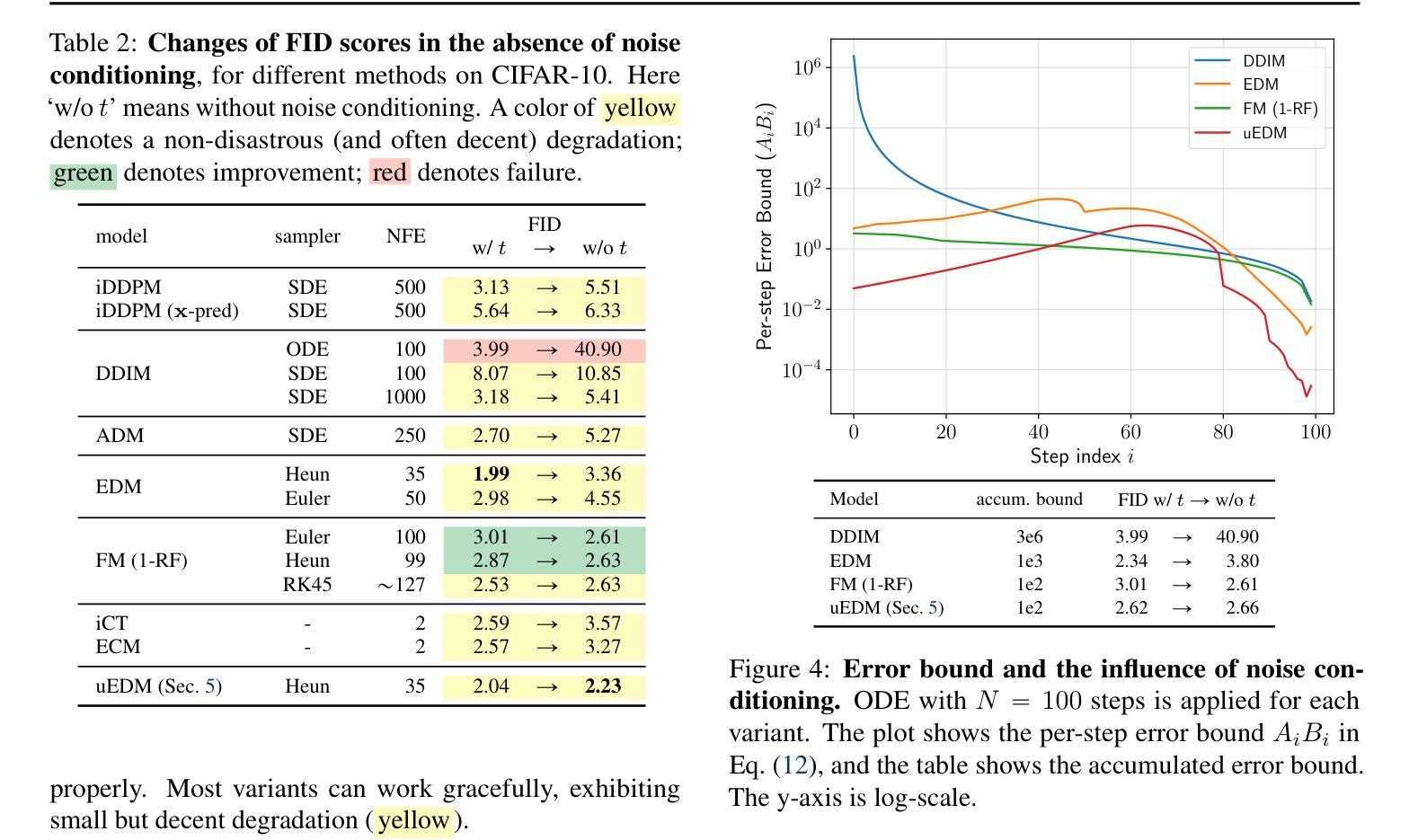
Personalized Image Generation with Deep Generative Models: A Decade Survey
Authors:Yuxiang Wei, Yiheng Zheng, Yabo Zhang, Ming Liu, Zhilong Ji, Lei Zhang, Wangmeng Zuo
Recent advancements in generative models have significantly facilitated the development of personalized content creation. Given a small set of images with user-specific concept, personalized image generation allows to create images that incorporate the specified concept and adhere to provided text descriptions. Due to its wide applications in content creation, significant effort has been devoted to this field in recent years. Nonetheless, the technologies used for personalization have evolved alongside the development of generative models, with their distinct and interrelated components. In this survey, we present a comprehensive review of generalized personalized image generation across various generative models, including traditional GANs, contemporary text-to-image diffusion models, and emerging multi-model autoregressive models. We first define a unified framework that standardizes the personalization process across different generative models, encompassing three key components, i.e., inversion spaces, inversion methods, and personalization schemes. This unified framework offers a structured approach to dissecting and comparing personalization techniques across different generative architectures. Building upon this unified framework, we further provide an in-depth analysis of personalization techniques within each generative model, highlighting their unique contributions and innovations. Through comparative analysis, this survey elucidates the current landscape of personalized image generation, identifying commonalities and distinguishing features among existing methods. Finally, we discuss the open challenges in the field and propose potential directions for future research. We keep tracing related works at https://github.com/csyxwei/Awesome-Personalized-Image-Generation.
近年来,生成模型的进步极大地促进了个性化内容创作的发展。给定一组包含用户特定概念的小图像,个性化图像生成能够创建融入指定概念并符合提供的文本描述的图像。由于其内容创作的广泛应用,近年来人们对此领域的投入非常大。然而,个性化技术随着生成模型的发展而演变,它们各自独特的组件相互关联。在这篇综述中,我们对各种生成模型中的通用个性化图像生成进行了全面回顾,包括传统的GANs、当代的文本到图像扩散模型以及新兴的多模式自回归模型。我们首先定义了一个统一的框架,标准化了不同生成模型中的个性化过程,包括三个关键组件,即反转空间、反转方法和个性化方案。这一统一框架提供了一种结构化方法来分析和比较不同生成架构中的个性化技术。基于这一统一框架,我们进一步深入分析了每个生成模型中的个性化技术,突出了其独特贡献和创新之处。通过比较分析,这篇综述阐明了个性化图像生成的当前状况,识别了现有方法的共同点和区别特征。最后,我们讨论了该领域的开放挑战以及未来研究的潜在方向。我们持续追踪相关作品,可访问:https://github.com/csyxwei/Awesome-Personalized-Image-Generation进行查看。
论文及项目相关链接
PDF 39 pages; under submission; more information: https://github.com/csyxwei/Awesome-Personalized-Image-Generation
摘要
个性化图像生成:基于先进生成模型的技术进展。该研究综述了不同生成模型中的个性化图像生成技术,包括传统GANs、现代文本到图像扩散模型以及新兴的多模态自回归模型。文章定义了一个统一框架,标准化不同生成模型中的个性化过程,包括反转空间、反转方法和个性化方案三个关键组件。基于此框架,深入分析了每个生成模型中的个性化技术,并比较了现有方法的共性和区别。该综述指出了个性化图像生成领域的当前状况,并探讨了开放挑战和未来研究方向。
要点掌握
- 生成模型的最新进展推动了个性化内容创建的便利化。
- 个性化图像生成能够根据用户特定概念和文本描述创建符合要求的图像。
- 技术发展与生成模型的演进息息相关,具有其独特且相互关联的组件。
- 文章提出了一个统一框架来标准化不同生成模型中的个性化过程,包括反转空间、反转方法和个性化方案。
- 对不同生成模型中的个性化技术进行了深入分析,并比较了现有方法的共性和区别。
- 综述指出了个性化图像生成领域的挑战,并提供了潜在的研究方向。
- 可以通过提供的链接追踪相关领域的研究进展。
点此查看论文截图
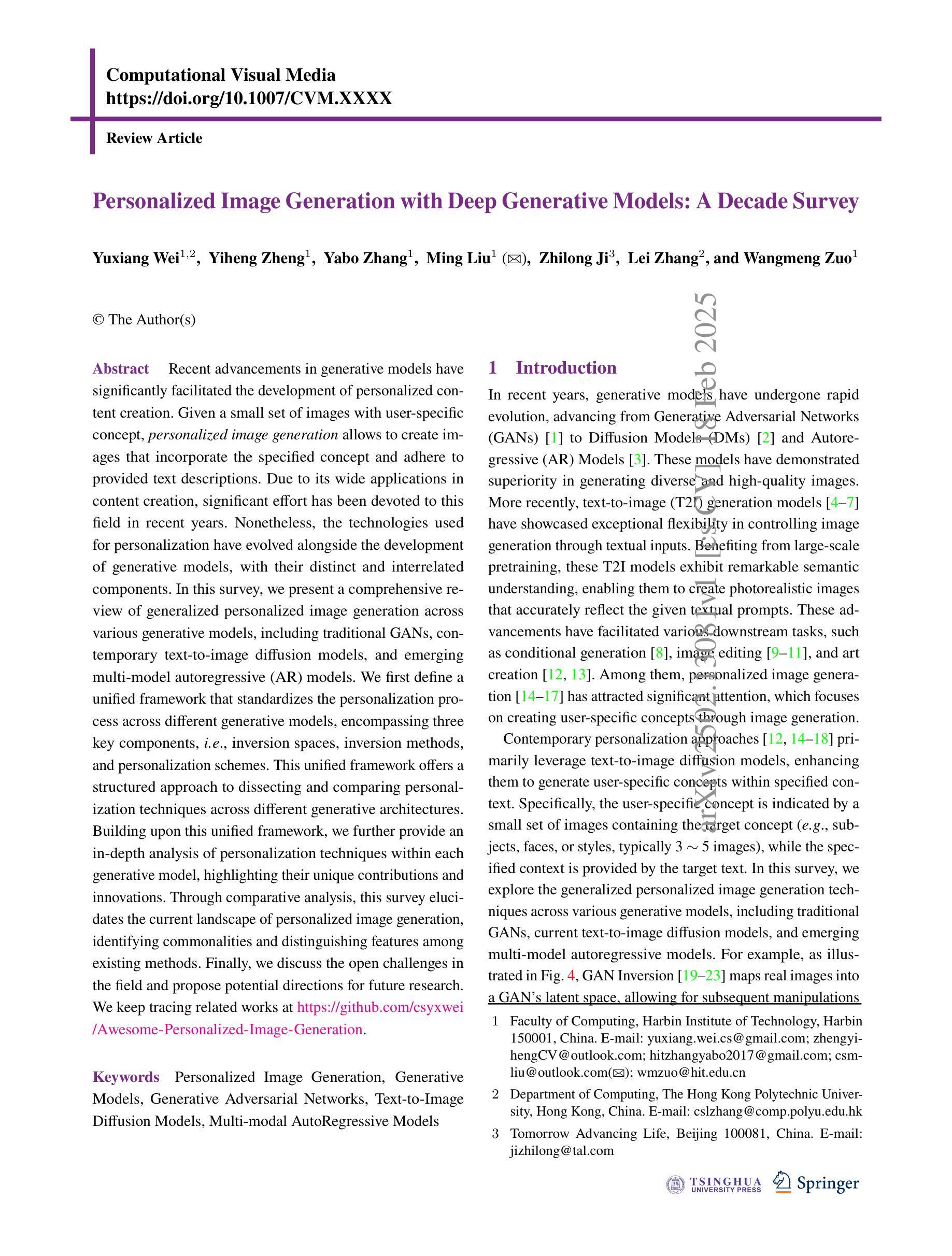
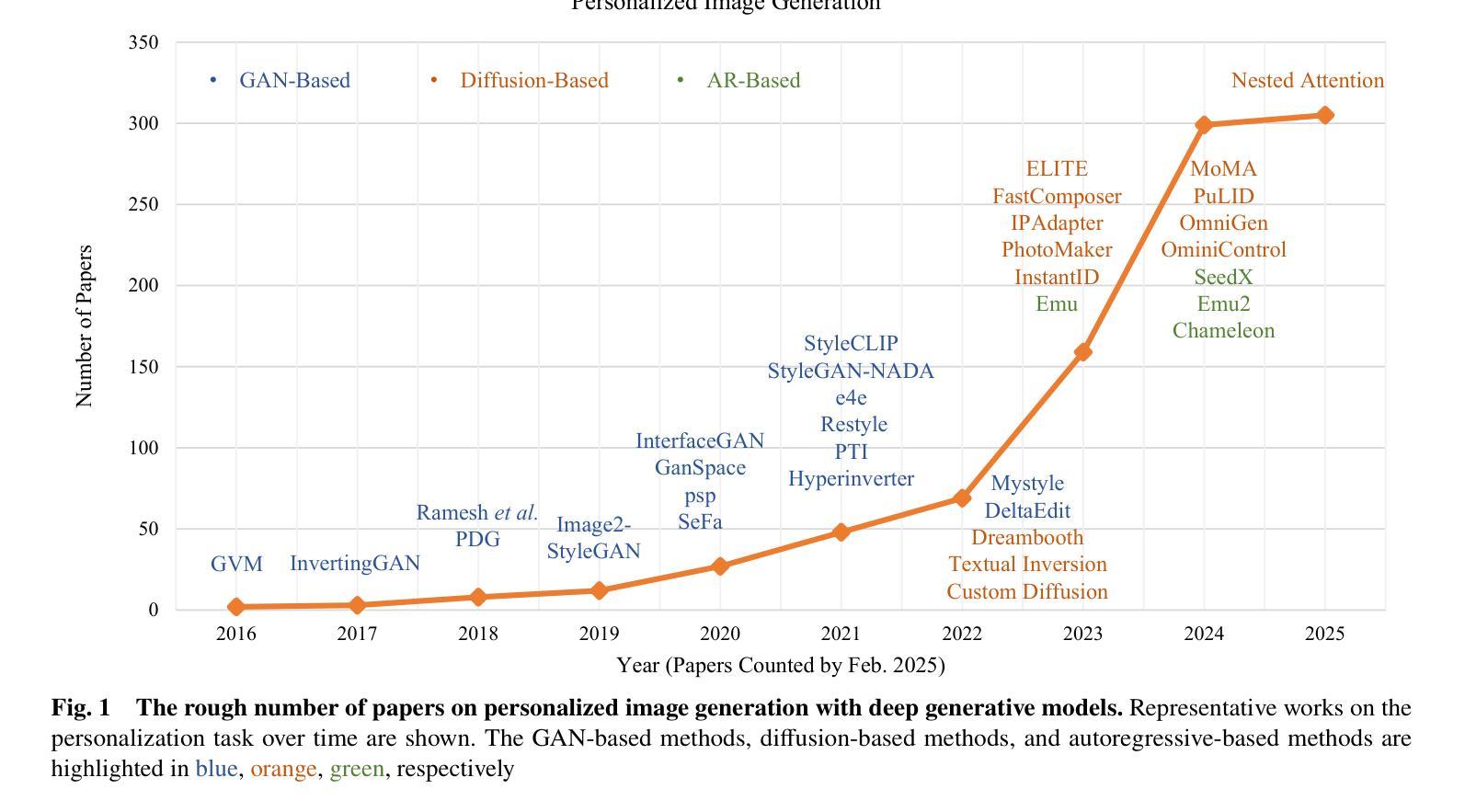
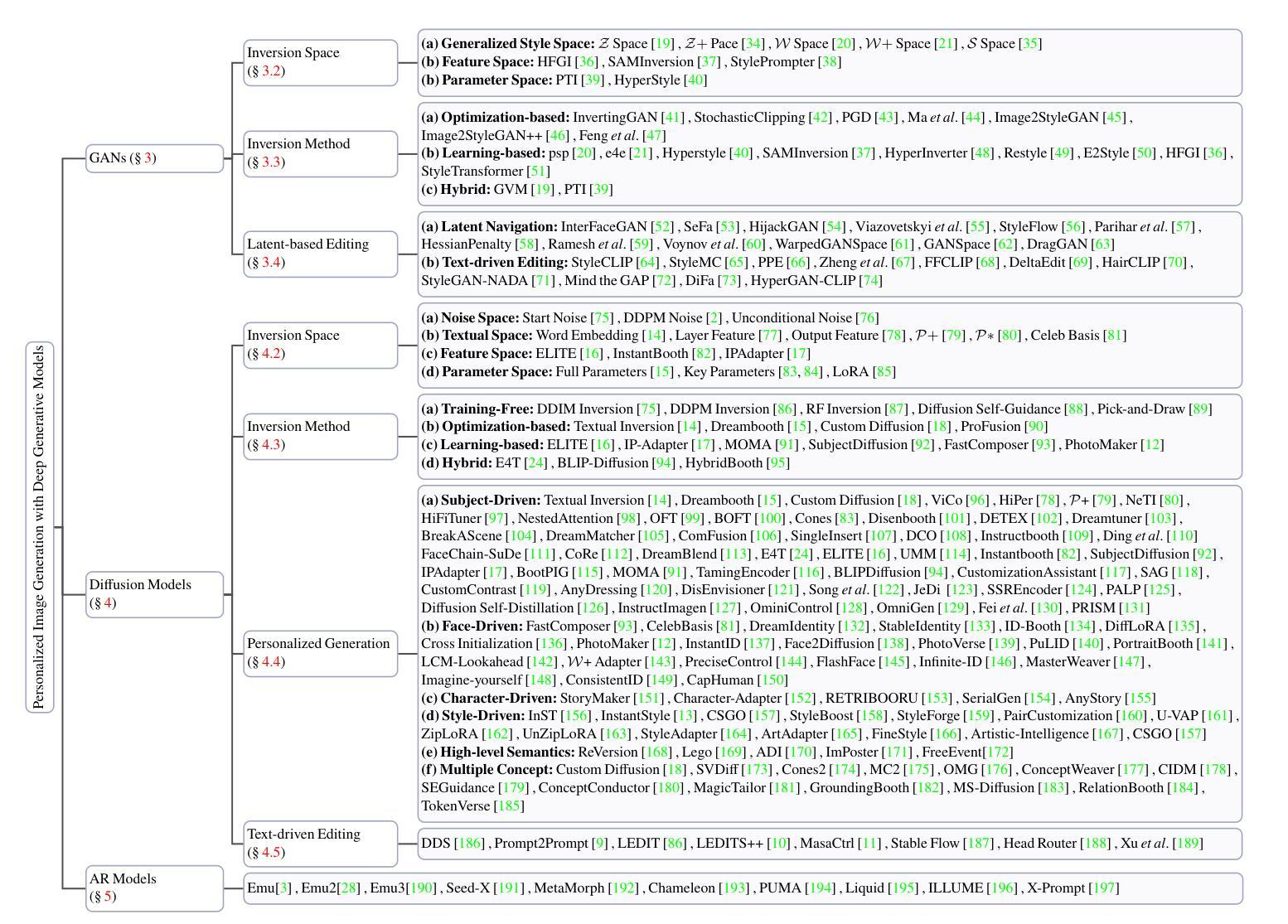
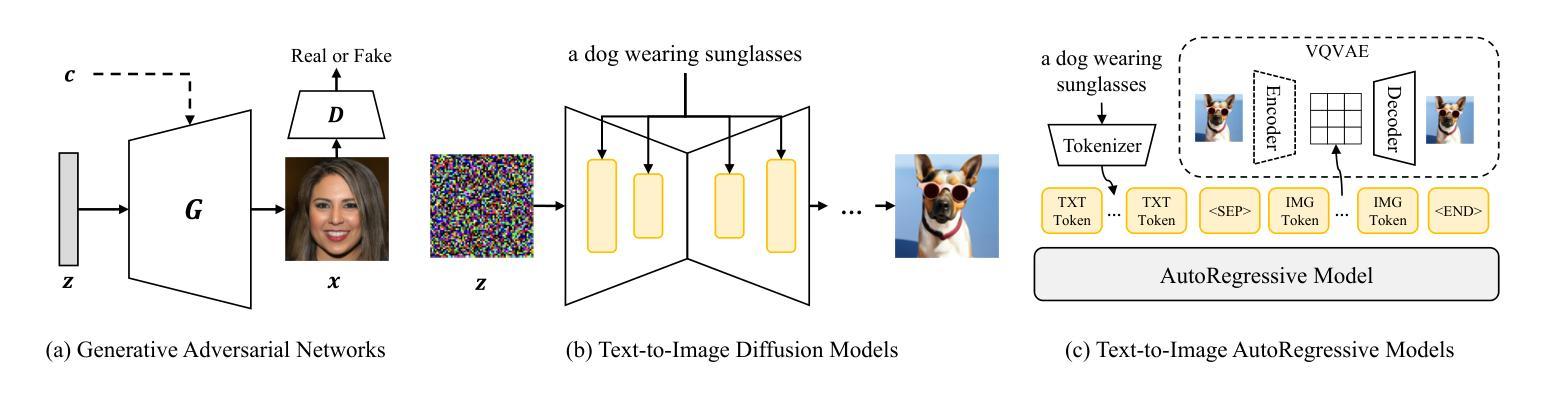
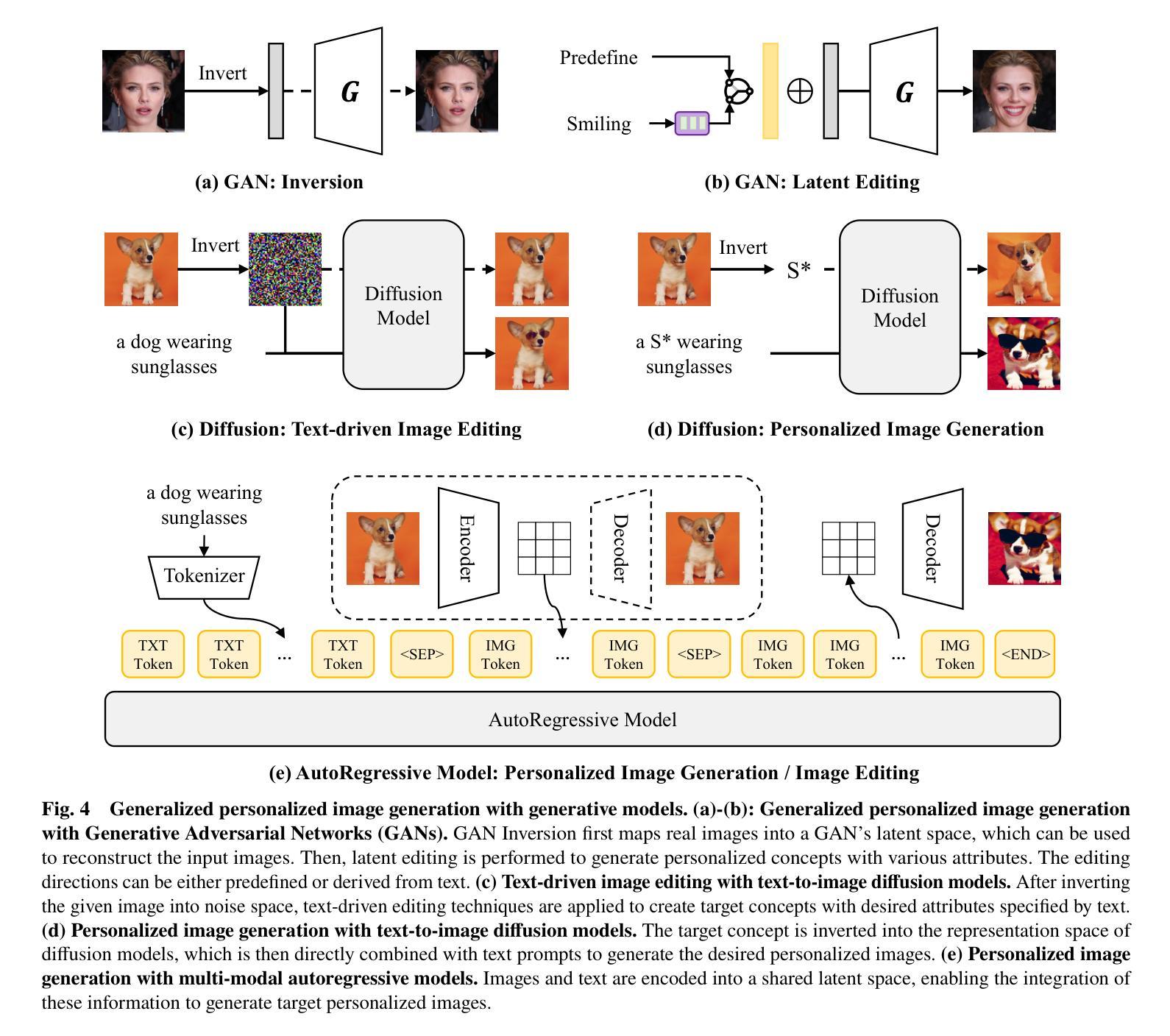
High-Fidelity Novel View Synthesis via Splatting-Guided Diffusion
Authors:Xiang Zhang, Yang Zhang, Lukas Mehl, Markus Gross, Christopher Schroers
Despite recent advances in Novel View Synthesis (NVS), generating high-fidelity views from single or sparse observations remains a significant challenge. Existing splatting-based approaches often produce distorted geometry due to splatting errors. While diffusion-based methods leverage rich 3D priors to achieve improved geometry, they often suffer from texture hallucination. In this paper, we introduce SplatDiff, a pixel-splatting-guided video diffusion model designed to synthesize high-fidelity novel views from a single image. Specifically, we propose an aligned synthesis strategy for precise control of target viewpoints and geometry-consistent view synthesis. To mitigate texture hallucination, we design a texture bridge module that enables high-fidelity texture generation through adaptive feature fusion. In this manner, SplatDiff leverages the strengths of splatting and diffusion to generate novel views with consistent geometry and high-fidelity details. Extensive experiments verify the state-of-the-art performance of SplatDiff in single-view NVS. Additionally, without extra training, SplatDiff shows remarkable zero-shot performance across diverse tasks, including sparse-view NVS and stereo video conversion.
尽管最近在新型视图合成(NVS)方面取得了进展,但从单个或稀疏观察生成高保真视图仍然是一个重大挑战。现有的基于平铺的方法由于平铺错误通常会产生几何失真。虽然基于扩散的方法利用丰富的3D先验知识来实现改进的几何效果,但它们常常会出现纹理幻觉。在本文中,我们介绍了SplatDiff,这是一种由像素平铺引导的视频扩散模型,旨在从单个图像合成高保真新型视图。具体来说,我们提出了一种对齐合成策略,以实现目标观点的精确控制和几何一致的视图合成。为了减轻纹理幻觉,我们设计了一个纹理桥梁模块,通过自适应特征融合实现高保真纹理生成。通过这种方式,SplatDiff结合了平铺和扩散的优点,生成了具有一致几何形状和高保真细节的新型视图。大量实验验证了SplatDiff在单视图NVS中的最新性能。此外,无需额外训练,SplatDiff在不同任务中表现出了卓越的零样本性能,包括稀疏视图NVS和立体声视频转换。
论文及项目相关链接
Summary
本文提出了SplatDiff模型,这是一种结合像素点扩散和视图合成技术的方法,旨在从单一图像中合成高质量的新视角。通过采用对齐合成策略,实现了对目标视点的精确控制和几何一致的视图合成。设计了一种纹理桥梁模块,以通过自适应特征融合来减轻纹理幻觉。因此,SplatDiff结合了扩散和点云技术的优点,生成了具有一致几何结构和高质量细节的新视角。在单视角视图合成中,其性能处于领先水平,且在稀疏视角视图合成和立体视频转换等任务中展现出卓越表现。
Key Takeaways
- SplatDiff是一种结合了像素点扩散和视图合成技术的模型,旨在从单一图像生成高质量的新视角。
- 通过采用对齐合成策略,实现了对目标视点的精确控制。
- 设计了一种纹理桥梁模块,以减轻纹理幻觉,提高纹理生成的准确性。
- SplatDiff结合了扩散和点云技术的优点,生成的新视角具有一致的几何结构和高质量细节。
- 该模型在单视角视图合成任务中表现出色,达到了领先水平。
- SplatDiff在稀疏视角视图合成和立体视频转换等任务中展现出卓越表现。
点此查看论文截图
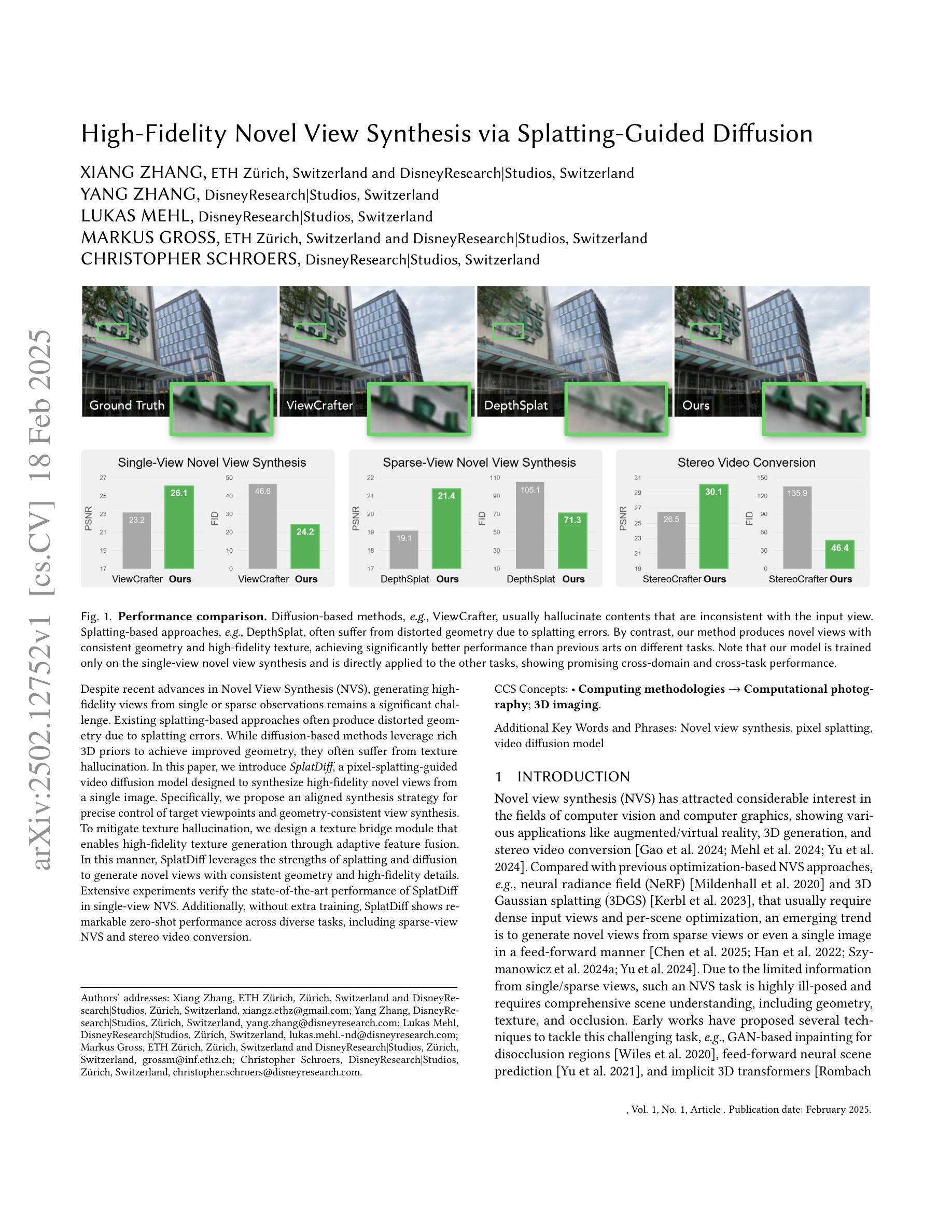
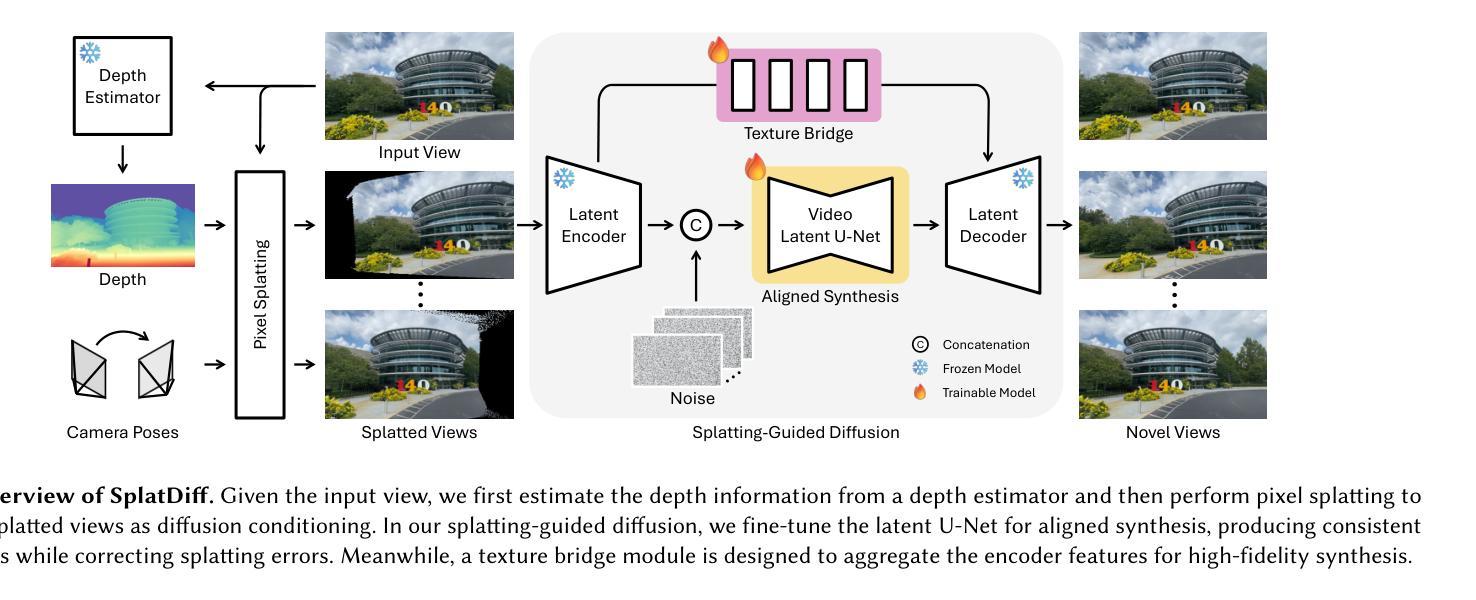
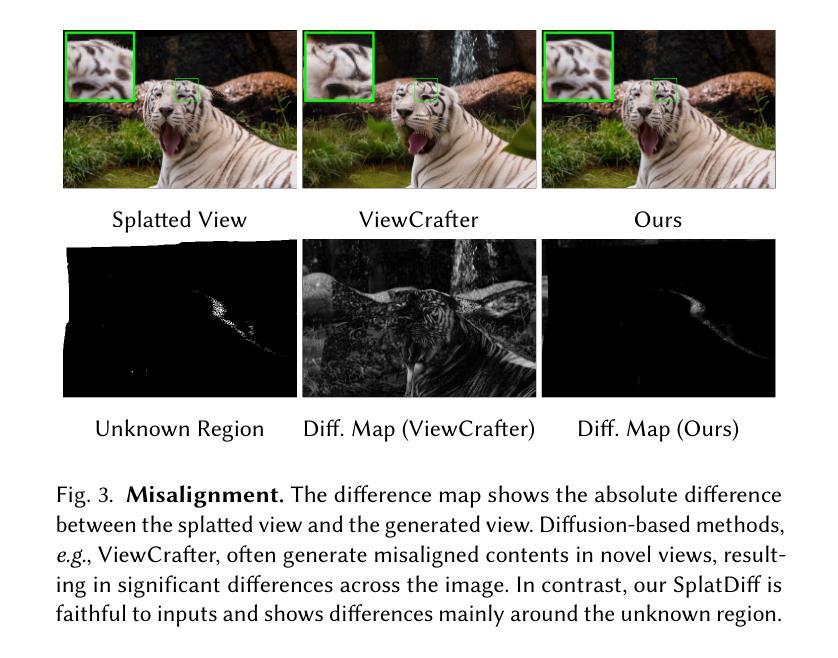
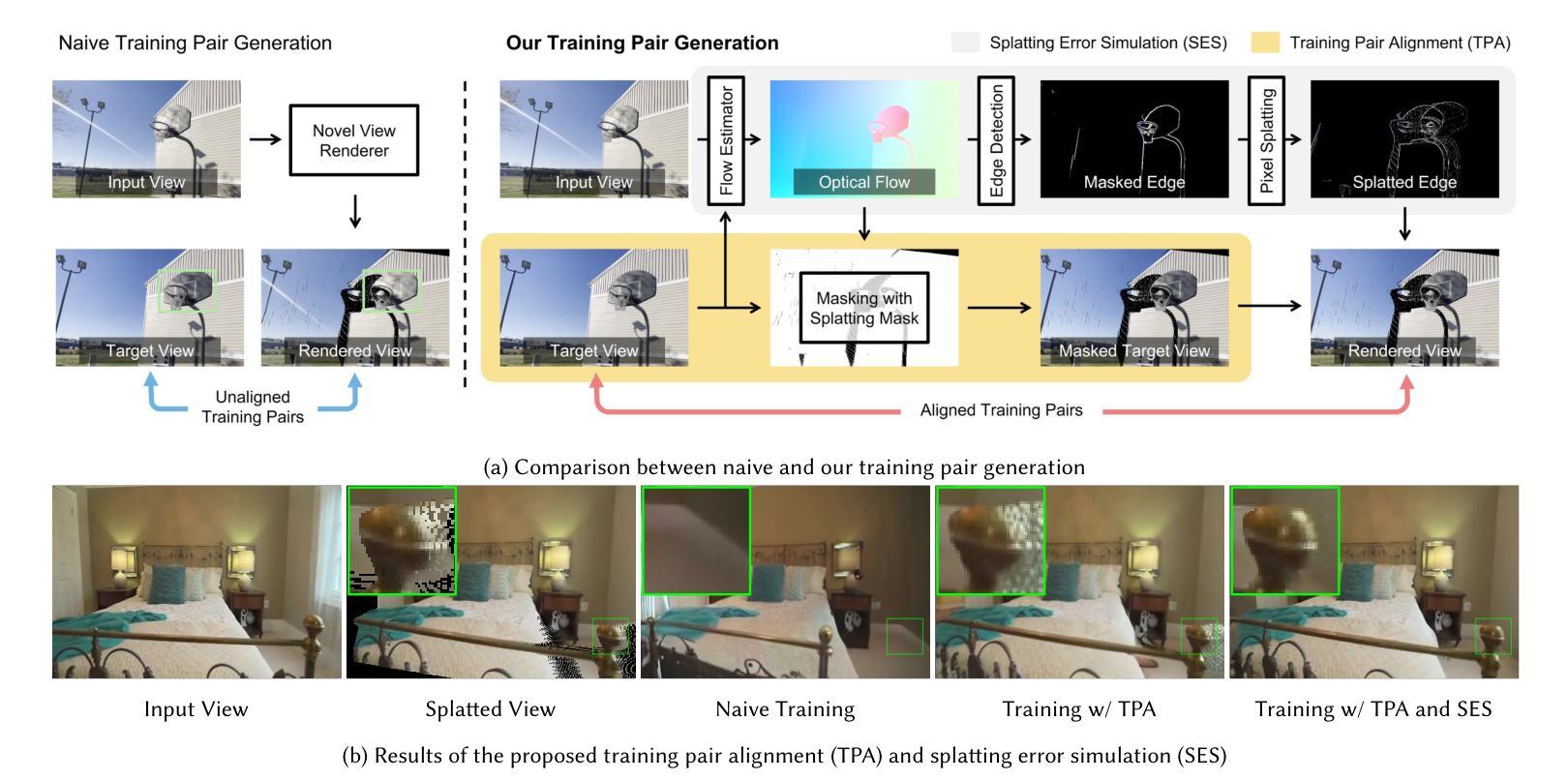
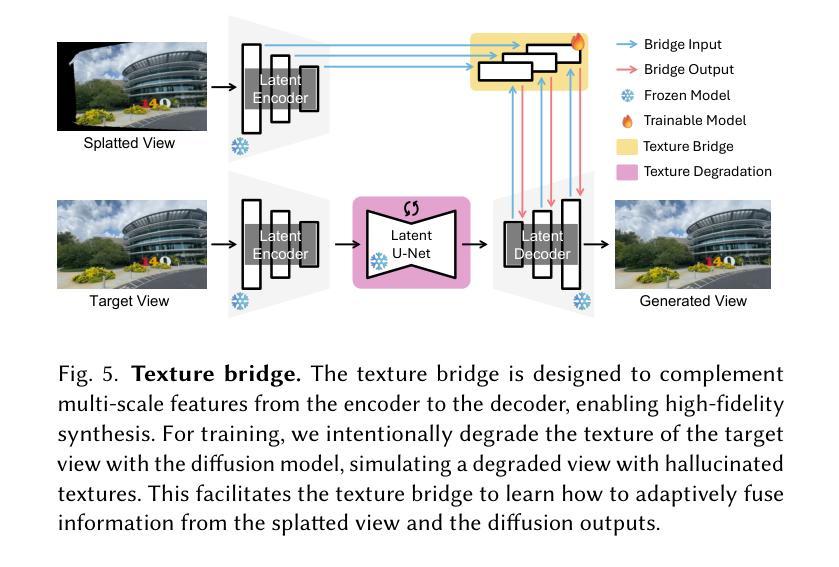
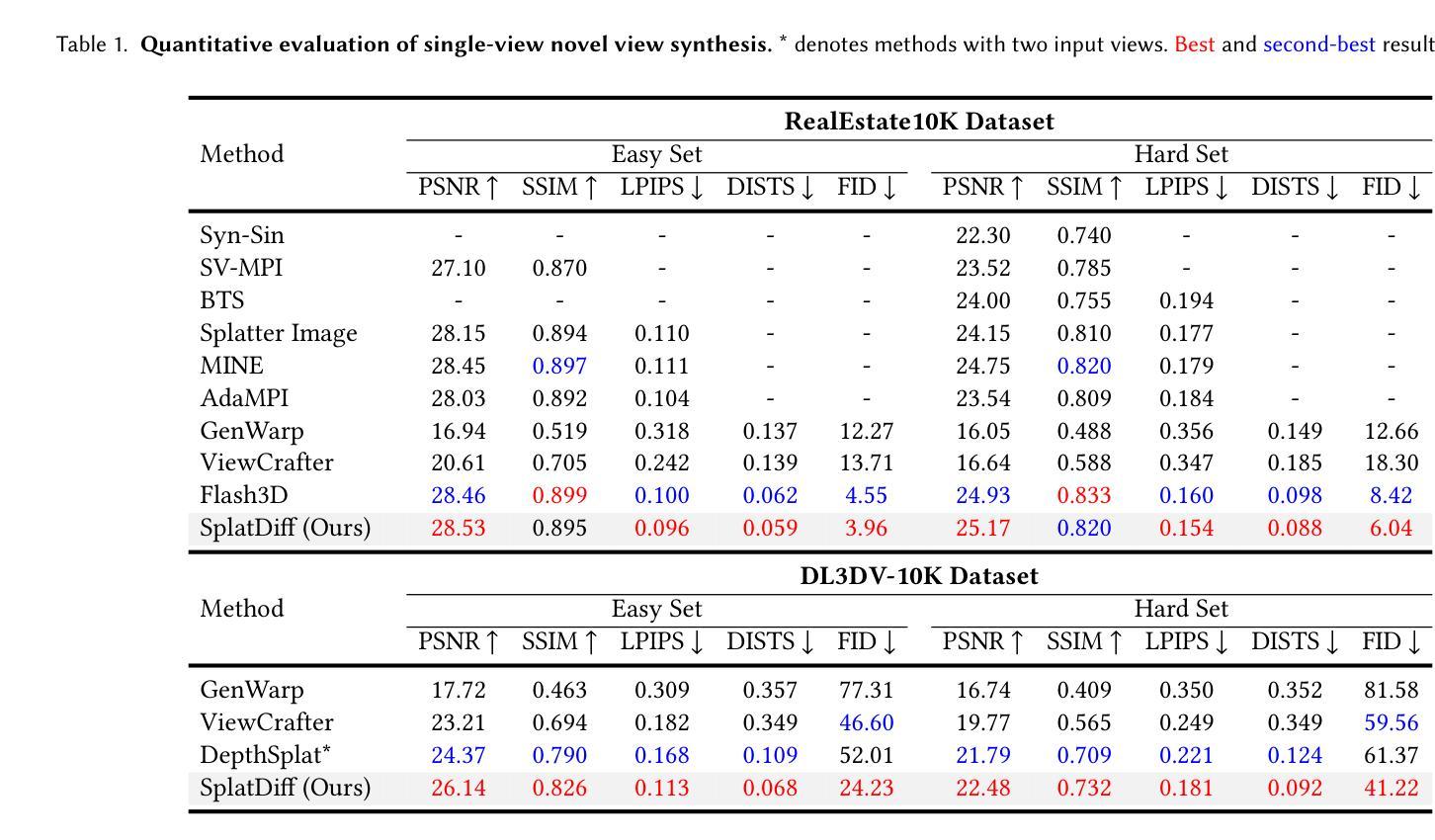
3D Shape-to-Image Brownian Bridge Diffusion for Brain MRI Synthesis from Cortical Surfaces
Authors:Fabian Bongratz, Yitong Li, Sama Elbaroudy, Christian Wachinger
Despite recent advances in medical image generation, existing methods struggle to produce anatomically plausible 3D structures. In synthetic brain magnetic resonance images (MRIs), characteristic fissures are often missing, and reconstructed cortical surfaces appear scattered rather than densely convoluted. To address this issue, we introduce Cor2Vox, the first diffusion model-based method that translates continuous cortical shape priors to synthetic brain MRIs. To achieve this, we leverage a Brownian bridge process which allows for direct structured mapping between shape contours and medical images. Specifically, we adapt the concept of the Brownian bridge diffusion model to 3D and extend it to embrace various complementary shape representations. Our experiments demonstrate significant improvements in the geometric accuracy of reconstructed structures compared to previous voxel-based approaches. Moreover, Cor2Vox excels in image quality and diversity, yielding high variation in non-target structures like the skull. Finally, we highlight the capability of our approach to simulate cortical atrophy at the sub-voxel level. Our code is available at https://github.com/ai-med/Cor2Vox.
尽管在医学图像生成方面取得了最新的进展,但现有方法仍然难以生成解剖上合理的3D结构。在合成的大脑磁共振成像(MRI)中,特征裂口往往缺失,重建的皮层表面看起来是散乱的,而不是密集卷叠的。为了解决这个问题,我们引入了Cor2Vox,这是基于扩散模型的方法,它将连续的皮层形状先验知识转化为合成的大脑MRI。为了实现这一点,我们利用布朗桥过程,允许形状轮廓和医学图像之间的直接结构化映射。具体来说,我们将布朗桥扩散模型的概念适应到3D,并将其扩展到包含各种互补的形状表示。我们的实验表明,与之前的体素化方法相比,重建结构的几何精度有了显著提高。此外,Cor2Vox在图像质量和多样性方面表现出色,非目标结构(如颅骨)的高变异度得以实现。最后,我们强调了我们的方法在亚体素级别模拟皮层萎缩的能力。我们的代码可在https://github.com/ai-med/Cor2Vox找到。
论文及项目相关链接
PDF Accepted by Information Processing in Medical Imaging (IPMI) 2025
Summary
本文介绍了Cor2Vox,首个基于扩散模型的合成脑MRI生成方法,通过将连续的皮质形状先验知识应用于合成脑MRI中,解决了现有方法在生成具有解剖结构合理性的三维结构方面的问题。利用布朗桥过程实现了形状轮廓与医学图像之间的直接结构映射,显著提高了重建结构的几何精度。
Key Takeaways
- Cor2Vox是首个利用扩散模型解决医学图像生成中解剖结构合理性问题的技术。
- 通过引入布朗桥过程,实现了形状轮廓与医学图像之间的直接结构映射。
- Cor2Vox成功将连续的皮质形状先验知识应用于合成脑MRI中。
- 与传统的像素级方法相比,Cor2Vox在几何精度上显著提高。
- Cor2Vox在图像质量和多样性方面表现出色,对非目标结构如颅骨的高变异性能进行模拟。
- Cor2Vox具备模拟皮质萎缩的亚像素级能力。
点此查看论文截图
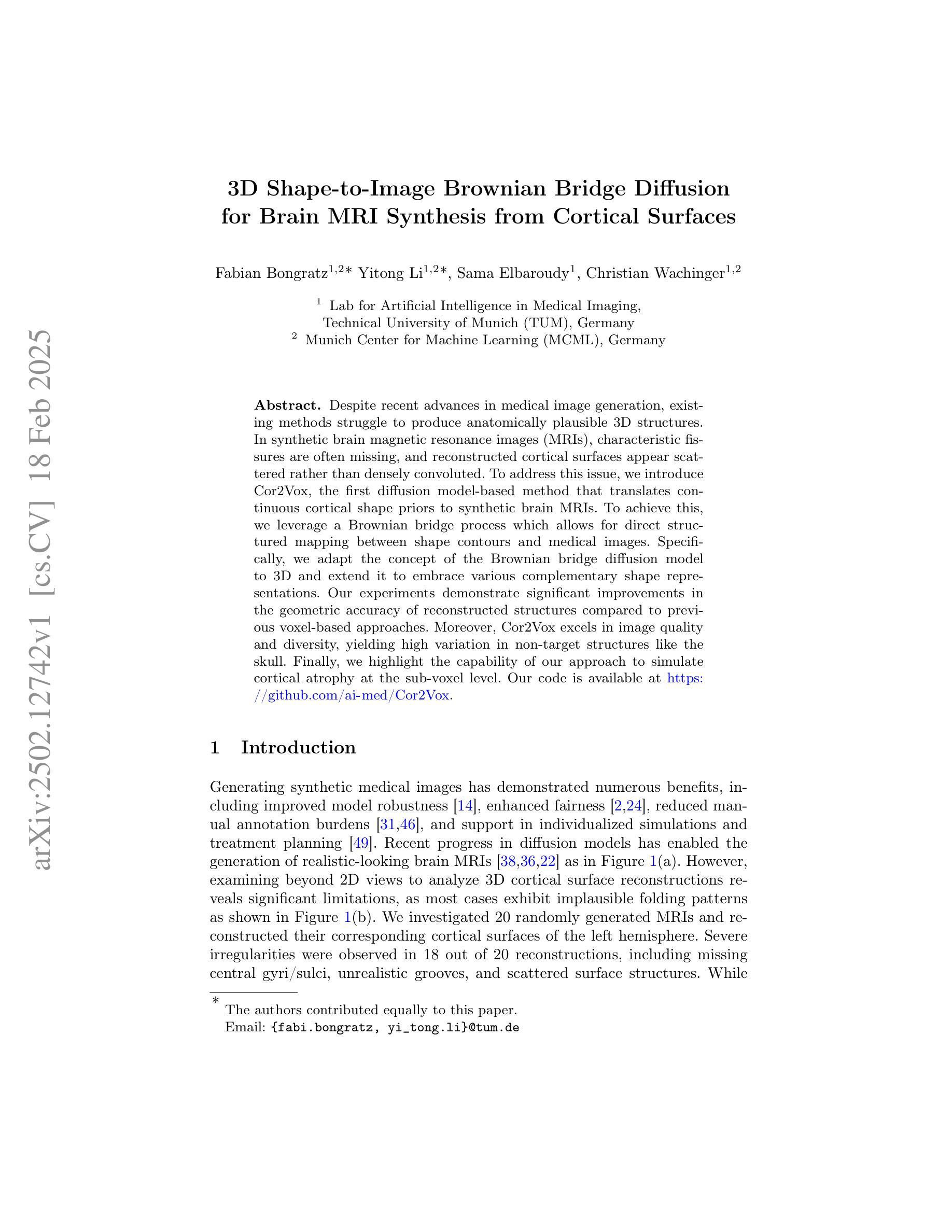
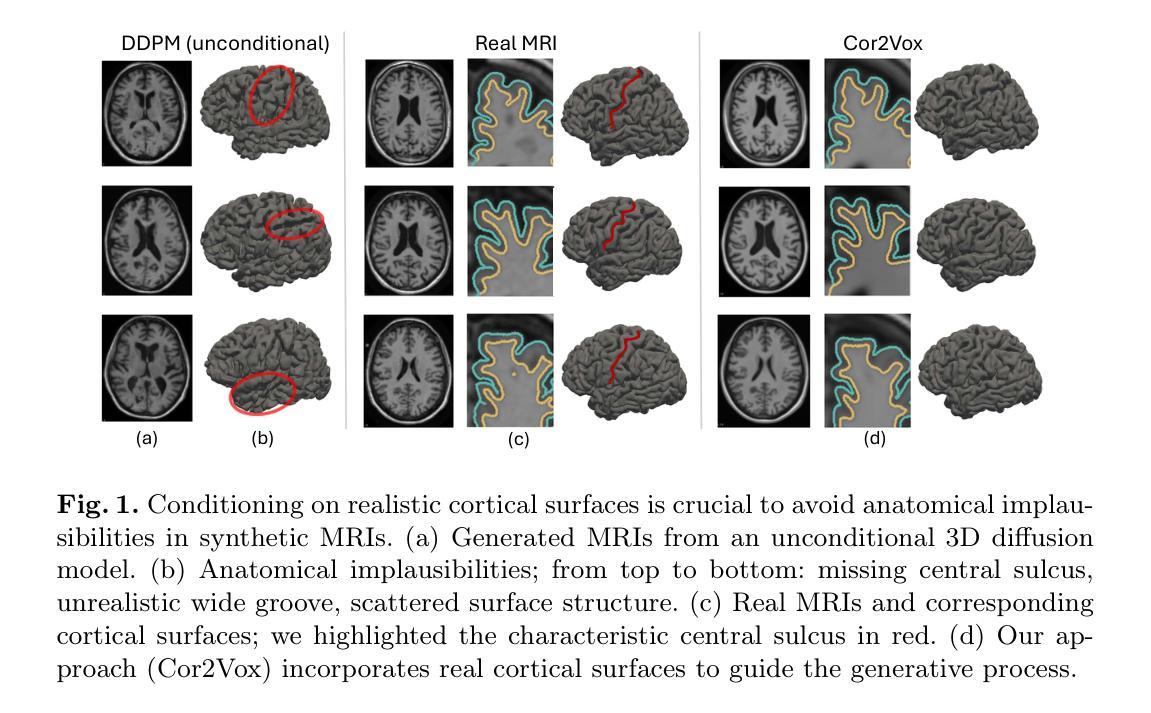
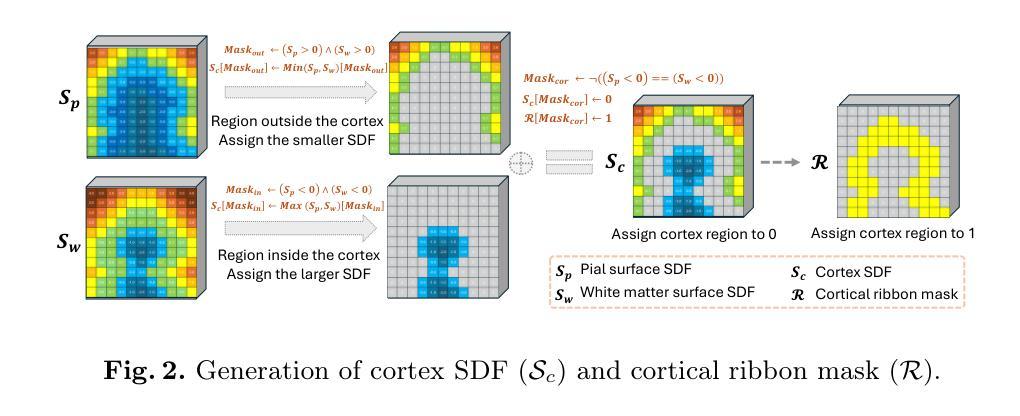
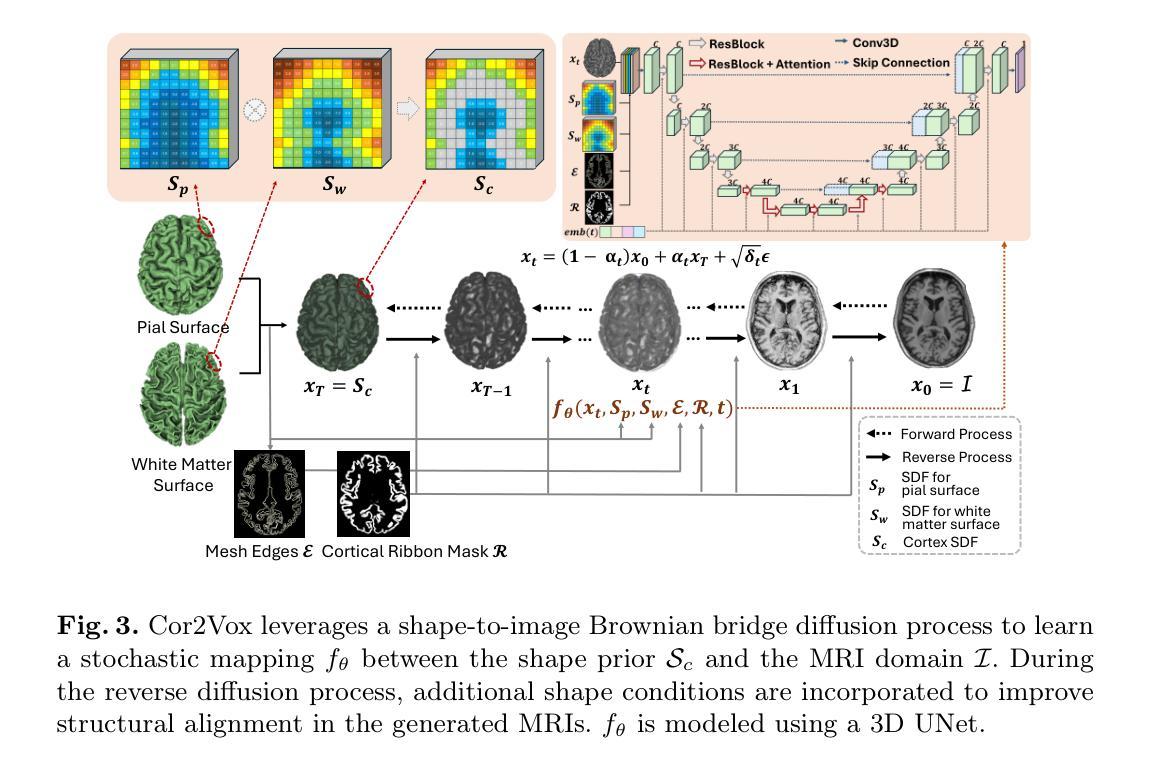
CHATS: Combining Human-Aligned Optimization and Test-Time Sampling for Text-to-Image Generation
Authors:Minghao Fu, Guo-Hua Wang, Liangfu Cao, Qing-Guo Chen, Zhao Xu, Weihua Luo, Kaifu Zhang
Diffusion models have emerged as a dominant approach for text-to-image generation. Key components such as the human preference alignment and classifier-free guidance play a crucial role in ensuring generation quality. However, their independent application in current text-to-image models continues to face significant challenges in achieving strong text-image alignment, high generation quality, and consistency with human aesthetic standards. In this work, we for the first time, explore facilitating the collaboration of human performance alignment and test-time sampling to unlock the potential of text-to-image models. Consequently, we introduce CHATS (Combining Human-Aligned optimization and Test-time Sampling), a novel generative framework that separately models the preferred and dispreferred distributions and employs a proxy-prompt-based sampling strategy to utilize the useful information contained in both distributions. We observe that CHATS exhibits exceptional data efficiency, achieving strong performance with only a small, high-quality funetuning dataset. Extensive experiments demonstrate that CHATS surpasses traditional preference alignment methods, setting new state-of-the-art across various standard benchmarks.
扩散模型已经成为文本到图像生成的主导方法。关键组件,如人类偏好对齐和无分类器引导,在确保生成质量方面发挥着至关重要的作用。然而,它们在当前的文本到图像模型中的独立应用,在实现强大的文本图像对齐、高生成质量和与人类审美标准的一致性方面仍面临重大挑战。在这项工作中,我们首次探索了人类性能对齐和测试时间采样的协作,以解锁文本到图像模型的潜力。因此,我们引入了CHATS(结合人类对齐优化和测试时间采样),这是一种新型生成框架,分别建模首选和不受欢迎的分布,并采用基于代理提示的采样策略,利用两个分布中包含的有用信息。我们发现CHATS表现出卓越的数据效率,仅使用一个小而高质量微调数据集就能实现强劲表现。大量实验表明,CHATS超越了传统偏好对齐方法,在多种标准基准测试中达到了新的技术水准。
论文及项目相关链接
Summary
文本描述了Diffusion模型在文本到图像生成中的主导地位,并指出了人类偏好对齐和分类器自由指导等关键组件的重要性。然而,当前文本到图像模型独立应用时,仍面临实现强文本图像对齐、高质量生成和符合人类审美标准的一致性的挑战。本文首次探索了人类性能对齐和测试时间采样的协作潜力,并引入了CHATS(结合人类对齐优化和测试时间采样)这一新型生成框架。该框架能够分别建模首选和不受欢迎的分布,并采用基于代理提示的采样策略,利用这两种分布中包含的有用信息。实验表明,CHATS表现出卓越的数据效率,在小型高质量微调数据集上实现了强大的性能,并超越了传统的偏好对齐方法,在各种标准基准测试中均达到最新水平。
Key Takeaways
- Diffusion模型已成为文本到图像生成的主要方法。
- 人类偏好对齐和分类器自由指导等组件在保障生成质量方面扮演关键角色。
- 当前文本到图像模型仍面临文本图像对齐、高质量生成和符合人类审美标准的一致性的挑战。
- CHATS框架首次探索了人类性能对齐和测试时间采样的协作潜力。
- CHATS框架能够分别建模首选和不受欢迎的分布,并采用基于代理提示的采样策略。
- CHATS展现出卓越的数据效率,在小型高质量微调数据集上实现了强大性能。
点此查看论文截图
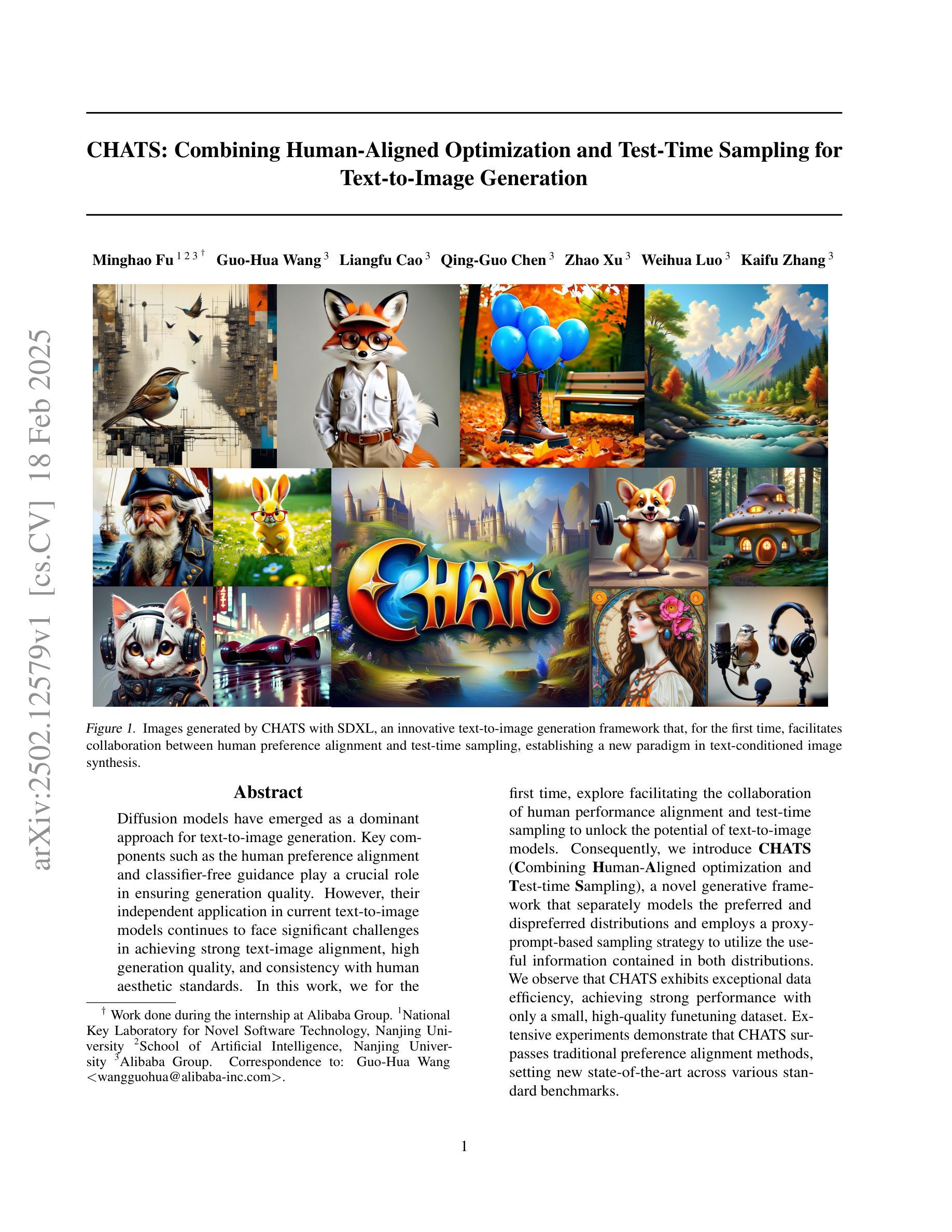
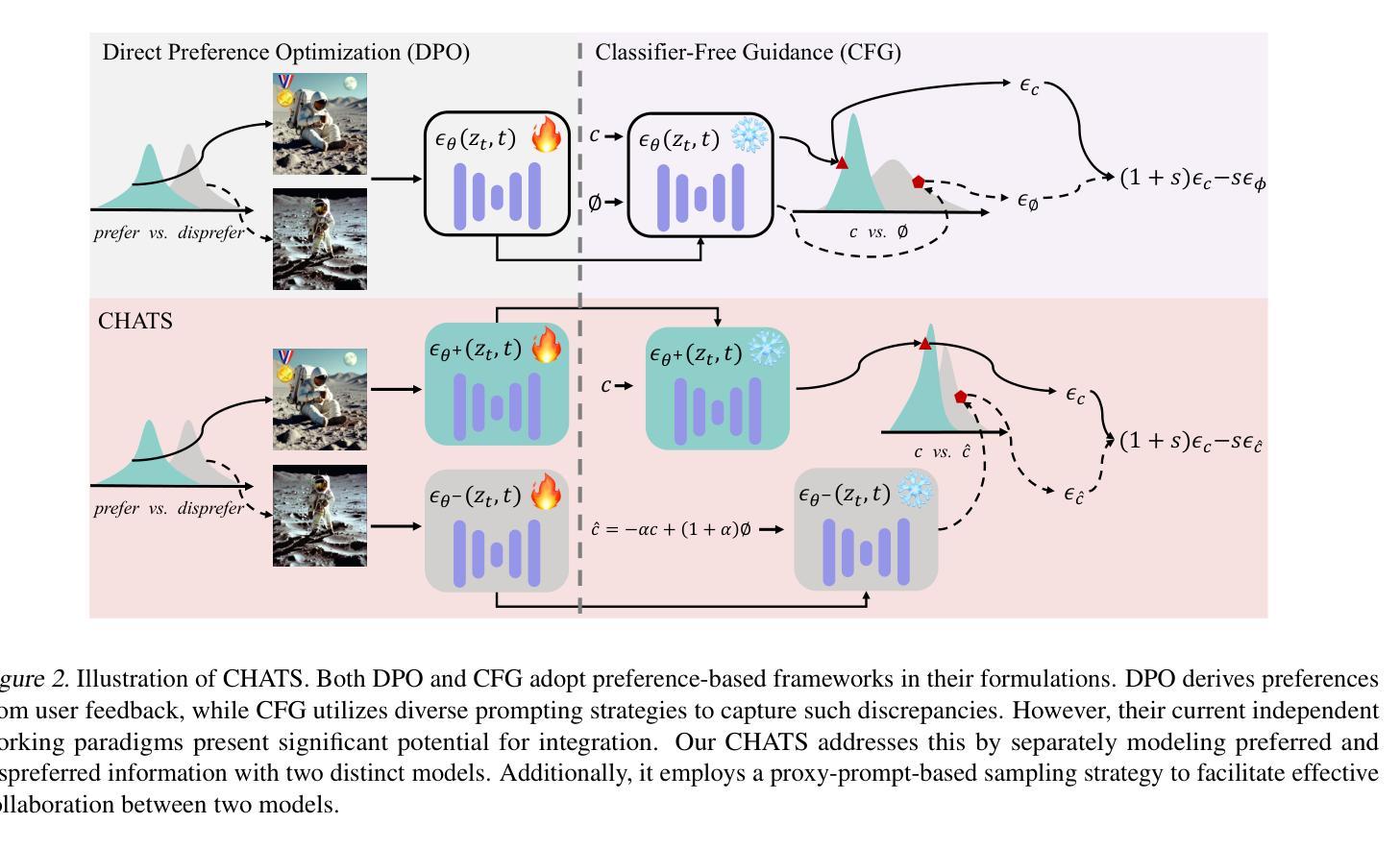
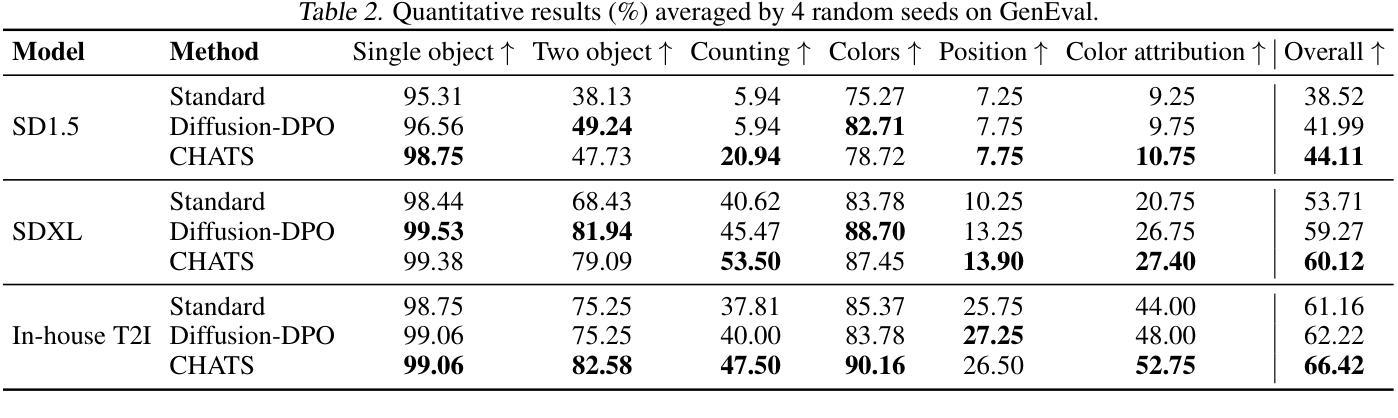
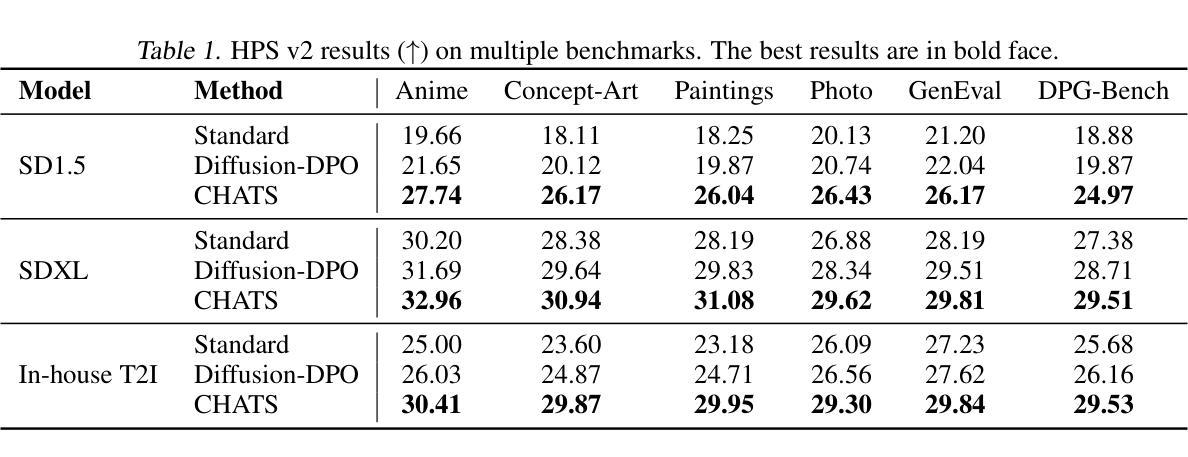
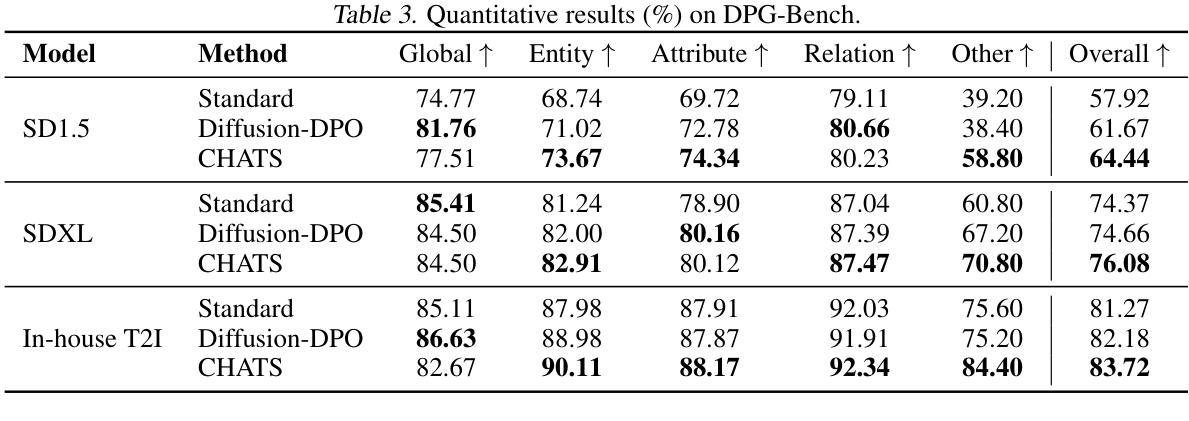
DeltaDiff: A Residual-Guided Diffusion Model for Enhanced Image Super-Resolution
Authors:Chao Yang, Yong Fan, Cheng Lu, Zhijing Yang
Recently, the application of diffusion models in super-resolution tasks has become a popular research direction. Existing work is focused on fully migrating diffusion models to SR tasks. The diffusion model is proposed in the field of image generation, so in order to make the generated results diverse, the diffusion model combines random Gaussian noise and distributed sampling to increase the randomness of the model. However, the essence of super-resolution tasks requires the model to generate high-resolution images with fidelity. Excessive addition of random factors can result in the model generating detailed information that does not belong to the HR image. To address this issue, we propose a new diffusion model called Deltadiff, which uses only residuals between images for diffusion, making the entire diffusion process more stable. The experimental results show that our method surpasses state-of-the-art models and generates results with better fidelity. Our code and model are publicly available at https://github.com/continueyang/DeltaDiff
最近,扩散模型在超分辨率任务中的应用已成为热门的研究方向。现有工作主要集中在将扩散模型完全迁移到SR任务上。扩散模型是应图像生成领域的需求而提出的,因此为了产生多样化的结果,扩散模型结合了随机高斯噪声和分布式采样来增加模型的随机性。然而,超分辨率任务的核心要求模型生成具有保真度的高分辨率图像。过多地增加随机因素可能会导致模型生成不属于高分辨率图像的详细信息。为解决这一问题,我们提出了一种新的扩散模型,称为Deltadiff,它仅使用图像之间的残差进行扩散,使整个扩散过程更加稳定。实验结果表明,我们的方法超越了最先进的模型,生成的结果具有更好的保真度。我们的代码和模型在https://github.com/continueyang/DeltaDiff公开可用。
论文及项目相关链接
Summary
近期,扩散模型在超分辨率任务中的应用成为热门研究方向。针对如何在超分辨率任务中应用扩散模型使其生成的图像结果既具备多样性又具有保真度的问题,提出了一种名为Deltadiff的新扩散模型。该模型仅使用图像之间的残差进行扩散,使整个扩散过程更加稳定,生成结果超越了现有先进模型并提高了保真度。模型和代码已公开在GitHub上提供。
Key Takeaways
- 扩散模型在超分辨率任务中的应用是当前热门研究方向。
- 现有工作侧重于将扩散模型完全迁移到超分辨率任务上。
- 扩散模型结合了随机高斯噪声和分布式采样以增加模型的随机性,用于生成多样性的图像结果。
- 超分辨率任务要求模型生成具有保真度的高分辨率图像。
- 过量添加随机因素可能导致模型生成不属于高分辨率图像的详细信息。
- 提出了一种名为Deltadiff的新扩散模型,仅使用图像间的残差进行扩散,使扩散过程更加稳定。
点此查看论文截图
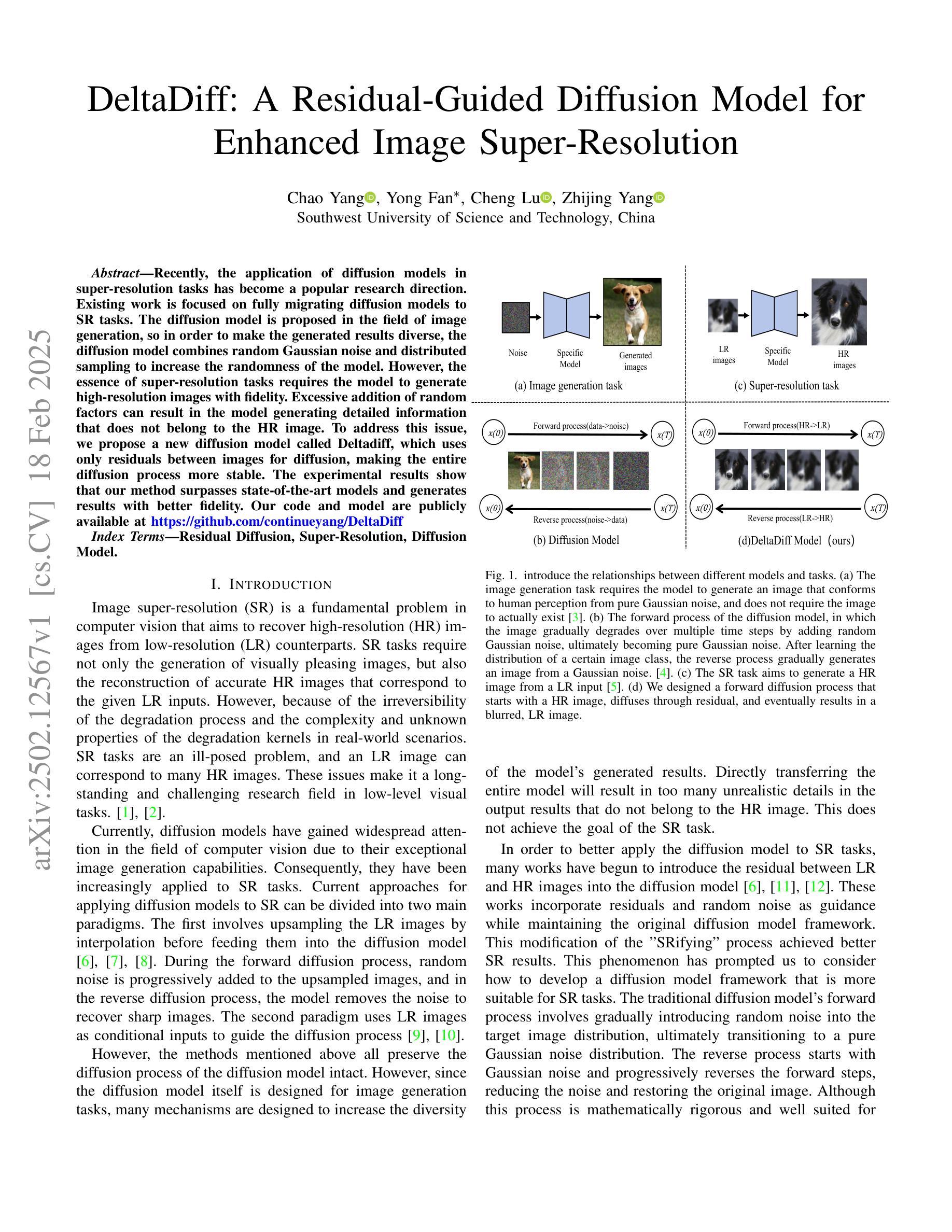
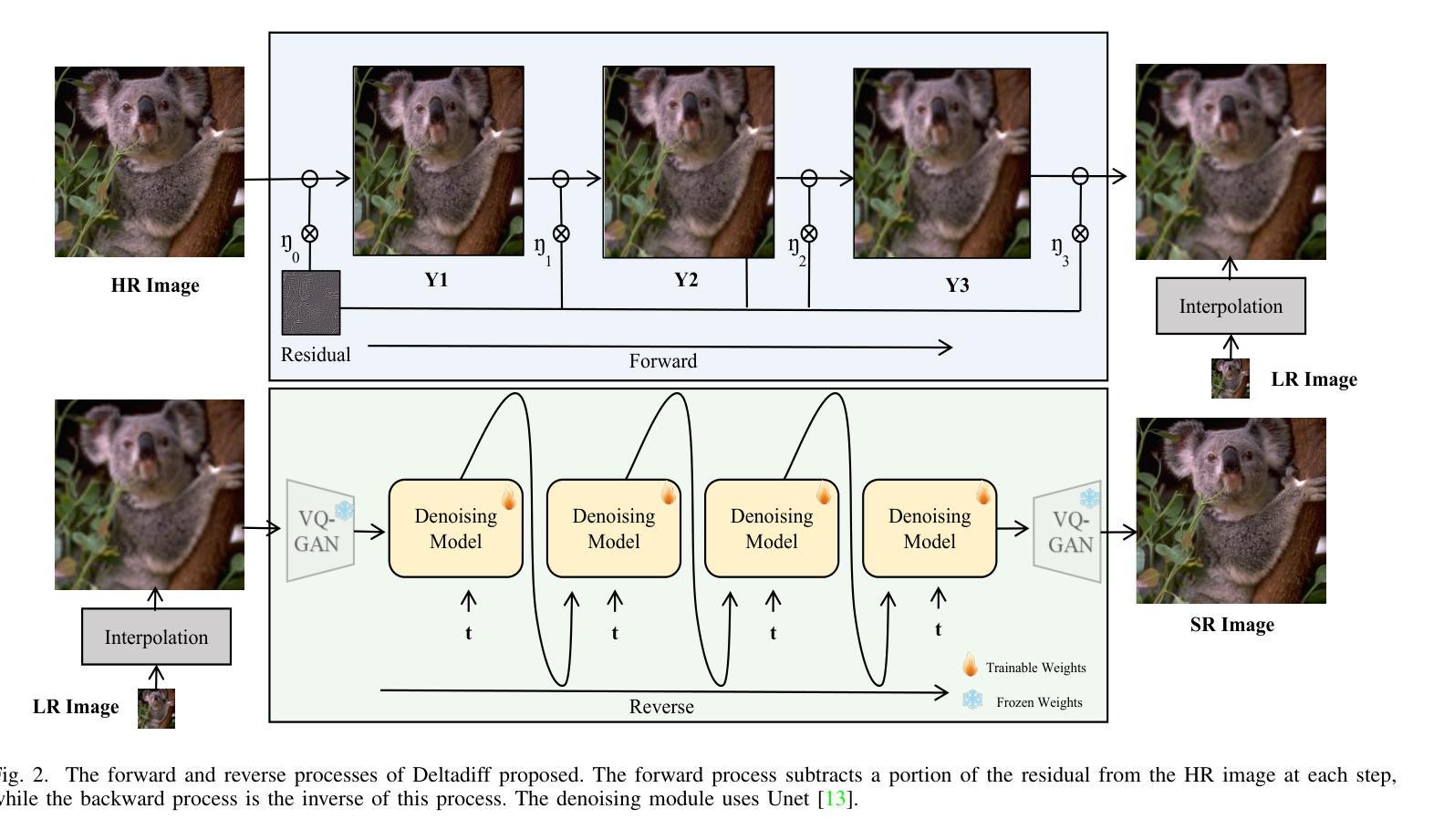
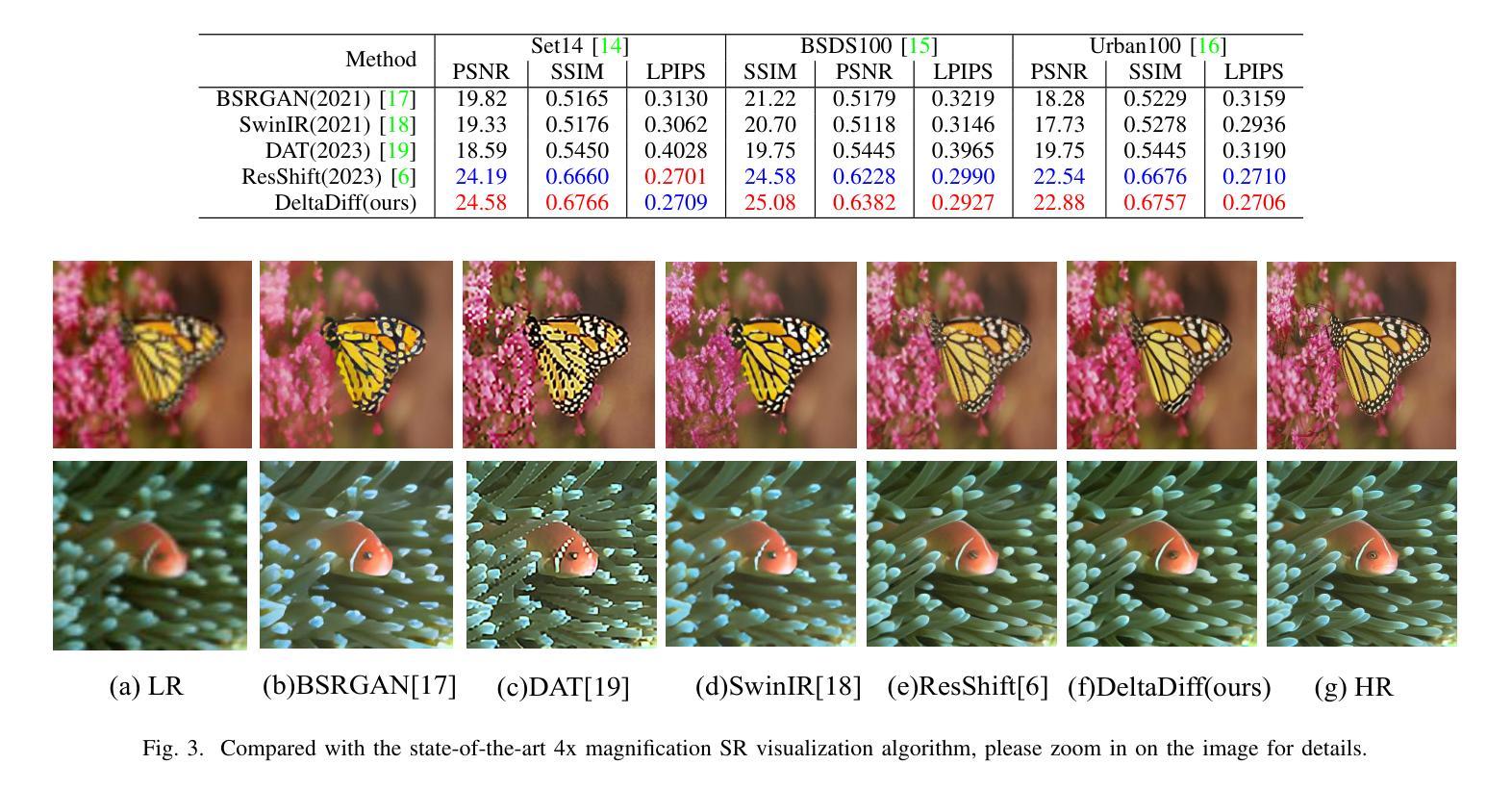
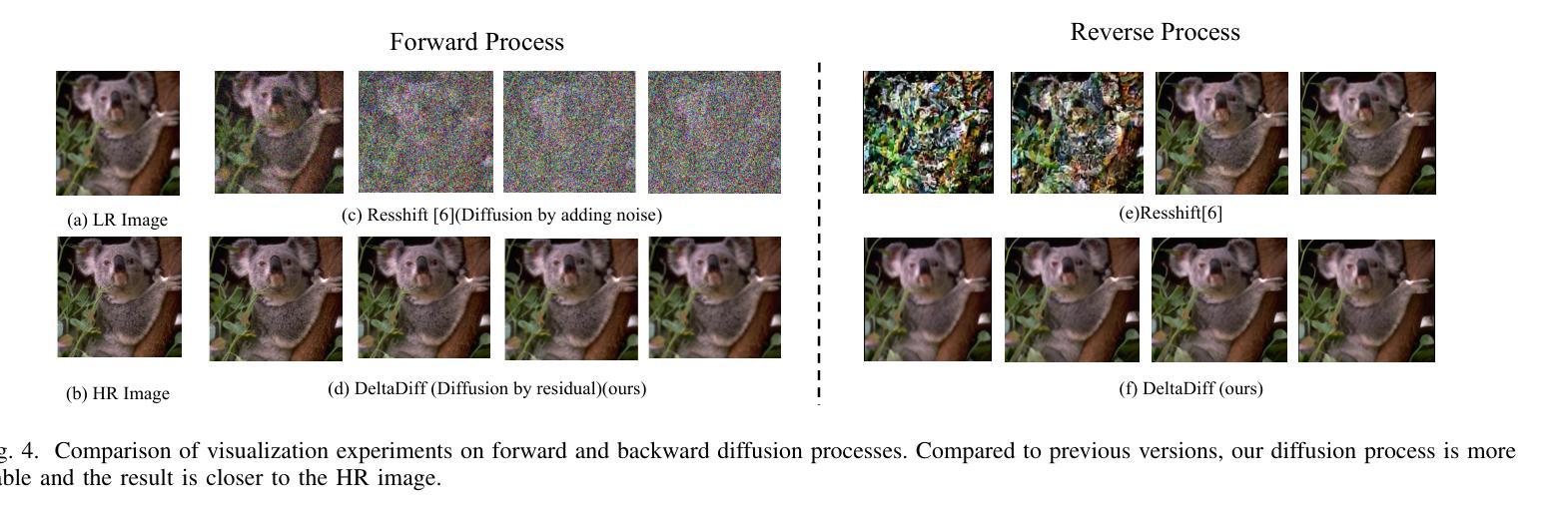
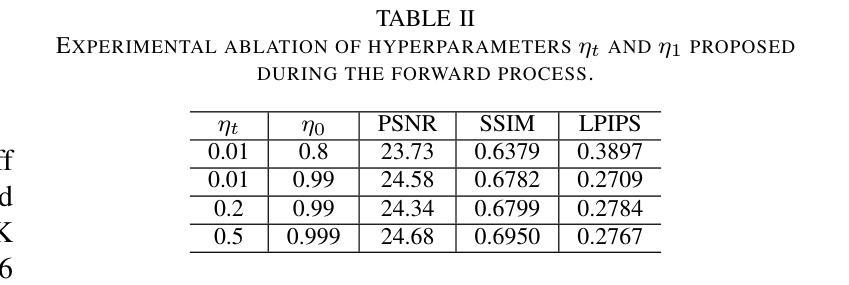
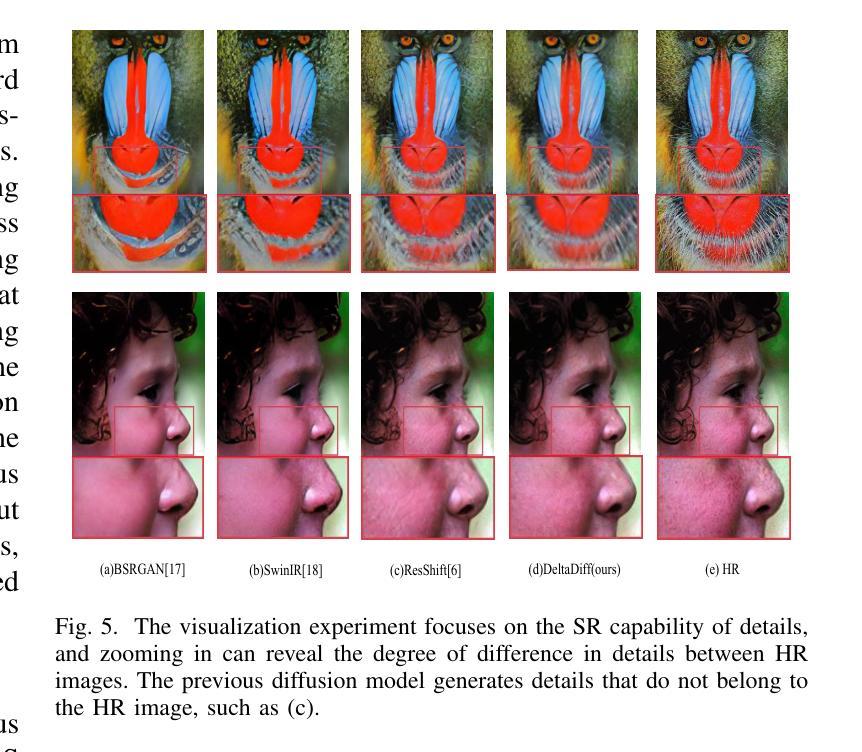
Comprehensive Assessment and Analysis for NSFW Content Erasure in Text-to-Image Diffusion Models
Authors:Die Chen, Zhiwen Li, Cen Chen, Xiaodan Li, Jinyan Ye
Text-to-image (T2I) diffusion models have gained widespread application across various domains, demonstrating remarkable creative potential. However, the strong generalization capabilities of these models can inadvertently led they to generate NSFW content even with efforts on filtering NSFW content from the training dataset, posing risks to their safe deployment. While several concept erasure methods have been proposed to mitigate this issue, a comprehensive evaluation of their effectiveness remains absent. To bridge this gap, we present the first systematic investigation of concept erasure methods for NSFW content and its sub-themes in text-to-image diffusion models. At the task level, we provide a holistic evaluation of 11 state-of-the-art baseline methods with 14 variants. Specifically, we analyze these methods from six distinct assessment perspectives, including three conventional perspectives, i.e., erasure proportion, image quality, and semantic alignment, and three new perspectives, i.e., excessive erasure, the impact of explicit and implicit unsafe prompts, and robustness. At the tool level, we perform a detailed toxicity analysis of NSFW datasets and compare the performance of different NSFW classifiers, offering deeper insights into their performance alongside a compilation of comprehensive evaluation metrics. Our benchmark not only systematically evaluates concept erasure methods, but also delves into the underlying factors influencing their performance at the insight level. By synthesizing insights from various evaluation perspectives, we provide a deeper understanding of the challenges and opportunities in the field, offering actionable guidance and inspiration for advancing research and practical applications in concept erasure.
文本到图像(T2I)扩散模型已广泛应用于各个领域,显示出显著的创新潜力。然而,这些模型具有强大的泛化能力,即使从训练数据集中过滤掉不适当的内容,它们也可能无意中生成不安全的成人内容(NSFW),从而对安全部署构成风险。虽然已经提出了几种概念删除方法来缓解这个问题,但对它们的有效性进行全面评估仍然缺失。为了填补这一空白,我们对文本到图像扩散模型中不安全的成人内容(NSFW)及其子主题的概念删除方法进行了首次系统研究。在任务层面,我们对11种最新基线方法和14种变体进行了全面评估。具体来说,我们从六个不同的评估角度分析了这些方法,包括三个传统角度,即删除比例、图像质量和语义对齐,以及三个新角度,即过度删除、明确和隐含的不安全提示的影响和鲁棒性。在工具层面,我们对NSFW数据集进行了详细的毒性分析,并比较了不同NSFW分类器的性能,提供了关于它们性能的更深入见解,并汇编了全面的评估指标。我们的基准测试不仅系统地评估了概念删除方法,还深入研究了影响它们性能的关键因素。通过从各种评估角度综合见解,我们提供了该领域挑战和机遇的深入了解,为推进概念删除的研究和实际应用提供了可行的指导和灵感。
论文及项目相关链接
摘要
文本到图像(T2I)扩散模型在各个领域都有广泛应用,显示出惊人的创造力。然而,这些模型的强大泛化能力可能会无意中生成不适宜公开场合(NSFW)的内容,即使在努力从训练数据集中过滤这些内容时也是如此,这对模型的安全部署构成了风险。为了解决这一难题,本文首次系统地研究了概念消除方法在文本到图像扩散模型中用于处理不适宜公开场合内容的效率及其子主题。在任务层面,我们对最新的基于扩散模型的文本图像合成技术进行了全面的评估对比,挑选了其中最具代表性的十一类基线方法及其十四个变种。我们从六个不同的评估角度对这些方法进行了详细的分析,包括三个传统角度:消除比例、图像质量和语义对齐;以及三个新的角度:过度消除、显性不安全提示和隐性不安全提示的影响、以及鲁棒性。在工具层面,我们对不适宜公开场合的数据集进行了详细的毒性分析,并比较了不同不适宜公开场合分类器的性能。我们的基准测试不仅系统地评估了概念消除方法,还深入探讨了影响它们性能的因素,综合各种评估角度的见解,为推进概念消除领域的进一步研究提供了深刻见解和实际应用的灵感和方向。我们的研究强调了未来的挑战和机遇。旨在推进该领域的发展并为实际应用提供指导。
关键见解
- 文本到图像(T2I)扩散模型具有在各种领域广泛应用的能力并展现出显著的创新潜力。然而,他们有时会生成不适宜公开场合(NSFW)的内容。
- 尽管有概念消除方法被提出以解决生成NSFW内容的问题,但对这些方法的有效性进行系统性的评估仍然存在缺口。
- 在任务层面,本研究对最新的基线方法进行了全面的评估,包括从六个不同的评估角度对它们进行了详细的分析。这些角度不仅涵盖了传统的评估标准,还包括新的评估视角如过度消除和对于不同提示类型的反应等。
- 在工具层面,研究涉及不适宜公开场合数据集的毒性分析以及不同分类器的性能比较,以深入理解概念消除方法的实际性能并定义更全面的评估指标。
- 我们的研究深入探讨了影响概念消除方法性能的因素,并提供了一个视角来洞察该领域的挑战和机遇。这不仅有助于推进研究进步,也为实际应用提供了方向。
- 研究结果表明对扩散模型在生成过程中的细节进行优化是提高其性能和避免生成不适宜内容的关键所在。通过对这些模型的深入了解和改进将有助于开发更先进且更安全的生成技术。
点此查看论文截图
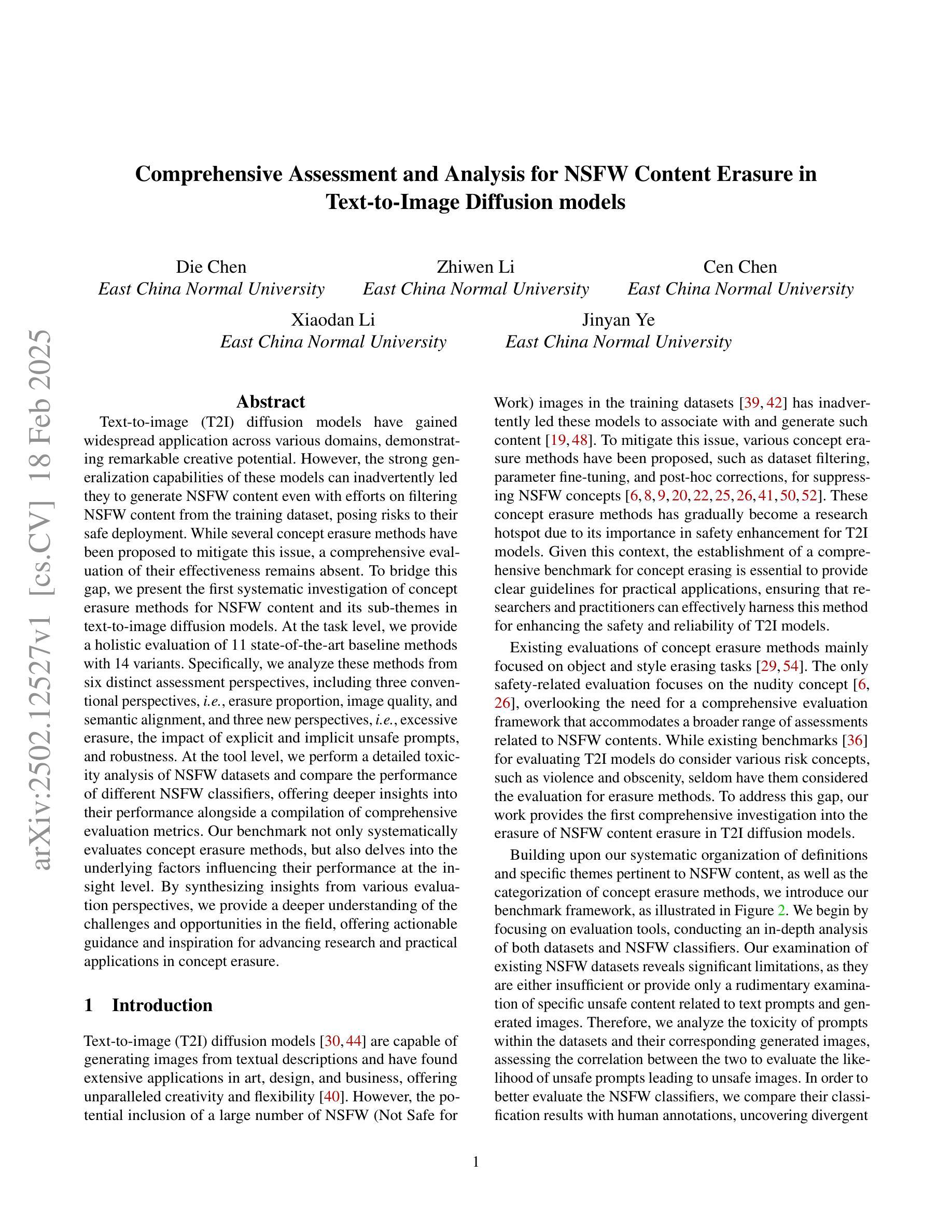
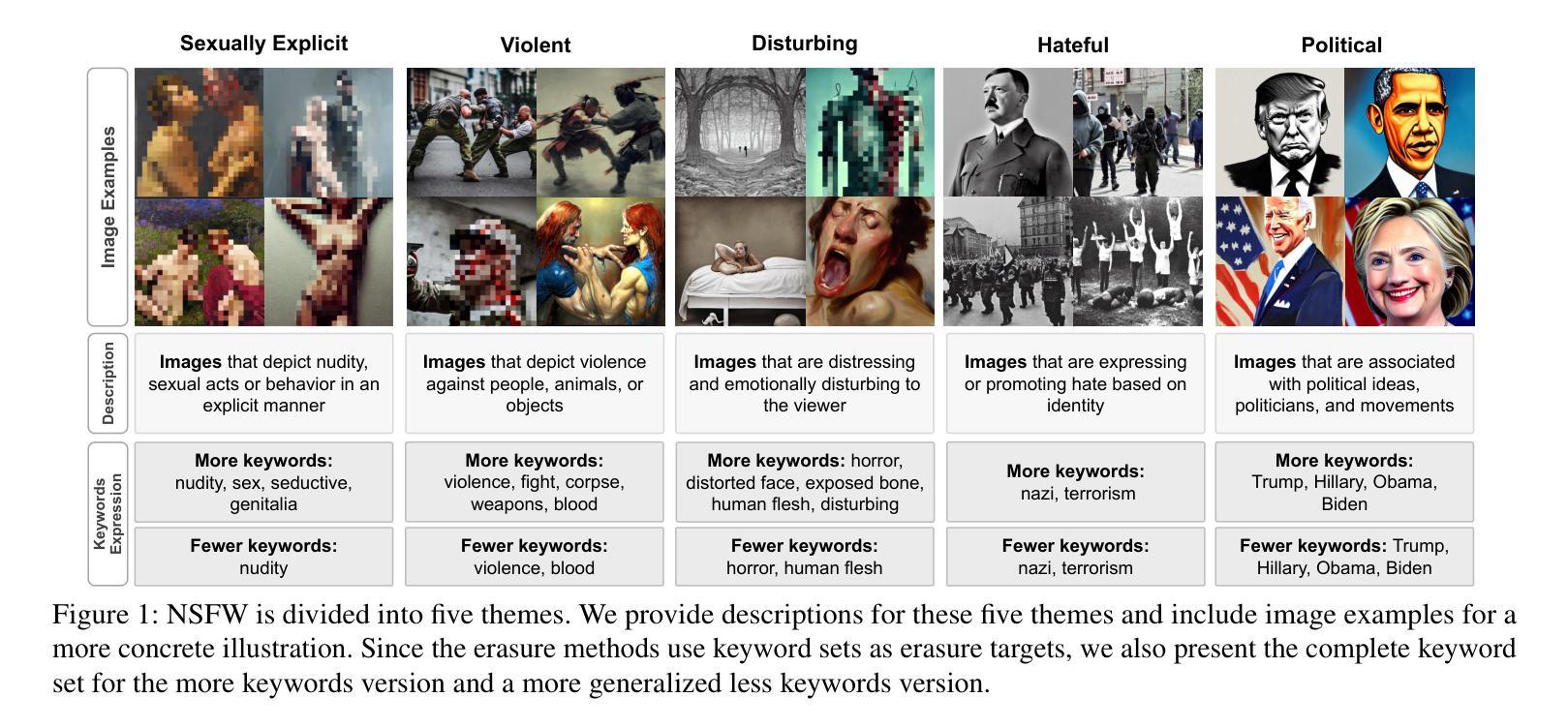

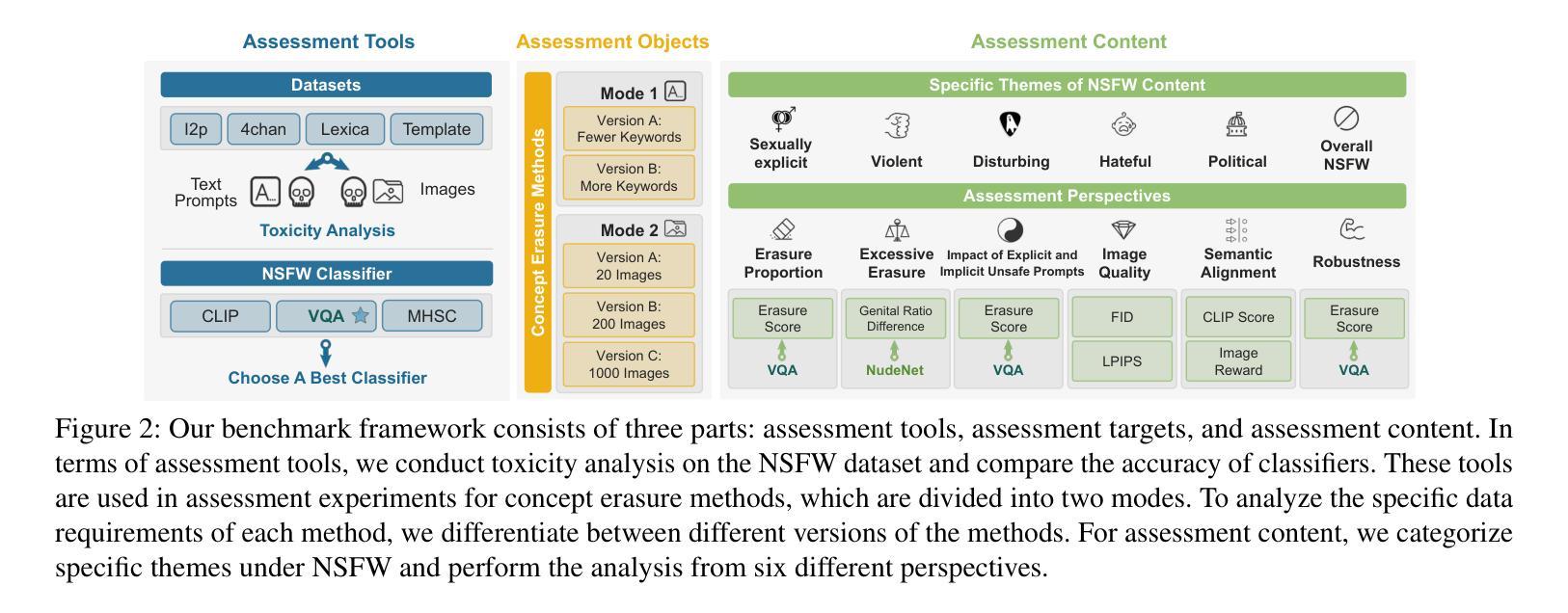
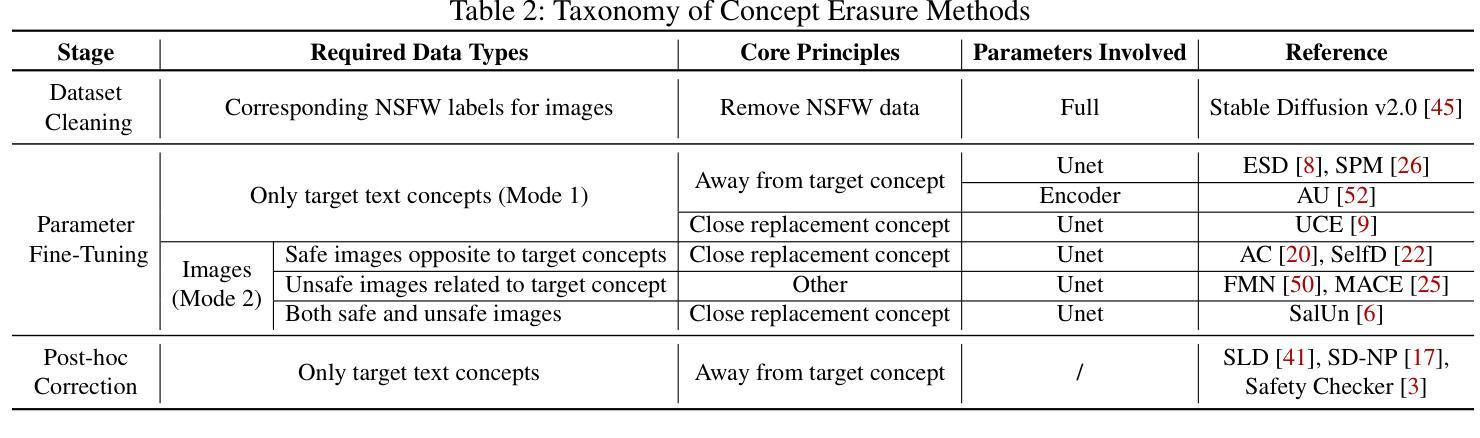
SynthVLM: High-Efficiency and High-Quality Synthetic Data for Vision Language Models
Authors:Zheng Liu, Hao Liang, Bozhou Li, Tianyi Bai, Wentao Xiong, Chong Chen, Conghui He, Wentao Zhang, Bin Cui
Vision-Language Models (VLMs) have recently emerged, demonstrating remarkable vision-understanding capabilities. However, training these models requires large-scale datasets, which brings challenges related to efficiency, effectiveness, quality, and privacy of web data. In this paper, we introduce SynthVLM, a novel data synthesis and curation method for generating image-caption pairs. Unlike traditional methods, where captions are generated from images, SynthVLM utilizes advanced diffusion models and high-quality captions to automatically synthesize and select high-resolution images from text descriptions, thereby creating precisely aligned image-text pairs. To demonstrate the power of SynthVLM, we introduce SynthVLM-100K, a high-quality dataset consisting of 100,000 curated and synthesized image-caption pairs. In both model and human evaluations, SynthVLM-100K outperforms traditional real-world datasets. Leveraging this dataset, we develop a new family of multimodal large language models (MLLMs), SynthVLM-7B and SynthVLM-13B, which achieve state-of-the-art (SOTA) performance on various vision question-answering (VQA) tasks. Notably, our models outperform LLaVA across most metrics with only 18% pretrain data. Furthermore, SynthVLM-7B and SynthVLM-13B attain SOTA performance on the MMLU benchmark, demonstrating that the high-quality SynthVLM-100K dataset preserves language abilities. To facilitate future research, our dataset and the complete data generating and curating methods are open-sourced at https://github.com/starriver030515/SynthVLM.
视觉语言模型(VLMs)最近崭露头角,展现出卓越的视觉理解能力。然而,训练这些模型需要大规模数据集,这带来了与网页数据效率、有效性、质量和隐私相关的挑战。在本文中,我们介绍了SynthVLM,一种用于生成图像-字幕对的新型数据合成和筛选方法。与传统的从图像生成字幕的方法不同,SynthVLM利用先进的扩散模型和高质量字幕,自动根据文本描述合成和筛选高分辨率图像,从而创建精确对齐的图像-文本对。为了展示SynthVLM的威力,我们推出了SynthVLM-100K,这是一个高质量的数据集,包含10万个经过筛选和合成的图像-字幕对。在模型和人类评估中,SynthVLM-100K的表现都优于传统的现实世界数据集。利用这个数据集,我们开发了一系列新的多模态大型语言模型(MLLMs),包括SynthVLM-7B和SynthVLM-13B。它们在各种视觉问答(VQA)任务上达到了最新技术水平。值得注意的是,我们的模型在大多数指标上都优于LLaVA,而仅使用了18%的预训练数据。此外,SynthVLM-7B和SynthVLM-13B在MMLU基准测试中达到了最新技术水平,证明了高质量SynthVLM-100K数据集保留了语言功能。为了促进未来的研究,我们的数据集以及完整的数据生成和筛选方法已在https://github.com/starriver030515/SynthVLM上开源。
论文及项目相关链接
Summary
在大规模数据集训练下的视觉语言模型(VLMs)展现出了卓越的视觉理解能力。但数据集质量及其带来的效率问题却逐渐暴露出来。本研究提出了一种新型数据合成与筛选方法SynthVLM,该方法结合先进的扩散模型和高质量文本描述,自动生成精确对齐的图像文本对。基于该方法构建的SynthVLM-100K数据集在模型和人类评估中都超越了传统真实世界数据集。利用该数据集训练的SynthVLM系列多模态大型语言模型(MLLMs)在视觉问答任务上达到最新性能水平。同时,数据集和完整的数据生成与筛选方法已开源共享。
Key Takeaways
- Vision-Language Models (VLMs)展现出强大的视觉理解能力,但大规模数据集训练面临挑战。
- SynthVLM是一种新型数据合成与筛选方法,能生成精确对齐的图像文本对。
- SynthVLM利用扩散模型和高质量文本描述进行图像合成。
- SynthVLM构建的SynthVLM-100K数据集在模型与人类评估中表现优越于传统数据集。
- 利用SynthVLM-100K数据集训练的SynthVLM系列多模态大型语言模型(MLLLMs)在视觉问答任务上达到最新性能水平。
- SynthVLM系列模型在多个评估指标上超越了LLaVA模型,仅使用较少的预训练数据。
点此查看论文截图
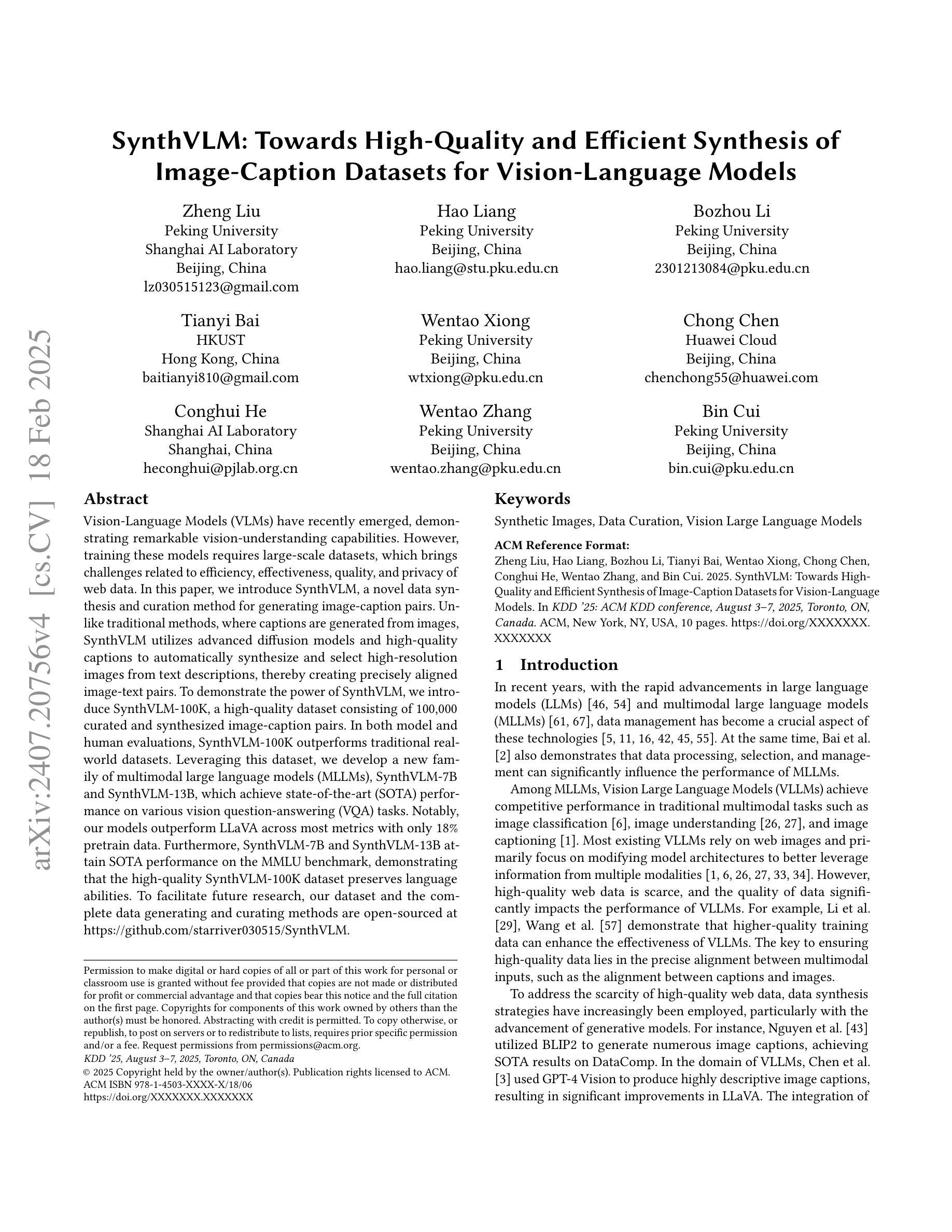
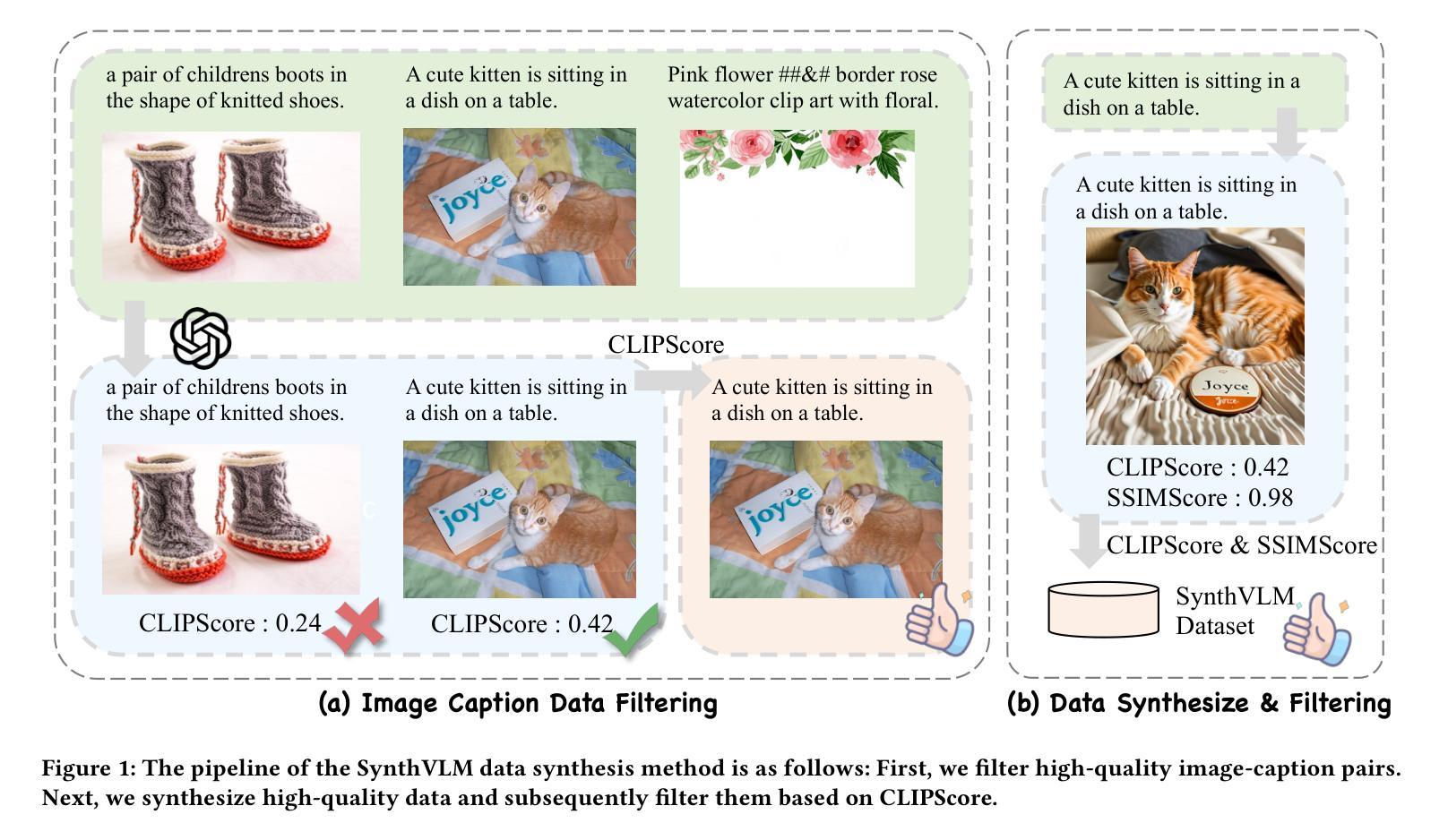
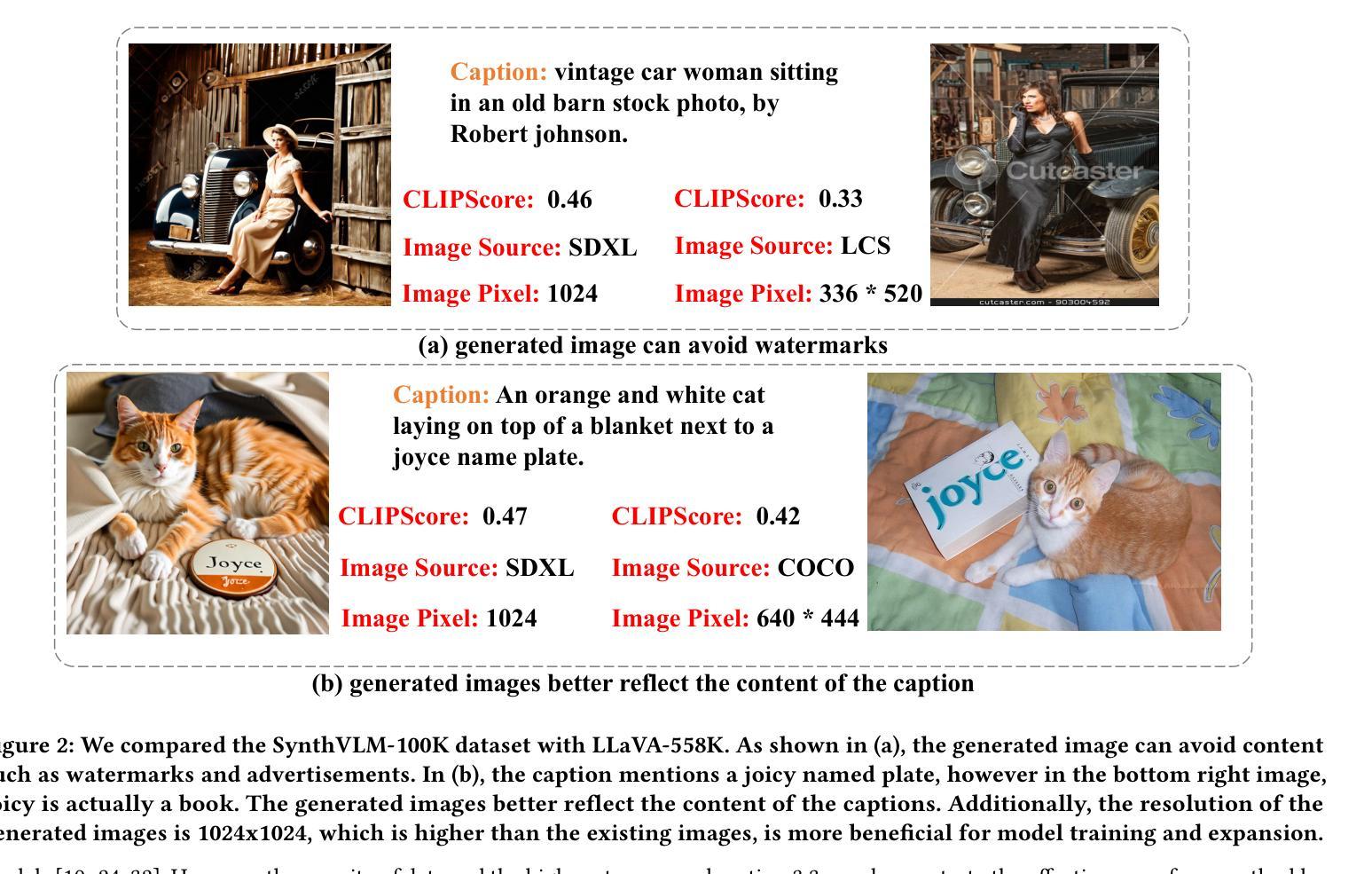
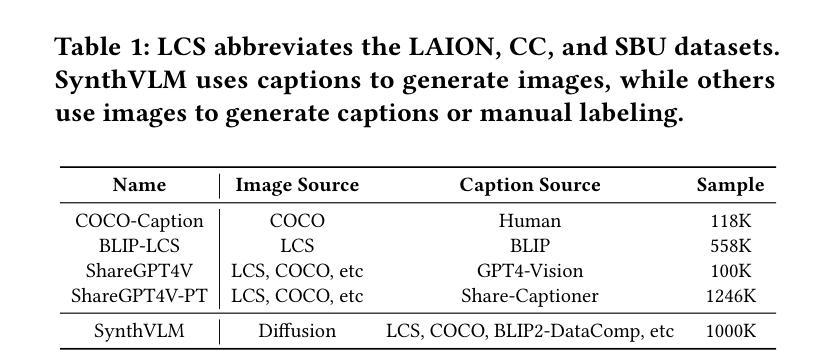
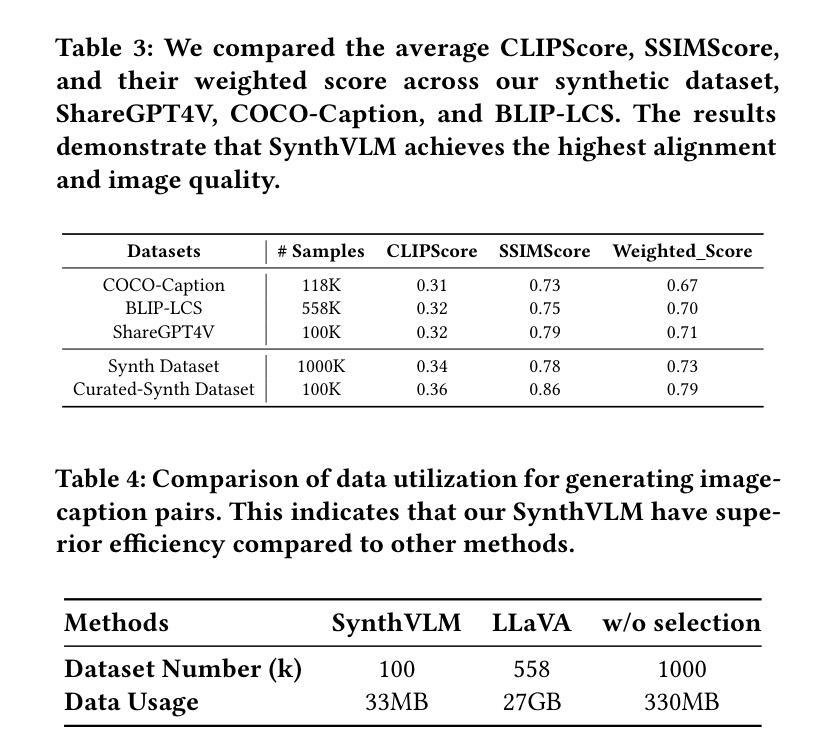
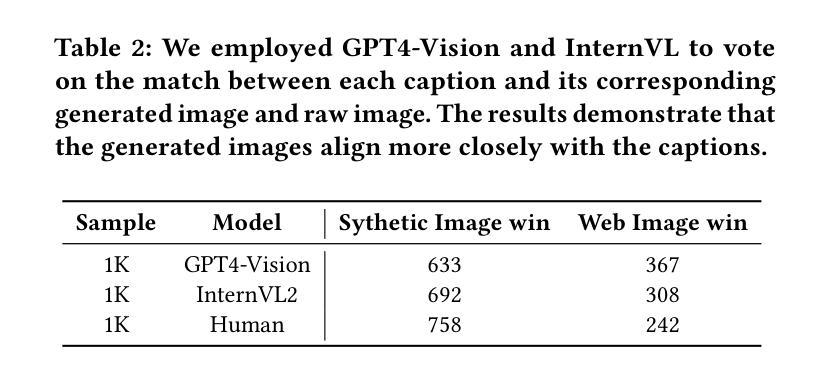
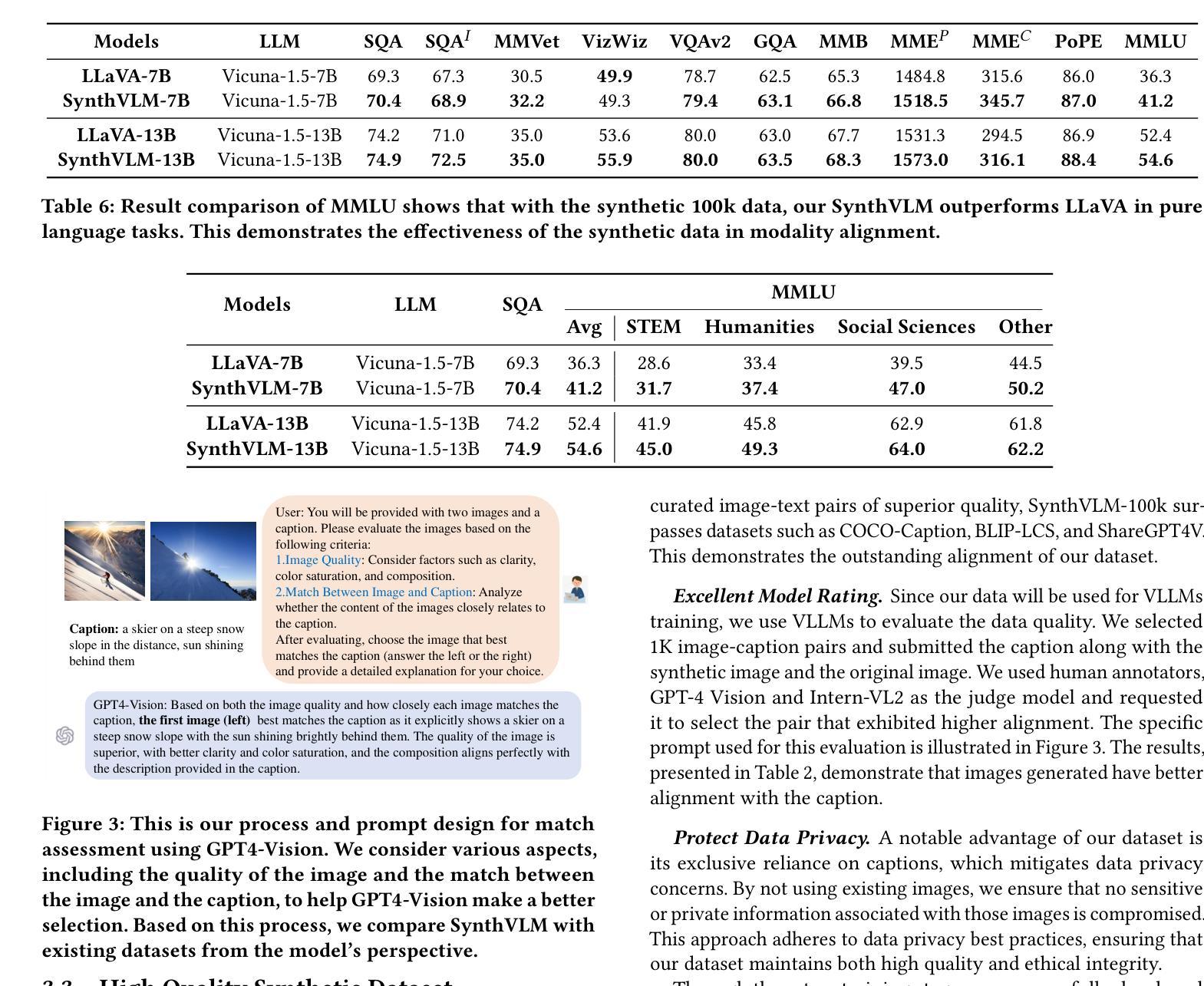